- Centre of Behavioral and Cognitive Sciences, University of Allahabad, Prayagraj, Uttar Pradesh, India
Introduction: Children transition from engaging control reactively as per the demands of a given situation, to using a proactive mode of control as an automatic response in all situations. Yet, it is crucial to study the age at which this transition takes place and to understand the development of the ability of flexible shifts in modes of control.
Methods: To investigate developmental changes in this ability of children and its relationship with school readiness and academic achievement, children in the continuous age groups of 5, 6–8, and 9–11 years performed a cued switching task. The task conditions were manipulated such that in the case of “Proactive Impossible” condition, only reactive control was available; in the case of “Proactive Encouraged” condition, only proactive control was available; and in the case of “Proactive Possible” condition, both proactive and reactive control could be used.
Results: A clear preference for reactive control was evident in 5-year-old children, along with the ability to engage in proactive control when encouraged. Nine to eleven-year-old children favored proactive control as their default mode. However, 6–8-year-olds displayed flexibility in shifting between control modes, compared to the other two age groups. This ability correlated with school readiness and academic achievement.
Discussion: These findings emphasize that 6–8 years of age marks the developmental period for flexible cognitive control, with the benefits of having access to and utilizing both control modes and their relationship with academic achievement.
Introduction
Children exercise cognitive control in a variety of ways in everyday life. Whether it is paying attention and following instructions in school, regulating their screen time, solving mathematical problems, participating in creative play, organizing their desk, or accompanying parents for grocery shopping, children are constantly utilizing different aspects of control. These aspects of control are often used flexibly depending on the ever-changing environment (Coldren, 2013; Chevalier, 2015). As children grow older, their repertoire of control strategies also expands. The present study investigates whether children possess the ability to flexibly shift between proactive and reactive modes of cognitive control, despite having a preference for a particular control mode. It is notable that the development of cognitive control is driven by qualitative changes when new control strategies emerge with age, i.e., new ways to implement or integrate executive processes, demonstrating greater flexibility in utilizing the expanding repertoire of control strategies (Munakata et al., 2012; Lucenet and Blaye, 2014; Chevalier, 2015).
Cognitive control, usually defined as flexible goal-directed regulation of thoughts and actions, is divided into two distinct control mechanisms in the Dual Mechanism of Control (DMC) framework: proactive control and reactive control (Braver, 2012). However, which of the two modes of control is more adaptable largely depends on the demands of the task. While proactive control usually works on an early selection mechanism involving active maintenance of goal-relevant information in working memory to better guide one's behavior at task onset, reactive control, on the other hand, works on a late correction mechanism where one waits for a control-demanding event to occur for detection and resolution of interference (Braver et al., 2009).
The development of cognitive control begins in infancy and is rapid during the first decade of life, transitioning from reliance on reactive control to proactive control. Research indicates that younger children respond to situations more reactively, even with the ability to engage proactive control if required, however, at the cost of their performance and efficiency. On the other hand, older children lean on proactive control whenever possible in all situations (Chatham et al., 2009; Chevalier et al., 2015; Gonthier et al., 2019; Yeshua and Berger, 2024). Notably, young children may rely on reactive control because of limited cognitive resources, such as working memory capacity, making it difficult to actively maintain task-relevant information long enough to engage proactive control (Troller-Renfree et al., 2020).
Previously, proactive and reactive control were represented as opposite poles on a continuum (Chiew and Braver, 2017), suggesting that using one mode would restrict the use of the other and vice versa, implying a strong dependency between the two modes of control. However, more recent research has challenged this “continuous process” account and suggested that the two modes of control are, in principle, functionally independent. This account referred to as the “independent processes” account (Mäki-Marttunen et al., 2019; Yeshua and Berger, 2024), postulates that the recruitment of one control mode would not necessarily hinder the use of the other control mode; rather, both can operate simultaneously and can be recruited depending on the circumstances. Further, this account emphasizes that proactive and reactive control modes have separate information-processing mechanisms, and they could coexist. If we consider the “independent processes” account, then both the modes of cognitive control can operate independently and simultaneously. Although the evidence supporting the co-existence of proactive and reactive control has been observed in adults and not in preschool- and kindergarten-aged children (3–5 years of age), when tested through measures of post-error slowing (marker of reactive control) and delayed disinhibition (marker of proactive control) suggesting a gradual maturation of cognitive control modes (Yeshua and Berger, 2024). Therefore, we intended to examine if children in the age range of 5–11 years would show reliance on either/both proactive and reactive control depending on the demands of the task.
Researchers have extensively discussed the developmental shift from reactive to proactive mode of cognitive control among children (Chatham et al., 2009; Chevalier et al., 2015) and the benefits of proactive control across various cognitive functions such as better preparedness during critical times, improved performance monitoring, increased metacognitive awareness or improved math ability (Blackwell and Munakata, 2014; Hadley et al., 2020; Niebaum et al., 2021; Wang et al., 2021). However, there is limited evidence about whether both modes can be operated simultaneously and can be used flexibly. It remains unclear whether the ability to shift between control modes offers any advantage beyond the use of proactive control itself. More specifically, understanding if this flexibility offers a unique advantage and determining whether promoting such adaptability at an early age is advantageous could be valuable. Indeed, children's inclination toward a specific mode of control prompts a crucial question: Whether it is beneficial to depend on a particular control mode or adapt control processes to the task demands? Flexibility in cognitive control largely examined in terms of shifting or switching ability has been found to be linked with arithmetic problem-solving in primary school children, indicating that the ability to flexibly shift between strategies and mental sets is critical for solving arithmetic word problems (Arán Filippetti and Richaud, 2016), mathematical achievement (Rosselli et al., 2009), reading comprehension and writing skills by enabling flexible thinking, engaging working memory for appropriate word generation and accessing semantic and phonological knowledge (Arán Filippetti and Krumm, 2020; Johann et al., 2020) and creativity (Zabelina and Robinson, 2010). These studies provided the motivation to explore how flexibility in the context of shifting between modes of cognitive control might benefit academic achievement or problem-solving abilities.
The present study aims to examine developmental changes in the engagement of proactive and reactive modes of cognitive control in children. Given the clear preferences of younger and older children toward a particular control mode, the first objective of the study is to explore the age group which marks the transition from reactive to proactive mode of cognitive control. For this reason, we used a continuous age group (5, 6–8- and 9–11 years). This age range is also supported by previous studies which suggest that children around 3–5 years predominantly choose a reactive mode of control, while those above 6 years are more inclined to use a proactive mode of control (Chatham et al., 2009; Chevalier et al., 2015; Elke and Wiebe, 2017). A cued-switching task (Chevalier et al., 2015) was employed to measure proactive and reactive control engagement in children. The second objective of the study was to explore whether children can flexibly shift between cognitive control modes depending on the demands of the task. To achieve this objective, we manipulated the task conditions based on the timing of cue presentation (Chevalier et al., 2015), which enables the participant to either employ only reactive control (“Proactive Impossible” Condition), only proactive control (“Proactive Encouraged” Condition) or have the possibility to choose between the two (“Proactive Possible” Condition) control modes. The Third objective of the study was to find out if flexibility in cognitive control modes would be associated with academic achievement. In the present study, academic achievement was measured through assessments of school readiness for 5-year-olds and English and Hindi reading and writing performance for 6–11-year-olds.
Building on prior research, we hypothesized that younger children would be inclined toward reactive control, while older children would be inclined toward proactive control. We anticipated that the proactive possible condition (PPC) (where both control modes are available), in comparison to the other two conditions, would provide insight into whether children could flexibly shift between cognitive control modes, and if so, at what age this occurred. We did not have a specific age-related hypothesis however flexible shift between cognitive control modes was expected in older children and this adaptability to correlate with their academic achievement. Previous literature has emphasized the importance of use of both proactive (Kubota et al., 2020) and reactive control (Valiente et al., 2011; Hernández et al., 2017) modes in children's academic achievement separately. Both control modes offer their own unique advantage to academic achievement, such as the ability to maintain online information, enhanced preparedness in various situations, and improved working memory.
Methods
Power analysis
To determine the appropriate sample size for our study, we conducted a power analysis using MorePower 6.0 (Campbell and Thompson, 2012). Based on prior work (Chevalier et al., 2015), we considered an effect size of = 0.079 with 0.8 power, resulting in a necessary sample size of N = 72.
Participants
A total of 94 children participated in the study with 30, 5-year-old children (15 boys and 15 girls), 31 children in the age range of 6–8-years (mean age = 7.37; SD = 0.71; 16 boys and 15 girls), and 33 children in the range of 9–11-years (mean age = 9.78; SD = 0.83; 17 boys and 16 girls). The participants were taken from four co-education schools in Prayagraj with English as the medium of instruction. Permission was obtained from school authorities, and written informed consent was obtained from each participant's parents. Demographic information such as age, grade (5-year-olds: Pre-Primary Stage; 6–8-year-olds: Grade-I to Grade-III; 9–11-year-olds: Grade-II to Grade-V), socioeconomic status (Kuppuswamy's SES scale; Ayoub and Raja, 2023) (9.57% belonging to Lower Middle Class, 60.64% belonging to Upper Middle Class and 29.79% belonging to Upper class), and languages known/spoken (all the participants were native Hindi speakers and started to learn English with formal schooling) were recorded. Raven's (2012) Colored Progressive Matrices (CPM) were administered as a measure of fluid intelligence and the participants obtained standard scores ranging from 90 to 120; Mean = 101.56; SD = 8.91 (82.98% with average and 17.02% with above average level of intelligence) (Table 1). Seven participants, including three with superior level of intelligence and four with low average or below, level of intelligence, were excluded from the study. One participant was diagnosed with ADHD as reported by the school counselor and was therefore excluded from the study. These eight participants, distributed across the three age-groups, were in addition to the 94 participants who participated in the study. For LISAS analysis, inclusion criterion was a minimum of 75% accuracy in the cued-switching task and none of the 94 participants were excluded based on this criterion. For IES analysis (N = 82), inclusion criterion was a minimum of 90% accuracy, resulting in the exclusion of 12 participants. The study was approved by the Institutional Ethics Review Board (IERB), University of Allahabad.
Materials and procedure
Each participant completed a Colored Progressive Matrices (CPM) prior to the experiment, based on which the participant was screened for the study. Later, participants completed an age-appropriate cued task-switching paradigm. After task completion, the participants received a stationary kit as a reward. The task was called “Santa Claus Game” (Chevalier et al., 2015; Figure 1). The task was designed using PsychoPy (v3.2.4) on a Dell 13-inch touchscreen laptop. Participants were instructed to help Santa prepare for next Christmas by sorting toys (i.e., targets) by either their color or shape. Different combinations of shapes and colors were used (one for each condition), bear-car-blue-red, elephant-airplane-green-orange, and train-horse-pink-purple.
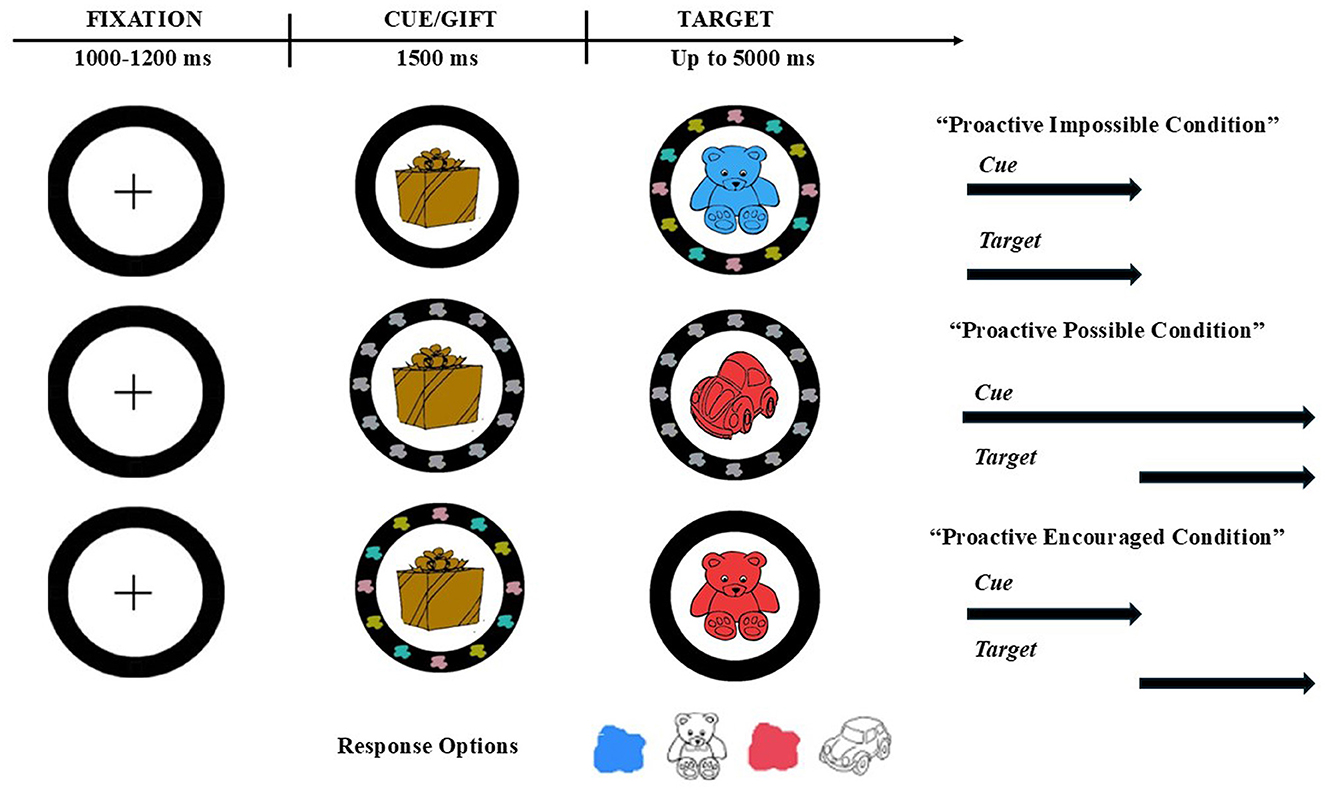
Figure 1. Schematic depiction of the cued task-switching paradigm (adapted from Chevalier et al., 2015). Children had to sort the target (Bear/Car) by shape or by color. The three conditions were designed based on the cue and target onset. In the “Proactive Impossible Condition”, the cue (colorful patches) and target (Blue Bear) appeared simultaneously, making it impossible to prepare proactively. In the “Proactive Possible Condition”, the cue (gray patches) appeared earlier and remained visible after the target (Red Car) onset, hence giving the choice to activate either of the control mode at dispersal. In the “Proactive Encouraged Condition”, the cue (colorful patches) disappeared before the target (Red Bear) onset, encouraging the child to use proactive control.
Each trial began with a jittered fixation cross within a black circle (15.5 × 15.45 cm) for 1,500–2,000 ms (with steps of 100 ms) at the center of the screen, which was followed by a gift box (brown-colored wrapped Christmas gift box irrelevant to the task and kept only for the engagement of the participant) that contained the toys they were supposed to sort. The gift box remained on the screen for 1,500 ms, which was then replaced by a bidimensional target (e.g., a blue car). The target remained on the screen until a response was made or up to 5,000 ms. Below the target, four unidimensional response pictures (~2 × 2 cm) (e.g., bear drawing, car drawing, red patch, blue patch) were constantly presented. Participants were supposed to touch the response option according to the task cues presented. Task cues were geometrical shapes (in case of shape cue) or colorful patches (in case of color cue) displayed on the black circle. Participants had to make responses based on the cues (color or shape) presented within the circle by touching one of the four response pictures on the screen. The timing of cue presentation was manipulated to create three conditions of the task (Chevalier et al., 2015; Doebel et al., 2017; Kubota et al., 2020, 2023). In the “Proactive Impossible” condition, the cue (geometrical shapes or colorful patches) was presented simultaneously with the target, making proactive preparation for the target impossible. In the “Proactive Possible” condition, the cue was presented before the target and remained visible on the screen when the target appeared. This presentation setup of the cue allowed the participants to proactively prepare to respond to the target although not necessary. Participants could also respond once the target appears since the cue remained visible even after the target onset. In the “Proactive Encouraged” condition, the cue appeared only with the gift box and then disappeared before the target onset. This made the use of reactive control more challenging (as in this case they will have to remember the task cue and prepare to respond to the target) for the participants and encouraging them to use the proactive mode of control.
The cue conditions were blocked, and participants were explicitly informed about the cue prior to each condition. Participants were given instructions and made familiar with the stimuli with the help of presentation slides prior to the start of the main experiment. Each condition started with 20 practice trials, along which guidance and feedback were provided. The test block consisted of 80 trials in each condition with no feedback. Prior to each condition, participants were given a short break. The switch and repeat trials within each block were in the proportion of 25–75% respectively (20 switch and 60 repeat trials). The trials were presented in a pseudo-random order across participants in such a manner that all the stimuli were presented with almost the same frequency and no two switch trials were presented consecutively. The experiment followed an Age (3) × Condition (3) × Trial Type (2) factorial design. After pre-processing, ~3.8% error rates were there in “Proactive Impossible” condition, 3.47% in “Proactive Possible” condition, and 8.49% in “Proactive Encouraged” condition.
Academic achievement
Academic achievement was measured differently for 5 and 6–11-year-olds. For 5-year-olds, School Readiness Assessment Tool by Learning and Teaching with Preschoolers (2017) was used which included knowledge of colors, shapes, numbers (1–20), uppercase and lowercase letters, beginning sounds, and name writing. For 6–11-year-olds, reading and writing ability in English and Hindi language was measured with the help of the Dyslexia Assessment for Languages of India (DALI) tool (Rao et al., 2021). This tool was used since it was standardized in Hindi and English language for the target age group on a large normative population in the age range of 5–11 years. It included sub-tests of letter and word reading, letter writing, letter writing, word spelling, and listening comprehension (verbal) for grades I and II and subtests of word reading, spelling, and reading comprehension (writing answers to the comprehension questions) for grades III to V. The reading-writing assessment took about 20–30 min to administer to each participant. Each correct response was assigned a score of 1 and an incorrect response a score of zero. School Readiness score was obtained by taking the percentage of all the subtests from the tool. Reading and writing scores for Hindi and English language were calculated separately and a total percentage score was obtained as an academic achievement score for older children. Both the scores were then transformed to z-scores within each age group and were then united as one dependent measure for hierarchical multiple regression analysis.
Statistical analysis
Accuracy was calculated as the percentage of correct responses on switch and repeat trials after discarding the contaminated trials. RTs were examined on correct trials. For RT calculation, error trials, missed trials, and trials following an error or a miss were removed. For error rate calculation, same procedure was used except that the error trials were retained. After this exclusion, RTs greater than Mean + 3 SD and less than Mean – 3SD were also removed. An average of 82.33% of trials in the case of RT calculation and 87.12% of trials in case of error rate calculation were retained after pre-processing. On average, 5.05% error rates were made across all conditions and trial types. To control age-related baseline differences, RTs were log-transformed, although we have used raw values in figures for a clear presentation of results (Meiran, 1996). A three-way mixed ANOVA [Age (3) × 3 Condition (3) × Trial type (2)] was performed with log-transformed RTs and error rates. Bonferroni's post-hoc test was performed for multiple comparisons. Inverse efficiency scores (IES) (Bruyer and Brysbaert, 2011; Hughes et al., 2014) were also analyzed to account for both speed and accuracy in a switching task. IES is an RT measure corrected for the proportion of errors committed. It is calculated by dividing the mean RT of the correct responses by 1-PE (proportion of errors) or by PC (proportion of correct responses).
OR
In addition, the Linear Integrated Speed-Accuracy Score (LISAS) (Vandierendonck, 2017, 2018, 2021), which is a linear combination of RT and PE was calculated. LISAS is defined as:
Where RTj is the participant's mean RT in condition j, PEj is the participant's proportion of error in condition j, and SRT and SPE are the participant's standard deviations of RT and PE respectively. A two-way ANOVA was performed with IES and three-way ANOVA was performed with LISAS.
In the IES method, RT and accuracy are not given equal weights. To address this limitation, we employed LISAS in addition to IES. Here, equal weights are given to RT and proportion of error (PE) by including the ratio of the standard deviation of the two performance measures in the formula, so that when their effects are opposite, they can balance each other out. IES provides cost to switching, whereas in LISAS we get different scores for switch and repeat trials, which can be compared using appropriate statistical tests (higher value implies poor performance). Since both analyses gave valuable information on the behavioral patterns in the task, we opted to present both to explain the results better.
The present study focused on the efficiency of the participants in performing the task utilizing RT, LISAS, and IES to test the hypotheses related to age-related differences in the recruitment of reactive and proactive control. RT as the base score and the integrated measures provided us with RT corrected for the number of errors committed (Vandierendonck, 2017). The statistical analysis was performed using R (version 4.2.3), JASP (v0.14), and Jamovi (version 2.5.6) and the codes used for analysis will be made available on request. For ease of presentation, we report the results based on RT, LISAS and IES. Results based on error rate analysis are provided in the Supplementary material.
The current study was not pre-registered. The pre-print of the study is available at https://doi.org/10.31234/osf.io/69nbd. The citation for the same is mentioned at the end of the manuscript. The data and study material will be made available on request.
Results
Reaction time analysis
A three-way ANOVA with age (5, 6–8 and 9–11 years), condition (“Proactive Impossible” Condition, “Proactive Possible” Condition and “Proactive Encouraged” Condition), and trial type (Switch and Repeat) was performed with log-transformed RTs as the dependent measure. Age F(2, 91) = 53.934; p < 0.001, = 0.542 and Condition F(2, 90) = 58.970; p < 0.001, = 0.393 significantly affected RTs. Interaction between Age and Condition, F(4, 182) = 4.303; p = 0.002, = 0.086, and Trial type and Age, F(2, 91) = 3.160; p = 0.047, = 0.065 was significant. Bonferroni's post-hoc test showed that 5-year-olds (7.833 log ms) had slower RTs compared to 6–8- (7.533 log ms) and 9–11-year-olds (7.383 log ms). Children responded faster in the “Proactive Encouraged” condition (7.475 log ms) compared to the “Proactive Possible” (7.599 log ms) and “Proactive Impossible” (7.676 log ms) condition (Figure 2A).
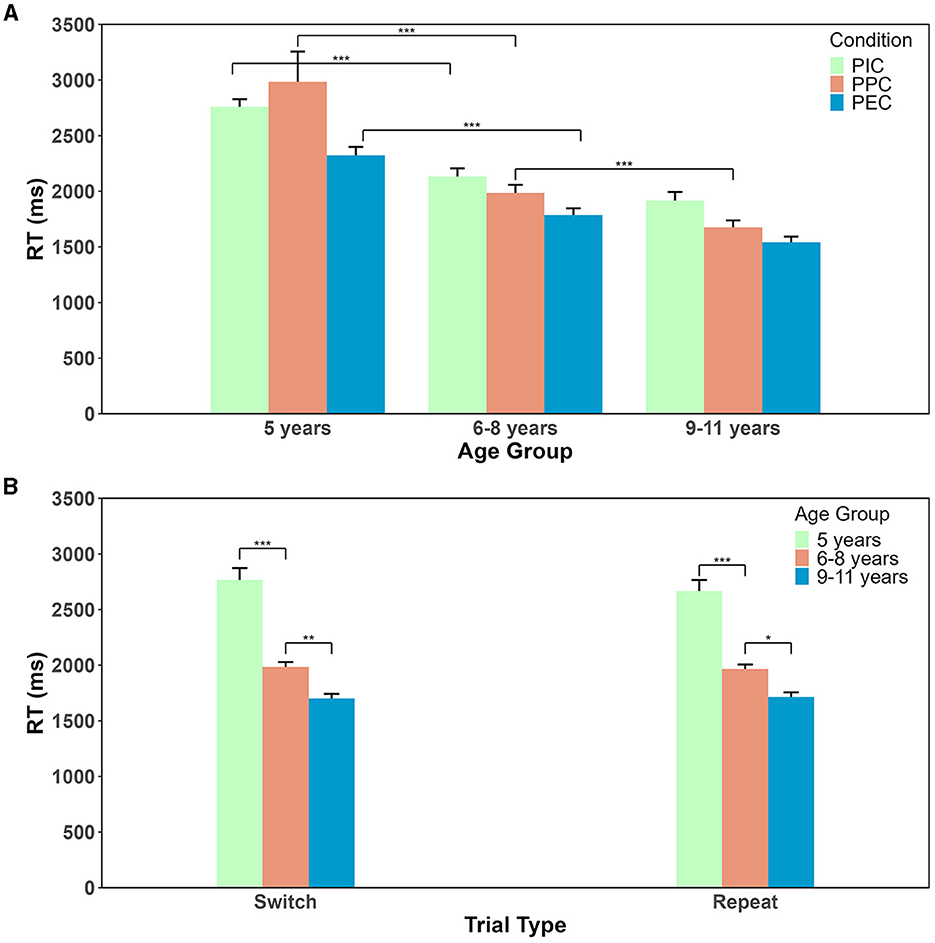
Figure 2. Mean RTs in each condition (A) and trial types (B) for 5, 6–8-, and 9–11-year-olds. Error bars indicate standard error of means. All age-groups performed faster in “Proactive Encouraged” condition (PEC) compared to “Proactive Possible” (PPC) and “Proactive Impossible” conditions (PIC). Five-year-old children responded significantly slow in “Proactive Possible” condition, indicating their difficulty in flexibly adapting to both control modes. *p < 0.05; **p < 0.01; ***p < 0.001.
For both 5- and 6–8-year-olds, there was no significant difference between the “Proactive Impossible” and “Proactive Possible” conditions. However, we find a significant difference between these two conditions in 9–11-year-old children, where they are faster in “Proactive Possible” condition (7.354 log ms) compared to “Proactive Impossible” condition (7.503 log ms), p < 0.001. There is no significant difference between “Proactive Possible” and “Proactive Encouraged” condition in 6–8- and 9–11-year-olds, however, the difference is seen in 5-year-olds, where they are faster in “Proactive Encouraged” condition (7.696 log ms) compared to “Proactive Possible” condition (7.91 log ms), p < 0.001. All age groups showed significantly faster RTs in “Proactive Encouraged” condition (7.696, 7.438, 7.291 log ms, in 5, 6–8-, and 9–11-year-olds respectively) compared to “Proactive Impossible” condition (7.893, 7.631, 7.503 log ms, in 5, 6–8-, and 9–11-year-olds respectively), p < 0.001 (Figure 2A). Results also suggest that 5-year-olds were slowest in “Proactive Possible” condition (7.91 log ms), whereas the older age groups were slowest in “Proactive Impossible” condition (7.631 and 7.503 log ms, respectively, Figure 2A). In both switch and repeat trials, 5-year-olds were found to have slower RTs compared to older age groups. However, there was no significant difference between switch and repeat trials across age groups (Figure 2B) (refer to Table A1 for descriptive statistics and Supplementary material for summary tables with ANOVA and post-hoc comparisons).
Thus, younger age groups performed similarly in “Proactive Impossible” and “Proactive Possible” conditions. In contrast, older age groups performed similarly in “Proactive Possible and “Proactive Encouraged” conditions, and 5-year-olds showed difficulty and slow performance in “Proactive Possible” condition, implying that they were having difficulty when both control modes were made available for use.
Switch cost
Switch costs were significant only across age groups, F(2, 91) = 3.160; p = 0.047, = 0.065. Five-year-olds had significantly larger switch costs compared to 9–11-year-olds, p = 0.042, however, no significant difference was found between 5 and 6–8-year-olds and 6–8- and 9–11-year-olds. Although insignificant, trends in the data showed that both older age groups showed the least switch cost in the “Proactive possible” condition.
Inverse efficiency scores
Significant main effects of Condition F(2, 78) = 14.214; p < 0.001, = 0.152 and Age F(2, 79) = 6.955; p = 0.002, = 0.150 were found for IES. Two-way interaction between Condition and Age F(4, 158) = 3.246; p = 0.024, = 0.076 was also significant. Condition-wise, there was no significant difference between “Proactive Impossible” (0.278) and “Proactive Possible” (0.316) conditions, although the “Proactive Encouraged” condition (0.9) had significantly higher costs than the other two conditions. There was a significant difference in costs between 5- and 9–11-year-olds (0.818 and 0.243, respectively), and the difference between 5 and 6–8-year-olds (0.432) was only marginally significant, where 5-year-olds had higher costs than older age groups. Post-hoc comparisons showed that 5-year-olds (1.491) had higher costs than 9–11-year-olds (0.292) in “Proactive Encouraged” condition. Only 6–8-year-olds (0.147) showed significantly lower costs in the “Proactive Possible” condition compared to the other two conditions (Figure 3A) (refer to Table A2 for descriptive statistics for mean and standard deviation of each condition by age for IES analysis and Supplementary material for ANOVA and post-hoc comparisons).
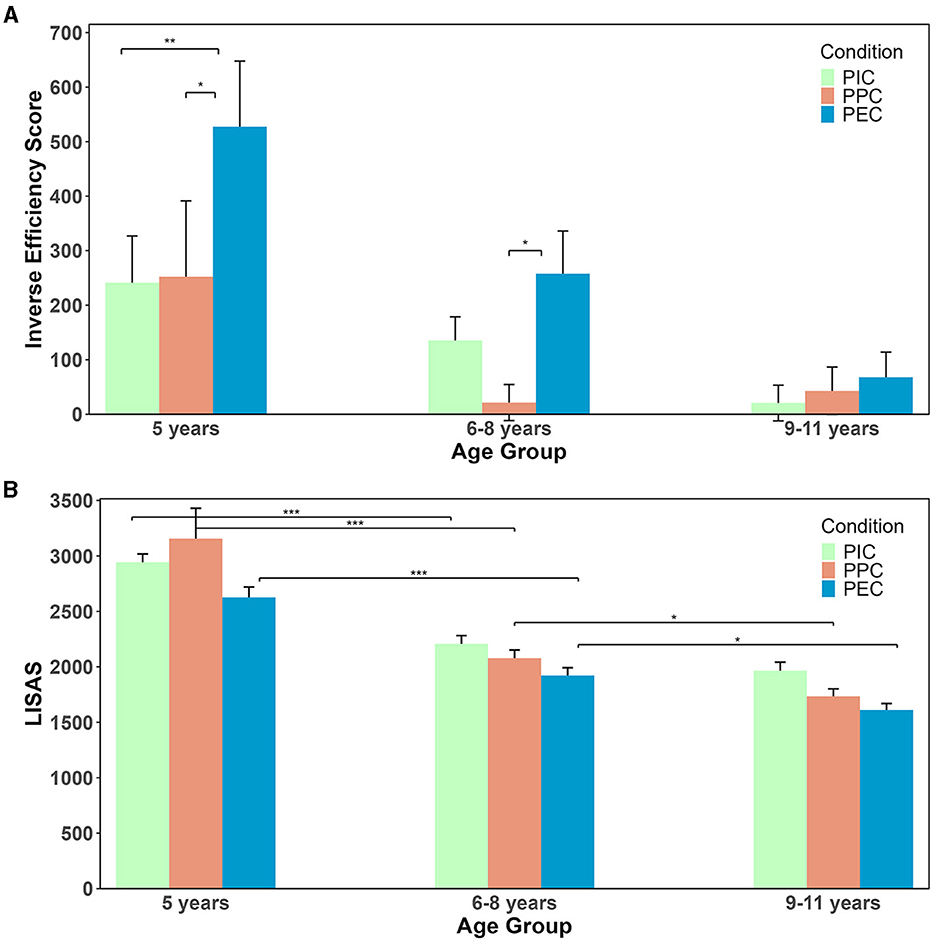
Figure 3. Inverse efficiency score (IES) (A) and Linear Integrated Speed-Accuracy Score (LISAS) (B) for each condition and age-group. Better flexible shifts in control modes, as shown by lower IES scores in “Proactive Possible” condition (A), by 6–8-year-olds. Difficulty in shifting between control modes, as shown by higher LISAS in “Proactive Possible” condition, by 5-year-olds (B). *p < 0.05; **p < 0.01; ***p < 0.001.
Linear Integrated Speed-Accuracy Score
Main effects of Age F(2, 91) = 62.899; p < 0.001, = 0.580, Condition F(2, 90) = 34.804; p < 0.001, = 0.277, and Trial type F(1, 91) = 23.309; p < 0.001, = 0.204 were found to be significant. Two-way interactions between Condition and Age F(4, 182) = 2.720; p = 0.031, = 0.056, and Trial type and Age F(2, 91) = 6.692; p = 0.002, = 0.128, was also be significant.
Condition-wise, the highest LISAS value was found in the “Proactive Impossible” condition (7.717) implying poor performance in this condition. Switch trials (7.66) had significantly higher values compared to repeat trials (7.619). Five-year-olds (7.922) significantly showed poor performance compared to older age groups (7.581 and 7.416 in 6–8- and 9–11-year-olds, respectively) (refer to Table A3 for descriptive statistics for mean and standard deviation of each condition by age for LISAS analysis).
Five-year-olds had significantly larger LISAS values compared to older age groups in all three conditions. Younger age groups (5 and 6–8-year-olds) had similar performance in “Proactive Impossible” and “Proactive Possible” conditions, implying they rely more on reactive control, whereas older age groups (6–8- and 9–11-year-olds) showed similar performance in “Proactive Possible” and “Proactive Encouraged” conditions, implying they rely more on proactive control. Five-year-olds' performance in “Proactive Possible” condition (7.971) (irrespective of trial type) was significantly poorer compared to “Proactive Encouraged” condition (7.833) (Figure 3B). In both switch and repeat trials, 5-year-olds had significantly higher LISAS values compared to older age groups. Also, differences between switch and repeat trials were only seen in 5-year-olds (refer to Table A3 for descriptive statistics for mean and standard deviation of each condition by trial type and age for LISAS analysis and Supplementary material for ANOVA and post-hoc comparisons).
We also analyzed the data with background factors such as Fluid intelligence (CPM scores) and Socio-economic status (SES) as covariates to test their interaction with the dependent variables (RT, error rates, LISAS, and IES). The main effect of fluid intelligence and SES were not found to be significant with any of the dependent variables. There was no significant interaction between fluid intelligence/SES with any of the independent variables except in the case of IES, where the interaction between fluid intelligence and conditions of the experiment was found significant (p = 0.036). The interaction between independent variables such as task condition (PPC, PIC, PEC) and age (5, 6–8, 9–11 years) was sustained for all the dependent measures [RT (p = 0.002), error rates (p < 0.001), LISAS (p = 0.027) and IES (p = 0.01)] with SES as a covariate and for RT (p = 0.6), error rates (p < 0.001) and IES (p = 0.029) with fluid intelligence as a covariate. The range of the level of fluid intelligence varied from average to above average intelligence after the initial exclusion of seven participants with extreme scores (superior or low average and below level of intelligence) and therefore would not have affected the results based on the covariate analysis. Details about the covariate analysis and results are presented in the Supplementary material.
Relationship between cognitive control and academic achievement
A hierarchical multiple regression was conducted to investigate how cognitive control measures alongside background factors (fluid intelligence and socio-economic status) would predict academic achievement in children. Reaction times (RTs) as the primary measure of performance on cued switching task across the three conditions (Proactive Impossible, Proactive Encouraged and Proactive Possible), were used as predictors. The z-scores of school readiness and reading/writing achievement were combined as one dependent variable within each age group. First, the background factors (SES and CPM) along with age group were taken for the first regression model (Model 1), which was found significant, F(4, 89) = 4.53, p = 0.002 and contributed 16.9% variance in the data. Only the Socio-economic status (SES) significantly predicted academic achievement, p < 0.001, in the first model. Model 2 included RT measures (across three conditions and trial type) and was found significant, adj. R2 = 0.323, F(13, 80) = 2.94, p = 0.002 accounting for 32.3% of variance in academic achievement's measures and contributed a significant increment of 15.4% variance explained when added to the model, = 0.154, Fchange(9, 80) = 2.02, p = 0.047. In the second model, we found that overall RT in “Proactive Possible” condition (p = 0.024), switch and repeat RT in PPC (p = 0.013 and p = 0.029) significantly predicted academic achievement (Table 2). SES significantly predicted academic achievement in both the models (ps < 0.001).
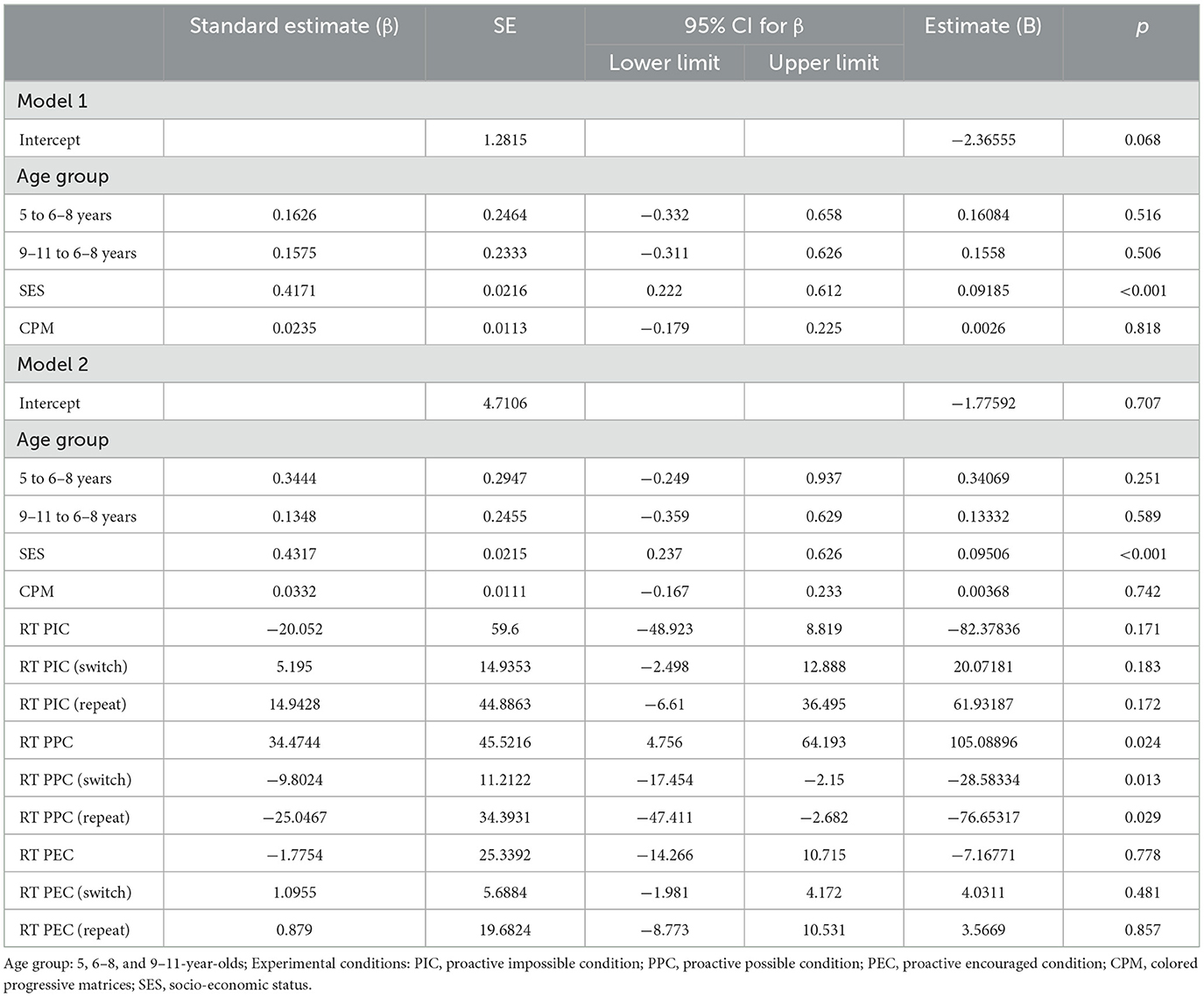
Table 2. Hierarchical multiple regression results for the prediction of academic achievements by cognitive control variables.
Discussion
The present study investigated developmental changes in the use of proactive and reactive modes of cognitive control in continuous age groups (5-, 6–8-, 9–11-years) using a cued task-switching paradigm. More specifically, we examined how children shift from the prominent use of reactive control toward a cognitively demanding proactive mode of control with age. The task was designed such that children were encouraged to use either proactive (“Proactive Encouraged”) or reactive control (“Proactive Impossible”) or had the opportunity to choose between the two modes of control (“Proactive Possible”). More specifically, in the “Proactive Encouraged” condition, proactive control would be the preferred strategy to optimize the performance. In the “Proactive Impossible” condition, utilizing reactive control would lead to optimal performance. Finally, in the “Proactive Possible” condition, children have the flexibility to optimize their performance by employing either of the control modes, and choosing the one they perceive as more advantageous. This flexibility would allow children to adapt to the changing demands in cognitive control more effectively.
We expected to find reactive control bias in younger children and proactive control bias in older children consistent with previous studies (Chatham et al., 2009; Chevalier et al., 2015, 2020). Further, we intended to examine flexible shifts in control modes depending on the task conditions and the age at which this transition could be observed. Results showed that overall, 5-year-olds were slow and less accurate on the cued switching task compared to 6–8- and 9–11-year-olds. Evidently, children in all age groups were faster and made more errors in the condition where the use of proactive control was encouraged, and the use of reactive control was restricted (“Proactive Encouraged”). Findings of the current study are discussed in terms of the flexible shifts in cognitive control modes across age groups, and its relationship with academic achievement.
Age-related differences in the flexible shifts in reactive and proactive control
Results of the current study demonstrate age-related differences in the use of reactive and proactive control in terms of the effect of task conditions on switch costs. Five-year-olds demonstrated a proactive profile when encouraged to use proactive control (exhibiting faster RTs in “Proactive Encouraged” condition). However, even though they were capable of using proactive control, they showed a reactive bias in the condition where proactive control was possible as evidenced by their similar performance in terms of RTs and error rates in “Proactive Possible” and “Proactive Impossible” conditions. On the other hand, 9–11-year-olds showed comparable performance in “Proactive Possible” and “Proactive Encouraged” conditions, indicating their ability to proactively prepare for the upcoming target by utilizing the cue (Figure 2). These findings further support the idea that 5-year-old children typically favor responses spurred by reactive control, while children aged 9–11 years tended to shift toward proactive control by actively maintaining the task cue and preparing for their responses. This finding aligns with previous research where 5-year-olds engaged control more reactively and could use proactive control when the use of reactive control was made difficult, whereas 10-year-olds engaged proactive control whenever possible (Chevalier et al., 2015). Chatham et al. (2009) also showed 3-year-olds' inclination toward reactive control when they manifested greater late mental effort after target onset in an AX-CPT task and 8-year-olds inclination toward proactive control when they manifested greater early mental effort before target onset. One of the recent studies by Yeshua and Berger (2024) with preschool and kindergarten-aged children also demonstrates that the development of reactive control precedes the development of proactive control. Lower levels of preparatory control have been found with eye movement studies on children and adolescents showing a slow maturation of cognitive flexibility in a cued switching task (Zheng and Church, 2021). In addition, we found comparable reaction times across the three age groups for both switch and repeat trials suggesting that goal representation or the ability to set goals may be similar for both trial types, also observed in case of young children (4–5 years of age) (Blaye and Chevalier, 2011). However, attentional flexibility to switch between task goals may be present in children as young as preschool age (4–6 years), particularly when the task and stimuli are age-appropriate (Dibbets and Jolles, 2006; Peng et al., 2018) which is also consistent with the stimuli and task used in the current study.
Integrated speed accuracy measures also validated the findings based on reaction time data. For instance, the 5-year-old children displayed a higher cost to switching when their preferred control mode, i.e., reactive control, was made difficult, and they could only rely on proactive control (Figure 3A) as suggested by the IES analysis. The inability of younger children to spontaneously engage proactive control can be attributed to their lower working memory capacity (Chevalier et al., 2015; Gonthier et al., 2019; Troller-Renfree et al., 2020), greater reliance on stimulus-related information to drive one's responses (Troller-Renfree et al., 2020), willingness to utilize available control resources, the cognitive effort required to do so (Chevalier, 2018) and tendency to overlook environmental cues and prioritizing objects/targets which can be directly acted upon (Chevalier et al., 2018). It is noteworthy that regardless of the type of trial, 5-year-olds exhibited higher LISAS values in the “Proactive Possible” condition (Figure 3B). This indicated that younger children encountered challenges when presented with a choice between control modes and shifting their strategy accordingly. These challenges could also be due to their over-reliance on reactive control, which might be their automatic strategy in any situation. In contrast, 9–11-year-olds showed no significant differences across conditions in IES scores, indicating that they can engage in proactive control whenever possible without consequences. With advancing age and practice, proactive control becomes less demanding, and children increasingly perceive it as more advantageous, leading to more frequent utilization (Chevalier et al., 2015). As children become adept at associating cues with their meaning and predictive value, they start prioritizing environmental cues. This enables them to use these cues proactively, not only to determine their immediate actions but also to prepare for future events (Chevalier et al., 2015).
We find that the performance of 6–8-year-olds in the “Proactive Possible” condition was comparable to both “Proactive Impossible” and “Proactive Encouraged” conditions in LISAS analysis (Figure 3B). In addition, they exhibited the least cost to switching in terms of IES scores, in the “Proactive Possible” condition (Figure 3A), where flexibility could be exercised by using either reactive or proactive control. Combining the findings from IES and LISAS analysis, it can be inferred that 6–8-year-olds demonstrate proficiency in employing both proactive and reactive control independently, as well as when given a choice, they displayed flexible shifting between them as per the demands of the situation. This outcome provides evidence of the critical role of this age group in fostering flexibility in children's cognitive development. The reason for only 6–8-year-olds showing such flexibility can be explained using the following example: in cases where children might have missed the cue in a “Proactive Possible” condition, they could switch to using reactive control to make up for their performance. On the other hand, if they focused on the cue from the beginning, they could employ proactive control efficiently and be well-prepared for the upcoming target. The 6–8-year-olds, with access to both control modes, seem to have mastered reactive control and could flexibly transition to proactive control when required. Another explanation for the developmental differences in cued task-switching comes from findings, which suggest that perceptual processing of the cues (that signal the need to switch or maintain the task goal), is beneficial while implementing the task switch in young children (5 years of age) (Chevalier et al., 2011). However, the difficulty in set shifting among young children may be due to the difficulty in monitoring the task goals and need to switch resulting in a preference for reactive mode of control.
The findings suggest that the critical period for transitioning from reactively approaching a task to proactively preparing for an upcoming task occurs around the age of 6–8 years. The 6–8-year-old children are likely to employ reactive control and switch to proactive control when the task requires the same. Unlike the clear preferences displayed by 5- and 9–11-year-olds for specific cognitive control modes, 6–8-year-olds can be assumed not to have a preferred mode of control rather they adapt to the task's demands, using either control mode as necessary. Gonthier and Blaye (2022) have shown that preschoolers can be instructed to use proactive control and that they are less likely to spontaneously engage in it. However, these accounts fail to offer evidence that the two control modes can be utilized independently or simultaneously within a specific context. Mäki-Marttunen et al. (2019) presented evidence supporting the idea that both control modes can be engaged independently and simultaneously, thus supporting the concept of “independent processes” rather than being two extremes within a continuum (Chiew and Braver, 2017). The results of the current study align with the “independent processes” account, given the use of flexible cognitive control in 6–8-year-olds. These findings can be explained in the light of the dual network view (Petersen and Posner, 2012) emphasizing the independent functional dynamics of the cingulo-opercular network engaged in background maintenance of task goals or top-down control (same as proactive control) and fronto-parietal network (ventral) engaged in stimulus-response mapping for online response adjustments (same as reactive control). These two networks are known to show rapid developmental changes from preschool age to late childhood supporting more flexible control of attention resources in older children (Rosario Rueda et al., 2015). Such flexibility in the recruitment of cognitive control has implications for self-regulation, education and behavior in general. The ability to flexibly shift between control modes demonstrated advantages in the academic domain as elaborated in the next section.
Flexible cognitive control and academic achievement
Cognitive abilities pertaining to working memory, inhibition, and shifting affect academic performance in children (St Clair-Thompson and Gathercole, 2006; Visu-Petra et al., 2011; Coldren, 2013; Gerst et al., 2017; Peng and Kievit, 2020). The current study demonstrates that flexible use of cognitive control modes predicts academic achievement in school. The ability to recruit cognitive control is a skill that is most likely transferrable to academic contexts. One of the recent studies that emphasized the role of proactive control in academic achievement in school showed how consistent use of proactive control across different tasks predicted academic abilities such as reasoning, math, and reading (Kubota et al., 2020). However, flexibility in using different modes of cognitive control may allow children to adapt to changing demands in learning academic skills, switch between tasks, and approach tasks from different perspectives. Cognitive flexibility has been found to play a crucial role in enhancing reading skills (Johann et al., 2020), which was also observed in the present study. Cognitive flexibility allows children to adjust their thinking and behavior depending on the varying circumstances, which facilitates their problem-solving abilities, and monitoring their performance (learning from mistakes and utilizing feedback), especially manifested in subjects like mathematics (Stad et al., 2018; Magalhães et al., 2020), reading comprehension and writing (Arán Filippetti and Krumm, 2020; Gordon et al., 2018). It also predicts rule learning (Feng et al., 2020) and contributes to academic achievement given the requirement to shift one's attention between the different concepts taught in school (Coldren, 2013). Background factors such as socio-economic status was found to be one of the strongest predictors of academic achievement across age groups. SES was found to have a modest relationship with academic achievement throughout primary and secondary education which persisted across countries and educational systems (Harwell et al., 2017; Liu et al., 2022), specifically in math achievement (Lawson and Farah, 2017).
Conclusion
The current study examined flexible shifts in cognitive control modes in children across continuous age groups (5, 6–8, and 9–11 years) and its relationship with academic achievement. We find that the 5-year-olds displayed slower and less accurate performance, with a preference for reactive control. They had difficulty in spontaneously engaging proactive control, likely due to lower working memory capacity and over-reliance on stimulus-related information. However, 9–11-year-olds showed greater reliance on proactive control, using the cue to prepare for the upcoming target. The study identifies the age group of 6–8 years as the critical period in the development of cognitive control. At this age, children demonstrate flexibility in using proactive and reactive control modes, adapting to the demands of the task. These findings align with the “independent process” account by demonstrating that children can use either control modes flexibly, strengthening the claim that both modes have separate information-processing mechanisms. The developmental transition toward flexible shifts in cognitive control modes was found to be correlated with improved academic achievement in children. When children can seamlessly shift between the control modes or can initiate, sustain and regulate their motivation and emotions toward goal-directed learning, it allows them to adapt to changing circumstances, switch between tasks, and think creatively. This adaptability is expected to enhance their problem-solving skills and academic performance.
One of the limitations of the current study is that the data was collected in a school environment, which constrained our control over extraneous factors, such as external noise. All the assessments were done during class hours, although not keeping the child out of the class for more than 30 min. Efforts were made to conduct the experiment in a controlled setting (as much as possible) in a dimly lit isolated room, which was provided by the school authorities. Secondly, the original plan to obtain academic achievement scores for multiple subjects from the school encountered few challenges. We were unable to receive scores of the last three exams, either due to timing issues or because of the busy schedule of the teachers. As a result, only reading and writing scores on the tests conducted by the authors were available for analysis. Initially, we also aimed to include 3–4-year-olds in the study. However, due to their shorter attention span and the tendency to get easily distracted, it was difficult to make them complete the experiment, even after providing rest pauses. Our future studies will also include math and problem-solving scores to comprehensively explore the relationship between flexible cognitive control and academic achievement. Additionally, future research may also refine the experimental paradigm to capture the nuanced aspects of flexibility in cognitive control, allowing children to assess when proactive or reactive control is most beneficial for optimal performance.
Author's note
The current study was not pre-registered. The pre-print of the study is available at https://doi.org/10.31234/osf.io/69nbd. The data and study material will be made available on request.
Data availability statement
The raw data supporting the conclusions of this article will be made available by the authors, without undue reservation.
Ethics statement
The studies involving humans were approved by Institutional Ethics Review Board, University of Allahabad. The studies were conducted in accordance with the local legislation and institutional requirements. Written informed consent for participation in this study was provided by the participants' legal guardians/next of kin.
Author contributions
TV: Conceptualization, Data curation, Formal analysis, Investigation, Methodology, Visualization, Writing – original draft. BK: Conceptualization, Funding acquisition, Investigation, Methodology, Project administration, Resources, Supervision, Validation, Visualization, Writing – review & editing.
Funding
The author(s) declare financial support was received for the research, authorship, and/or publication of this article. This work was supported by the Cognitive Science Research Initiative (CSRI), Department of Science and Technology (DST), Government of India [Project No.: DST/CSRI/2021/452 (G)].
Acknowledgments
The authors thank the school authorities and parents for their support in the data collection procedure. The authors would also like to acknowledge the support from the Cognitive Science Research Initiative (CSRI), Department of Science and Technology (DST), Government of India [Project No.: DST/CSRI/2021/452 (G)] for the funding provided by them. The authors would also like to acknowledge the use of ChatGPT, a language model developed by OpenAI, for its assistance in refining the language of the manuscript OpenAI. (2024). ChatGPT [Large language model]/gpts.
Conflict of interest
The authors declare that the research was conducted in the absence of any commercial or financial relationships that could be construed as a potential conflict of interest.
The author(s) declared that they were an editorial board member of Frontiers, at the time of submission. This had no impact on the peer review process and the final decision.
Publisher's note
All claims expressed in this article are solely those of the authors and do not necessarily represent those of their affiliated organizations, or those of the publisher, the editors and the reviewers. Any product that may be evaluated in this article, or claim that may be made by its manufacturer, is not guaranteed or endorsed by the publisher.
Supplementary material
The Supplementary Material for this article can be found online at: https://www.frontiersin.org/articles/10.3389/fdpys.2024.1435912/full#supplementary-material
References
Arán Filippetti, V., and Krumm, G. (2020). A hierarchical model of cognitive flexibility in children: extending the relationship between flexibility, creativity and academic achievement. Child Neuropsychol. 26, 770–800. doi: 10.1080/09297049.2019.1711034
Arán Filippetti, V., and Richaud, M. C. (2016). A structural equation modeling of executive functions, IQ and mathematical skills in primary students: differential effects on number production, mental calculus and arithmetical problems. Child Neuropsychol. 23, 864–888. doi: 10.1080/09297049.2016.1199665
Ayoub, S., and Raja, R. (2023). Economic parameter of modified Kuppuswamy socioeconomic status scale for the year 2023. Ind. J. For. Commun. Med. 10, 99–101. doi: 10.18231/j.ijfcm.2023.017
Blackwell, K. A., and Munakata, Y. (2014). Costs and benefits linked to developments in cognitive control. Dev. Sci. 17, 203–211. doi: 10.1111/desc.12113
Blaye, A., and Chevalier, N. (2011). The role of goal representation in preschoolers' flexibility and inhibition. J. Exp. Child Psychol. 108, 469–483. doi: 10.1016/j.jecp.2010.09.006
Braver, T. S. (2012). The variable nature of cognitive control: A Dual mechanisms framework. Trends Cogn. Sci. 16, 106–113. doi: 10.1016/j.tics.2011.12.010
Braver, T. S., Paxton, J. L., Locke, H. S., and Barch, D. M. (2009). Flexible neural mechanisms of cognitive control within human prefrontal cortex. Proc. Nat. Acad. Sci. U. S. A. 106, 7351–7356. doi: 10.1073/pnas.0808187106
Bruyer, R., and Brysbaert, M. (2011). Combining speed and accuracy in cognitive psychology: is the inverse efficiency score (IES) a better dependent variable than the mean reaction time (RT) and the percentage of errors (PE)? Psychol. Belg. 51:5. doi: 10.5334/pb-51-1-5
Campbell, J. I. D., and Thompson, V. A. (2012). MorePower 6.0 for ANOVA with relational confidence intervals and Bayesian analysis. Behav. Res. Methods 44, 1255–1265. doi: 10.3758/s13428-012-0186-0
Chatham, C. H., Frank, M. J., and Munakata, Y. (2009). Pupillometric and behavioral markers of a developmental shift in the temporal dynamics of cognitive control. Proc. Nat. Acad. Sci. U. S. A. 106, 5529–5533. doi: 10.1073/pnas.0810002106
Chevalier, N. (2015). The development of executive function: toward more optimal coordination of control with age. Child Dev. Perspect. 9, 239–244. doi: 10.1111/cdep.12138
Chevalier, N. (2018). Willing to think hard? The subjective value of cognitive effort in children. Child Dev. 89, 1283–1295. doi: 10.1111/cdev.12805
Chevalier, N., Dauvier, B., and Blaye, A. (2018). From prioritizing objects to prioritizing cues: a developmental shift for cognitive control. Dev. Sci. 21:e12534. doi: 10.1111/desc.12534
Chevalier, N., Martis, S. B., Curran, T., and Munakata, Y. (2015). Metacognitive processes in executive control development: the case of reactive and proactive control. J. Cogn. Neurosci. 27, 1125–1136. doi: 10.1162/jocn_a_00782
Chevalier, N., Meaney, J. A., Traut, H. J., and Munakata, Y. (2020). Adaptiveness in proactive control engagement in children and adults. Dev. Cogn. Neurosci. 46:100870. doi: 10.1016/j.dcn.2020.100870
Chevalier, N., Wiebe, S. A., Huber, K. L., and Espy, K. A. (2011). Switch detection in preschoolers' cognitive flexibility. J. Exp. Child Psychol. 109,353–370. doi: 10.1016/j.jecp.2011.01.006
Chiew, K. S., and Braver, T. S. (2017). “Context processing and cognitive control: from gating models to dual mechanisms,” in The Wiley Handbook of Cognitive Control, 1st Edn, ed. T. Egner (John Wiley & Sons Ltd), 143–166.
Coldren, J. T. (2013). Cognitive control predicts academic achievement in kindergarten children. Mind Brain Educ. 7, 40–48. doi: 10.1111/mbe.12006
Dibbets, P., and Jolles, J. (2006). The switch task for children: measuring mental flexibility in young children. Cogn. Dev. 21, 60–71. doi: 10.1016/j.cogdev.2005.09.004
Doebel, S., Barker, J. E., Chevalier, N., Michaelson, L. E., Fisher, A. V., and Munakata, Y. (2017). Getting ready to use control: advances in the measurement of young children's use of proactive control. PLoS ONE 12:e0175072. doi: 10.1371/journal.pone.0175072
Elke, S., and Wiebe, S. A. (2017). Proactive control in early and middle childhood: an ERP study. Dev. Cogn. Neurosci. 26, 28–38. doi: 10.1016/j.dcn.2017.04.005
Feng, X., Perceval, G. J., Feng, W., and Feng, C. (2020). High cognitive flexibility learners perform better in probabilistic rule learning. Front. Psychol. 11:415. doi: 10.3389/fpsyg.2020.00415
Gerst, E. H., Cirino, P. T., Fletcher, J. M., and Yoshida, H. (2017). Cognitive and behavioral rating measures of executive function as predictors of academic outcomes in children. Child Neuropsychol. 23, 381–407. doi: 10.1080/09297049.2015.1120860
Gonthier, C., and Blaye, A. (2022). Preschoolers can be instructed to use proactive control. Cogn. Dev. 62:101175. doi: 10.1016/j.cogdev.2022.101175
Gonthier, C., Zira, M., Colé, P., and Blaye, A. (2019). Evidencing the developmental shift from reactive to proactive control in early childhood and its relationship to working memory. J. Exp. Child Psychol. 177, 1–16. doi: 10.1016/j.jecp.2018.07.001
Gordon, R., Smith-Spark, J. H., Newton, E. J., and Henry, L. A. (2018). Executive function and academic achievement in primary school children: the use of task-related processing speed. Front. Psychol. 9:582. doi: 10.3389/fpsyg.2018.00582
Hadley, L. V., Acluche, F., and Chevalier, N. (2020). Encouraging performance monitoring promotes proactive control in children. Dev. Sci. 23:e12861. doi: 10.1111/desc.12861
Harwell, M., Maeda, Y., Bishop, K., and Xie, A. (2017). The surprisingly modest relationship between SES and educational achievement. J. Exp. Educ. 85, 197–214. doi: 10.1080/00220973.2015.1123668
Hernández, M. M., Valiente, C., Eisenberg, N., Berger, R. H., Spinrad, T. L., VanSchyndel, S. K., et al. (2017). Elementary students' effortful control and academic achievement: the mediating role of teacher–student relationship quality. Early Child. Res. Q. 40, 98–109. doi: 10.1016/j.ecresq.2016.10.004
Hughes, M. M., Linck, J. A., Bowles, A. R., Koeth, J. T., and Bunting, M. F. (2014). Alternatives to switch-cost scoring in the task-switching paradigm: their reliability and increased validity. Behav. Res. Methods 46, 702–721. doi: 10.3758/s13428-013-0411-5
Johann, V., Könen, T., and Karbach, J. (2020). The unique contribution of working memory, inhibition, cognitive flexibility, and intelligence to reading comprehension and reading speed. Child Neuropsychol. 26, 324–344. doi: 10.1080/09297049.2019.1649381
Kubota, M., Hadley, L. V., Schaeffner, S., Könen, T., Meaney, J.-A., Auyeung, B., et al. (2020). Consistent use of proactive control and relation with academic achievement in childhood. Cognition 203:104329. doi: 10.1016/j.cognition.2020.104329
Kubota, M., Hadley, L. V., Schaeffner, S., Könen, T., Meaney, J.-A., Morey, C. C., et al. (2023). The effect of metacognitive executive function training on children's executive function, proactive control, and academic skills. Dev. Psychol. 59, 2002–2020. doi: 10.1037/dev0001626
Lawson, G. M., and Farah, M. J. (2017). Executive function as a mediator between SES and academic achievement throughout childhood. Int. J. Behav. Dev. 41, 94–104. doi: 10.1177/0165025415603489
Learning and Teaching with Preschoolers (2017). School Readiness Assessment Tool. Available at: https://www.teacherspayteachers.com/Product/School-Readiness-Assessment-Tool-133558 (accessed March 21, 2022).
Liu, J., Peng, P., Zhao, B., and Luo, L. (2022). Socioeconomic status and academic achievement in primary and secondary education: a meta-analytic review. Educ. Psychol. Rev. 34, 2867–2896. doi: 10.1007/s10648-022-09689-y
Lucenet, J., and Blaye, A. (2014). Age-related changes in the temporal dynamics of executive control: a study in 5- and 6-year-old children. Front. Psychol. 5:831. doi: 10.3389/fpsyg.2014.00831
Magalhães, S., Carneiro, L., Limpo, T., and Filipe, M. (2020). Executive functions predict literacy and mathematics achievements: The unique contribution of cognitive flexibility in grades 2, 4, and 6. Child Neuropsychol. 26, 934–952. doi: 10.1080/09297049.2020.1740188
Mäki-Marttunen, V., Hagen, T., and Espeseth, T. (2019). Proactive and reactive modes of cognitive control can operate independently and simultaneously. Acta Psychol. 199:102891. doi: 10.1016/j.actpsy.2019.102891
Meiran, N. (1996). Reconfiguration of processing mode prior to task performance. J. Exp. Psychol. 22, 1423–1442. doi: 10.1037//0278-7393.22.6.1423
Munakata, Y., Snyder, H. R., and Chatham, C. H. (2012). Developing cognitive control: three key transitions. Curr. Dir. Psychol. Sci. 21, 71–77. doi: 10.1177/0963721412436807
Niebaum, J. C., Chevalier, N., Guild, R. M., and Munakata, Y. (2021). Developing adaptive control: age-related differences in task choices and awareness of proactive and reactive control demands. Cogn. Affect. Behav. Neurosci. 21, 561–572. doi: 10.3758/s13415-020-00832-2
Peng, A., Kirkham, N. Z., and Mareschal, D. (2018). Task switching costs in preschool children and adults. J. Exp. Child Psychol. 172, 59–72. doi: 10.1016/j.jecp.2018.01.019
Peng, P., and Kievit, R. A. (2020). The development of academic achievement and cognitive abilities: a bidirectional perspective. Child Dev. Perspect. 14, 15–20. doi: 10.1111/cdep.12352
Petersen, S. E., and Posner, M. I. (2012). The attention system of the human brain: 20 years after. Annu. Rev. Neurosci. 35, 73–89. doi: 10.1146/annurev-neuro-062111-150525
Rao, C., TA, S., Midha, R., Oberoi, G., Kar, B., Khan, M., et al. (2021). Development and standardization of the DALI-DAB (dyslexia assessment for languages of India – dyslexia assessment battery). Ann. Dyslexia 71, 439–457. doi: 10.1007/s11881-021-00227-z
Raven, J. (2012). Coloured Progressive Matrices and Crichton Vocabulary Scale: India Edition. NCS Pearson Inc, India.
Rosario Rueda M. P. Pozuelos J. M. Cómbita L. Deptartment of Experimental Psychology Center for Research on Mind, Brain, Behavior (CIMCYC), Universidad de Granada, Spain (2015). Cognitive neuroscience of attention from brain mechanisms to individual differences in efficiency. AIMS Neurosci. 2, 183–202. doi: 10.3934/Neuroscience.2015.4.183
Rosselli, M., Ardila, A., Matute, E., and Inozemtseva, O. (2009). Gender differences and cognitive correlates of mathematical skills in school-aged children. Child Neuropsychol. 15, 216–231. doi: 10.1080/09297040802195205
St Clair-Thompson, H. L., and Gathercole, S. E. (2006). Executive functions and achievements in school: Shifting, updating, inhibition, and working memory. Q. J. Exp. Psychol. 59, 745–759. doi: 10.1080/17470210500162854
Stad, F. E., Van Heijningen, C. J. M., Wiedl, K. H., and Resing, W. C. M. (2018). Predicting school achievement: differential effects of dynamic testing measures and cognitive flexibility for math performance. Learn. Individ. Differ. 67, 117–125. doi: 10.1016/j.lindif.2018.07.006
Troller-Renfree, S. V., Buzzell, G. A., and Fox, N. A. (2020). Changes in working memory influence the transition from reactive to proactive cognitive control during childhood. Dev. Sci. 23:e12959. doi: 10.1111/desc.12959
Valiente, C., Eisenberg, N., Haugen, R., Spinrad, T. L., Hofer, C., Liew, J., et al. (2011). Children's effortful control and academic achievement: mediation through social functioning. Early Educ. Dev. 22, 411–433. doi: 10.1080/10409289.2010.505259
Vandierendonck, A. (2017). A comparison of methods to combine speed and accuracy measures of performance: a rejoinder on the binning procedure. Behav. Res. Methods 49, 653–673. doi: 10.3758/s13428-016-0721-5
Vandierendonck, A. (2018). Further tests of the utility of integrated speed-accuracy measures in task switching. J. Cognit. 1:8. doi: 10.5334/joc.6
Vandierendonck, A. (2021). On the utility of integrated speed-accuracy measures when speed-accuracy trade-off is present. J. Cognit. 4:22. doi: 10.5334/joc.154
Visu-Petra, L., Cheie, L., Benga, O., and Miclea, M. (2011). Cognitive control goes to school: the impact of executive functions on academic performance. Proc. Soc. Behav. Sci. 11, 240–244. doi: 10.1016/j.sbspro.2011.01.069
Wang, C., Li, B., and Yao, Y. (2021). Proactive control mediates the relationship between working memory and math ability in early childhood. Front. Psychol. 12:611429. doi: 10.3389/fpsyg.2021.611429
Yeshua, M., and Berger, A. (2024). The development of cognitive control in preschoolers and kindergarteners: the case of post-error slowing and delayed disinhibition. J. Intell. 12:41. doi: 10.3390/jintelligence12040041
Zabelina, D. L., and Robinson, M. D. (2010). Creativity as flexible cognitive control. Psychol. Aesthet. Creat. Arts 4, 136–143. doi: 10.1037/a0017379
Keywords: cognitive control, proactive control, reactive control, flexibility, academic achievement
Citation: Verma T and Kar BR (2024) Development of flexible shifts in control modes and its relationship with academic achievement. Front. Dev. Psychol. 2:1435912. doi: 10.3389/fdpys.2024.1435912
Received: 21 May 2024; Accepted: 06 August 2024;
Published: 22 August 2024.
Edited by:
Stephanie M. Carlson, University of Minnesota Twin Cities, United StatesReviewed by:
Maor Yeshua, Ben-Gurion University of the Negev, IsraelAslı Aktan-Erciyes, Kadir Has University, Türkiye
Copyright © 2024 Verma and Kar. This is an open-access article distributed under the terms of the Creative Commons Attribution License (CC BY). The use, distribution or reproduction in other forums is permitted, provided the original author(s) and the copyright owner(s) are credited and that the original publication in this journal is cited, in accordance with accepted academic practice. No use, distribution or reproduction is permitted which does not comply with these terms.
*Correspondence: Bhoomika R. Kar, Ymhvb21pa2FAY2Jjcy5hYy5pbg==