- 1Department of Biology, Barnard College, New York, NY, United States
- 2Department of Ecology, Evolution, and Environmental Biology, Columbia University, New York, NY, United States
- 3Data Science Institute, Columbia University, New York, NY, United States
- 4Ruy V. Lourenço Center for Emerging and Re-emerging Pathogens, Rutgers New Jersey Medical School, Newark, NJ, United States
- 5Department of Microbiology, Biochemistry and Molecular Genetics, Rutgers New Jersey Medical School, Newark, NJ, United States
Nucleotide metabolism plays a central role in bacterial physiology, producing the nucleic acids necessary for DNA replication and RNA transcription. Recent studies demonstrate that nucleotide metabolism also proactively contributes to antibiotic-induced lethality in bacterial pathogens and that disruptions to nucleotide metabolism contributes to antibiotic treatment failure in the clinic. As antimicrobial resistance continues to grow unchecked, new approaches are needed to study the molecular mechanisms responsible for antibiotic efficacy. Here we review emerging technologies poised to transform understanding into why antibiotics may fail in the clinic. We discuss how these technologies led to the discovery that nucleotide metabolism regulates antibiotic drug responses and why these are relevant to human infections. We highlight opportunities for how studies into nucleotide metabolism may enhance understanding of antibiotic failure mechanisms.
Introduction
In the nearly 100 years since the discovery of penicillin, antibiotics have revolutionized medical practice and have become a cornerstone of modern medicine. However, growing rates of antimicrobial resistance pose an urgent and looming threat to public health and economic stability (1). These are compounded by a diminished antimicrobial discovery pipeline (2), creating a critical need to understand mechanisms responsible for antibiotic treatment failures and to discover new effective antimicrobials.
Clinical microbiology traditionally relies on general microbiology and molecular biology laboratory techniques, such as polymerase chain reaction and gene deletion/over-expression, to elucidate molecular mechanisms responsible for clinical phenotypes. However, experimental throughput by these methods limits progress toward understanding mechanisms of antibiotic treatment failure. In recent years several new experimental and digital technologies have emerged with promise to increase clinical microbiology laboratory throughput and enhance clinical management of bacterial infections (3–5). Moreover, advances in prokaryotic systems biology (6, 7) and interpretable machine learning (8) are for the first time accelerating discovery of mechanisms underlying antibiotic efficacy (9, 10).
Here, we review emerging digitalization technologies poised to transform research into mechanisms of antibiotic treatment failure in the clinic. We describe several antibiotic resistance, tolerance and persistence mechanisms discovered from clinical strains. We discuss in detail the recent discovery that nucleotide metabolism actively participates in antibiotic lethality and the clinical relevance of these findings (11). We propose new opportunities for digitalization technologies to advance clinical practice and to open frontiers for basic research into nucleotide metabolism and antibiotic efficacy.
Digitalization in Clinical and Research Settings
The most important goal in clinical microbiology is to identify an infectious pathogen and determine its drug susceptibility profile (12). Traditionally, clinical microbiology laboratories rely on culture-based methods for pathogen identification and susceptibility testing. These approaches require the successful isolation and culture of pathogen cells from a clinical sample, followed by in vitro screening with standardized antibiotics.
In vitro studies in research settings have enabled the discovery of antibiotic resistance mechanisms. For example, following the initial detection of clinical tetracycline resistance, several microbiology studies identified decreased drug transport as the mechanism responsible for reduced efficacy (13, 14). Subsequent studies identified multi-drug resistant efflux pumps in multiple pathogenic species (e.g., AcrB in Escherichia coli and MexB in Pseudomonas aeruginosa) (15). As with their clinical counterparts, these fundamental studies rely on culture-based growth and targeted sequencing; however, such experimental technologies are resource- and labor-intensive and do not scale well with the plethora of pathogen variants, drug mechanisms, and resistance strategies found in the clinic.
In recent years, advances in laboratory evolution, high-throughput sequencing, and computational biology have greatly expanded the scope of addressable questions in microbiology and the study of antibiotic resistance (16). For instance, adaptive laboratory evolution can simulate natural selection pressures (17), allowing researchers to study the emergence of novel antibiotic treatment phenotypes (18), as well as their relationship to environmental conditions (19). In many cases, these granular experimental techniques invite complementary computational modeling activities, from mechanistically simulating drug-target binding to predicting complex ecological dynamics, yielding deeper insights into clinical resistance phenomena.
Concurrently, whole-genome sequencing has transformed the study of antibiotic resistance, enabling the identification of all possible gene variants that can give rise to clinical phenotypes (20). Whole-genome sequencing has proven instrumental in revealing population- and epidemiological-level insights into pathogen detection and emergence. For example, the 2011 outbreak of the Shiga-toxin producing enteroaggregative E. coli O104:H4 resulted in over 3,000 infections and more than 50 deaths – rapid, open-access whole-genome sequencing analysis revealed the phylogenetic relationships between this strain and 40 previously published pathogen genomes (21). These analyses conclusively demonstrated that O104:H4's virulence was attributable to the horizontal acquisition of stx2, along with other unexpected traits heretofore unseen in this lineage (22). Indeed, whole-genome sequencing enables insights into a pathogen's plasticity and facilitates real-time epidemiological tracing (23).
Whole-genome sequencing has spurred the development of advanced computational techniques capable of inferring meaningful biological relationships. Advances in mathematical modeling and machine learning are now, for the first time, enabling the direct identification of antibiotic resistance determinants from the genomes of clinical isolates in as Staphylococcus aureus, P. aeruginosa, and E. coli (24). Moreover, mathematical modeling and high-throughput sequencing approaches have revealed that sub-inhibitory selection and step-wise adaptation play just as important a role in antibiotic treatment failure as canonical antibiotic resistance mechanisms (25). Indeed, clinical isolates from patients with relapsed Mycobacterium tuberculosis infection exhibit sub-breakpoint minimum inhibitory concentrations (MICs) in comparison to strains from patients durably cured (26). Mutations responsible for such subtle cellular phenotypes are readily overlooked using previous methods. Additionally, machine learning can complement traditional culture-based methods and enable the direct prediction of pathogen MICs (27, 28) and provide experimentally testable insights into antibiotic mechanisms of action (9).
Antibiotic Treatment Failure Mechanisms in Clinical Pathogens
Antibiotic treatment failure is conventionally understood to be fully explained by antibiotic resistance, in which a pathogen acquires a genetic mutation either to reduce the ability of an antibiotic to inhibit its target or reduce the effective intracellular concentration of an antibiotic (15, 29). Indeed, antibiotic resistance mutations from sequenced clinical isolates frequently appear in either the target of the antibiotic, modifying the ability of an antibiotic to bind, or in the promoter regions of drug efflux pumps, inducing antibiotic export (30). Other antibiotic resistance alleles, such as genes encoding β-lactamases, commonly appear in mobile genetic elements and can become exchanged by horizontal gene transfer (31).
However, in recent years there has been a growing recognition that alternative bacterial phenotypes, such as antibiotic tolerance (in which isogenic bacteria exhibit slower killing by an antibiotic) and antibiotic persistence (in which isogenic bacteria exhibit a shallower antibiotic killing plateau), also lead to treatment failure and relapsed infection (32). Additionally, there is growing appreciation that the local microenvironment of infection can act on several aspects of bacterial physiology to alter antibiotic treatment efficacy (33, 34). In fact, the local metabolic microenvironment of an infection is highly dynamic and local metabolites induced by either infection or antibiotic treatment itself can inhibit a pathogen's cellular response to antibiotic exposure (35).
It is clear that antibiotic-target interactions alone are insufficient for explaining antibiotic treatment failure in human patients. To address these knowledge gaps, interpretable machine learning approaches are being developed, which seek to rapidly generate experimentally testable hypotheses for biological phenomena. In one of the earliest demonstrations of these, a biochemical screen was performed to measure changes in antibiotic efficacy following metabolic stimulation, and genome-scale metabolic modeling simulations were performed to estimate metabolic reaction activities in each screening condition (Figure 1A). By applying machine learning to these data, purine biosynthesis was identified as a prominent player that governs antibiotic efficacy (9), highlighting a target-independent aspect of bacterial physiology is commonly involved in the lethal process of diverse bactericidal antibiotics. In light of the central role that purine metabolites also play in regulating the immune system (36), these results are also suggestive of mechanisms by which the patient-specific metabolic environment of an infection can promote drug tolerance or antibiotic treatment failure.
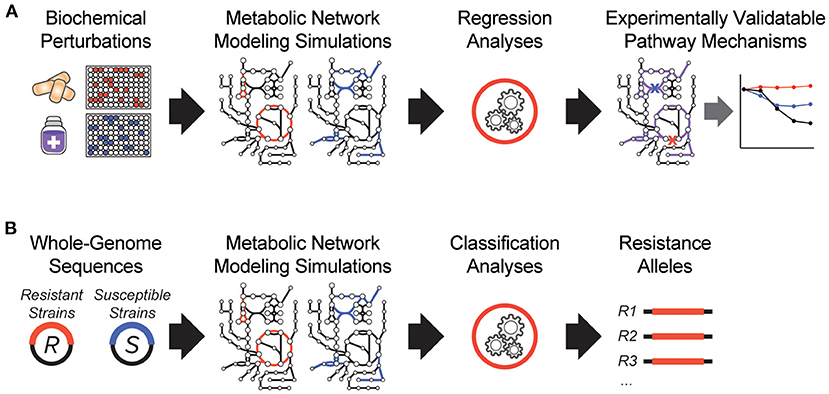
Figure 1. Recent innovations in interpretable machine learning for studying antibiotic treatment failure. (A) A biochemical screen was combined with metabolic network modeling and machine learning regression analyses to elucidate pathway mechanisms of antibiotic lethality. This led to the discovery that purine biosynthesis is a critical component of bactericidal antibiotic lethality (9). (B) Whole-genome sequencing data from antibiotic resistant (R) and susceptible (S) strains from clinical strains were applied as modeling constraints to genome-scale metabolic models. Machine learning classification analyses were applied (10).
In another study, a metabolic model-based machine learning classifier was developed, which uses flux balance analysis to estimate the biochemical effects of genetic mutations characterized from clinical isolates (Figure 1B). Applying this approach to a large collection of genomes from drug-tested M. tuberculosis strains, novel metabolic resistance mechanisms to first-line tuberculosis antibiotics were discovered (10). These two examples illustrate how network models can serve as quantitative knowledgebases (37) and be combined with machine learning analyses to learn molecular mechanisms responsible for antibiotic treatment failures directly from clinical isolates (38).
Nucleotide Metabolism in Antibiotic Treatment Failure
Bacterial metabolism is now understood to be an important physiological regulator of antibiotic efficacy (39). Across living systems, cellular metabolism is governed by the synthesis, allocation, and utilization of energy; and a growing number of studies demonstrate that metabolic dormancy protects cells from antibiotic treatment by inducing a phenotypically tolerant physiological state (29). Moreover, ATP synthesis correlates with the lethality of bactericidal antibiotics better than bacterial growth rates (40), suggesting that antibiotic-induced lethality is an active process and not merely a passive consequence of the loss-of-function of an essential gene product.
In particular, bactericidal antibiotics have been shown to elevate central carbon metabolism activity (41, 42) and trigger the formation of byproduct reactive oxygen species (43, 44), which damage DNA and cause bacterial lethality (45–47). These phenomena are not restricted to antibiotics, as reactive oxygen species also actively contribute to the lethality of bacterial secretase dysfunction (48) and thymine depletion (49). Moreover, defects in central carbon metabolism activity are linked to antibiotic tolerance and persistence across many bacterial species (50–53) and can be stimulated to enhance antibiotic efficacy (54, 55). However, antibiotic treatment perturbs several aspects of bacterial metabolism beyond central carbon metabolism (56), highlighting important knowledge gaps in understanding how different metabolic pathways may contribute to antibiotic treatment failure.
It may come to no surprise that nucleotide metabolism is actively involved in antibiotic efficacy (9). Nucleotides are essential metabolites and are ubiquitous to all living cells; in addition to their roles as fundamental building blocks for DNA and RNA molecules, constituting more than 20% of cellular biomass (57), nucleobases also form the molecular basis of primary energy currencies such as ATP and NADH, and many coenzymes are derived from nucleobase monomers. In fact, the thermodynamic properties of nucleobases are so special, that these metabolites synchronize cell biochemistry and regulate biochemical group transfers across diverse physiological processes (58). Moreover, the concentration of intracellular ATP is tightly regulated across the tree of life and heavily buffered across environmental conditions (59).
De novo nucleotide biosynthesis from carbohydrates begins with the pentose phosphate pathway, which supplies phosphoribosyl pyrophosphate (prpp) as a shared substrate to the purine and pyrimidine biosynthesis pathways (Figure 2). These pathways produce nucleotide triphosphates which can be incorporated into DNA and RNA or processed into energy currencies that can power virtually all other biochemical processes in the cell. Interestingly, nucleotide biosynthesis is itself an energetically demanding process, costing a cell 8 ATP molecules to synthesize one adenine molecule from one glucose molecule. Indeed, cells employ a multitude of strategies to manage these tradeoffs, including prioritized nutrient usage, maintenance metabolism, and nucleotide salvage.
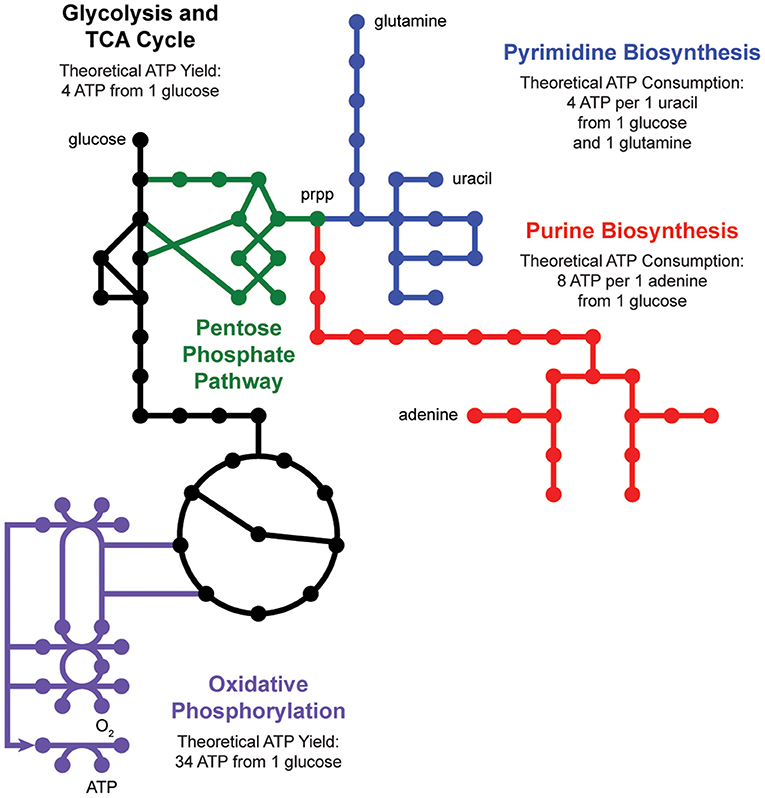
Figure 2. Nucleotide metabolism is energetically expensive. De novo biosynthesis of purines (red) and pyrimidines (blue) begins with the pentose phosphate pathway (green), which generates phosphoribosyl pyrophosphate (prpp) from glycolysis (black). The energetic demand for ATP molecules to power purine and pyrimidine biosynthesis drives activity through the tricarboxylic acid (TCA) cycle (black) and oxidative phosphorylation (purple).
Antibiotic treatment imposes additional layers of complexity on these processes; cells must expend energy to mount defensive stress responses, and many antibiotics preferentially kill metabolically active cells. Specific components of nucleotide metabolism have been shown to contribute to antibiotic efficacy and protection both in vitro and in vivo. In many cases, defects in nucleotide biosynthesis have been shown to induce antibiotic persistence, suggesting these may represent a key metabolic strategy for evading antibiotic efficacy. For example, several chemogenomic screens identify nucleotide biosynthesis genes, as well as global regulators of nucleotide metabolism, as important regulators of antibiotic tolerance (60, 61). Likewise, antibiotic drug screening under nutrient limitation identified several compounds that interfere in core or peripheral nucleotide metabolism branching points (62).
Of note, purine biosynthesis frequently emerges as a key pathway responsible for antibiotic efficacy. For example, in an antibiotic persistence screen using a S. aureus transposon mutant library, 29% of all depleted genes were related to cellular metabolism, and of these, five were involved in purine biosynthesis (63). These ex vivo observations are important for understanding clinical antibiotic treatment failure, as methicillin-resistant S. aureus clones isolated from patients enduring multi-drug antibiotic treatment were found to possess mutations in purR, a transcriptional repressor of purine synthesis, within 1 week of treatment. In vitro follow-up experiments confirmed that this mutation reduced the rate of vancomycin-induced killing, revealing the evolution of antibiotic tolerance in vivo (64). Importantly, this mutation preceded the onset of canonical resistance evolution; these and other studies suggest that mutations in nucleotide metabolism may help create a reservoir of pathogen cells primed to subsequently evolve target-specific antibiotic resistance alleles.
Recent microbiological studies are beginning to clarify how nucleotide metabolism contributes to antibiotic efficacy (Figure 3). Interpretable machine learning analyses reveals that several metabolic pathways proximal to purine biosynthesis contribute to the lethality of bactericidal antibiotics in E. coli (9). Purine biosynthesis becomes induced by bactericidal stress-induced adenine limitation, which can be directly measured by targeted metabolomics (56). Consequently, oxidative phosphorylation becomes elevated to meet the increased energetic demand of enhanced purine biosynthesis, increasing cellular respiration and central carbon metabolism and providing substrates for toxic reactive oxygen species (42, 43). Indeed, regulation of nucleotide metabolism appears to be a well-conserved mechanism that bacteria have evolved to handle diverse stresses (65).
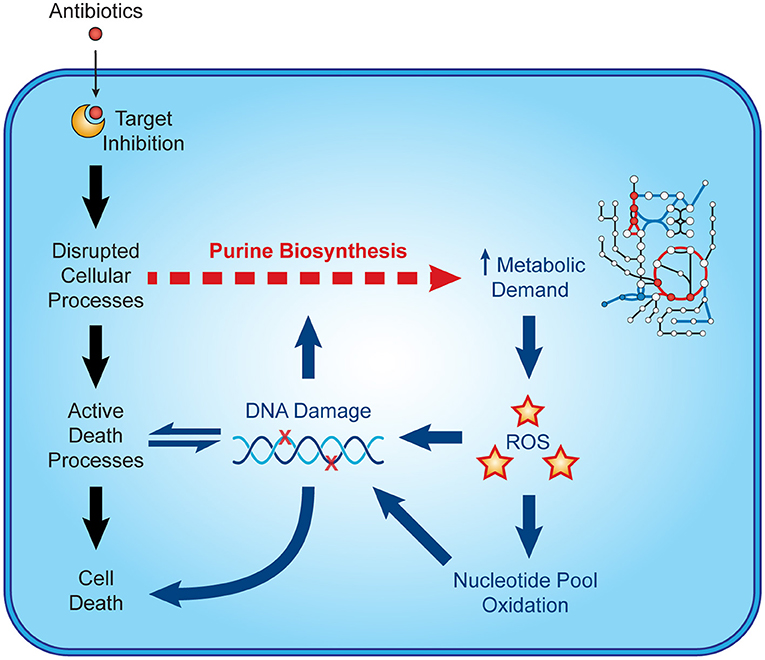
Figure 3. Nucleotide metabolism contributes to antibiotic lethality. In addition to their target-specific effects, bactericidal antibiotics induce purine biosynthesis, which increases activity in central metabolism. Increases in central metabolism stimulate the production of toxic reactive oxygen species, which oxidize nucleotides and damage DNA. These insults to DNA and the nucleotide pool induce bacterial death and may further potentiate purine biosynthesis.
Consistent with these, purine nucleotides such as (p)ppGpp function as universal alarmones for transcriptionally activating the stringent response and other bacterial stress responses as evolutionally conserved strategies for surviving nutrient limitation and other environmental stressors (66, 67). Intracellular accumulation of (p)ppGpp and related purine alarmones can induce antibiotic tolerance by promoting growth arrest (68) and entry to antibiotic persister states (69). Recent studies demonstrate that in additional to these transcriptionally mediated programs, (p)ppGpp can also inhibit nucleotide metabolism directly by binding several enzymes involved in purine biosynthesis, including PurF and Gsk (70, 71). These data collectively support a central role for nucleotide metabolism in antibiotic treatment efficacy.
It is interesting to note that nucleotide metabolism is also very important for the in vivo pathogenesis of diverse bacterial infections, and may be required for a pathogen's growth and survival within the host environment (72). For instance, S. aureus cells with transposon insertions in purB fail to establish bone infections in mice (73) and deletion of purine biosynthesis genes prevents uropathogenic E. coli from expanding into intracellular bladder epithelial cells (74). Likewise, in vivo studies of methicillin-resistant S. aureus showed that purine biosynthesis was causally linked to survival during endovascular infection (11). Collectively, it is clear that nucleotide metabolism, particularly purine biosynthesis, plays an important role in bacterial pathogenesis and in the response to antibiotic stress.
Discussion
The growing challenge of clinical antibiotic failure demands renewed attention into the study of antibiotic mechanisms of action and the discovery of new antimicrobial compounds. Digital technologies such as whole-genome sequencing, machine learning, mass spectrometry and predictive modeling are likely to transform the clinical management of bacterial infections in the coming decades. Exciting developments in machine learning are, for the first time, enabling the rapid discovery of novel classes of antimicrobial compounds (75) and the rapid identification of bacterial pathogens in the clinic (5). Advances in mass spectrometry-based metabolomics are enabling the rapid discovery of antimicrobial mechanisms of action (76). Advances in predictive modeling (7) are enabling new understanding into the complex ecology of microbial communities (77).
The discovery that nucleotide metabolism is involved in antibiotic efficacy has several translational implications. Unlike the Mueller-Hinton or Luria-Bertani media commonly used by clinical and academic microbiology laboratories, the metabolic microenvironment of a bacterial infection is dynamically enriched for nucleotide metabolites during infection (35). In fact, purine metabolites are important regulators of innate immunity (36), playing dual roles in regulating the host response to infection and the pathogen response to antibiotics. Nucleotide analogs are also commonly used to treat human cancers and viral infections and have potential to address antimicrobial resistance in the clinic (78, 79).
Nucleotide metabolism is one of the oldest areas of bacterial physiology to be investigated, with early studies into bacterial purine and pyrimidine metabolism predating the discovery of the lac operon (80, 81). Interest in nucleotide metabolism is mounting a resurgence, spurred by the growing recognition that nucleotides play important roles in both immunometabolism (82, 83) and cancer pathogenesis (84). Given that purine and pyrimidines exert opposing effects on antibiotic efficacy and carbon metabolism in bacteria (9), nucleotide metabolism represents an exciting open frontier for future studies in bacterial physiology and antibiotic treatment failure.
Concurrently, new digitalization techniques are becoming increasingly democratized and are poised to transform our basic and translational understanding of how nucleotide metabolism may contribute to antibiotic efficacy. Advances in predictive modeling (7) and non-targeted metabolomics (85) are revealing the diverse systems-level consequences of antibiotic stress. Quantitative microscopy advances (86) are enabling detection of antibiotic tolerance and resistance at single-cell resolution. Advances in transposon insertion sequencing (87) and adaptive lab evolution (88) are revealing new mechanisms for antibiotic resistance. Indeed, it would be exciting for future discoveries to reveal how nucleotide metabolism may contribute to antibiotic failure mechanisms beyond persistence (11) and potentially rewrite our understanding of antimicrobial resistance (29).
Author Contributions
AL and JY planned, wrote, and edited the manuscript. All authors critically read, reviewed, and approved the final version of the manuscript.
Funding
This work was supported by grant R00-GM118907 from the National Institutes of Health to JY.
Conflict of Interest
The authors declare that the research was conducted in the absence of any commercial or financial relationships that could be construed as a potential conflict of interest.
References
1. World Health Organization. Antimicrobial Resistance: Global Report on Surveillance. Geneva (2014).
2. Brown ED, and Wright GD. Antibacterial drug discovery in the resistance era. Nature. (2016) 529:336–43. doi: 10.1038/nature17042
3. Buchan BW, and Ledeboer NA. Emerging technologies for the clinical microbiology laboratory. Clin Microbiol Rev. (2014) 27:783–822. doi: 10.1128/CMR.00003-14
4. Han D, Li Z, Li R, Tan P, Zhang R, and Li J. mNGS in clinical microbiology laboratories: on the road to maturity. Crit Rev Microbiol. (2019) 45:668–85. doi: 10.1080/1040841X.2019.1681933
5. Smith KP, and Kirby JE. Image analysis and artificial intelligence in infectious disease diagnostics. Clin Microbiol Infect. (2020) 26:1318–23. doi: 10.1016/j.cmi.2020.03.012
6. O'brien EJ, Monk JM, and Palsson BO. Using genome-scale models to predict biological capabilities. Cell. (2015) 161:971–87. doi: 10.1016/j.cell.2015.05.019
7. Lopatkin AJ, and Collins JJ. Predictive biology: modelling, understanding and harnessing microbial complexity. Nat Rev Microbiol. (2020) 18:507–20. doi: 10.1038/s41579-020-0372-5
8. Yu MK, Ma J, Fisher J, Kreisberg JF, Raphael BJ, and Ideker T. Visible machine learning for biomedicine. Cell. (2018) 173:1562–5. doi: 10.1016/j.cell.2018.05.056
9. Yang JH, Wright SN, Hamblin M, Mccloskey D, Alcantar MA, Schrubbers L, et al. A white-box machine learning approach for revealing antibiotic mechanisms of action. Cell. (2019) 177:1649–61.e1649. doi: 10.1016/j.cell.2019.04.016
10. Kavvas ES, Yang L, Monk JM, Heckmann D, and Palsson BO. A biochemically-interpretable machine learning classifier for microbial GWAS. Nat Commun. (2020) 11:2580. doi: 10.1038/s41467-020-16310-9
11. Li L, Abdelhady W, Donegan NP, Seidl K, Cheung A, Zhou YF, et al. Role of purine biosynthesis in persistent methicillin-resistant Staphylococcus aureus (MRSA) infection. J Infect Dis. (2018) 218:1367–77. doi: 10.1093/infdis/jiy340
12. Didelot X, Bowden R, Wilson DJ, Peto TEA, and Crook DW. Transforming clinical microbiology with bacterial genome sequencing. Nat Rev Genet. (2012) 13:601–12. doi: 10.1038/nrg3226
13. Levy SB, and Mcmurry L. Plasmid-determined tetracycline resistance involves new transport systems for tetracycline. Nature. (1978) 276:90–2. doi: 10.1038/276090a0
14. Ball PR, Shales SW, and Chopra I. Plasmid-mediated tetracycline resistance in Escherichia coli involves increased efflux of the antibiotic. Biochem Biophys Res Commun. (1980) 93:74–81. doi: 10.1016/S0006-291X(80)80247-6
15. Blair JM, Webber MA, Baylay AJ, Ogbolu DO, and Piddock LJ. Molecular mechanisms of antibiotic resistance. Nat Rev Microbiol. (2015) 13:42–51. doi: 10.1038/nrmicro3380
16. Hughes D, and Andersson DI. Evolutionary trajectories to antibiotic resistance. Annu Rev Microbiol. (2017) 71:579–96. doi: 10.1146/annurev-micro-090816-093813
17. Lopatkin AJ, Bening SC, Manson AL, Stokes JM, Kohanski MA, Badran AH, et al. Clinically relevant mutations in core metabolic genes confer antibiotic resistance. Science. (2021) 371:eaba0862. doi: 10.1126/science.aba0862
18. Lazar V, Nagy I, Spohn R, Csorgo B, Gyorkei A, Nyerges A, et al. Genome-wide analysis captures the determinants of the antibiotic cross-resistance interaction network. Nat Commun. (2014) 5:4352. doi: 10.1038/ncomms5352
19. Baym M, Lieberman TD, Kelsic ED, Chait R, Gross R, Yelin I, et al. Spatiotemporal microbial evolution on antibiotic landscapes. Science. (2016) 353:1147–51. doi: 10.1126/science.aag0822
20. Anahtar MN, Yang JH, and Kanjilal S. Applications of machine learning to the problem of antimicrobial resistance: an emerging model for translational research. J Clin Microbiol. (2021). doi: 10.1128/JCM.01260-20. [Epub ahead of print].
21. Rasko DA, Webster DR, Sahl JW, Bashir A, Boisen N, Scheutz F, et al. Origins of the E. coli strain causing an outbreak of hemolytic-uremic syndrome in Germany. N Engl J Med. (2011) 365:709–17. doi: 10.1056/NEJMoa1106920
22. Karch H, Denamur E, Dobrindt U, Finlay BB, Hengge R, Johannes L, et al. The enemy within us: lessons from the 2011 European Escherichia coli O104:H4 outbreak. EMBO Mol Med. (2012) 4:841–8. doi: 10.1002/emmm.201201662
23. Hadfield J, Megill C, Bell SM, Huddleston J, Potter B, Callender C, et al. Nextstrain: real-time tracking of pathogen evolution. Bioinformatics. (2018) 34:4121–3. doi: 10.1093/bioinformatics/bty407
24. Hyun JC, Kavvas ES, Monk JM, and Palsson BO. Machine learning with random subspace ensembles identifies antimicrobial resistance determinants from pan-genomes of three pathogens. PLoS Comput Biol. (2020) 16:e1007608. doi: 10.1371/journal.pcbi.1007608
25. Deurenberg RH, Bathoorn E, Chlebowicz MA, Couto N, Ferdous M, Garcia-Cobos S, et al. Application of next generation sequencing in clinical microbiology and infection prevention. J Biotechnol. (2017) 243:16–24. doi: 10.1016/j.jbiotec.2016.12.022
26. Colangeli R, Jedrey H, Kim S, Connell R, Ma S, Chippada Venkata UD, et al. Bacterial factors that predict relapse after tuberculosis therapy. N Engl J Med. (2018) 379:823–33. doi: 10.1056/NEJMoa1715849
27. Monk JM. Predicting antimicrobial resistance and associated genomic features from whole-genome sequencing. J Clin Microbiol. (2019) 57:e01610–8. doi: 10.1128/JCM.01610-18
28. Nguyen M, Long SW, Mcdermott PF, Olsen RJ, Olson R, Stevens RL, et al. Using machine learning to predict antimicrobial MICs and associated genomic features for nontyphoidal salmonella. J Clin Microbiol. (2019) 57:e01260–18. doi: 10.1128/JCM.01260-18
29. Schrader SM, Vaubourgeix J, and Nathan C. Biology of antimicrobial resistance and approaches to combat it. Sci Translat Med. (2020) 12:eaaz6992. doi: 10.1126/scitranslmed.aaz6992
30. Mcarthur AG, Waglechner N, Nizam F, Yan A, Azad MA, Baylay AJ, et al. The comprehensive antibiotic resistance database. Antimicrob Agents Chemother. (2013) 57:3348–57. doi: 10.1128/AAC.00419-13
31. Maiden MC. Horizontal genetic exchange, evolution, and spread of antibiotic resistance in bacteria. Clin Infect Dis. (1998) 27(Suppl. 1):S12–20. doi: 10.1086/514917
32. Brauner A, Fridman O, Gefen O, and Balaban NQ. Distinguishing between resistance, tolerance and persistence to antibiotic treatment. Nat Rev Microbiol. (2016) 14:320–30. doi: 10.1038/nrmicro.2016.34
33. Yang JH, Bening SC, and Collins JJ. Antibiotic efficacy-context matters. Curr Opin Microbiol. (2017) 39:73–80. doi: 10.1016/j.mib.2017.09.002
34. Radlinski L, and Conlon BP. Antibiotic efficacy in the complex infection environment. Curr Opin Microbiol. (2018) 42:19–24. doi: 10.1016/j.mib.2017.09.007
35. Yang JH, Bhargava P, Mccloskey D, Mao N, Palsson BO, and Collins JJ. Antibiotic-induced changes to the host metabolic environment inhibit drug efficacy and alter immune function. Cell Host Microbe. (2017) 22:757–65.e753. doi: 10.1016/j.chom.2017.10.020
36. Cekic C, and Linden J. Purinergic regulation of the immune system. Nat Rev Immunol. (2016) 16:177–92. doi: 10.1038/nri.2016.4
37. Monk JM, Lloyd CJ, Brunk E, Mih N, Sastry A, King Z, et al. iML1515, a knowledgebase that computes Escherichia coli traits. Nat Biotechnol. (2017) 35:904–8. doi: 10.1038/nbt.3956
38. Hicks ND, Yang J, Zhang X, Zhao B, Grad YH, Liu L, et al. Clinically prevalent mutations in Mycobacterium tuberculosis alter propionate metabolism and mediate multidrug tolerance. Nat Microbiol. (2018) 3:1032–42. doi: 10.1038/s41564-018-0218-3
39. Stokes JM, Lopatkin AJ, Lobritz MA, and Collins JJ. Bacterial metabolism and antibiotic efficacy. Cell Metab. (2019) 30:251–9. doi: 10.1016/j.cmet.2019.06.009
40. Lopatkin AJ, Stokes JM, Zheng EJ, Yang JH, Takahashi MK, You L, et al. Bacterial metabolic state more accurately predicts antibiotic lethality than growth rate. Nat Microbiol. (2019) 4:2109–17. doi: 10.1038/s41564-019-0536-0
41. Dwyer DJ, Belenky PA, Yang JH, Macdonald IC, Martell JD, Takahashi N, et al. Antibiotics induce redox-related physiological alterations as part of their lethality. Proc Natl Acad Sci USA. (2014) 111:E2100–9. doi: 10.1073/pnas.1401876111
42. Lobritz MA, Belenky P, Porter CB, Gutierrez A, Yang JH, Schwarz EG, et al. Antibiotic efficacy is linked to bacterial cellular respiration. Proc Natl Acad Sci USA. (2015) 112:8173–80. doi: 10.1073/pnas.1509743112
43. Kohanski MA, Dwyer DJ, Hayete B, Lawrence CA, and Collins JJ. A common mechanism of cellular death induced by bactericidal antibiotics. Cell. (2007) 130:797–810. doi: 10.1016/j.cell.2007.06.049
44. Zhao X, and Drlica K. Reactive oxygen species and the bacterial response to lethal stress. Curr Opin Microbiol. (2014) 21:1–6. doi: 10.1016/j.mib.2014.06.008
45. Foti JJ, Devadoss B, Winkler JA, Collins JJ, and Walker GC. Oxidation of the guanine nucleotide pool underlies cell death by bactericidal antibiotics. Science. (2012) 336:315–9. doi: 10.1126/science.1219192
46. Fan X-Y, Tang B-K, Xu Y-Y, Han A-X, Shi K-X, Wu Y-K, et al. Oxidation of dCTP contributes to antibiotic lethality in stationary-phase mycobacteria. Proc Natl Acad Sci USA. (2018) 115:2210–5. doi: 10.1073/pnas.1719627115
47. Hong Y, Zeng J, Wang X, Drlica K, and Zhao X. Post-stress bacterial cell death mediated by reactive oxygen species. Proc Natl Acad Sci USA. (2019) 116:10064–71. doi: 10.1073/pnas.1901730116
48. Takahashi N, Gruber CC, Yang JH, Liu X, Braff D, Yashaswini C, et al. Lethality of MalE-LacZ hybrid protein shares mechanistic attributes with oxidative component of antibiotic lethality. Proc Natl Acad Sci USA. (2017) 114:9164–9. doi: 10.1073/pnas.1707466114
49. Hong Y, Li L, Luan G, Drlica K, and Zhao X. Contribution of reactive oxygen species to thymineless death in Escherichia coli. Nat Microbiol. (2017) 2:1667–75. doi: 10.1038/s41564-017-0037-y
50. Baek SH, Li AH, and Sassetti CM. Metabolic regulation of mycobacterial growth and antibiotic sensitivity. PLoS Biol. (2011) 9:e1001065. doi: 10.1371/journal.pbio.1001065
51. Shan Y, Brown Gandt A, Rowe SE, Deisinger JP, Conlon BP, and Lewis K. ATP-dependent persister formation in Escherichia coli. MBio. (2017) 8:e02267–16. doi: 10.1128/mBio.02267-16
52. Lee JJ, Lee SK, Song N, Nathan TO, Swarts BM, Eum SY, et al. Transient drug-tolerance and permanent drug-resistance rely on the trehalose-catalytic shift in Mycobacterium tuberculosis. Nat Commun. (2019) 10:2928. doi: 10.1038/s41467-019-10975-7
53. Zalis EA, Nuxoll AS, Manuse S, Clair G, Radlinski LC, Conlon BP, et al. Stochastic variation in expression of the tricarboxylic acid cycle produces persister cells. MBio. (2019) 10:e01930–19. doi: 10.1128/mBio.01930-19
54. Gutierrez A, Jain S, Bhargava P, Hamblin M, Lobritz MA, and Collins JJ. Understanding and sensitizing density-dependent persistence to quinolone antibiotics. Mol Cell. (2017) 68:1147–54.e1143. doi: 10.1016/j.molcel.2017.11.012
55. Meylan S, Porter CBM, Yang JH, Belenky P, Gutierrez A, Lobritz MA, et al. Carbon sources tune antibiotic susceptibility in Pseudomonas aeruginosa via tricarboxylic acid cycle control. Cell Chem Biol. (2017) 24:195–206. doi: 10.1016/j.chembiol.2016.12.015
56. Belenky P, Ye JD, Porter CB, Cohen NR, Lobritz MA, Ferrante T, et al. Bactericidal antibiotics induce toxic metabolic perturbations that lead to cellular damage. Cell Rep. (2015) 13:968–80. doi: 10.1016/j.celrep.2015.09.059
57. Milo R, and Phillips R. Cell Biology by the Numbers. New York, NY: Garland Science, Taylor & Francis Group (2016).
58. Walsh CT, Tu BP, and Tang Y. Eight kinetically stable but thermodynamically activated molecules that power cell metabolism. Chem Rev. (2018) 118:1460–94. doi: 10.1021/acs.chemrev.7b00510
59. Chapman AG, and Atkinson DE. Adenine nucleotide concentrations and turnover rates. Their correlation with biological activity in bacteria and yeast. Adv Microb Physiol. (1977) 15:253–306. doi: 10.1016/S0065-2911(08)60318-5
60. Hansen S, Lewis K, and Vulic M. Role of global regulators and nucleotide metabolism in antibiotic tolerance in Escherichia coli. Antimicrob Agents Chemother. (2008) 52:2718–26. doi: 10.1128/AAC.00144-08
61. Stokes JM, Gutierrez A, Lopatkin AJ, Andrews IW, French S, Matic I, et al. A multiplexable assay for screening antibiotic lethality against drug-tolerant bacteria. Nat Methods. (2019) 16:303–6. doi: 10.1038/s41592-019-0333-y
62. Zlitni S, Ferruccio LF, and Brown ED. Metabolic suppression identifies new antibacterial inhibitors under nutrient limitation. Nat Chem Biol. (2013) 9:796–804. doi: 10.1038/nchembio.1361
63. Yee R, Cui P, Shi W, Feng J, and Zhang Y. Genetic screen reveals the role of purine metabolism in Staphylococcus aureus persistence to rifampicin. Antibiotics. (2015) 4:627–42. doi: 10.3390/antibiotics4040627
64. Liu J, Gefen O, Ronin I, Bar-Meir M, and Balaban NQ. Effect of tolerance on the evolution of antibiotic resistance under drug combinations. Science. (2020) 367:200–4. doi: 10.1126/science.aay3041
65. Fitzsimmons LF, Liu L, Kim JS, Jones-Carson J, and Vazquez-Torres A. Salmonella reprograms nucleotide metabolism in its adaptation to nitrosative stress. MBio. (2018) 9:e002118. doi: 10.1128/mBio.00211-18
66. Hauryliuk V, Atkinson GC, Murakami KS, Tenson T, and Gerdes K. Recent functional insights into the role of (p)ppGpp in bacterial physiology. Nat Rev Microbiol. (2015) 13:298–309. doi: 10.1038/nrmicro3448
67. Irving SE, Choudhury NR, and Corrigan RM. The stringent response and physiological roles of (pp)pGpp in bacteria. Nat Rev Microbiol. (2020). doi: 10.1038/s41579-020-00470-y
68. Spira B, and Ospino K. Diversity in E. coli (p)ppGpp levels and its consequences. Front Microbiol. (2020) 11:1759. doi: 10.3389/fmicb.2020.01759
69. Amato SM, Orman MA, and Brynildsen MP. Metabolic control of persister formation in Escherichia coli. Mol Cell. (2013) 50:475–87. doi: 10.1016/j.molcel.2013.04.002
70. Wang B, Dai P, Ding D, Del Rosario A, Grant RA, Pentelute BL, et al. Affinity-based capture and identification of protein effectors of the growth regulator ppGpp. Nat Chem Biol. (2019) 15:141–50. doi: 10.1038/s41589-018-0183-4
71. Wang B, Grant RA, and Laub MT. ppGpp coordinates nucleotide and amino-acid synthesis in E. coli during starvation molecular. Cell. (2020) 80:29–42.e10. doi: 10.1016/j.molcel.2020.08.005
72. Samant S, Lee H, Ghassemi M, Chen J, Cook JL, Mankin AS, et al. Nucleotide biosynthesis is critical for growth of bacteria in human blood. PLoS Pathog. (2008) 4:e37. doi: 10.1371/journal.ppat.0040037
73. Potter AD, Butrico CE, Ford CA, Curry JM, Trenary IA, Tummarakota SS, et al. Host nutrient milieu drives an essential role for aspartate biosynthesis during invasive Staphylococcus aureus infection. Proc Natl Acad Sci USA. (2020) 117:12394–401. doi: 10.1073/pnas.1922211117
74. Shaffer CL, Zhang EW, Dudley AG, Dixon BREA, Guckes KR, Breland EJ, et al. purine biosynthesis metabolically constrains intracellular survival of uropathogenic Escherichia coli. Infect Immun. (2017) 85:e00471–16. doi: 10.1128/IAI.00471-16
75. Stokes JM, Yang K, Swanson K, Jin W, Cubillos-Ruiz A, Donghia NM, et al. A deep learning approach to antibiotic discovery. Cell. (2020) 181:475–83. doi: 10.1016/j.cell.2020.04.001
76. Zampieri M, Szappanos B, Buchieri MV, Trauner A, Piazza I, Picotti P, et al. High-throughput metabolomic analysis predicts mode of action of uncharacterized antimicrobial compounds. Sci Transl Med. (2018) 10:eaal3973. doi: 10.1126/scitranslmed.aal3973
77. Meredith HR, Andreani V, Ma HR, Lopatkin AJ, Lee AJ, Anderson DJ, et al. Applying ecological resistance and resilience to dissect bacterial antibiotic responses. Sci Adv. (2018) 4:eaau1873. doi: 10.1126/sciadv.aau1873
78. Liu Y, Yang K, Jia Y, Shi J, Tong Z, and Wang Z. Thymine sensitizes gram-negative pathogens to antibiotic killing. Front Microbiol. (2021) 12:622798. doi: 10.3389/fmicb.2021.622798
79. Serpi M, Ferrari V, and Pertusati F. Nucleoside derived antibiotics to fight microbial drug resistance: new utilities for an established class of drugs? J Med Chem. (2016) 59:10343–82. doi: 10.1021/acs.jmedchem.6b00325
80. Koch AL, Putnam FW, and Evans EA. The purine metabolism of Escherichia coli. J Biol Chem. (1952) 197:105–12. doi: 10.1016/S0021-9258(18)55658-1
81. Friedman S, and Gots JS. The purine and pyrimidine metabolism of normal and phage-infected Escherichia coli. J Biol Chem. (1953) 201:125–35. doi: 10.1016/S0021-9258(18)71354-9
82. Mazumdar C, Driggers EM, and Turka LA. The untapped opportunity and challenge of immunometabolism: a new paradigm for drug discovery. Cell Metab. (2020) 31:26–34. doi: 10.1016/j.cmet.2019.11.014
83. Palsson-Mcdermott EM, and O'neill LJ. Targeting immunometabolism as an anti-inflammatory strategy. Cell Res. (2020) 30:300–14. doi: 10.1038/s41422-020-0291-z
84. Campos-Contreras ADR, Diaz-Munoz M, and Vazquez-Cuevas FG. Purinergic signaling in the hallmarks of cancer. Cells. (2020) 9:1612. doi: 10.3390/cells9071612
85. Zampieri M, Zimmermann M, Claassen M, and Sauer U. Nontargeted metabolomics reveals the multilevel response to antibiotic perturbations. Cell Rep. (2017) 19:1214–28. doi: 10.1016/j.celrep.2017.04.002
86. Herricks T, Donczew M, Mast FD, Rustad T, Morrison R, Sterling TR, et al. ODELAM, rapid sequence-independent detection of drug resistance in isolates of Mycobacterium tuberculosis. Elife. (2020) 9:e56613. doi: 10.1101/2020.03.17.995480
87. Cain AK, Barquist L, Goodman AL, Paulsen IT, Parkhill J, and Van Opijnen T. A decade of advances in transposon-insertion sequencing. Nat Rev Gene. (2020) 21:526–40. doi: 10.1038/s41576-020-0244-x
Keywords: antibiotic resistance, antibiotic tolerance, antibiotic persistence, nucleotide metabolism, whole genome sequencing, machine learning, metabolic modeling, predictive modeling
Citation: Lopatkin AJ and Yang JH (2021) Digital Insights Into Nucleotide Metabolism and Antibiotic Treatment Failure. Front. Digit. Health 3:583468. doi: 10.3389/fdgth.2021.583468
Received: 15 July 2020; Accepted: 02 February 2021;
Published: 03 March 2021.
Edited by:
Belén Rodriguez-Sanchez, Gregorio Marañón Hospital, SpainReviewed by:
Juan Liu, Huazhong University of Science and Technology, ChinaHao Wang, Shenzhen University General Hospital, China
Copyright © 2021 Lopatkin and Yang. This is an open-access article distributed under the terms of the Creative Commons Attribution License (CC BY). The use, distribution or reproduction in other forums is permitted, provided the original author(s) and the copyright owner(s) are credited and that the original publication in this journal is cited, in accordance with accepted academic practice. No use, distribution or reproduction is permitted which does not comply with these terms.
*Correspondence: Allison J. Lopatkin, YWxvcGF0a2lAYmFybmFyZC5lZHU=; Jason H. Yang, amFzb24ueUBydXRnZXJzLmVkdQ==
†These authors have contributed equally to this work