- 1Family and Human Development, Sanford Harmony Institute, Arizona State University, Tempe, AZ, United States
- 2Department of Educational Psychology, University of Georgia, Athens, GA, United States
- 3Sanford Harmony Institute, Children's Equity Project, Arizona State University, Tempe, AZ, United States
- 4Sanford Harmony Institute, Arizona State University, Tempe, AZ, United States
A key goal of the U.S. and its citizens is to promote positive development for young children and their families, particularly those who are marginalized and vulnerable. However, suspending young children from pre-kindergarten (Pre-K) undermines this goal. The primary purpose of the present research was to document the extent to which young children are suspended from Pre-K and how these suspensions are related to the quality of the Pre-K neighborhood. To do this, we used national data from the 26,122 public Pre-K schools in the 2017-2018 Civil Rights Data Collection and examined how the quality of neighborhood resources related to the use of out-of-school suspensions (OSS) for all children, as well as separately for Black and white children, and male and female students. We found that children living in low-opportunity neighborhoods tended to be enrolled in U.S. Pre-K public school programs that had high rates of OSS. We also found that disparities in the rates of use of OSS were greater for Black relative to white students and male relative to female students in Pre-K schools that resided in relatively low-resourced neighborhoods. The findings highlight that one of the ways that neighborhoods influence children's health and well-being is through the disciplinary culture of its schools. The present findings also highlight that disparities in the use of OSS are tied to these neighborhood opportunities and conditions.
Introduction
A key goal of the U.S. and its citizens is to promote positive development for young children and their families, particularly those who are marginalized and vulnerable. One way to achieve this goal is to provide access to high-quality early childhood education programs that help prepare young children to be successful when they make the transition into formal schooling and, ultimately, become responsible and productive members of society. Early childhood educators seek to produce these outcomes by helping young children be engaged and ready to learn (Williams et al., 2023). Achieving this goal requires, in part, that early childhood program educators successfully manage the behaviors of the young children in their care to ensure that the caregiving and learning environments are safe, secure, and conducive to learning.
Although there are many elements to the management of early education classrooms (Banghart et al., 2020), the discipline of young children's behavior is a significant facet. Discipline is an adult decision, typically in response to a child's behavior or perceived behavior, that is intended to change that behavior. Positive, proactive forms of discipline promote academic and socio-emotional development and can improve emotional and behavioral regulation. Other forms of discipline are harsh, harm student development, and catalyze a set of events that negatively impacts a student's life trajectory (see Sege and Siegel, 2018). In particular, the use of out-of-school suspensions (OSS) has been found to undermine student wellbeing and educational achievement, as well as contributing to the “school to prison pipeline” by initiating a possible transition from the educational system to the criminal justice system (Morris and Perry, 2016; Welsh and Little, 2018b; Zinsser and Wanless, 2020). Additionally, researchers have found children who were suspended early in their schooling suffer long-term educational consequences (Andrew and Blake, 2023).
Considerable attention has been given to the use of OSS in U.S. public K-12 schools (Fabes et al., 2021a; Perry and Morris, 2014; Skiba and Losen, 2016), yet, with few exceptions (Chow et al., 2021; Gilliam and Shahar, 2006; Zinsser, 2023), there remains little published information in the peer-reviewed scientific literature focusing on the predictors of OSS among children enrolled in U.S. publicly funded pre-kindergarten (Pre-K) schools. Such an oversight is significant as almost 1.4 million children are enrolled in state-funded Pre-K schools with almost 30% of all 4-year-olds in the U.S. enrolled in such schools (National Institute for Early Education Resarch, 2022).
Importantly, the degree to which public Pre-K schools are capable of providing safe and high-quality care and attention is likely in part a reflection of local community resources and opportunities. In the present research, we focus on neighborhood opportunity—the extent to which neighborhoods provide conditions and resources that matter for children's healthy development (e.g., access to healthy food, clean air, homeownership, etc.; see Clemens et al., 2020). Thus, the extent to which a Pre-K school is located in a neighborhood that can provide adequate resources and safety, as well as opportunities for healthy growth and development, likely affects the quality of the Pre-K program (Valentino, 2018). These neighborhood opportunities also likely affect the degree to which a Pre-K school relies on the use of OSS with its young students, although no research exists on this topic. Given this, there is a need for research that can help us better understand how neighborhood opportunity is related to the use of OSS in Pre-K. Such information can then be used to develop strategies to curtail and interrupt the adverse cycle associated with the use of OSS in early childhood education programs. The present study was designed to fill these gaps by examining how the relative level of neighborhood opportunity predicts the prevalence of OSS use with Pre-K public school students, as well as how racial/ethnic and gender disparities in the use of OSS were related to neighborhood opportunity.
The use of OSS in pre-kindergarten
Although early childhood education in the U.S. is intended to be inclusive and provide equitable access to developmentally appropriate early childhood education that helps children, families, and communities thrive, many young children are suspended from Pre-K and are thus deprived of the opportunities needed to be successful in school and beyond. Suspension, or the temporary removal of a child from her/his classroom, undermines the goals of helping young children and their families thrive and be prepared for the start of formal schooling (American Academy of Pediatrics Committee on School Health, 2013). Moreover, the adverse consequences of early OSS can be serious and cumulative, as students are separated from their peers, their teachers, and the early educational environment that serves as the foundation for later success. For example, children who experience early suspensions are more likely to hold negative attitudes about school, perform poorly in school, dropout, and experience more subsequent suspensions than children who do not experience OSS (Petras et al., 2011; Sundius and Farneth, 2008). OSS also puts added stress on families who must unexpectedly manage the care of their young child who has been suspended from Pre-K.
Although the use of OSS undermines these goals of supporting and sustaining students, precise national estimates of the use of OSS in U.S. public Pre-K schools are lacking. Gilliam (2005) conducted the first-ever analysis of preschool expulsions in a sample that included data from the 40 U.S. states that provided funding for preschool. Findings from that study suggested that the rates of expulsion (suspension was not included) were three times that of their older peers in K-12 grades and that the rates varied considerably from one state to another. In another study, Gilliam and Shahar (2006) examined rates and predictors of preschool exclusion and suspension in a random sample of schools in Massachusetts and found that 39% of preschool teachers reported expelling at least one child and 15% reported suspending at least one child. Moreover, the expulsion rate for children was found to be 34 times greater than the state-level K-12 rate and more than 13 times greater than the national K-12 expulsion rate. In contrast, rates of preschool suspensions were less than those for K-12. National rates of Pre-K OSS were, however, not reported.
Although Gilliam's research captured national media attention, it was not until nearly a decade later that the U.S. Department of Education's Office for Civil Rights (OCR) began collecting suspension data in public Pre-K schools. The Pre-K public school discipline data were released to the public for the first time in 2014. In those data, the U. S. Department of Education (2016) found that about 6% of public Pre-K schools reported suspending at least one child. Moreover, the report identified inequities in the use of suspension for Pre-K children, where Black preschool children were 3.6 times more likely to receive one or more out-of-school suspensions than white preschoolers, and Black boys, despite comprising 19% of all preschool students, accounted for 45% of all Pre-K suspensions. Similarly, Black girls represented 54% of all female out-of-school suspensions but made up only 20% of the Pre-K female public school enrollment.
Using a different dataset in which preschool OSS was reported by parents (in the 2016 National Child Health Study), the Center for American Progress (Malik, 2017) found that an estimated 50,000 preschoolers were suspended at least once and that about 17,000 preschoolers were expelled. Across all types of settings, these data suggest that roughly 250 preschoolers were being expelled or suspended every school day. Additionally, Chow et al. (2021) found that at least one preschool-aged child was subject to some form of exclusionary discipline in more than 25% of responding teachers' classrooms.
These limited findings provide a foundation for understanding the use of OSS in Pre-K, but as noted by Stegelin (2018), there is a critical need to conduct such research across multiple contexts, including national, state, and community levels. Such research is needed as OSS in U.S. public schooling use has been found to vary substantially from one state to another, from one school district to another, and from one school to another (Fabes et al., 2021b). For instance, in work with K-12 schools, it was found that 10% of the schools were responsible for 50% of the suspensions (Skiba and Rausch, 2006). To date, however, no such work exists for public U.S. Pre-K schools. The present research was designed to address this issue by focusing on the use of OSS in public Pre-K schools and how its use may vary as a function of the quality of neighborhood opportunities that facilitate the growth and wellbeing of young children. Such research is essential for understanding the role of neighborhood factors in predicting the prevalence of and disparities in the use of OSS in Pre-K.
Neighborhood opportunity and the use of OSS in pre-k
Researchers have found that the degree to which a neighborhood provides resources and opportunities for the healthy development and wellbeing of children has important consequences for both the short- and longer-term outcomes for them, their families, and their broader communities (Acevedo-Garcia et al., 2020a). Supportive neighborhood conditions (e.g., safe playgrounds and parks, clean air, healthy foods, etc.) can provide protective and promotive opportunities that help mitigate the effects of adverse experiences (Kim et al., 2019; Newburger et al., 2011). Unfortunately, many children live in neighborhoods that limit access to these supportive resources and conditions and thus do not have access to some of the protective and promotive opportunities that exist in more advantaged neighborhoods, including key elements of housing and food security.
Although a growing body of research has linked neighborhood characteristics to the physical, social, and academic development of young children (e.g., Dupere et al., 2010; Leventhal and Brooks-Gunn, 2000; Witherspoon et al., 2023), much of this research has focused on income-related disparities within a neighborhood (Dupere et al., 2010). For example, schools in low-income neighborhoods tend to have fewer social, financial, and instructional resources, and poorer student achievement than schools in high-income neighborhoods (Baker et al., 2018; Owens, 2010). Moreover, when neighborhoods are highly segregated by income, these disparities in school quality and their associated outcomes have been found to be exacerbated (Owens and Candipan, 2019). Bassok and Galdo (2016), for instance, found that low-income communities of color offered state preschool classrooms that were rated significantly lower in classroom quality than were classrooms that served high-income, low-minority communities. In another study, childcare center quality was found to be lower in disadvantaged neighborhoods than it was in advantaged neighborhoods (Burchinal et al., 2008).
Such disparities in Pre-K quality have significant implications for the children who attend these programs (Hatfield et al., 2016; Weiland et al., 2013). Schools that serve students from disadvantaged backgrounds often employ teachers and administrators with less experience and poorer credentials, have less equipment and technology, and enroll children who are lower-performing and experience more Adverse Childhood Experiences (ACEs). Research has found, for example, that almost half of all public-school teacher turnover takes place in just one quarter of the population of public schools; namely, high-poverty public schools that serve large number of students of color (Ingersoll et al., 2018). These race/ethnicity-based gaps in Pre-K quality likely also reflect income-based quality disparities, as research suggests that programs that are racially segregated tend to be economically segregated as well (Orfield and Frankenberg, 2014). For instance, Latham et al. (2020) found that the large racial disparities in the quality of Pre-K programs in New York City were partially explained by lack of proximal access to Pre-K programs that are of high quality. It follows that understanding the disparities between neighborhoods and their conditions are important to informing policies and practices that reduce disparities in education and related outcomes.
Despite a rich history of research focused on the impact of neighborhood characteristics on children's development (e.g., Leventhal et al., 2015; McCulloch and Joshi, 2001; Minh et al., 2017), there has been a call for more multidimensional conceptualizations of neighborhood opportunities (McCoy et al., 2023; Wei et al., 2021) and that reflect young children's experiences in their neighborhoods (Browning and Rigolon, 2019; Kiziltaş and Sak, 2018). In the present research, we utilized such a multidimensional assessment of neighborhood opportunity and examined how this predicts how young children are disciplined in their neighborhood Pre-K schools.
Although there is limited research exploring how neighborhood opportunities influence the use of OSS in Pre-K, Zeng et al. (2019) provide some preliminary evidence for this. In this research, community-level ACEs were found to contribute to the likelihood that children received OSS. Based on parent-reported OSS data, these researchers found that the odds ratio for OSS increased by 80% per unit increase in ACEs and suggested that exposure to toxic stress and unsafe and unstable neighborhood environments increases the risk of suspension in Pre-K. Given that ACEs are more likely to be greater in neighborhoods that do not provide the opportunities and resources that foster healthy development, Zeng et al.'s findings support the hypothesis that, compared to high-opportunity neighborhoods, OSS use will be greater among Pre-K children enrolled in programs located in low-opportunity neighborhoods.
Moreover, based on the large body of research documenting the disproportional use of OSS with Black relative to white children and with boys relative to girls (see, for example, Fabes et al., 2021b), we predicted that disparities in OSS would be greater in for young students enrolled in Pre-K schools located in low-opportunity neighborhoods relative to those located in neighborhoods that provide greater opportunities to their young children. Such a prediction is based on the expectation that conditions in low-opportunity neighborhoods undermine teachers' effective classroom management and reinforce implicit and explicit bias, thereby increasing the likelihood that the use of OSS will be disproportionally used with Black and male Pre-K students. This prediction is also based on the fact disadvantaged families tend to be segregated into communities that are low-resourced, high in insecurity and stress, and are subject to stereotyped and discriminative beliefs and practices that propagate experiences related that foster marginalization, bias, and racism (e.g., Chetty et al., 2019; Leventhal et al., 2015).
We tested these hypotheses by integrating national school-level data on the use of OSS in public Pre-K schools with a measure that reflects neighborhood resources and conditions that matter for children's healthy development—The Child Opportunity Index (COI) 2.0. The COI is a summary measure of the quality of the neighborhood for all census tracts in the U.S. in 2015 and reflects a multidimensional characterization of Pre-K communities (see Methods section for more details about the COI). We used these data to ascertain the degree to which disparities exist in the use of OSS in Pre-K programs that are located in neighborhoods that vary in the resources and opportunities they provide.
The current study
In the present research, we have three related goals. First, to address the lack of published documentation on the use of OSS in U.S. public Pre-K schools, we present descriptive information detailing the landscape of its use. Second, we examined how variation in the use of OSS in public Pre-K schools was associated with the opportunities provided within their school neighborhoods. We predicted that, relative to Pre-K schools in relatively high-opportunity neighborhoods, both the likelihood of using OSS in a Pre-K school and the count of incidences of use of OSS (controlling for enrollment) will be greater in Pre-K schools located in low-opportunity neighborhoods relative to Pre-K schools in high-opportunity neighborhoods.
The final goal was to examine disparities in the use OSS and how these varied between Pre-K schools located in relatively low- vs. high-opportunity neighborhoods. We expected to find evidence that the use of OSS would be greater for Black relative to white children and for boys relative to girls. We focused our attention on disparities for Black children rather than other marginalized racial/ethnic groups of students based on data showing that Black students receive the most inequitable use of OSS (e.g., McIntosh et al., 2021). Importantly, we expected that these disparities would be greater in Pre-K schools in low-opportunity neighborhoods compared to those located in high-opportunity neighborhoods.
Methods
Data
The data for this study were retrieved from three publicly available secondary datasets. Specially, we accessed data from: (1) the Civil Rights Data Collection for 2017–18 (CRDC; U. S. Department of Education, 2020), (2) the Child Opportunity Index 2.0 data for 2015 (Clemens et al., 2020), and (3) the Education Demographic and Geographic Estimates program (EDGE; Geverdt, 2018).
CRDC
The CRDC is a biennial survey that requires every public school and school district in the U.S. to report key information on education and civil rights issues. The 2017–18 CRDC collected data from the universe of all Local Education Agencies and schools and contains information on school characteristics, student enrollment and demographics, educational programs and services, and disciplinary practices such as suspension and expulsion. The CRDC includes a wide range of information on Pre-K schools and students and, as such, it is the most suitable dataset available for analyzing the use of OSS in U.S. public Pre-K schools.
COI
As noted, this study used the 2015 version of the COI to assess the neighborhood contexts in which Pre-K schools were located (see http://data.diversitydatakids.org/dataset/coi20-child-opportunity-index-2-0-database/resource/080cfe52-90aa-4925-beaa-90efb04ab7fb). The COI is a measure of structural opportunity for children and consists of a composite index constructed from 29 indicators that assess neighborhood-based opportunities in three domains: education (e.g., third-grade reading proficiency, high school graduation rate, teacher experience), health and environment (e.g., access to green space, ozone concentration, health insurance coverage), and society and economy (e.g., homeownership and employment rates, single-headed households, poverty rate). Each domain was standardized and weighted to create domain scores, as well as an overall index score. The indicators comprising the COI were drawn from numerous public sources such as the U.S. Census Bureau, National Center for Health Statistics, Department of Education, Environmental Protection Agency, and others. The only proprietary data included was a school-level dataset with math and reading achievement, as well as high school graduation data licensed from Great Schools (Clemens et al., 2020). COI data are provided at several geographical levels, including Census tracks.
To obtain a national COI score, the census tracts were ranked on a single overall score and then divided the tracts into 100 groups, from 1 to 100. The bottom 1% of tracts were assigned a score of 1, the next 1% were assigned a score of 2, and so on. Thus, COI national scores ranged from 1 to 100 with 100 reflecting the top 1% of all (Clemens et al., 2020; Noelke et al., 2020).
EDGE
The U.S. Department of Education distributes data that describe the geographical contexts of school-age children through the Education Demographic and Geographic Estimates (EDGE) program. To link individual schools to their Census tract, we used the 2018 Geographic Relationship Files (Geverdt, 2018). These data are tables that identify geographic associations for each U.S. public school, with their National Center for Educational Statistics (NCES) ID, including school boundaries and the latitude and longitude of the school that then can be directly linked to Census tracts (using https://www.geocod.io/for the geocoding). The tables were constructed from the Census Bureau's Topologically Integrated Geographic Encoding and Referencing database.
Dataset creation
Sample
From the total sample of 97,633 public schools included in the 2017–2018 CRDC data collection, 31.6%) were identified as public Pre-K schools. Of these, 1,882 were identified as alternative types of schools (e.g., special education, juvenile justice, virtual, magnet, special placement, etc.) and were not included in the final sample. For the 28,368 Pre-K that remained in the sample, 1,185 Pre-K schools failed to report any enrollment and were excluded.
Each of the 27,183 Pre-K schools remaining in the CRDC data set has a unique school ID number that reflects both their LEA and school ID numbers. The EDGE data set uses the NCES ID, which was used to connect the various data sets. Our first step was to merge COI and EDGE data to link Census tract information for each school by matching the CRDC and EDGE school ID numbers. We next used the Census tract data to link schools in the CRDC data set to their corresponding COI data. When these data integration efforts were finalized, 1,061 (3.9%) Pre-K schools were missing COI data due to a lack of matching CRDC and NCES ID numbers. Thus, the final sample of Pre-K schools that had full data and were included in the analyses of this study was 26,122 Pre-K public schools (see Figure 1 for a flowchart of the steps used to create the final dataset).
Comparisons of those schools that did and did not have COI data revealed that the 26,122 Pre-K schools with COI data tended to be smaller in enrollment (M = 48.37, enrolling 1,263,352 Pre-K students) than those schools without COI data (M = 63.26, enrolling 67,116 Pre-K students). Schools with COI data also were less likely to enroll proportionally fewer non-white students (M = 50%) relative to those Pre-K schools that did not have COI data (M = 69%). Finally, relative to schools that did not have COI data, COI schools also were more likely to be charter than non-charter schools (Ms = 2.41 and 1.70%, respectively) and were more likely to use OSS (M proportion of schools using OSS = 5.52 and 1.69%, respectively).
Some of these differences are likely a result of the reasons why the CRDC and EDGE school IDs could not be matched. For example, there were a relatively large number of schools in New York City (n = 489) that did not have matching IDs. The missing information is due to the fact that New York City Public School data are reported as a single district in the CRDC but as multiple districts in EDGE data. Thus, the New York City Public School District has a single ID in the CRDC but multiple ID numbers in EDGE that could not be matched to COI Census tract data. Additionally, in some states, charter schools are reported differently in the CRDC compared with EDGE data collection. For instance, some charter schools in California were reported as independent (with each school serving as its own school district) in the CRDC but as a single combined school district in the EDGE data. Thus, each school had its own ID in the CRDC, but there would be one ID number for the combined district in EDGE and thus could not be matched to COI Census tract data (n = 148). Finally, some of the missing COI data was due to differences in the timing of the CRDC and EDGE data, as there can be a lag between when the CRDC survey was planned and when the data collection actually began. During this lag time, a new school may open. Since the school was not yet assigned an ID number, it was reported in the CRDC as a new school and would not be matched in the EDGE data. In these cases, the schools would not have COI Census tract data (see NCES, 2020).
Out-of-school suspension
The total number of students who received at least one OSS incident in each Pre-K school was calculated by summing two count OSS variables: the total number of students without disabilities who received only one OSS and the total number of students without disabilities who received more than one OSS. Reports of OSS also identify the number of cases broken down by gender and race (as well as other information such as disability status). For the present purposes, we calculated the total number of cases of OSS for each school as a whole, as well as separately for male and female students and for Black and white students.
For some of the analyses, we also computed the rate of use of OSS for each school. The rate was calculated by taking the number of OSS reported by a school, dividing it by the enrollment for that school, and then multiplying this by 1,000 to create a rate per 1,000 students enrolled. We also did this with the various subgroups of students. We then used these rates to calculate a school-level OSS risk ratio for each school for Black students relative to their white peers and for male students relative to their female peers. The OSS risk ratio for Black/white students was computed by dividing the rate for Black students in a school by the OSS rate for white students in a school. We computed a similar school-level OSS risk ratio for male/female students by dividing the OSS rate for male students in a school by the OSS rate for female students in a school. We corrected for the issue of dividing by zero by adjusting the rates using the Haldane-Anscombe correction of adding 0.5 to every OSS rate before calculating the risk ratio (Anscombe, 1956; Haldane, 1940; Ruxton and Neuhäuser, 2013).
School-level demographics
Although the CRDC data are reported only at the school level (not the child level), each school reports the number of children enrolled and these enrollment data are broken down by both gender and race/ethnicity. As such, we were able to calculate the number (and proportions) of male and female students, as well as the number (and proportions) of Black and white students enrolled in a school. Thus, the demographic comparisons presented in the analyses reflect school- rather than child-level analyses. As such, we could not include child-level interaction terms in our analyses (e.g., race X gender) and examined these school-level demographics as separate main effects instead.
Covariates
Because OSS rates tend to be higher in charter than in non-charter schools (Center for Learner Equity, 2021), we included charter status (0 = not charter, 1 = charter) as a covariate in the primary analyses. Additionally, to control for the size of the school in the primary analyses, we included enrollment for a school or for a student group (e.g., enrollment of female students) and included that as a covariate in the primary analyses.
Analytic plan
To address our goal to provide descriptive national data that illuminate the prevalence with which U.S. public Pre-K schools use OSS with its students, we provide descriptive data that summarize rates of OSS for all schools, as well as for those Pre-K schools that reported at least one case of OSS. We then analyze for the expected disparities in the use of OSS in Pre-K by computing repeated measures analyses of school-level comparisons of Black and white students and for male and female students. Because of the large sample size, for all analyses, we only discuss findings that are significant at or beyond the p < 0.01 level.
These descriptive analyses were then followed by the primary analyses that focus on the role of neighborhood opportunity in predicting the use of OSS in Pre-K. Because schools were nested in districts and districts were nested in states, we used multilevel models that accounted for these nesting effects. Moreover, because most Pre-K public schools do not report using OSS (a value of zero), variables for OSS have excessive zeros exhibiting overdispersion. Consequently, we computed a multilevel zero-inflated Poisson (ZIP) model that allowed us to simultaneously estimate (1) a logistic regression that predicted the likelihood of being in the nonuse category (i.e., a latent class of Pre-K school that did not report using OSS) and (2) a regression that used a Poisson distribution to predict the count of OSS use among the latent class of those who would use OSS, including users estimated to use it zero times according to the Poisson distribution. Thus, the zero-inflated Poisson model predicts the probability of being unable to assume any value except zero using a logistic regression as well as the count level of OSS (Lambert, 1992; Mullahy, 1986).
As noted, in a ZIP model zeroes come from the two sources: the point mass and the count component (Zeileis et al., 2008). The first part models the probability that schools report zero OSS incident (vs. with at least one incident; Equation 1) and the second part models the OSS counts (Equation 2).
In these equations, Yijk is the count of OSS of school i in district j in State k. The probability that Yijk = 0 is modeled (on the logit scale) as a function of an overall intercept across schools, districts, and States () and a set of covariates (), the multilevel components are captured by the random effects associated with States and districts ( and ). For interpretation, captures the overall probability that a Pre-K program in the U.S. uses OSS, and quantify the association between the probability that Pre-K programs use OSS and the covariates. In Equation 2, the OSS counts are denoted as Yijk and these counts are modeled as a function of an overall intercept (), a set of covariates (), and captures the average OSS rate, and measures the associations between OSS counts and the covariates. and are the random effects associated with the states and districts, which capture the deviance of the state k from the over average and the district j from the state average, respectively.
For the multilevel structure, the analyses used three-level models to account for schools nested within districts within states. Data analyses for multilevel modeling, including the multilevel ZIP model, were conducted in lme4 and glmmTMB packages in the R platform (Bates et al., 2015; R Core Team, 2024).
The final multilevel ZIP model includes three school-level predictors: COI, enrollment for all students, and charter school status, while accounting for nesting of schools in districts and districts in states.
We next assessed the degree to which our measure of childhood community opportunity predicted Black-White and Male-Female disparities in the use of OSS. To do this, we computed two linear multilevel multiple regressions in which the outcomes were the risk ratios for Black-White or Male-Female with COI, enrollment for the relevant student groups (Black enrollment and white enrollment or male enrollment and female enrollment), and charter school status as school-level control predictors entered simultaneously, while once again accounting for state and district nesting
Results
Use of OSS in U.S. public Pre-K schools
Across all the 26,122 Pre-K schools, there was a total of 2,633 reports of use of OSS (either single or multiple; M = 0.10; SD = 0.59; range = 0–29). These 2,633 cases were reported by 1,442 Pre-K schools. Thus, about 5.5% of public Pre-K schools in the U.S. reported at least one instance of using OSS with their students (M of these schools = 1.83; SD = 1.76). Of these Pre-K schools that reported at least one case of using OSS, a relatively small number of schools accounted for a disproportionate number of cases of OSS. Specifically, about 17.5% of Pre-K schools using OSS accounted for 43% of all cases of OSS in U.S. public Pre-K schools.
Because there was considerable variation in the enrollments in the Pre-K schools (M = 48.37, SD = 61.22, range = 1–2,700), it was important to account for this enrollment variation in understanding the use of OSS within a Pre-K school. In many of the analyses, we did this by using OSS rates per 1,000 students enrolled (see Methods section). The average OSS rate for all U.S. public Pre-K schools (n = 26,122) was 2.56 (SD = 17.43). However, the average rate was more than 20 times higher when only focusing on Pre-K schools that reported at least one case of OSS (n = 1,442, M = 46.44, SD = 58.86).
Table 1 presents the school-level mean rates of OSS for Black and white students and for male and female students, separately for all schools and for those reporting at least one case of OSS. The means in Table 1 revealed the expected disparities for Black students relative to white students and for male students relative to female students.
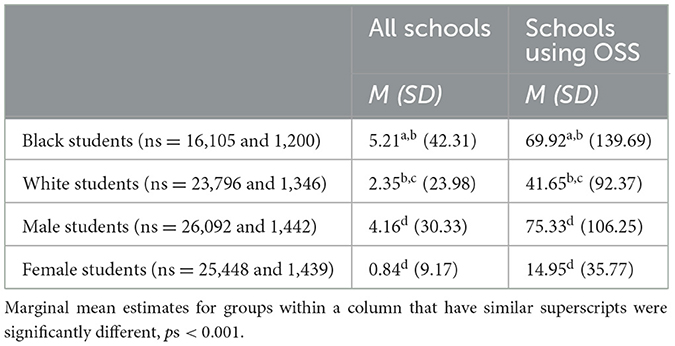
Table 1. Mean school-level OSS rates per 1,000 students for all schools and for those using OSS: by student demographics.
To determine if these disparities were statistically significant (p < 0.01), we conducted a series of 1-way repeated measures analyses of co-variance with school-level race or gender as within-school factors controlling for total enrollment and charter school status (0 = no and 1 = yes). We did this for all Pre-K schools as well as for those Pre-K schools that reported at least one case of OSS.
For the repeated measures analyses of covariance including all Pre-K schools, there were significant within-school main effects for the analysis of race and for the analysis of gender, Fs(1, 14,555 and 1, 25,415) = 41.49 and 260.73, ps < 0.001, partial etas2 = 0.02, respectively. For the contrasts of estimated marginal means, Black students had significantly higher OSS rates than did white students. For the gender analysis, boys had higher OSS rates than did girls (see Table 1).
Similar patterns were found for the repeated measures analyses using only those Pre-K schools that reported at least one case of OSS. Once again, there were significant within-school main effects for the analysis of race and for the analysis of gender, Fs(1, 1,111 and 1, 1,436) = 45.42 and 414.42, ps < 0.001, partial etas2 = 0.04 and 0.22, respectively. For the contrasts of estimated marginal means, Black students had significantly higher OSS rates than did white students and boys were once again found to have higher OSS rates than did girls. Not surprisingly, the rates of OSS in schools using OSS were considerably higher compared to all schools combined (see Table 1).
Analyses of the role of COI in the use of OSS in public Pre-K schools
The mean COI national score for the 26,122 Pre-K schools included in our sample was 46.76 (SD = 27.12; range = 1–100). Zero-order correlations with school demographic qualities revealed that Pre-K schools with higher COI national scores tended to be those that had smaller enrollment, non-charter status, enrolled proportionally more white students and proportionally fewer Black students, rs(26,120) = −0.09, −0.07, 0.31, and −0.36, all ps < 0.001, respectively.
Multilevel zero-inflated Poisson regression model of count and use/nonuse
For the primary goal focused on the relation of COI and the use of OSS in U.S. public Pre-K schools, we report the results of zero-inflated Poisson multilevel regression model. As noted, the zero-inflated Poisson model predicts the value of the dependent variable (the count of OSS) as well as the probability of being in the zero-OSS use group using a logistic regression estimated with maximum likelihood with robust standard errors. The school-level predictors included in this model were national COI score, total enrollment, and charter/not-charter status (accounting for schools nested in districts and districts nested in states).
The results for this ZIP model are presented in Table 2. COI in the zero-inflated Poisson model predicted both the count of OSS and the probability of not using OSS. Specifically, Pre-K schools with greater community opportunities (i.e., higher COI scores) had lower numbers of OSS and had a relatively higher probability of not using OSS, controlling for enrollment and charter school status. Additionally, schools with larger numbers of enrollment and those that were charter schools were relatively likely to have greater counts of OSS and were relatively less likely to report zero OSS incidents (see Table 2).
Multilevel linear regression models of black/white and male/female risk ratios
The average school-level OSS risk ratios for Black/white and for male/female students were 9.44 and 8.12 (SDs = 80.58 and 63.46, Ns = 14,558 and 25,418, respectively). Thus, Black students were more than 9 times more likely to receive an OSS than were their white peers and boys were more than 8 times more likely to receive an OSS than were girls.
The multilevel linear regression (accounting for district and state nesting) for the predictions of school-level Black/white and male/female OSS risk ratios are presented in Table 3. For the OSS risk-ratio analysis for Black/white students, COI was significantly and inversely related to the OSS risk-ratio outcome. Thus, for those Pre-K schools in Census tracts that had lower levels of community opportunity, the Black/white disparity in the use of OSS was greater than for those schools located in Census tracts with higher levels of community opportunity. Additionally, charter schools tended to have higher OSS Black/white disparities than did non-charter schools (see Table 3).
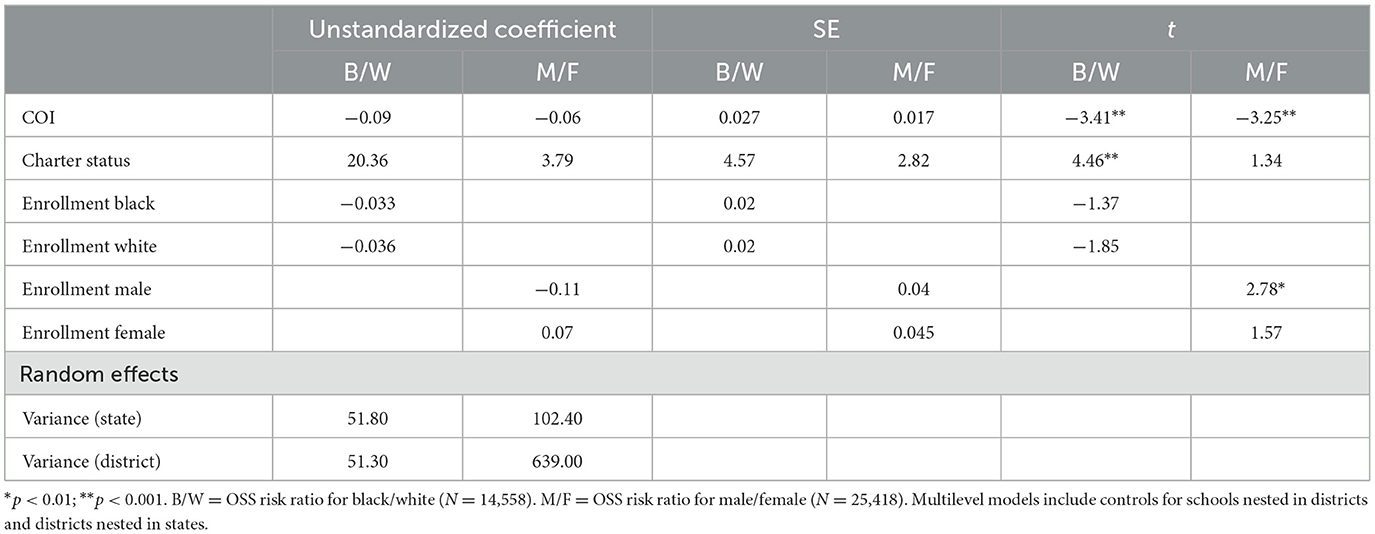
Table 3. Multilevel prediction of OSS risk ratios by COI: for black-white and male-female disparities.
For the multilevel linear regression for the school-level OSS risk-ratio analysis for male/female students, COI was again significantly and inversely related to the OSS risk-ratio outcome. Thus, for those Pre-K schools in Census tracts that had lower levels of community opportunity, the male/female disparity in the use of OSS was greater than for those Pre-K schools located in Census tracts with higher levels of community opportunity. Additionally, schools that enrolled more male students and those that were charter schools had greater levels of male/female disparities than those schools enrolling fewer male students or that were non-charter schools (see Table 3).
Discussion
Despite a robust literature on out-of-school suspensions (OSS) among K-12 populations, limited national estimates of OSS are available for publicly funded U.S. Pre-K schools. Moreover, knowledge of the role of neighborhood factors in the use of OSS is greatly understudied. The present research was designed to address these limitations by examining the relation of community opportunities to the use of OSS with Pre-K children enrolled in U.S. public schools. Using national data, we found that Pre-K schools located in low-opportunity neighborhoods were more likely to suspend young students than those schools located in neighborhoods that were relatively richer in the opportunities and resources they provide. Additionally, we found that the Black/white and male/female disparities in the use of OSS were greater in Pre-K schools located in lower- than higher-opportunity neighborhoods. These findings highlight the role of neighborhood context related to how Pre-K schools function and the link this has to the use of OSS with young students.
The use of out-of-school suspensions in U.S. public Pre-K schools
The sample used in the present research included more than 26,000 public Pre-K schools across the U.S., enrolling more than 1.26 million Pre-K students. Of these young students, about 2,600 were reported to be suspended at least once from U.S. public Pre-K schools. Across all Pre-K public schools in the U.S. in 2017–2018, there were about 2.5 Pre-K students suspended for every 1,000 enrolled.
The findings of this research study suggest that there is a pattern of harsh discipline that develops within certain Pre-K schools and the educational systems in which they are located. In support of this conclusion, Fabes et al. (2021a) compared rates of Pre-K suspensions and expulsions to those for K-12 students enrolled in the same school and found that the overall rates for K-12 were about 10 times higher than the rates for Pre-K. However, when Pre-K schools reported at least one case of suspension/expulsion, they did so at rates that were comparable to those of for K-12 students in the same school. Moreover, the suspension/expulsion rates for Pre-K students were significantly positively correlated with the rates for K-12 students. Thus, within a school there appears to be an ecology that affects policies and practices related to the use of discipline across all grades in that school. That rates were significantly correlated within a school suggest that there is likely a climate or culture that reinforces and promotes the use of exclusionary discipline with all of its students, including those who have yet to enter formal schooling (Huang and Cornell, 2018). Despite important differences in what is taught, the expectations for younger vs. older students, teacher qualities and experiences, peer group dynamics, and how students are evaluated, the tendency to use exclusionary discipline as a method of student discipline across all grades within a school emphasizes the need to understand factors beyond individual students and teachers and may reflect a broader set of factors that affect the public school systemically (Heilbrun et al., 2018; Kennedy et al., 2017), a notion we return to in the discussion related to the COI findings.
We also found racial and gender disparities in the use of OSS in Pre-K schools. In general, we found that the use of OSS in public Pre-K schools was disproportionally greater for Black students relative to white students and that this disparity was present when we looked at all schools as well as only those schools that reported at least one case of use of OSS in Pre-K (see Table 1). Such patterns were found despite the fact that Black students do not have worse or more severe behavior than white U.S. students (for example, see Anyon et al., 2014; Bradshaw et al., 2012).
For the gender disparities, our data revealed that boys were significantly more likely to be suspended than were their female classmates in Pre-K. This disparity also was found for all schools as well as when we looked only at schools that reported at least one case of OSS (see Table 1).
These findings are consistent with past research (Anyon et al., 2014; Skiba et al., 2016) and highlight the historical and ongoing inequities in the use of OSS. As such, it is critical to recognize that there are systemic legacies of racial and gender bias that relate to the use of OSS (and other forms of discipline) in U.S. public schools, including public Pre-K schools (Ispa-Landa, 2017; Skiba et al., 2011; Zulauf-McCurdy and Zinsser, 2021).
Community opportunity and the use of out-of-school suspensions in public Pre-K schools
As more children from disadvantaged backgrounds attend public Pre-K programs in the U.S., there has been a heightened interest in understanding the degree to which such attendance promotes greater equity in school readiness, performance, and success (Heckman, 2011). Much of the existing research in this area has focused on the qualities of the school, teachers, and/or students, but little is known about how the features that characterize the neighborhoods of Pre-K schools affect outcomes and processes associated with Pre-K education. The primary purpose of the present research was to examine the relation of the opportunities a community provides to its residents and the use of OSS in its local public Pre-K schools. Using the COI, a multi-dimensional measure of neighborhood-based conditions and resources (e.g., safe housing, access to parks and playgrounds, availability of healthy food, etc.; Acevedo-Garcia et al., 2014), we found that the use of OSS was related to neighborhood opportunities. Specifically, we found that relative to neighborhoods that provided children with more opportunities and resources, the use of OSS was greater in schools located in low-opportunity neighborhoods (accounting for nesting of schools in districts and districts in states). Additionally, the Black/white and male/female disparities noted above were greater in low- relative to high-opportunity neighborhoods. These results are consistent with research documenting the relation between adverse children's neighborhood qualities and disparities in the health and wellbeing for children and their families (Acevedo-Garcia et al., 2020a; Chetty et al., 2016).
Such findings add to the existing research base by supporting the notion that one of the ways in which neighborhood opportunity contributes to young children's outcomes is through the type of early disciplinary environments they experience in their neighborhood public Pre-K schools. Relative to young children who are not suspended, those who experience early suspension are likely to be less successful in school, hold negative attitudes about school, have higher dropout rates, and be involved in the juvenile justice systems (American Psychological Association Zero Tolerance Task Force, 2008; Petras et al., 2011). Additionally, other findings suggest that it is not just the suspended students in a classroom who are detrimentally affected by the use of exclusionary discipline, but those students who remain in the classroom are negatively affected. For instance, Perry and Morris (2014) found that in schools with high levels of suspensions, even well-behaved students who had never been suspended evidenced lowered academic performance. As such, Pre-K schools that are more likely to use OSS with their young students put all of their students at-risk for poor developmental outcomes.
Our findings showed that Pre-K schools in low-opportunity neighborhoods had higher rates of OSS and were more likely to use OSS than those Pre-K schools in high-opportunity neighborhoods. Such findings provide new evidence regarding one of the ways in which childhood disparities in Pre-K outcomes across neighborhoods may result. That these disparities were magnified for Black relative to white students and for male relative to female students, also highlight the many factors that exist within these neighborhood contexts that contribute to the disproportionality in the use of OSS and the disproportionate outcomes that result from these disparities.
One such factor pertains to the access to high-quality Pre-K programs. As noted previously, low-resourced communities that include relatively large numbers of people of color tend to offer Pre-K schools of lower quality than those schools that serve high-resourced predominantly white communities (Bassok and Galdo, 2016; Burchinal et al., 2008). For instance, Gillispie (2019) found that only 4% of Black children were enrolled in high-quality Pre-K schools. Additionally, an analysis of New York City's universal public Pre-K schooling found that Black children had providers of lower quality than their white peers (Latham et al., 2021). Compared to high-quality Pre-K schools, lower-quality schools tend to have fewer curricular supports, fewer teachers with training in early childhood education, less professional development for staff, larger class sizes, higher staff-child ratios, more teacher turnover and absenteeism, and lack age-appropriate learning and behavioral standards (Gillispie, 2019; NIEER, 2018). Additionally, parental stress, adversity, history of trauma, family illness or death, conflict, and divorce can exacerbate the child's behavior at preschool (Bufferd et al., 2011). Families in low-opportunity neighborhoods are more likely to experience these traumas and thus have children who might present a challenge to the teacher relative to those families in high-opportunity neighborhoods. Teachers in Pre-K schools in low-opportunity neighborhoods are also less likely to have access to early childhood mental health consultants, a factor that has been found to predict adverse outcomes in early childhood education settings and has been associated with disproportionally high rates of exclusionary discipline (Stegelin, 2018). Thus, Pre-K teachers in low-opportunity neighborhoods may be more likely to use exclusionary discipline because in part they lack the skills and resources to effectively help children with challenging behavior than teachers in high-opportunity neighborhoods (Hemmeter et al., 2008).
Relative to female students, we found that male students were disproportionately suspended. In the present findings, male Pre-K students were more likely to be suspended than their female peers even though there were approximately equal numbers of male and students enrolled (see Table 1). Such findings are consistent with a plethora of previous research and highlight the role that gender plays in school discipline (Fabes et al., 2021a; Leung-Gagné et al., 2022), even in Pre-K (Fabes et al., 2021b). In general, previous research has found that female students typically behave better in school whereas male students have been found to be more disruptive and aggressive in class (Bertrand and Pan, 2013). These behavioral differences, whether due to cultural or biological processes, lend themselves to greater suspensions for boys than girls. Additionally, conformity to gender stereotypes appears to play a role – the more a male student feels the need to conform to gender stereotypes the more likely they are to misbehave (Heyder et al., 2021). Teachers also perceive male students to be more disruptive than female students and may be more vigilant in attending to such behavior for their male students relative to their female students (Hares et al., 2022). Thus, the gender stereotypes and expectations educators hold about their students may put boys at a disproportionately greater risk for OSS.
Although Black and male students were disproportionately suspended from public Pre-K, these disparities were greater for Pre-K schools in low- relative to high-opportunity neighborhoods. As such, it appears that the conditions that contribute to the greater use of OSS within Pre-K schools also contribute to the racial and gender disparities found in the present study. Under conditions of concentrated disadvantage and low levels of neighborhood resources, children, parents, and educators find themselves living and working under stressful conditions and in adverse environments. Environmental stressors can influence teachers' perceptions of and responses to a student's behavior. For instance, Gion et al. (2018) found that implicit bias and the disproportionate use of OSS by teachers was greater at the end of the day when teachers were more stressed, tired, and fatigued than it was for the same behavior earlier in the day. Moreover, Sanchez (2022) found that the cumulative nature of stress and fatigue can increase implicit bias in the use of OSS. Specifically, Sanchez found that disproportionality in the use of OSS increased across the school year. Thus, the cumulative impact of environmental stress and the challenging work conditions for Pre-K teachers in schools located in low-opportunity neighborhoods can wear down school staff as the year progresses, thereby increasing implicit racial and gender bias and the risk of disproportionate use of OSS under these conditions.
Importantly, the disproportionate use of OSS creates conditions that can undermine students' safety, security, and sense of belonging at school. Black students enrolled in schools that had high levels of disproportionate OSS perceived their school to be less equitable, felt less school belonging, and had increased adjustment problems even after accounting for student demographics and school-level contextual factors (Bottiani et al., 2017). Such findings reflect the vicious cycle associated with the disproportionate use of OSS; the disenfranchised feelings reported by students who are subjected to disproportionate discipline may actually be a contributing factor to their discipline infractions (Sanchez, 2022). That OSS is more likely to be used in low-opportunity Pre-K schools appears to add to the set of conditions that support its biased and disproportionate use.
Conclusions
As noted previously, there is considerable research documenting the problems associated with concentrated neighborhood advantage/disadvantage (Catherine et al., 2024; Coulton et al., 1996). The current research adds to the extant research by focusing on the role of neighborhood advantage/disadvantage in the use of OSS with Pre-K students but does so using a more multidimensional assessment of neighborhood opportunity (e.g., the Child Opportunity Index 2.0; Acevedo-Garcia et al., 2014). Using national data, the results of this research suggest that OSS is more likely to occur and be used at higher rates in Pre-K schools in low- relative to high-opportunity neighborhoods. Additionally, the results revealed that disparities in the use of OSS for Black and male students were greater in low-opportunity Pre-K schools.
These findings are in line with other research on the use of OSS that has found that Black and male students are disproportionally likely to be suspended (Bottiani et al., 2017; Ispa-Landa, 2017; U. S. Government Accountability Office, 2018; Welsh and Little, 2018a). Fabes et al. (2024) explored these disproportionalities in depth and found strong evidence of consistency in across time, place, and a wide variety of school and district qualities. Fabes et al. (2024) also found that although there has been a general decline in suspension rates over the past decade or so, the disparities in the use of OSS remain generally at the same levels across this period.
Such findings suggest that when OSS is used, there is a strong tendency to use it inequitably (Zinsser and Wanless, 2020). Moreover, there is a growing need for research directed at understanding the ways in which schools might disrupt inequities in the use of OSS (see Sartain et al., 2015; Welsh et al., 2023). Using the sample of all U.S. public schools, Fabes et al. (2024) identified schools that stopped the use of OSS and noted that there were thousands that did so. Importantly, preliminary analyses suggested that these schools did not suffer negative consequences as a result of their decisions to stop using OSS. Thus, many schools were able to find alternative ways to manage classroom behavior that did not rely on removing students from school. One important avenue for further research is to gain a better understanding about these schools and how these schools addressed classroom management in ways that do not exclude students from the classroom.
As with any research, there are important limitations to consider when interpreting our findings. Although the sample of Pre-K public schools was large, the data were only available at the school level. As such, we could not determine how individual children were affected or differentially impacted by the use of OSS. Moreover, the results presented in this research are correlational and caution must be used to avoid drawing causal conclusions.
That only about 5% of public Pre-K schools reported using OSS may reflect a limitation as to how widespread the issues of OSS in Pre-K really are. Given that the data were obtained only from public Pre-K schools the findings tell us nothing about OSS in private Pre-K schools or other childcare settings, contexts where many children are placed in their early years (only about 40% of 3- to 5-year-olds were enrolled in public Pre-K programs in 2022; NCES, 2023). Additionally, the CRDC does not contain information about “soft suspensions” that are less formal than traditional suspensions (e.g., sending a child home early due to an incident related to behavior, requiring partial day attendance, etc.). As such, the counts and rates of OSS are likely to be underestimated, especially in a Pre-K setting where these “soft suspensions” are commonly used but not officially reported.
Despite these limitations, the findings of the present study add significantly to our understanding of the use of OSS and disparities in its use, in public Pre-K schools in the U.S. That the use of OSS in public Pre-K schools was found to be tied to the neighborhood resources in which the schools reside is a new finding that highlights the fact that one of the ways that neighborhoods influence children's health and wellbeing is through the disciplinary culture of its schools. The present findings also highlight that disparities in the use of OSS are tied to these neighborhood opportunities and conditions. To improve outcomes for children, these findings suggest that we must move beyond a focus on individual behaviors and school qualities and focus on changing neighborhood conditions and on reducing neighborhood inequities (Acevedo-Garcia et al., 2020b).
Data availability statement
The original contributions presented in the study are included in the article/supplementary material, further inquiries can be directed to the corresponding author.
Ethics statement
The studies involving humans were approved by Institutional Review Board, Arizona State University. The studies were conducted in accordance with the local legislation and institutional requirements. The Ethics Committee/Institutional Review Board waived the requirement of written informed consent for participation from the participants or the participants' legal guardians/next of kin because national data at school level, no child-level data included or identified.
Author contributions
RF: Conceptualization, Data curation, Formal analysis, Funding acquisition, Investigation, Methodology, Project administration, Resources, Supervision, Validation, Writing – original draft, Writing – review & editing. ZS: Data curation, Formal analysis, Writing – original draft, Writing – review & editing. EC: Conceptualization, Writing – review & editing. AM: Conceptualization, Writing – review & editing. SL: Conceptualization, Writing – review & editing. FX: Conceptualization, Writing – review & editing.
Funding
The author(s) declare financial support was received for the research, authorship, and/or publication of this article. This research was partially supported by the Administration for Children and Families (ACF) of the United States (U.S.) Department of Health and Human Services (HHS) as part of a financial assistance award [Grant #: (90Y#0122-01-00) totaling $95,790 with 25% funded by ACF/HHS and 75% funded by non-government source(s)]. A Spencer Grant (#202300167) also partially supported this research. The contents are those of the author(s) and do not necessarily represent the official views of, nor an endorsement, by ACF/HHS, the U.S. Government, or the Spencer Foundation. For more information, please visit the ACF website, Administrative and National Policy Requirements.
Conflict of interest
The authors declare that the research was conducted in the absence of any commercial or financial relationships that could be construed as a potential conflict of interest.
The author(s) declared that they were an editorial board member of Frontiers, at the time of submission. This had no impact on the peer review process and the final decision.
Publisher's note
All claims expressed in this article are solely those of the authors and do not necessarily represent those of their affiliated organizations, or those of the publisher, the editors and the reviewers. Any product that may be evaluated in this article, or claim that may be made by its manufacturer, is not guaranteed or endorsed by the publisher.
References
Acevedo-Garcia, D., McArdle, N., Hardy, E. F., Crisan, U. I., Romano, B., Norris, D., et al. (2014). The Child Opportunity Index: improving collaboration between community development and public health. Health Aff. 33, 1948–1957. doi: 10.1377/hlthaff.2014.0679
Acevedo-Garcia, D., Noelke, C., and McArdle, N. (2020a). The Geography of Child Opportunity: Why Neighborhoods Matter for Equity. Available at: http://diversitydatakids.org/sites/default/files/file/ddk_the-geography-of-child-opportunity_2020v2_0.pdf (accessed June 1, 2022).
Acevedo-Garcia, D., Noelke, C., McArdle, N., Sofer, N., Hardy, E. F., Weiner, M., et al. (2020b). Racial and ethnic inequities in children's neighborhoods: evidence from the new Child Opportunity Index 2.0. Health Aff. 39, 1693–1701. doi: 10.1377/hlthaff.2020.00735
American Academy of Pediatrics Committee on School Health (2013). Out-of-school suspension and expulsion. Pediatrics 131, 1206–1209. doi: 10.1542/peds.112.5.1206
American Psychological Association Zero Tolerance Task Force (2008). Are zero tolerance policies effective in the schools?: an evidentiary review and recommendations. Am. Psychol. 63, 852–862. doi: 10.1037/0003-066X.63.9.852
Andrew, M., and Blake, M. K. (2023). The long arm of early exclusionary school discipline? A multi-model analysis. Youth Soc. 55, 238–258. doi: 10.1177/0044118X211042643
Anscombe, F. J. (1956). On estimating binomial response relations. Biometrika 43, 461–464. doi: 10.1093/biomet/43.3-4.461
Anyon, Y., Jenson, J. M., Altschul, I., Farrar, J., McQueen, J., Greer, E., et al. (2014). The persistent effect of race and the promise of alternatives to suspension in school discipline outcomes. Child. Youth Serv. Rev. 44, 379–386. doi: 10.1016/j.childyouth.2014.06.025
Baker, B. D., Weber, M., Srikanth, A., Kim, R., and Atzbi, M. (2018). The Real Shame of the Nation: The Causes and Consequences of Interstate Inequity in Public School Investments. Available at: https://www.shankerinstitute.org/sites/default/files/The%20Real%20Shame%20of%20the%20Nation.pdf (accessed June 1, 2022).
Banghart, P., Halle, T., Cook, M., Redd, Z., Cox, A., and Carlson, J. (2020). A Review of the Literature on Access to High-Quality Care for Infants and Toddlers. Available at: https://www.childtrends.org/wp-content/uploads/2020/05/HighQualityCareLitReview_ChildTrends_May2020.pdf~2022/01/26/ (accessed June 1, 2022).
Bassok, D., and Galdo, E. (2016). Inequality in preschool quality? Community-level disparities in access to high-quality learning environments. Early Educ. Dev. 27, 128–144. doi: 10.1080/10409289.2015.1057463
Bates, D., Mächler, M., Bolker, B., and Walker, S. (2015). Fitting linear mixed-effects models using lme4. J. Stat. Softw. 67, 1–48. doi: 10.18637/jss.v067.i01
Bertrand, M., and Pan, J. (2013). The trouble with boys: social influences and the gender gap in disruptive behavior. Am. Econ. J. 5, 32–64. doi: 10.1257/app.5.1.32
Bottiani, J. H., Bradshaw, C. P., and Mendelson, T. (2017). A multilevel examination of racial disparities in high school discipline: black and white adolescents' perceived equity, school belonging, and adjustment problems. J. Educ. Psychol. 109, 532–545. doi: 10.1037/edu0000155
Bradshaw, C. P., Waasdorp, T. E., and Leaf, P. J. (2012). Effects of school-wide positive behavioral interventions and supports on child behavior problems. Pediatrics 130, e1136–e1145. doi: 10.1542/peds.2012-0243
Browning, M. H. E. M., and Rigolon, A. (2019). School green space and its impact on academic performance: a systematic literature review. Int. J. Environ. Res. Public Health 16:429. doi: 10.3390/ijerph16030429
Bufferd, S. J., Dougherty, L. R., Carlson, G. A., and Klein, D. N. (2011). Parent-reported mental health in preschoolers: findings using a diagnostic interview. Compr. Psychiatry 52, 359–369. doi: 10.1016/j.comppsych.2010.08.006
Burchinal, M., Nelson, L., Carlson, M., and Brooks-Gunn, J. (2008). Neighborhood characteristics and child care type and quality. Early Educ. Dev. 19, 702–725. doi: 10.1080/10409280802375273
Catherine, E., Alexander, B., Iruka, I. U., Meek, S., and Edwards, M. C. (2024). Review of Policy Effects on Black Families and Children: Advancing the Black Child National Agenda. Available at: https://cep.asu.edu/resources/AdvancingBlackChild-NationalAgenda (accessed March 15, 2024).
Center for Learner Equity (2021). Technical Brief 4: School Discipline and Engagement of Law Enforcement in Charter and Traditional Public Schools. Available at: https://www.centerforlearnerequity.org/wp-content/uploads/CLE-CRDC_FINAL-Brief-4a.pdf (accessed June 1, 2022).
Chetty, R., Hendren, N., Jones, M. R., and Porter, S. R. (2019). Race and economic opportunity in the United States: an intergenerational perspective. Q. J. Econ. 135, 711–783. doi: 10.1093/qje/qjz042
Chetty, R., Hendren, N., and Katz, L. F. (2016). The effects of exposure to better neighborhoods on children: new evidence from the moving to opportunity experiment. Am. Econ. Rev. 106, 855–902. doi: 10.1257/aer.20150572
Chow, K. A., Gaylor, E., Grindal, T., Tunzi, D., Wei, X., and Tiruke, T. (2021). Associations of teacher characteristics with preschool suspensions and expulsions: implications for supports. Child. Youth Serv. Rev. 129:106162. doi: 10.1016/j.childyouth.2021.106162
Clemens, N., McArdle, N., Baek, M., NHuntington, N., Huber, R., Hardy, E., et al. (2020). Child Opportunity Index. Available at: http://www.diversitydatakids.org/sites/default/files/2020-02/ddk_coi2.0_technical_documentation_20200212.pdf (accessed June 1, 2022).
Coulton, C. J., Chow, J., Wang, E. C., and Su, M. (1996). Geographic concentration of affluence and poverty in 100 metropolitan areas, 1990. Urban Affairs Rev. 32, 186–216.
Dupere, V., Leventhal, T., Crosnoe, R., and Dion, E. (2010). Understanding the positive role of neighborhood socioeconomic advantage in achievement: the contribution of the home, child care, and school environments. Dev. Psychol. 46, 1227–1244. doi: 10.1037/a0020211
Fabes, R. A., Catherine, E., McDonald, A., and O'Rourke, H. (2024). Exclusionary Discipline in U.S. Public Schools: An Interactive Data Story of Change and Variation in its Use, Disproportionality, and Impact Over Time. Available at: https://pedstudy.org/data-story (accessed March 15, 2024).
Fabes, R. A., Catherine, E., Quick, M., Blevins, D., and Musgrave, A. (2021a). The price of punishment: days missed due to suspension in U.S. K-12 public schools. Psychol. Sch. 58, 1980–1994. doi: 10.1002/pits.22565
Fabes, R. A., Quick, M., Catherine, E., and Musgrave, A. (2021b). Exclusionary discipline in U. S. public schools: a comparative examination of use in pre-kindergarten and K-12 grades. Educ. Stud. 3, 1–18. doi: 10.1080/03055698.2021.1941782
Geverdt, D. (2018). Education Demographic and Geographic Estimates (EDGE). Geocodes: Public schools and local education agencies, 2016–2017 (NCES 2018-080). Available at: https://nces.ed.gov/programs/edge/docs/EDGE_GEOCODE_PUBLIC1617_FILEDOC.pdf (accessed June 1, 2022).
Gilliam, W. S. (2005). Prekindergarteners Left Behind: Expulsion Rates in State Prekindergarten Programs. Available at: https://challengingbehavior.cbcs.usf.edu/docs/prekindergarteners-left-behind_expulsion-in-state-programs.pdf (accessed June 1, 2022).
Gilliam, W. S., and Shahar, G. (2006). Prekindergarten expulsion and suspension. Infants Young Child. 19, 228–245. doi: 10.1097/00001163-200607000-00007
Gillispie, C. (2019). Young Learners, Missed Opportunities: Ensuring That Black and Latino childRen Have Access to High-Quality State-Funded Preschool. Available at: https://edtrust.org/wp-content/uploads/2014/09/Young-Learners-Missed-Opportunities.pdf (accessed June 1, 2022).
Gion, C., McIntosh, K., and Smolkowski, K. (2018). Examination of American indian/alaska native school discipline disproportionality using the vulnerable decision points approach. Behav. Disord. 44, 40–52. doi: 10.1177/0198742918773438
Haldane, J. B. S. (1940). The mean and variance of the moments of chi-squared when used as a test of homogeneity, when expectations are small. Biometrika 29, 133–134. doi: 10.2307/2332411
Hares, S., Nagesh, R., and Konate, M. (2022). Was Your Teacher Sexist? Teacher Beliefs and Student Outcomes. Available at: https://www.cgdev.org/blog/was-your-teacher-sexist-teacher-beliefs-and-students-outcomes (accessed June 1, 2022).
Hatfield, B. E., Burchinal, M. R., Pianta, R. C., and Sideris, J. (2016). Thresholds in the association between quality of teacher–child interactions and preschool children's school readiness skills. Early Child. Res. Q. 36, 561–571. doi: 10.1016/j.ecresq.2015.09.005
Heckman, J. J. (2011). The economics of inequality: the value of early childhood education. Am. Educ. 35, 31–35, 47.
Heilbrun, A., Cornell, D., and Konold, T. (2018). Authoritative school climate and suspension rates in middle schools: implications for reducing the racial disparity in school discipline. J. Sch. Violence 17, 324–338. doi: 10.1080/15388220.2017.1368395
Hemmeter, M. L., Santos, R. M., and Ostrosky, M. M. (2008). Preparing early childhood educators to address young children's social-emotional development and challenging behavior: a survey of higher education programs in nine states. J. Early Interv. 30, 321–340. doi: 10.1177/1053815108320900
Heyder, A., van Hek, M., and Van Houtte, M. (2021). When gender stereotypes get male adolescents into trouble: a longitudinal study on gender conformity pressure as a predictor of school misconduct. Sex Roles 84, 61–75. doi: 10.1007/s11199-020-01147-9
Huang, F. L., and Cornell, D. (2018). The relationship of school climate with out-of-school suspensions. Child. Youth Serv. Rev. 94, 378–389. doi: 10.1016/j.childyouth.2018.08.013
Ingersoll, R. M., Merrill, E., Stuckey, D., and Collins, G. (2018). Seven Trends: The Transformation of the Teaching Force - Updated October 2018. CPRE Research Reports., Issue. Available at: https://repository.upenn.edu/cpre_researchreports/108/ (accessed June 1, 2022).
Ispa-Landa, S. (2017). Racial and gender inequality and school discipline: toward a more comprehensive view of school policy. Soc. Curr. 4, 511–517. doi: 10.1177/2329496517704876
Kennedy, B. L., Murphy, A. S., and Jordan, A. (2017). Title I middle school administrators' beliefs and choices about using corporal punishment and exclusionary discipline. Am. J. Educ. 123, 243–280. doi: 10.1086/689929
Kim, Y., Lee, S., Jung, H., Jaime, J., and Cubbin, C. (2019). Is neighborhood poverty harmful to every child? Neighborhood poverty, family poverty, and behavioral problems among young children. J. Commun. Psychol. 47, 594–610. doi: 10.1002/jcop.22140
Kiziltaş, E., and Sak, R. (2018). Integrating field-trip activities with other activities in the preschool curriculum: its effects on the preschoolers' social–emotional skills. Int. J. Child Care Educ. Policy 12:8. doi: 10.1186/s40723-018-0047-0
Lambert, D. (1992). Zero-inflated poisson regression, with an application to defects in manufacturing. Technometrics 34, 1–14. doi: 10.2307/1269547
Latham, S., Corcoran, S. P., Sattin-Bajaj, C., and Jennings, J. L. (2020). Racial Disparities in Pre-k Quality: Evidence From New York City's Universal Pre-k Program. Available at: https://www.edworkingpapers.com/sites/default/files/ai20-248.pdf (accessed June 1, 2022).
Latham, S., Corcoran, S. P., Sattin-Bajaj, C., and Jennings, J. L. (2021). Racial disparities in pre-k quality: evidence from New York City's universal pre-k program. Educ. Res. 50, 607–617. doi: 10.3102/0013189X211028214
Leung-Gagné, M., McCombs, J., Scott, C., and Losen, D. J. (2022). Pushed Out: Trends and Disparities in Out-of-School Suspension. Available at: https://learningpolicyinstitute.org/product/crdc-school-suspension-report (accessed June 1, 2020).
Leventhal, T., and Brooks-Gunn, J. (2000). The neighborhoods they live in: the effects of neighborhood residence on child and adolescent outcomes. Psychol. Bull. 126, 309–337. doi: 10.1037/0033-2909.126.2.309
Leventhal, T., Dupéré, V., and Shuey, A. E. (2015). “Children in neighborhoods,” in Handbook of Child Psychology and Developmental Science, ed. R. M. Lerner (Hoboken, NJ: Wiley), 1–41.
Malik, R. (2017). New Data Reveal 250 Preschoolers are Suspended or Expelled Every Day. Available at: https://www.americanprogress.org/issues/early-childhood/news/2017/11/06/442280/new-data-reveal-250-preschoolers-suspended-expelled-every-day/ (accessed June 1, 2022).
McCoy, D. C., Sabol, T. J., Wei, W., Busby, A., and Hanno, E. C. (2023). Pushing the boundaries of education research: a multidimensional approach to characterizing preschool neighborhoods and their relations with child outcomes. J. Educ. Psychol. 115, 143–159. doi: 10.1037/edu0000728
McCulloch, A., and Joshi, H. E. (2001). Neighbourhood and family influences on the cognitive ability of children in the British national child development study. Soc. Sci. Med. 53, 579–591. doi: 10.1016/S0277-9536(00)00362-2
McIntosh, K., Girvan, E. J., Fairbanks Falcon, S., McDaniel, S. C., Smolkowski, K., Bastable, E., et al. (2021). Equity-focused PBIS approach reduces racial inequities in school discipline: a randomized controlled trial. Sch. Psychol. 36, 433–444. doi: 10.1037/spq0000466
Minh, A., Muhajarine, N., Janus, M., Brownell, M., and Guhn, M. (2017). A review of neighborhood effects and early child development: how, where, and for whom, do neighborhoods matter? Health Place 46, 155–174. doi: 10.1016/j.healthplace.2017.04.012
Morris, E. W., and Perry, B. L. (2016). The punishment gap: school suspension and racial disparities in achievement. Soc. Probl. 63, 68–86. doi: 10.1093/socpro/spv026
Mullahy, J. (1986). Specification and testing of some modified count data models. J. Econom. 33, 341–365. doi: 10.1016/0304-4076(86)90002-3
National Institute for Early Education Resarch (2022). The State of Preschool 2021. Available at: https://nieer.org/wp-content/uploads/2022/09/YB2021_Full_Report.pdf (accessed June 1, 2022).
NCES (2020). NCES blog. Available at: https://nces.ed.gov/blogs/nces/2020/11/24/default (accessed June 1, 2022).
NCES (2023). Digest of Education Statistics. Available at: https://nces.ed.gov/programs/coe/indicator/cfa/enrollment-of-young-children (accessed March 15, 2024).
Newburger, H. B., Birch, E. L., and Wachter, S. M. (2011). Neighborhood and Life Chance: How Place Matters in Modern America. Philadelpha, PA: University of Pennsylvania Press.
NIEER (2018). Implementing 15 Essential Elements for High-Quality Pre-k: An Updated Scan of State Policies. Available at: https://nieer.org/sites/default/files/2023-08/Essential-Elements-FINAL-9.14.18.pdf (accessed June 1, 2022).
Noelke, C., McArdle, N., Huntington, N., Huber, R., and Acevedo-Garcia, D. (2020). Child Opportunity Index 2.0: Technical documentation. Available at: https://www.diversitydatakids.org/sites/default/files/2020-02/ddk_coi2.0_technical_documentation_20200212.pdf (accessed June 1, 2022).
Orfield, G., and Frankenberg, E. (2014). Increasingly segregated and unequal schools as courts reverse policy. Educ. Administr. Q. 50, 718–734. doi: 10.1177/0013161X14548942
Owens, A. (2010). Neighborhoods and schools as competing and reinforcing contexts for educational attainment. Sociol. Educ. 83, 287–311. doi: 10.1177/0038040710383519
Owens, A., and Candipan, J. (2019). Social and spatial inequalities of educational opportunity: a portrait of schools serving high- and low-income neighbourhoods in us metropolitan areas. Urban Stud. 56, 3178–3197. doi: 10.1177/0042098018815049
Perry, B. L., and Morris, E. W. (2014). Suspending progress: collateral consequences of exclusionary punishment in public schools. Am. Sociol. Rev. 79, 1067–1087. doi: 10.1177/0003122414556308
Petras, H., Masyn, K. E., Buckley, J. A., Ialongo, N. S., and Kellam, S. (2011). Who is most at risk for school removal? A multilevel discrete-time survival analysis of individual- and context-level influences. J. Educ. Psychol. 103, 223–237. doi: 10.1037/a0021545
R Core Team (2024). R: A Language and Environment for Statistical Computing. R Foundation for Statistical Computing. Available at: https://www.R-project.org (accessed June 1, 2022).
Ruxton, G. D., and Neuhäuser, M. (2013). Review of alternative approaches to calculation of a confidence interval for the odds ratio of a 2 × 2 contingency table. Methods Ecol. Evol. 4, 9–13. doi: 10.1111/j.2041-210x.2012.00250.x
Sanchez, J. P. (2022). Snap Judgements: Vulnerable Decision Points Leading to Disproportionate Discipline (Publication Number AAI28542615) (D.Ed., University of Oregon). APA PsycInfo®. Available at: https://www-proquest-com.ezproxy1.lib.asu.edu/docview/2572564882?sourcetype=Dissertations%20&%20Theses (accessed June 1, 2022).
Sartain, L., Allensworth, E. M., and Porter, S. (2015). Suspending Chicago's Students: Differences in Discipline Practices Across Schools. Available at: https://consortium.uchicago.edu/sites/default/files/2018-10/Suspending%20Chicagos%20Students.pdf (accessed June 1, 2022).
Sege, R. D., and Siegel, B. S. (2018). Effective discipline to raise healthy children. Pediatrics 142:e20183112. doi: 10.1542/peds.2018-3112
Skiba, R. J., Arredondo, M. I., Gray, C., and Rausch, M. K. (2016). “What do we know about discipline disparities? New and emerging research,” in Inequality in School Discipline: Research and Practice to Reduce Disparities, eds. R. J. Skiba, K. Mediratta, and M. K. Rausch (Palgrave Macmillan US), 21–38.
Skiba, R. J., Horner, R. H., Chung, C.-G., Rausch, M. K., May, S. L., and Tobin, T. (2011). Race is not neutral: a national investigation of african American and Latino disproportionality in school discipline. School Psych. Rev. 40, 85–107. doi: 10.1080/02796015.2011.12087730
Skiba, R. J., and Losen, D. J. (2016). From reaction to prevention: turn the page on school discipline. Am. Educ. 4–11.
Skiba, R. J., and Rausch, M. K. (2006). “Zero tolerance, suspension and expulsion: questions of equity and effectiveness,” in Handbook of Classroom Management: Research, Practice and Contemporary Issues, eds. C. M. Evertson, and C. S. Weinstein (Hillsdale, NJ: Erlbaum), 1063–1089.
Stegelin, D. A. (2018). Preschool Suspensions and Expulsion: Defining the Issues. Available at: https://www.instituteforchildsuccess.org/wp-content/uploads/2018/12/ICS-2018-PreschoolSuspensionBrief-WEB.pdf (accessed June 1, 2022).
Sundius, J., and Farneth, M. (2008). Putting Kids Out of School: What's Causing High Suspension Rates and Why They Are Detrimental to Students, Schools, and Communities. Available at: https://www.opensocietyfoundations.org/uploads/fc2dea1b-4260-433e-a86e-e2f5ee8fdf68/whitepaper2_20080919.pdf (accessed June 1, 2022).
U. S. Department of Education (2016). 2013-2014 Civil Right Data Collection: A First Look. Available at: https://ocrdata.ed.gov/assets/downloads/2013-14-first-look.pdfeism.html (accessed June 1, 2022).
U. S. Department of Education (2020). Civil Rights Data Collection (CRDC) for the 2017-2018 School Year. Available at: https://www2.ed.gov/about/offices/list/ocr/docs/crdc-2017-18.html (accessed June 1, 2022).
U. S. Government Accountability Office (2018). Discipline Disparities for Black Students, Boys, and Students With Disabilities. Washington, DC: U.S. Goverment Accountability Office. Available at: https://www.gao.gov/products/GAO-18–258 (accessed June 1, 2022).
Valentino, R. (2018). Will public pre-k really close achievement gaps? Gaps in prekindergarten quality between students and across states. Am. Educ. Res. J. 55, 79–116. doi: 10.3102/0002831217732000
Wei, W. S., McCoy, D. C., Busby, A. K., Hanno, E. C., and Sabol, T. J. (2021). Beyond neighborhood socioeconomic status: exploring the role of neighborhood resources for preschool classroom quality and early childhood development. Am. J. Community Psychol. 67, 470–485. doi: 10.1002/ajcp.12507
Weiland, C., Ulvestad, K., Sachs, J., and Yoshikawa, H. (2013). Associations between classroom quality and children's vocabulary and executive function skills in an urban public prekindergarten program. Early Child. Res. Q. 28, 199–209. doi: 10.1016/j.ecresq.2012.12.002
Welsh, R. O., and Little, S. (2018a). Caste and control in schools: a systematic review of the pathways, rates and correlates of exclusion due to school discipline. Child. Youth Serv. Rev. 94, 315–339. doi: 10.1016/j.childyouth.2018.09.031
Welsh, R. O., and Little, S. (2018b). The school discipline dilemma: a comprehensive review of disparities and alternative approaches. Rev. Educ. Res. 88, 752–794. doi: 10.3102/0034654318791582
Welsh, R. O., Rodriguez, L. A., and Joseph, B. B. (2023). Beating the school discipline odds: conceptualizing and examining inclusive disciplinary schools in New York city. Sch. Eff. Sch. Improv. 34, 271–297. doi: 10.1080/09243453.2023.2182795
Williams P. G. Yogman M. Council on Early Childhood Committee on Psychosocial Aspects of Child Family Health (2023). Addressing early education and child care expulsion. Pediatrics 152:e064049. doi: 10.1542/peds.2023-064049
Witherspoon, D. P., White, R. M. B., Bámaca, M. Y., Browning, C. R., Leech, T. G. J., Leventhal, T., et al. (2023). Place-based developmental research: conceptual and methodological advances in studying youth development in context. Monogr. Soc. Res. Child Dev. 88, 7–130. doi: 10.1111/mono.12472
Zeileis, A., Kleiber, C., and Jackman, S. (2008). Regression models for count data in R. J. Stat. Softw. 27, 1–25. doi: 10.18637/jss.v027.i08
Zeng, S., Corr, C. P., O'Grady, C., and Guan, Y. (2019). Adverse childhood experiences and preschool suspension expulsion: a population study. Child Abuse Negl. 97:104149. doi: 10.1016/j.chiabu.2019.104149
Zinsser, K. M. (2023). No Longer Welcome: The Epidemic of Expulsion From Early Childhood Education. Oxford: Oxford University Press.
Zinsser, K. M., and Wanless, S. B. (2020). “Racial disproportionality in the school-to-prison pipeline,” in The Legacy of Racism for Children: Psychology, Law, and Public Policy, eds. M. C. Stevenson, B. L. Bottoms, and K. C. Burke (Oxford: Oxford University Press), 129–149, 264.
Keywords: pre-kindergarten children, pre-kindergarten education, suspension, discipline, neighborhood, disparities
Citation: Fabes RA, Shen Z, Catherine E, McDonald A, Low S and Xie F (2024) Relations of the use of out-of-school suspensions in U.S. public pre-kindergarten programs to neighborhood opportunity. Front. Dev. Psychol. 2:1479290. doi: 10.3389/fdpys.2024.1479290
Received: 11 August 2024; Accepted: 25 November 2024;
Published: 20 December 2024.
Edited by:
Sara Schmitt, University of Oregon, United StatesReviewed by:
Charles Beekman, Independent Researcher, SwitzerlandRobert Duncan, Colorado State University, United States
Copyright © 2024 Fabes, Shen, Catherine, McDonald, Low and Xie. This is an open-access article distributed under the terms of the Creative Commons Attribution License (CC BY). The use, distribution or reproduction in other forums is permitted, provided the original author(s) and the copyright owner(s) are credited and that the original publication in this journal is cited, in accordance with accepted academic practice. No use, distribution or reproduction is permitted which does not comply with these terms.
*Correspondence: Richard A. Fabes, cmZhYmVzQGFzdS5lZHU=