- Institute of Psychology, University of Bern, Bern, Switzerland
When assessing their certainty, children are often poor at accurately monitoring their level of learning. The study examined the relationships between memory performance, intellectual ability, and metacognitive monitoring accuracy in kindergarten children. We also explored whether specific thresholds in memory performance and non-verbal intellectual ability influence metacognitive monitoring accuracy to identify group-specific patterns that might be masked by an overall linear analysis. We assessed the monitoring discrimination of 290 kindergarteners (Mage 6 years) using a paired-associates learning task. Results showed small correlations between task performance, intellectual ability, and metacognitive monitoring. Non-verbal intellectual ability provided explanatory value for monitoring accuracy beyond memory performance. We observed group-specific results consistent with the unskilled-and-unaware effect; children with the highest memory skills were more effective at discriminating between correct and incorrect answers than their peers with the lowest memory skills. However, kindergarteners with the highest non-verbal intellectual abilities did not demonstrate greater cognitive adaptability in novel tasks, as their monitoring accuracy was comparable to that of peers with average or lower intellectual abilities. Findings indicate that both task performance and non-verbal intellectual ability are relevant for monitoring accuracy, but the impact of non-verbal intellectual ability was less significant than anticipated. The modest correlation suggests that kindergarteners' non-verbal intellectual ability and metacognitive monitoring abilities operate relatively independently.
1 Introduction
Imagine a kindergarten child playing a memory game with peers. When trying to remember the correct location of the matching card, she may demonstrate metacognitive monitoring skills by evaluating different candidate positions and selecting the card for which she is most certain. However, her performance in the game may also benefit from overall and generally good memory skills or superior intellectual abilities compared to her peers. While in the adult literature, memory skills and intellectual abilities have been found to influence metacognitive monitoring, very little is known about the impact of one or both factors on children's emerging metacognitive monitoring skills. In the present approach, therefore, we will shed light on these intertwined and interacting cognitive processes in kindergarten children. Metacognitive monitoring is a fundamental part of metacognition (Flavell, 1979), referring to the ability to accurately monitor the certainty or uncertainty of one's ongoing cognitive activity and is critical for detecting errors and making informed decisions about the strategic regulation of behavior (Nelson and Narens, 1990; Lyons and Ghetti, 2011; Ghetti et al., 2013; Coughlin et al., 2015). Metacognitive monitoring is important in many everyday life situations, such as remembering shopping lists, memory cards' locations, peers' names, trains' departure times, and the like. In early childhood, children's metacognitive monitoring skills are shaped by their everyday experiences. These experiences, such as interactions with caregivers (Fukkink et al., 2024), play activities (Moore et al., 1986), and early childhood educational programs (Eckhardt and Egert, 2020), form the basis for cognitive and metacognitive skills. Individual differences in developmental trajectories can be attributed, at least in part, to contextual factors such as the interactions children have at home or at school (Ornstein et al., 2008). For example, with increasing experience, children become more skillful at using conscious strategies to remember sets of words, objects, and pictures (Schneider and Pressley, 1997). Metacognitive monitoring skills are also considered a prerequisite for self-regulated learning as it enables an individual to identify knowledge gaps, recognize and correct errors, and control and orchestrate the different cognitive processes involved (Flavell et al., 1997; Efklides, 2008; Schneider and Löffler, 2016).
There is no doubt that metacognitive monitoring processes depend on the underlying memory being monitored and the person's general intellectual abilities. When considered separately, both intellectual ability and metacognitive monitoring have consistently been shown to be strong predictors of performance across different studies involving children and adults (Neisser et al., 1996; Roth et al., 2015; Roebers, 2017; Ohtani and Hisasaka, 2018). Given that these cognitive processes undergo rapid development in children aged 5 to 7 and are not yet functioning optimally (Blair and Raver, 2015; Roebers, 2017), it prompts the question: to what extent are these interrelated processes associated during early development? Might general and abstract intellectual abilities potentially serve as a driving force in this dynamic interplay? Or are individual differences in the task at hand, the memory task, more important for children's emerging metacognitive monitoring abilities?
Indeed, research has shown that students with higher intellectual abilities not only excel in task performance but also possess a superior ability to monitor performance (Sternberg, 1985, 1999; Alexander et al., 1995; Efklides, 2019). At the same time, metacognitive monitoring appears to be distinctly influenced by the individual's level of task performance, indicating that better task performance is often associated with more accurate monitoring (Roderer and Roebers, 2013; Destan and Roebers, 2015; Händel and Dresel, 2018). However, the influence of intellectual differences on task performance, and consequently on metacognitive monitoring, has often been overlooked in previous research (Roebers, 2017). To understand the early development of metacognitive monitoring, researchers might want to consider a child's cognitive resources alongside her memory skills. This is especially the case as both metacognitive monitoring and intelligence are considered higher-order cognitive processes. It has been suggested that metacognitive monitoring ability in adolescents and adults may not entirely depend on their intellectual abilities (Veenman and Elshout, 1999; Veenman and Beishuizen, 2004).
In the present study, we aimed to investigate the relationship between metacognitive monitoring and non-verbal intellectual ability during the early stages of cognitive development, as these different cognitive processes unfold. In other words, this study aimed to understand factors contributing to differences in metacognitive monitoring among kindergarten children, especially because children in this age range typically show pronounced difficulties in assessing their memory abilities. To do so, we examined the relationships between non-verbal intellectual ability and task performance in a memory task, on the one hand, with monitoring accuracy, on the other hand, in a large sample of kindergarten children. In the following, we consistently refer to non-verbal intellectual ability as intellectual ability. We sought to explore to what extent memory performance, intellectual ability, and metacognitive monitoring are interrelated and how individual differences in intellectual ability influence metacognitive monitoring over and above task performance. Understanding this interplay may – in the long run – be informative for the development of kindergarten programs that strengthen not only children's cognitive but also metacognitive skills.
One way to study the impact of performance on metacognitive monitoring abilities is to compare students with lower and higher achievement levels. Research examining a variety of outcome variables suggests that high performers within a sample, defined as those with scores above the median, tend to provide more accurate metacognitive monitoring judgments than students performing below the median in that sample (e.g., Hacker et al., 2000; Händel and Fritzsche, 2016; Serra and DeMarree, 2016; Händel and Bukowski, 2019), but slightly underestimate their actual performance (Kruger and Dunning, 1999). Conversely, low performers often show less accurate monitoring, along with a tendency to overestimate their performance (Hacker et al., 2008; Roderer and Roebers, 2013). Kruger and Dunning (1999) referred to this cognitive bias as the “unskilled-and-unaware-effect,” whereby poor performers not only lack sufficient task-relevant knowledge but also experience a deficit in metacognitive monitoring ability. This effect has been documented in studies with adults in a variety of contexts, such as logical reasoning tasks, grammar (Kruger and Dunning, 1999), card games (Simons, 2013), medicine (Hodges et al., 2001), or mathematics (Händel and Dresel, 2018).
The “unskilled-and-unaware effect” is also evident in children. Lucangeli et al. (1997) demonstrated that students who achieved higher assessment scores had a more comprehensive understanding of the sequential steps and were more familiar with the rules and criteria required to complete tasks effectively compared to lower-achieving age-mates. This deeper understanding not only helped higher achievers to complete tasks efficiently, but also improved their ability to judge the accuracy and quality of their own work, which in turn contributed to their more accurate monitoring judgments.
A body of literature focusing on children with and without learning disabilities has highlighted the importance of possessing task-relevant knowledge for accurate monitoring (Klassen, 2007; Job and Klassen, 2012; Crane et al., 2017). These studies revealed that children facing learning difficulties who lack such task-specific knowledge tend to exhibit lower accuracy levels and are more prone to overconfidence in their judgments. For example, Desoete et al. (2006) conducted a study with third graders. They found that children with learning disability in math, regardless of their intelligence level, not only had difficulty understanding the knowledge required for the tasks but also made significantly more errors and generally had lower monitoring accuracy than children without learning disabilities. By providing children with learning difficulties with both task-relevant and metacognitive knowledge (i.e., awareness of their own strengths and weaknesses as learners), Lucangeli et al. (2019) aimed to train the children's ability to accurately monitor their performance and identify errors. Post-training results showed that students with mathematical difficulties outperformed the control group in both performance and monitoring accuracy. After the training, these children were more active and independent in applying metacognitive monitoring, better at recognizing difficult tasks, and more engaged in identifying errors and finding solutions compared to the untrained control group.
To date, research on the unskilled-and-unaware effect in typically developing samples of young children is limited. Roderer and Roebers (2013) addressed this gap by conducting research involving fifth graders in a real-world school setting over a year. Their study focused on students' performance estimations and the deviation from their actual mathematics and science performance. Based on their test scores, these students were categorized as low, average, or high achievers. The results revealed significant differences in monitoring accuracy between lower and higher achievers, with high-achieving children consistently demonstrating higher monitoring accuracy across most of the tests. Importantly, only the two extreme groups differed from one another; that is, children with average performance did not significantly differ from either group with respect to their monitoring accuracy.
In addition to task performance, intellectual ability is known to have a direct impact on metacognitive monitoring skills. Several studies have shown that intellectual ability is a significant factor in memory and learning situations (Shore and Dover, 1987; Alexander et al., 1995; Hannah and Shore, 1995; Sternberg, 1999). A review of the literature reveals a variety of theoretical assumptions regarding the relationship between metacognition and its facets (metacognitive knowledge, metacognitive monitoring, and control) and intelligence (see for a review Alexander et al., 1995). A prominent example is Sternberg's Theory of Adaptive Intelligence, which views metacognition as a manifestation of intelligent thinking. According to Sternberg's definition of intelligence, a key ability is to adapt effectively to the environment (Sternberg, 1980, 1988, 2019). Intellectual differences determine how effectively individuals can approach new, challenging tasks or adapt to new situations. In this context, Sternberg's (1999) concept of metacognition (referred to as metacomponent) is of particular importance, as it considers metacognition as a fundamental skill essential for navigating and excelling in complex tasks. This concept emphasizes a profound link between metacognitive monitoring and intellectual differences, ultimately suggesting that these interrelated aspects converge into a single, overarching skill essential for effective task performance. Empirical support for Sternberg's theoretical considerations originates from giftedness research showing that gifted children tend to outperform their peers in metacognitive knowledge (i.e., knowledge about cognition see Flavell, 1979; Schneider et al., 1987; Alexander et al., 2006) and metacognitive control strategies (Carr et al., 1994). Interestingly, gifted children typically display advanced metacognitive monitoring abilities when presented with new and challenging tasks, confirming the assumed adaptive nature of metacognitive processes (Carr et al., 1996).
Studies examining intelligence and metacognition across non-gifted samples of participants, however, consistently report only small amounts of shared variance, suggesting two rather than one overarching ability (Veenman and Spaans, 2005; van der Stel and Veenman, 2008). For example, a meta-analysis examined the influence of metacognition on intelligence across various age groups, including adults, adolescents, as well as primary school and kindergarten children (Ohtani and Hisasaka, 2018). The study revealed a moderate overall correlation between metacognition and fluid intelligence (r = 0.27). The relationship between metacognition and general intelligence, which also encompasses the component of crystallized intelligence, has been shown to vary depending on age. That is, the effect sizes for primary school children (r = 0.25) and kindergarteners (r = 0.29) were smaller than those for adolescents (r = 0.38) and adults (r = 0.34). When focusing solely on fluid intelligence and offline measures of metacognition (i.e., typically measured after a cognitive task or learning, e.g., questionnaires), consistently weaker correlations were found. A correlation of r = 0.23 was found for children in primary school, r = 0.28 for adolescents, and r = 0.22 for adults. However, these findings are based on only four studies, and no study was identified examining kindergarten children. Nevertheless, the overall findings of this meta-analysis suggest that correlations between fluid intelligence and monitoring accuracy in kindergarteners are most likely low.
The limited research underscores a critical need to examine how metacognitive monitoring and intellectual ability and their mutual influence within specific learning contexts, such as memory learning, among kindergarten children. Including young children offers the advantage of exploring the interdependence of these central information-processing skills while each of these components is emerging and developing. Is their development during early ages characterized by greater independence, influenced primarily by specific everyday life experiences, while later on, they develop a mutual influence? This question is of theoretical and practical relevance as it helps to better understand developmental progression in each of the components and may inform educators how to best support children who face difficulties in either of these processes.
As to the existing methods of assessing metacognitive monitoring, there is large heterogeneity across studies. Prospective monitoring judgments, such as judgments-of-learning or feeling-of-knowing judgments, are typically assessed beforehand. In contrast, our study used retrospective monitoring judgments (confidence judgments) provided after completing a task. We chose confidence judgments because prior studies have shown that retrospective judgments are more accurate than prospective judgments (Hacker et al., 2000; van Loon and Roebers, 2021). Repeatedly, retrospective judgments have been documented to become (a) more precise with age (for reviews, see Schneider and Löffler, 2016; Roebers, 2017) and (b) task experience (Hacker et al., 2000; Bol and Hacker, 2012). That is, children become increasingly better at discriminating in their monitoring judgments between correct and incorrect task performance by giving higher judgments after correct and lower judgments after incorrect responses. Monitoring accuracy, namely, the ability to metacognitively discriminate between correct and incorrect performance, was used as the primary monitoring measure in the present study (Schraw, 2009).
1.1 Current study
Since both task performance and intellectual abilities have been shown to have a unique influence on metacognitive monitoring (e.g., Neisser et al., 1996; Veenman and Spaans, 2005; van der Stel and Veenman, 2008; Destan and Roebers, 2015; Schneider and Löffler, 2016), their interplay is of particular interest in this study. In our study, we could only build on the previously inconsistent and scarce findings on the relationship between intellectual abilities, memory performance, and monitoring performance in young children. In the context of a paired-associates learning task, a typical sample of 6-year-old kindergarteners studied Japanese symbols and their meanings and were then tested on their ability to recognize these paired-associates and provide confidence judgments about their accuracy.
Firstly, we investigated whether and how intellectual ability and memory performance are related to the ability to monitor one's own thought processes. Based on theoretical considerations and the aforementioned empirical studies showing interrelations between metacognitive monitoring and memory performance (Klassen, 2007; Crane et al., 2017), on the one side, and metacognitive monitoring and intellectual ability (van der Stel and Veenman, 2008), on the other side, we expected metacognitive monitoring, memory performance, and intellectual ability to be weakly but positively related to each other.
Secondly, we paid particular attention to whether the nature of these relationships changes when a specific threshold of either memory performance or intellectual ability is exceeded or not reached.
In the context of memory performance, our study aimed to determine whether we could observe a pattern similar to the “unskilled-and-unaware effect” proposed by Kruger and Dunning (1999) within our sample of typically developing kindergarten children. In other words, we wanted to find out whether children in the highest quartile of memory performance are better at detecting errors and distinguishing between correct and incorrect answers than children in the lowest threshold range of memory performance. Based on existing studies with adults and older children that have examined the difference between estimated and actual performance (Hacker et al., 2000; Job and Klassen, 2012; Simons, 2013), we anticipated that discrimination ability would follow a similar pattern. Specifically, we expected children whose memory performance ranked in the lowest quartile to display a substantially lower level of monitoring accuracy than those age mates whose memory performance ranked in the highest quartile.
Regarding intellectual ability, we aimed to find out, for example, whether children within the highest quartile of the distribution of intellectual abilities in our sample, similar to gifted children, have a superior ability to recognize errors and thus an advantage in monitoring their cognitive processes. This follows the findings of some intelligence research studies suggesting such an advantage (Swanson, 1992; Alexander et al., 1995), as well as Sternberg's (1985, 1988) assumption that exceptional intelligence is relevant for dealing effectively with novel situations in different cognitive domains. Thus, we expected that children with high levels of intellectual ability would exhibit high monitoring accuracy, as their cognitive abilities enable them to evaluate their performance more effectively and vice versa.
Thirdly, we wanted to go beyond existing research by investigating the extent to which intellectual abilities can predict the ability to metacognitively discriminate between correct and incorrect answers beyond task performance. That is, we aimed to explore whether intellectual abilities and task performance interact in predicting monitoring accuracy, as higher intellectual abilities may support task performance, which – in turn – positively impacts metacognitive monitoring. To date, there has been a lack of research on this specific issue in the context of kindergarten children. Previous research encompassing all three variables—intellectual ability, metacognitive monitoring, and task performance—primarily investigated whether intellectual ability predicts academic achievement over and above what is predicted by monitoring (van der Stel and Veenman, 2008). In contrast, we focused on the predictive value of memory performance and intellectual ability for monitoring accuracy. Despite the sparse empirical evidence available, we expected that intellectual ability would significantly contribute to monitoring accuracy, over and above task performance, uncovering interactional effects of intellectual ability and task performance on monitoring discrimination.
2 Methods
2.1 Participants
We recruited 294 children from local kindergartens in the central German-speaking part of Switzerland, each completing their mandatory second year in a kindergarten. Teachers confirmed that all but one child were sufficiently proficient in the German language to follow the verbal instructions. Two children declined participation, and one was excluded due to insufficient German language proficiency. One child was excluded from the analysis due to a technical error in a task. The final sample consisted of 290 children (Mage = 6 years, 4 months, SD = 0.3 years; age range between 5.5 to 7.5 years, 50% girls), with 63% being native German speakers and 30% non-native German speakers. Demographic data for 7% of the children were unavailable. Parents provided written consent, and children gave oral assent. The study was approved by the Faculty's Ethics Committee of the University of Bern and was conducted in accordance with the Declaration of Helsinki.
2.2 Materials and procedures
We utilized pretest data stemming from a broader intervention study. The pretest spanned two non-consecutive days, separated by a minimum of one day and a maximum of two days interval, with each session lasting between 45 and 60 min. We tested children in small groups ranging from three to eight children within a quiet environment at their kindergarten. Children individually completed a memory learning task, as well as a subscale of a non-verbal intelligence assessment on Samsung Galaxy Tablets A7 (10.4′′) and S4 (10.5′′). All the children completed the tasks on the tablets independently and easily and reported to have prior experiences with such devices. The design of the tasks, which only required simple touch gestures, was intuitive and enabled easy interaction with the technology. In addition, the tasks had no time limits, which made it even easier for the children to use the technology.
Data were anonymized and securely transferred to a server. Two trained assistants gave children general instructions about test material and provided technical support. Children received task-specific instructions through headphones.
2.2.1 Paired-associates learning task
We used a paired-associates learning task (Kanji) with a recognition test and confidence judgments to assess children's metacognitive monitoring. This task was previously used in other studies and has been proven effective as learning materials for younger children (Bayard et al., 2021; Buehler et al., 2021). We selected 32 paired associates of varying difficulty from a pool of 66 items. We randomly divided these selected items into two sets, each containing 16 Japanese characters (Kanjis). Children were then randomly assigned to either set A or B for the pretest and received the opposite set for the posttest (not included in this study). Each item was presented for 5 s in a random order. We provided items varied in difficulty for each measurement point to ensure sufficient variability in the monitoring judgments. The different difficulty levels were established based on prior studies and based on the perceptual demands required for memory encoding, as illustrated in the Supplementary Figure S1 with an example of a complex and a simple Kanji character. The examples demonstrate the variance in difficulty and the associated cognitive load required to encode them into memory. Post-hoc item difficulty for the Kanji task was determined by the number of correct answers divided by the total number of participants. The mean item difficulty was M = 0.33, SD = 0.08, and ranged from 0.22 (difficult) to 0.48 (easy). The tasks comprised 19% easy, 59% average, and 22% difficult items. Both sets of items demonstrated high internal consistency α > 0.92.
Prior to the test, children were introduced to four short stories illustrating varying levels of certainty and uncertainty in everyday situations (see Supplementary Figure S2). These stories aimed to familiarize them with a 7-point Likert scale presented as a thermometer (adapted from Koriat and Shitzer-Reichert, 2002, see Supplementary Figure S3). They were instructed to use this scale to rate their feelings of certainty or uncertainty, such as feeling unsure when the content of a jar was unknown or invisible versus feeling sure when the content was known or visible. The thermometer scale consisted of seven colored fields, each corresponding to a different level of certainty, ranging from blue (very unsure = 1) to red (very sure = 7). To ensure that the children could accurately apply the scale and become familiar with the task steps, they conducted a practice trial on the tablet, which they completed successfully. Children understood the rationale of the scale with ease. On average, participants completed the task within 15 min.
The paired-associates learning task consisted of four phases: a study phase, a filler task, a recognition phase, and a monitoring phase. In the study phase, children were required to learn 16 Japanese characters (Kanji) and their corresponding pictorial interpretations (see Figure 1 for the schematic task procedure). Following the study phase, a 1-min filler task was introduced to discourage rehearsal strategies. Children used their fingers to interact with a moving cat on the screen. Immediately after that, the recognition phase started. A Kanji appeared on the left side of the screen, accompanied by four pictures on the right side. Among the four randomly presented alternatives, one picture matched the meaning of the Kanji, while the other three corresponded to different learned picture pairs. Each test trial concluded with the monitoring phase, in which children provided confidence judgments in their given answer being correct by using the thermometer scale. We calculated the percentage of correctly recognized items out of 16 items to measure memory performance.
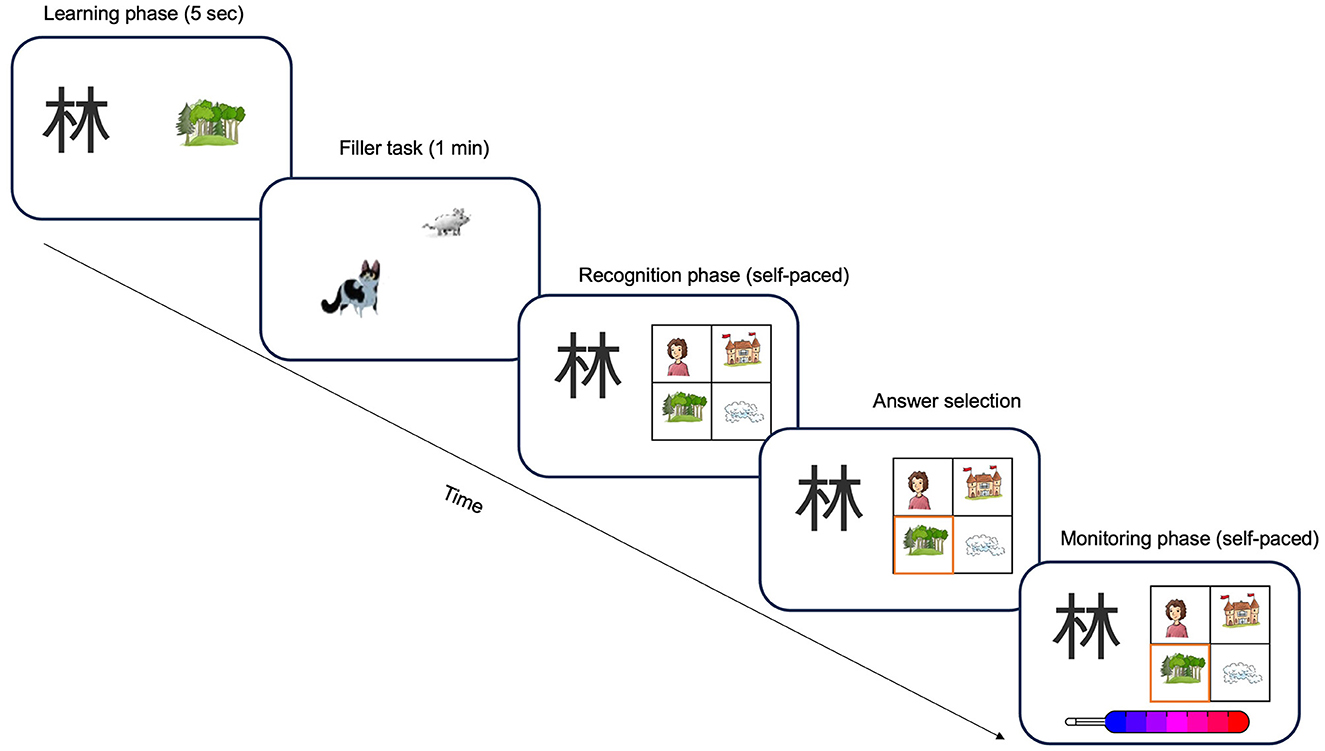
Figure 1. Task procedure at pretest and posttest. Schematic representation of Kanji task procedure. Children learned 16 items.
To assess monitoring, we calculated a monitoring discrimination score that quantifies the ability to discriminate between confidence judgments for correct and incorrect answers (Schraw, 2009; Fleming and Lau, 2014). Monitoring discrimination measures the accuracy with which participants metacognitively discriminate between correct and incorrect answers in their monitoring judgments. Thus, we computed the difference between the mean confidence judgment after correct answers and the mean confidence judgments after incorrect answers. A positive monitoring discrimination score suggests that an individual is able to discriminate the accuracy of given answers by giving higher judgments after correct than after incorrect responses.
2.2.2 Intellectual ability
To assess children's intellectual ability, we administered the computerized Odd-Item Out RIAS subscale (Reynolds and Kamphaus, 2009, German adaptation: Hagmann-von Arx and Grob, 2014). This subtest not only assesses non-verbal reasoning skills but also necessitates the application of spatial ability, visual imagery, and a range of other non-verbal skills across various items. It essentially serves as a reverse form of non-verbal analogy. We chose this subscale due to its capability to assess intellectual ability from the earliest stages of development effectively, its excellent psychometric properties (Andrews, 2007), and its adaptability for computerized administration, ensuring the test's independence from reading skills.
In this test, the children were required to identify one stimulus out of five to six possible alternatives by choosing an unrelated item (see Supplementary Figure S3 for an example item). Patterns progressively increased in difficulty. The test consisted of four practice trials and 51 test items. If the given answer was correct and provided within 30 s, the participants received two points. If the answer was correct and provided within 50 s, the participants received one point. Otherwise, the participant received zero points, and the next array was shown. After three consecutive incorrect answers, the test ended. Test scores could range from 0 to 102 points. The score for intellectual ability was calculated as the sum of received points.
2.3 Statistical analyses
We conducted all analyses in R version 4.2.3 (R Core Team, 2022). We ran Pearson correlations in the correlation package version 0.8.4 (Makowski et al., 2020) and hierarchical linear regression models in the stats package version 4.2.3. We used an alpha level of 5% for significance tests. For the effect sizes, we reported eta squared (η2). Values are defined as small = 0.01, medium =0.06, and large =0.14 effects, respectively. For the effect sizes Cohen's d, values are defined as small = 0.2, medium = 0.5, and large = 0.8, respectively (Field et al., 2012).
3 Results
The descriptive statistics for children's memory performance, non-verbal intelligence, confidence judgments following correct and incorrect memory performance, and monitoring discrimination are presented in Table 1.
Although the memory task turned out to be rather difficult, kindergarteners demonstrated a significant ability to distinguish between correct and incorrect answers [t(289) = −7.33, p < 0.001, d = 0.27]. They consistently assigned higher confidence judgments to correct responses compared to incorrect ones, suggesting an emerging ability to metacognitively differentiate between what they could recognize and what not. Despite these early signs of monitoring skills, inspection of Table 1 showed that the mean confidence judgments of incorrect answers were still quite high, leaving ample room for more accurate monitoring of uncertainty.
3.1 Interrelations between metacognitive monitoring, memory performance, and intellectual ability
Pearson correlations revealed significant relationships among monitoring discrimination, intellectual ability, and memory performance. A significant correlation was found between monitoring and intellectual ability (r = 0.16, p = 0.01), as well as between monitoring and memory performance (r = 0.22, p < 0.001). Furthermore, a significant correlation was also observed between memory performance and intellectual ability (r = 0.12, p = 0.04). That is, children who either had higher intellectual ability or higher memory accuracy were better able to discriminate between correct and incorrect answers. In sum, our expectations that relations between metacognitive and cognitive study variables would be positively intercorrelated were confirmed.
3.2 Impact of memory performance levels on monitoring accuracy
To investigate the influence of variations in memory performance on monitoring, that is, to explore whether there is a certain threshold of memory performance under or above which differences in monitoring will occur (unskilled-and-unaware-effect) or a non-linear relationship, we analyzed the variation in children's monitoring across memory performance quartiles. Descriptive statistics for monitoring accuracy as a function of memory performance quartile are presented in Table 2. We conducted a one-way ANOVA with monitoring discrimination as the dependent variable and memory performance (four performance quartiles) as the independent variable. The results revealed a significant difference in memory performance on monitoring discrimination, F(3, 286) = 5.04, p = 0.01, η2 = 0.05, showing that monitoring discrimination seemed to be more accurate in high compared to low performers (see Figure 2). Subsequent analyses using Tukey HSD post-hoc tests revealed a significant contrast in monitoring accuracy between groups only at the extremes of memory performance (see Supplementary Table S1). This finding supports our hypothesis that children with higher memory performance are more capable of discriminating between correct and incorrect answers than children with the lowest memory performance.
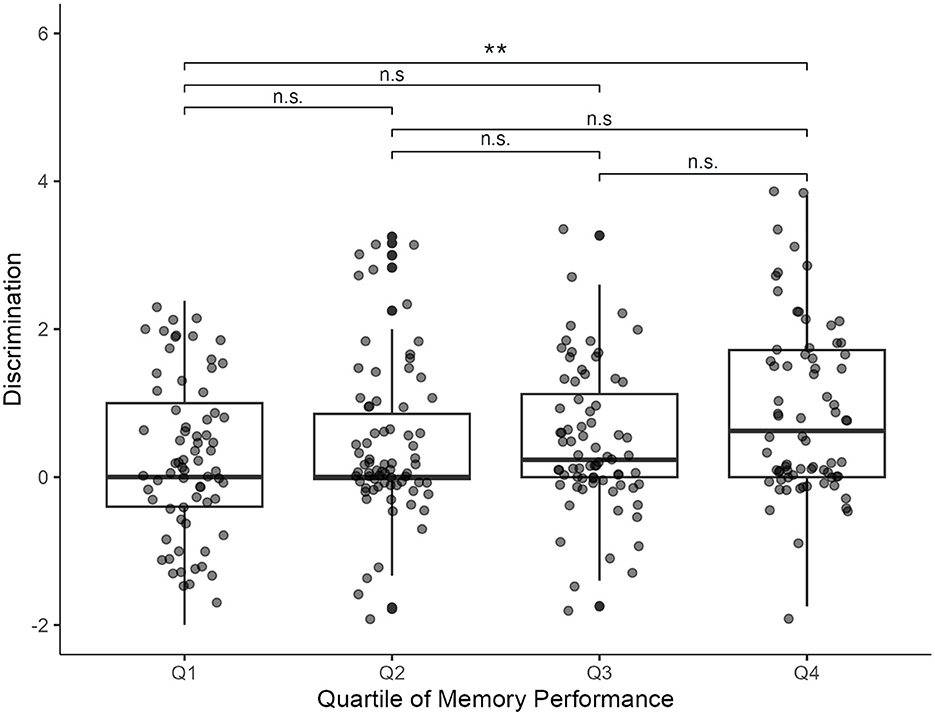
Figure 2. Distribution of monitoring discrimination as a function of memory performance quartiles. n.s, not significant, **p = 0.01.
3.3 Impact of intellectual ability levels on monitoring
To explore whether there is a non-linear relationship or whether there is a certain threshold of intellectual ability under or above which differences in monitoring will occur, we analyzed children's levels of intellectual ability by segmenting intellectual ability into quartiles. Descriptive statistics are presented in Table 3. We conducted a one-way ANOVA with monitoring discrimination as the dependent variable and intellectual ability (four quartiles) as the independent variable. The results showed no specific threshold of intellectual ability influencing monitoring discrimination [F(3, 286) = 2.25, p = 0.08, η2 = 0.02] (see Figure 3). Unexpectedly, children with high intellectual ability did not outperform those with the lowest memory performance in distinguishing between correct and incorrect answers.
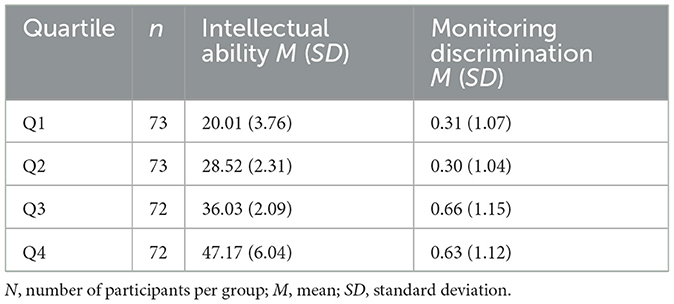
Table 3. Mean intellectual ability and monitoring discrimination as a function of intellectual ability quartiles.
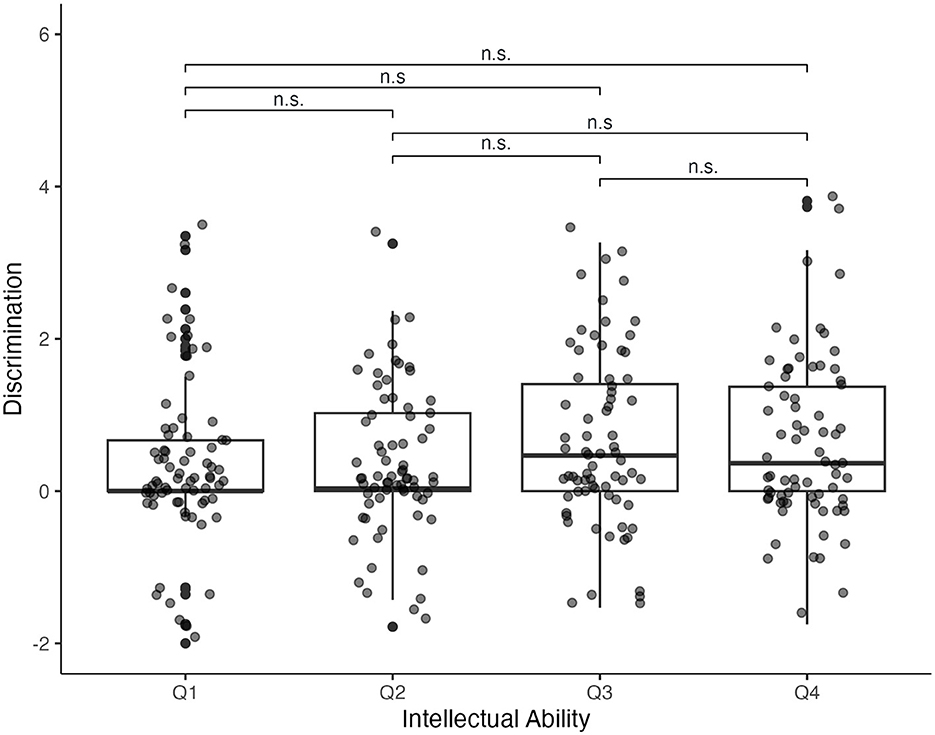
Figure 3. Distribution of monitoring discrimination as a function of intellectual ability quartiles. n.s, not significant.
3.4 Role of memory performance and intellectual abilities in predicting monitoring discrimination
To investigate the extent to which intellectual abilities can predict the monitoring of one's own thought processes beyond task performance, we conducted a three-stage hierarchical linear regression with monitoring discrimination as the dependent variable. Memory performance was introduced at stage one, followed by intellectual ability at stage two, and their interaction at stage three. Prior to conducting hierarchical regression analysis, several assumptions need to be met to ensure the validity of the results. Therefore, we reviewed the linearity and homogeneity of our final hierarchical model by reviewing Q-Q plots, which plot residuals against predicted values. The plot has no visible patterns, indicating that our model's assumption was not met. The Breusch-Pagan test was conducted to assess the presence of heteroscedasticity. No evidence was found χ2(2) = 0.03, p > 0.05. For the predictor variables memory performance and intellectual ability, the VIF values were found to be VIF = 1.014, indicating no issues of multicollinearity. As anticipated, Model 1 revealed that memory performance significantly predicted monitoring discrimination independently (b = 1.60, p < 0.001, R2 = 0.05), aligning with our expectations. Similarly, including intellectual ability in Model 2 explained an additional 2% of the variance (b = 0.02, p = 0.02), which is also consistent with our hypothesis. However, contrary to our expectations, the interaction between memory performance and non-verbal intelligence in Model 3 was not significant and did not yield a significant increase in explained variance (b = 0.01, p = 0.24). Both memory performance and intellectual ability were predictive of monitoring discrimination, suggesting that higher levels of memory performance and intellectual ability are associated with increased monitoring accuracy. However, the non-significant interaction indicated that the relationship between performance and monitoring discrimination does not significantly differ across levels of memory performance and intellectual ability.
4 Discussion
Our investigation explored the relationship between metacognitive monitoring, memory performance, and non-verbal intellectual ability in kindergarten children, using a paired-associate paradigm, allowing them to metacognitively monitor the accuracy of their responses in a recognition test. We investigated how task performance and intellectual ability relate to monitoring accuracy by focusing on children's ability to metacognitively discriminate between correctly and incorrectly identified pairs of items. Additionally, we sought to assess the extent to which intellectual abilities can predict the metacognitive discrimination of correct and incorrect responses beyond task performance. Finally, we explored the possibility of non-linear relationships and therefore investigated whether there are specific thresholds for memory performance and intellectual ability that, when exceeded or not exceeded, have different effects on monitoring accuracy.
In line with our expectations, we found memory children's memory performance to be positively related to metacognitive discrimination, indicating that children who recognized more item pairs demonstrated an improved ability to distinguish between their correct and incorrect responses than children with lower memory performance. This is in line with previous work showing that a higher level of test performance is linked to higher monitoring accuracy (Roebers et al., 2009; Roderer and Roebers, 2013, 2014). This underscores the significance of task performance for metacognitive judgments, even among kindergarteners (Ohtani and Hisasaka, 2018).
Additionally, our findings revealed a small correlation between children's intellectual ability and monitoring accuracy, suggesting that those with higher intellectual ability exhibited greater precision in their metacognitive monitoring. This finding supports the results of recent studies that have also found small to moderate effects between metacognitive processes and intelligence in primary school children, suggesting that these two cognitive processes are only partially dependent (Sarac et al., 2014; Ohtani and Hisasaka, 2018). Yet, the finding contrasts with Sternberg's (1999) proposition of a singular overarching higher-order cognitive construct. Furthermore, we explored whether intellectual ability could independently account for variance in metacognitive monitoring ability beyond memory performance. Consistent with our expectations and previous research, our results revealed that intellectual ability has additional explanatory value for the monitoring accuracy in kindergarten children beyond memory performance (Veenman and Beishuizen, 2004; van der Stel and Veenman, 2008, 2010). Nevertheless, the unique contribution of intellectual ability to the prediction of discrimination performance was noticeably lower compared to memory performance, possibly implying that task performance holds greater significance than intellectual ability. The absence of a significant interaction between memory performance and intellectual ability in predicting monitoring accuracy was somewhat unexpected, especially considering that both variables individually contributed to monitoring accuracy (Hannah and Shore, 1995; Neisser et al., 1996; Roth et al., 2015; Roebers, 2017).
Our results suggest that although there is a relationship between memory performance, intellectual ability, and the ability for metacognitive monitoring in young children, this is only a weak association. Thereby, our findings support previous research that cognitive and metacognitive abilities function largely independently during childhood (Veenman and Elshout, 1999; Veenman and Beishuizen, 2004). In other words, children's ability to recognize information from memory, their intellectual ability, and their ability to monitor the accuracy of their own answers appear to operate more in parallel than in close dependence on each other.
Another aim of our study was to explore the existence of specific thresholds in memory performance and intellectual ability above or below which metacognitive monitoring accuracy might be significantly affected. Our approach aimed, firstly, to identify group-specific patterns within the distributions of memory and intellectual ability that might remain masked in overall linear analysis and, secondly, to quantify the magnitude of group differences necessary to have a meaningful impact on monitoring accuracy. Thus, the sample was divided into quartiles based on their memory performance and intellectual ability.
The results obtained for memory performance levels were consistent with the pattern of the often-replicated unskilled-and-unaware effect (Kruger and Dunning, 1999). Even among kindergarten children, those who ranked in the top quartile in memory performance not only made fewer errors but were also more accurate in their metacognitive discrimination compared to their peers with the lowest memory performance quartile. This means that children with substantially better memory skills were better able to metacognitively distinguish between their correct and incorrect answers than children who struggled with the memory task. However, in this young age group, the unskilled-and-unaware-effect was only detectable between the two extreme groups of memory performance. Our results thus support Kruger and Dunning's assumption that a high level of task performance, such as knowing how to master a particular task, may be necessary to accurately judge one's own performance, which may lead to more accurate monitoring between correct and incorrect responses (Kruger and Dunning, 1999; Dunning et al., 2003; Ehrlinger et al., 2008). Another possible explanation for the present results could be that the children's metacognitive judgments are influenced by different cues at the level of the individual tasks (e.g., Koriat, 1997; see also Koriat and Levy-Sadot, 1999). It is conceivable that high-achieving vs. low-achieving children use different cues to assess their confidence (Koriat et al., 2009a,b). For example, high-achieving children may have more task-specific experience that enables them to make judgments about their own learning or work processes based on concrete information and data.
In contrast, low-performing children may rely more on intuitive judgments because they lack the necessary knowledge or experience to analyze their assessments more systematically (Schneider and Löffler, 2016). Applied to our sample, it might be that high-performing children rely on the content of information that they may know from previous experiences (Schneider, 1993; Bjorklund and Schneider, 1996). It is known that in this age group, features of the home environment, such as playing memory games with family members and discussing these experiences with parents and other relatives, play a crucial role in children's cognitive development (Gottfried, 1984; Harris and Almutairi, 2016). For example, Carr et al. (1989) showed that the amount and type of parental interaction, understanding of game rules, and task-related knowledge are positively related in second graders. In particular, children who learned strategies in situations relevant to everyday life and had access to games promoting strategic thinking showed more pronounced metacognition than their peers from families with less metacognitive, strategy-based interactions. The findings from previous studies, along with our results, suggest that early experiences with task-based knowledge and strategic thinking are likely to positively influence memory and recall.
Furthermore, young children seem to develop a kind of “expertise” through prior experiences, potentially helping them discover and apply memory strategies later on (Ornstein et al., 2006, 2008). For example, Schneider (2015) showed that children show more strategic behavior in familiar environments and in familiar tasks, such as memory tasks, compared to new tasks. This means that a wide range of experiences can help to quickly apply previous experience to new situations. Through these interactions, children learn to reflect on their own thought processes, identify effective strategies, and adapt their learning approaches accordingly. This hands-on engagement facilitated by parents is instrumental in nurturing children's metacognitive skills and enhancing their overall cognitive development (Moore et al., 1986; Laakso, 1995).
Low-performing children, on the other hand, may lack the task mastery skills to utilize information-based processing. In other words, children with lower performance levels may base their confidence on subjective feelings, such as the ease with which information comes to mind or their motivation and wishful thinking to give their best. However, this approach may not be as reliable as confidence judgments based on the processing of memory information and could, therefore, be more prone to error and result in inaccurate monitoring judgments (Efklides, 2008). However, as we did not directly measure children's previous experiences with memory games at home in our study — for example, by asking parents how often they play them at home — this explanation remains speculative. Nevertheless, future research could investigate the home environment in more detail to find out how it influences error detection and the acquisition of metacognitive monitoring skills (Schneider, 2015; Roebers, 2022). In summary, the findings from our sample suggest that during the preschool years, understanding how to effectively tackle a task is important for success in memory tasks. This implies that having at least a fundamental understanding of task-specific knowledge, likely acquired through prior experiences with memory tasks, is necessary for children to learn how to recognize errors and accurately assess resulting uncertainties (Schneider et al., 1989; Schneider, 1993; Brod, 2021).
The assumption that metacognitive discrimination in task-specific confidence judgments might also have varied as a function of intellectual ability was based on theoretical assumptions on the adaptive nature of intellectual thinking (Sternberg, 1985, 1999, 2019). This perspective suggests that children with higher intellectual ability are able to adapt their thinking more quickly to new and challenging tasks, possibly using both their advanced mental activities and metacognitive skills to respond flexibly to new and challenging memory tasks. Our results did not align with this assumption. That is, our findings indicated that in our sample kindergarteners with the highest intellectual abilities did not demonstrate advanced metacognitive adaptability in the novel task by showing higher monitoring accuracy compared to children with average or below-average intellectual abilities. This finding is noteworthy considering previous research exploring differences in metacognition between gifted and non-gifted children (Alexander et al., 1995; Alexander and Schwanenflugel, 1996; Snyder et al., 2011; Efklides, 2019; Straka et al., 2021; Tibken et al., 2022). Several explanations could be considered for our unexpected finding. Firstly, our results do not necessarily contradict previous research on giftedness, as it could be that our sample did not include children who fall into this specific range of giftedness (Alexander et al., 1995; Efklides, 2019), and it was also not our primary interest to include specifically gifted children. Secondly, despite the apparent homogeneity of the overall sample, variations or disparities within the quartiles could have influenced the relationship between the variables. Such intra-quartile variability may preclude the identification of consistent linear patterns in the relationships between these variables, which we did find in the regression analysis (van der Stel and Veenman, 2014). Thirdly, the methodology for measuring intellectual ability might not have adequately captured the specific relationships between the quartiles. Relying solely on non-verbal abilities as a measure of intelligence is a limitation in our approach (Ohtani and Hisasaka, 2018). This restricted approach may fail to capture other, more crucial aspects of intelligence, such as verbal abilities, problem-solving skills, and cognitive flexibility. Future research could explore alternative methodologies for measuring intellectual ability that encompass a broader range of cognitive functions, including both verbal and non-verbal abilities, for achieving a more comprehensive measurement of intelligence.
It is also worth noting that participants in previous studies were older than those in our study, which may have implications for the interpretation of our findings. Specifically, young children's confidence judgments may be more strongly influenced by motivational factors compared to other participants (Efklides, 2019). For example, young children are known to base their monitoring – at least in part – on their desire for good performance (wishful thinking; Schneider, 1998) or their effort in completing the task well (effort heuristic; Koriat et al., 2009a). The often observed monitoring accuracy increases in older children can thus also be attributed to decreasing self-protective biases, especially as they typically and more frequently acknowledge the possibility of errors (Efklides and Tsiora, 2002; van Loon et al., 2017; van Loon and Roebers, 2020).
4.1 Limitations
Our study, while providing important and new insights, is not without limitations. Firstly, our study's correlational design necessitates a cautious interpretation of the findings, particularly given the assumed interplay between cognitive and metacognitive factors (Flavell, 1979; Zimmerman, 1995; Boekaerts, 1999; Dignath and Büttner, 2008). While we observed a relationship between monitoring accuracy and memory performance, we did not investigate a possible bidirectional nature of cognitive and metacognitive processes, as discussed in self-regulation models (e.g., Efklides, 2008, 2011). It is equally plausible that high monitoring accuracy fosters improved memory performance, potentially through enhanced metacognitive regulation and more effective use of learning strategies (Lockl and Schneider, 2007; Roebers et al., 2014; Godfrey et al., 2023). This possibility aligns with the notion that metacognitive experiences and internal feedback loops generated through repeated exposure to learning materials—as was the case with the 16 items in our study—play a critical role in shaping learning behavior and performance (Efklides, 2006; Efklides and Metallidou, 2020). Therefore, our correlational findings could reflect a dynamic and reciprocal relationship between monitoring accuracy and memory performance rather than a unidirectional influence. To disentangle these complex interactions and clarify the directionality of these relationships, future research should employ longitudinal or experimental designs, which would allow for a more detailed exploration of the causality and bidirectionality between these key factors in learning.
Secondly, given the practical feasibility constraints of conducting a large-scale intervention study (as a reminder, this study utilized pretest data), we were compelled to strike a balance between conducting a comprehensive assessment and addressing the practical challenges associated with testing young children. As a result, we chose to assess intellectual ability exclusively through the Odd-Item Out subscale of the Reynolds scale (i.e., RIAS; Hagmann-von Arx and Grob, 2014) to provide an economical and largely stress-free test experience for our young participants. We acknowledge this choice as a limitation and recommend that future research should encompass a broader range of intellectual ability measures. Finally, although the paired-associates test was designed to be challenging, the actual performance was somewhat low (M = 33%, SD = 13%). Although there were no pronounced floor effects, it is possible that the somewhat poor memory performance, on average, has impacted the results.
5 Conclusion
In summary, this study analyzed the relationships between metacognitive monitoring, memory performance, and intellectual abilities in a sample of kindergarten children based on their metacognitive ability to discriminate between correct and incorrect responses in a memory task. While the findings revealed that children's monitoring accuracy did relate to memory performance and intellectual ability, the extent of this relationship, especially for intellectual ability, was less significant than expected. The small correlation suggests that cognitive and metacognitive abilities function rather independently in kindergarteners. Furthermore, our analysis of group differences revealed that only the extreme ranges of task performance—specifically, very low and very high levels—affected kindergarteners' ability to accurately distinguish between correct and incorrect answers. In contrast, variations in intellectual ability, whether at lower or higher levels, did not impact children's monitoring accuracy. Thus, our findings emphasize the importance of task performance yielding direct and positive effects on metacognitive monitoring, while intellectual abilities appear to play a more subordinate role. Against this background, the present study underscores the necessity to include different cognitive factors operating at the task at hand to increase our understanding regarding children's early stages of metacognitive development.
Data availability statement
The raw data supporting the conclusions of this article will be made available by the authors, without undue reservation.
Ethics statement
The studies involving humans were approved by the Ethics Committee Department of Psychology, University of Bern. The studies were conducted in accordance with the local legislation and institutional requirements. Written informed consent for participation in this study was provided by the participants' legal guardians/next of kin.
Author contributions
KK: Conceptualization, Formal analysis, Investigation, Methodology, Project administration, Visualization, Writing – original draft. CR: Conceptualization, Funding acquisition, Methodology, Supervision, Writing – review & editing.
Funding
The author(s) declare that financial support was received for the research, authorship, and/or publication of this article. The research leading to these results received funding from the Swiss National Science Foundation under Grant agreement no. 197336. The grant was awarded to CR.
Conflict of interest
The authors declare that the research was conducted in the absence of any commercial or financial relationships that could be construed as a potential conflict of interest.
Publisher's note
All claims expressed in this article are solely those of the authors and do not necessarily represent those of their affiliated organizations, or those of the publisher, the editors and the reviewers. Any product that may be evaluated in this article, or claim that may be made by its manufacturer, is not guaranteed or endorsed by the publisher.
Supplementary material
The Supplementary Material for this article can be found online at: https://www.frontiersin.org/articles/10.3389/fdpys.2024.1417197/full#supplementary-material
References
Alexander, J. M., Carr, M., and Schwanenflugel, P. J. (1995). Development of metacognition in gifted children: directions for future research. Dev. Rev. 15, 1–37. doi: 10.1006/drev.1995.1001
Alexander, J. M., Johnson, K. E., Albano, J., Freygang, T., and Scott, B. (2006). Relations between intelligence and the development of metaconceptual knowledge. Metacognit. Learn. 1, 51–67. doi: 10.1007/s11409-006-6586-8
Alexander, J. M., and Schwanenflugel, P. J. (1996). Development of metacognitive concepts about thinking in gifted and non-gifted children: recent research. Learn. Ind. Diff. 8, 305–325. doi: 10.1016/S1041-6080(96)90021-7
Andrews, J. J. W. (2007). Test Reviews: Reynolds, C. R., and Kamphaus, R. W. (2003). RIAS: Reynolds Intellectual Assessment Scales. Lutz, FL: Psychological Assessment Resources, Inc. J. Psychoeduc. Assessm. 25, 402–408. doi: 10.1177/0734282907300381
Bayard, N. S., van Loon, M. H., Steiner, M., and Roebers, C. M. (2021). Developmental improvements and persisting difficulties in children's metacognitive monitoring and control skills: cross-sectional and longitudinal perspectives. Child Dev. 92, 1118–1136. doi: 10.1111/cdev.13486
Bjorklund, D. F., and Schneider, W. (1996). The interaction of knowledge, aptitude, and strategies in children's memory performance. Adv. Child Dev. Behav. 26, 59–89. doi: 10.1016/S0065-2407(08)60506-6
Blair, C., and Raver, C. C. (2015). School readiness and self-regulation: a developmental psychobiological approach. Ann. Rev. Psychol. 66, 711–731. doi: 10.1146/annurev-psych-010814-015221
Boekaerts, M. (1999). Self-regulated learning: where we are today. Int. J. Educ. Res. 31, 445–457. doi: 10.1016/S0883-0355(99)00014-2
Bol, L., and Hacker, D. (2012). Calibration research: Where do we go from here? Front. Psychol. 3:229. doi: 10.3389/fpsyg.2012.00229
Brod, G. (2021). Generative learning: Which strategies for what age? Educ. Psychol. Rev. 33, 1295–1318.
Buehler, F. J., van Loon, M. H., Bayard, N. S., Steiner, M., and Roebers, C. M. (2021). Comparing metacognitive monitoring between native and non-native speaking primary school students. Metacogn. Learn. 16, 749–768. doi: 10.1007/s11409-021-09261-z
Carr, M., Alexander, J., and Folds-Bennett, T. (1994). Metacognition and mathematics strategy use. Appl. Cognit. Psychol. 8, 583–595. doi: 10.1002/acp.2350080605
Carr, M., Alexander, J., and Schwanenflugel, P. (1996). Where gifted children do and do not excel on metacognitive tasks. Roeper Rev. 18, 212–217. doi: 10.1080/02783199609553740
Carr, M., Kurtz, B. E., Schneider, W., Turner, L. A., and Borkowski, J. G. (1989). Strategy acquisition and transfer among American and German children: environmental influences on metacognitive development. Dev. Psychol. 25, 765–771. doi: 10.1037/0012-1649.25.5.765
Coughlin, C., Hembacher, E., Lyons, K. E., and Ghetti, S. (2015). Introspection on uncertainty and judicious help-seeking during the preschool years. Dev. Sci. 18, 957–971. doi: 10.1111/desc.12271
Crane, N., Zusho, A., Ding, Y., and Cancelli, A. (2017). Domain-specific metacognitive calibration in children with learning disabilities. Contemp. Educ. Psychol. 50, 72–79. doi: 10.1016/j.cedpsych.2016.09.006
Desoete, A., Roeyers, H., and Huylebroeck, A. (2006). Metacognitive skills in Belgian third grade children (age 8 to 9) with and without mathematical learning disabilities. Metacognit. Learn. 1, 119–135. doi: 10.1007/s11409-006-8152-9
Destan, N., and Roebers, C. M. (2015). What are the metacognitive costs of young children's overconfidence? Metacognit. Learn. 10, 347–374. doi: 10.1007/s11409-014-9133-z
Dignath, C., and Büttner, G. (2008). Components of fostering self-regulated learning among students. A meta-analysis on intervention studies at primary and secondary school level. Metacognit. Learn. 3, 231–264. doi: 10.1007/s11409-008-9029-x
Dunning, D., Johnson, K., Ehrlinger, J., and Kruger, J. (2003). Why people fail to recognize their own incompetence. Curr. Direct. Psychol. Sci. 12, 83–87. doi: 10.1111/1467-8721.01235
Eckhardt, A. G., and Egert, F. (2020). Predictors for the quality of family child care: a meta-analysis. Childr. Youth Serv. Rev. 116:105205. doi: 10.1016/j.childyouth.2020.105205
Efklides, A. (2006). Metacognitive experiences: the missing link in the self-regulated learning process. Educ. Psychol. Rev. 18, 287–291. doi: 10.1007/s10648-006-9021-4
Efklides, A. (2008). Metacognition: defining its facets and levels of functioning in relation to self-regulation and co-regulation. Eur. Psychol. 13, 277–287. doi: 10.1027/1016-9040.13.4.277
Efklides, A. (2011). Interactions of metacognition with motivation and affect in self-regulated learning: the MASRL model. Educ. Psychol. 46, 6–25. doi: 10.1080/00461520.2011.538645
Efklides, A. (2019). Gifted students and self-regulated learning: the MASRL model and its implications for SRL. High Ability Stu. 30, 79–102. doi: 10.1080/13598139.2018.1556069
Efklides, A., and Metallidou, P. M. (2020). Applying Metacognition and Self-Regulated Learning in the Classroom. The Oxford Encyclopedia of Educational Psychology. Oxford: Oxford University Press. doi: 10.1093/acrefore/9780190264093.013.961
Efklides, A., and Tsiora, A. (2002). Metacognitive experiences, self-concept, and self-regulation. Psychol. Int. J. Psychol. Orient 45, 222–236. doi: 10.2117/psysoc.2002.222
Ehrlinger, J., Johnson, K., Banner, M., Dunning, D., and Kruger, J. (2008). Why the unskilled are unaware: further explorations of (absent) self-insight among the incompetent. Org. Behav. Hum. Dec. Proc. 105, 98–121. doi: 10.1016/j.obhdp.2007.05.002
Field, A., Miles, J., and Field, Z. (2012). Discovering Statistics Using R. London: Sage Publications.
Flavell, J. H. (1979). Metacognition and cognitive monitoring: A new area of cognitive–developmental inquiry. Am. Psychol. 34, 906–911. doi: 10.1037/0003-066X.34.10.906
Flavell, J. H., Green, F. L., Flavell, E. R., and Grossman, J. B. (1997). The development of children's knowledge about inner speech. Child Dev. 68, 39–47. doi: 10.2307/1131923
Fleming, S. M., and Lau, H. C. (2014). How to measure metacognition. Front. Hum. Neurosci. 8:443. doi: 10.3389/fnhum.2014.00443
Fukkink, R. G., Sluiter, R. M. V., and Fekkes, M. (2024). Transition from childcare to school: surgency, center-based care and caregiver-child relationship predict self-regulation, social competence and well-being. Learn. Ind. Diff. 110:102409. doi: 10.1016/j.lindif.2024.102409
Ghetti, S., Hembacher, E., and Coughlin, C. A. (2013). Feeling uncertain and acting on it during the preschool years: a metacognitive approach. Child Dev. Persp. 7, 160–165. doi: 10.1111/cdep.12035
Godfrey, M., Casnar, C., Stolz, E., Ailion, A., Moore, T., Gioia, G., et al. (2023). A review of procedural and declarative metamemory development across childhood. Child Neuropsychol. 29, 183–212. doi: 10.1080/09297049.2022.2055751
Gottfried, A. W. W. (1984). “Home environment and early cognitive development: Integration, meta-analyses, and conclusions,” in Home Environment and Early Cognitive Development: Longitudinal Research, ed. A. W. Gottfried (London: Academic Press), 329–342.
Hacker, D. J., Bol, L., and Bahbahani, K. (2008). Explaining calibration accuracy in classroom contexts: the effects of incentives, reflection, and explanatory style. Metacognit. Learn. 3, 101–121. doi: 10.1007/s11409-008-9021-5
Hacker, D. J., Bol, L., Horgan, D. D., and Rakow, E. A. (2000). Test prediction and performance in a classroom context. J. Educ. Psychol. 92, 160–170. doi: 10.1037/0022-0663.92.1.160
Hagmann-von Arx, P., and Grob, A. (2014). RIAS - Reynolds Intellectual Assessment Scales and Screening: Deutschsprachige Adaptation der Reynolds Intellectual Assessment Scales (RIAS). London: Hans Huber.
Händel, M., and Bukowski, A. K. (2019). The gap between desired and expected performance as predictor for judgment confidence. J. Appl. Res. Mem. Cognit. 8, 347–354. doi: 10.1016/j.jarmac.2019.05.005
Händel, M., and Dresel, M. (2018). Confidence in performance judgment accuracy: the unskilled and unaware effect revisited. Metacognit. Learn. 13, 265–285. doi: 10.1007/s11409-018-9185-6
Händel, M., and Fritzsche, E. S. (2016). Unskilled but subjectively aware: metacognitive monitoring ability and respective awareness in low-performing students. Mem. Cognit. 44, 229–241. doi: 10.3758/s13421-015-0552-0
Hannah, C. L., and Shore, B. M. (1995). Metacognition and high intellectual ability: Insights from the study of learning-disabled gifted students. Gifted Child Q. 39, 95–109. doi: 10.1177/001698629503900206
Harris, Y. R., and Almutairi, S. (2016). A commentary on parent–child cognitive learning interaction research: What have we learned from two decades of research? Front. Psychol. 7:1210. doi: 10.3389/fpsyg.2016.01210
Hodges, B., Regehr, G., and Martin, D. (2001). Difficulties in recognizing one's own incompetence: novice physicians who are unskilled and unaware of it. Acad. Med. 76:S87. doi: 10.1097/00001888-200110001-00029
Job, J. M., and Klassen, R. M. (2012). Predicting performance on academic and non-academic tasks: a comparison of adolescents with and without learning disabilities. Contemp. Educ. Psychol. 37, 162–169. doi: 10.1016/j.cedpsych.2011.05.001
Klassen, R. M. (2007). Using predictions to learn about the self-efficacy of early adolescents with and without learning disabilities. Contemp. Educ. Psychol. 32, 173–187. doi: 10.1016/j.cedpsych.2006.10.001
Koriat, A. (1997). Monitoring one's own knowledge during study: a cue-utilization approach to judgments of learning. J. Exp. Psychol. Gen. 126, 349–370. doi: 10.1037/0096-3445.126.4.349
Koriat, A., Ackerman, R., Lockl, K., and Schneider, W. (2009a). The easily learned, easily remembered heuristic in children. Cognit. Dev. 24, 169–182. doi: 10.1016/j.cogdev.2009.01.001
Koriat, A., Ackerman, R., Lockl, K., and Schneider, W. (2009b). The memorizing effort heuristic in judgments of learning: a developmental perspective. J. Exp. Child Psychol. 102, 265–279. doi: 10.1016/j.jecp.2008.10.005
Koriat, A., and Levy-Sadot, R. (1999). “Processes underlying metacognitive judgments: Information-based and experience-based monitoring of one's own knowledge,” in Dual-Process Theories in Social Psychology, eds. S. Chaiken and Y. Trope (London: Guilford), 483–502.
Koriat, A., and Shitzer-Reichert, R. (2002). “Metacognitive judgments and their accuracy,” in Metacognition: Process, Function and Use, eds. P. Chambres, M. Izaute, and P.-J. Marescaux (New York, NY: Springer US), 1–17.
Kruger, J., and Dunning, D. (1999). Unskilled and unaware of it: how difficulties in recognizing one's own incompetence lead to inflated self-assessments. J. Pers. Soc. Psychol. 77, 1121–1134. doi: 10.1037/0022-3514.77.6.1121
Laakso, M. L. (1995). Mothers' and fathers' communication clarity and teaching strategies with their school-aged children. J. Appl. Dev. Psychol. 16, 445–461. doi: 10.1016/0193-3973(95)90029-2
Lockl, K., and Schneider, W. (2007). Knowledge about the mind: links between theory of mind and later metamemory. Child Dev. 78, 148–167. doi: 10.1111/j.1467-8624.2007.00990.x
Lucangeli, D., Coi, G., and Bosco, P. (1997). Metacognitive awareness in good and poor math problem solvers. Learn. Disab. Res. Prac. 12, 209–212.
Lucangeli, D., Fastame, M. C., Pedron, M., Porru, A., Duca, V., Hitchcott, P. K., et al. (2019). Metacognition and errors: the impact of self-regulatory trainings in children with specific learning disabilities. ZDM 51, 577–585. doi: 10.1007/s11858-019-01044-w
Lyons, K. E., and Ghetti, S. (2011). The development of uncertainty monitoring in early childhood. Child Dev. 82, 1778–1787. doi: 10.1111/j.1467-8624.2011.01649.x
Makowski, D., Ben-Shachar, M. S., Patil, I., and Lüdecke, D. (2020). Methods and algorithms for correlation analysis in R. J. Open Source Softw. 5:2306. doi: 10.21105/joss.02306
Moore, J. J., Mullis, R. L., and Mullis, A. K. (1986). Examining metamemory within the context of parent-child interactions. Psychol. Rep. 59, 39–47. doi: 10.2466/pr0.1986.59.1.39
Neisser, U., Boodoo, G., Bouchard Jr, T. J, Boykin, A. W., Brody, N., et al. (1996). Intelligence: knowns and unknowns. Am. Psychol. 51:77. doi: 10.1037/0003-066X.51.2.77
Nelson, T. O., and Narens, L. (1990). “Metamemory: a theoretical framework and new findings,” in Psychology of Learning and Motivation: Advances in Research and Theory, Vol. 26, ed. G. H. Bower (London: Academic Press), 125–173.
Ohtani, K., and Hisasaka, T. (2018). Beyond intelligence: a meta-analytic review of the relationship among metacognition, intelligence, and academic performance. Metacogni. Learn. 13, 179–212. doi: 10.1007/s11409-018-9183-8
Ornstein, P. A., Haden, C. A., and Elischberger, H. B. (2006). Children's Memory Development: Remembering the Past and Preparing for the Future. Lifespan Cognition: Mechanisms of Change (Oxford: Oxford University Press), 143–161.
Ornstein, P. A., Haden, C. A., and San Souci, P. (2008). “The development of skilled remembering in children,” in Earning and Memory: A Comprehensive Reference, Vol. 2, eds. H. L. Roediger III and J. H. Byrne (Amsterdam: Elsevier), 715–744.
R Core Team (2022). A Language and Environment for Statistical Computing. Vienna: R Foundation for Statistical Computing (Version 4.2.1) [Computer software]./
Reynolds, C. R., and Kamphaus, R. W. (2009). “Development and application of the Reynolds intellectual assessment scales (RIAS),” in Practitioner's Guide to Assessing Intelligence and Achievement, eds. J. A. Naglieri and S. Goldstein (New York, NY: John Wiley and Sons Inc), 95–126.
Roderer, T., and Roebers, C. (2013). Children's performance estimation in mathematics and science tests over a school year: a pilot study. Electr. J. Res. Educ. Psychol. 11, 5–24.
Roderer, T., and Roebers, C. M. (2014). Can you see me thinking (about my answers)? Using eye-tracking to illuminate developmental differences in monitoring and control skills and their relation to performance. Metacognit. Learn. 9, 1–23. doi: 10.1007/s11409-013-9109-4
Roebers, C. M. (2017). Executive function and metacognition: towards a unifying framework of cognitive self-regulation. Dev. Rev. 45, 31–51. doi: 10.1016/j.dr.2017.04.001
Roebers, C. M. (2022). “The development of semantic memory: the role of memory strategies and metacognition,” in The Development of Memory in Infancy and Childhood, eds. M. L. Courage and N. Cowan (London: Routledge).
Roebers, C. M., Krebs, S. S., and Roderer, T. (2014). Metacognitive monitoring and control in elementary school children: their interrelations and their role for test performance. Learn. Ind. Diff. 29, 141–149. doi: 10.1016/j.lindif.2012.12.003
Roebers, C. M., Schmid, C., and Roderer, T. (2009). Metacognitive monitoring and control processes involved in primary school children's test performance. Br. J. Educ. Psychol. 79, 749–767. doi: 10.1348/978185409X429842
Roth, B., Becker, N., Romeyke, S., Schäfer, S., Domnick, F., Spinath, F. M., et al. (2015). Intelligence and school grades: a meta-analysis. Intelligence 53, 118–137. doi: 10.1016/j.intell.2015.09.002
Sarac, S., Önder, A., and Karakelle, S. (2014). The relations among general intelligence, metacognition and text learning performance. Egitim ve Bilim 39:173.
Schneider, W. (1993). Domain-specific knowledge and memory performance in children. Educ. Psychol. Rev. 5, 257–273. doi: 10.1007/BF01323047
Schneider, W. (1998). Performance prediction in young children: effects of skill, metacognition and wishful thinking. Dev. Sci. 1, 291–297. doi: 10.1111/1467-7687.00044
Schneider, W. (2015). Memory Development From Early Childhood Through Emerging Adulthood, 1st Edn. Cham: Springer International Publishing.
Schneider, W., Körkel, J., and Weinert, F. (1987). The effects of intelligence, self-concept, and attributional style on metamemory and memory behaviour. Int. J. Behav. Dev. 10, 281–299. doi: 10.1177/016502548701000302
Schneider, W., Körkel, J., and Weinert, F. E. (1989). Domain-specific knowledge and memory performance: a comparison of high- and low-aptitude children. J. Educ. Psychol. 81, 306–312. doi: 10.1037/0022-0663.81.3.306
Schneider, W., and Löffler, E. (2016). “The development of metacognitive knowledge in children and adolescents,” in The Oxford Handbook of Metamemory, eds. J. Dunlosky and Sarah (Uma) K. Tauber (Oxford: Oxford University Press), 491–518.
Schneider, W., and Pressley, M. (1997). Memory Development Between Two and Twenty, 2nd Edn. Psychology Press. doi: 10.4324/9780203774496
Schraw, G. (2009). A conceptual analysis of five measures of metacognitive monitoring. Metacognit. Learn. 4, 33–45. doi: 10.1007/s11409-008-9031-3
Serra, M. J., and DeMarree, K. G. (2016). Unskilled and unaware in the classroom: college students' desired grades predict their biased grade predictions. Memory Cognit. 44, 1127–1137. doi: 10.3758/s13421-016-0624-9
Shore, B. M., and Dover, A. C. (1987). Metacognition, intelligence and giftedness. Gifted Child Q. 31, 37–39. doi: 10.1177/001698628703100108
Simons, D. J. (2013). Unskilled and optimistic: overconfident predictions despite calibrated knowledge of relative skill. Psychon. Bullet. Rev. 20, 601–607. doi: 10.3758/s13423-013-0379-2
Snyder, K. E., Nietfeld, J. L., and Linnenbrink-Garcia, L. (2011). Giftedness and metacognition: A short-term longitudinal investigation of metacognitive monitoring in the classroom. Gifted Child Q. 55, 181–193. doi: 10.1177/0016986211412769
Sternberg, R. J. (1980). Sketch of a componential subtheory of human intelligence. Behav. Brain Sci. 3, 573–584. doi: 10.1017/S0140525X00006932
Sternberg, R. J. (1985). Beyond IQ: A Triarchic Theory of Human Intelligence. Cambridge: Cambridge University Press.
Sternberg, R. J. (1988). The Triarchic Mind: A New Theory of Human Intelligence. London: Viking Penguin.
Sternberg, R. J. (1999). Intelligence as developing expertise. Contemp. Educ. Psychol. 24, 359–375. doi: 10.1006/ceps.1998.0998
Sternberg, R. J. (2019). A theory of adaptive intelligence and its relation to general intelligence. J. Int. 7:23. doi: 10.3390/jintelligence7040023
Straka, O., Portešová, Š., Halámková, D., and Jaburek, M. (2021). Metacognitive monitoring and metacognitive strategies of gifted and average children on dealing with deductive reasoning task. J. Eye Movem. Res. 14:4. doi: 10.16910/jemr.14.4.1
Swanson, H. L. (1992). The relationship between metacognition and problem solving in gifted children. Roeper Rev. 15:43. doi: 10.1080/02783199209553457
Tibken, C., Richter, T., von der Linden, N., Schmiedeler, S., and Schneider, W. (2022). The role of metacognitive competences in the development of school achievement among gifted adolescents. Child Dev. 93, 117–133. doi: 10.1111/cdev.13640
van der Stel, M., and Veenman, M. V. J. (2008). Relation between intellectual ability and metacognitive skillfulness as predictors of learning performance of young students performing tasks in different domains. Learn. Ind. Diff. 18, 128–134. doi: 10.1016/j.lindif.2007.08.003
van der Stel, M., and Veenman, M. V. J. (2010). Development of metacognitive skillfulness: a longitudinal study. Learn. Ind. Diff. 20, 220–224. doi: 10.1016/j.lindif.2009.11.005
van der Stel, M., and Veenman, M. V. J. (2014). Metacognitive skills and intellectual ability of young adolescents: a longitudinal study from a developmental perspective. Eur. J. Psychol. Educ. 29, 117–137. doi: 10.1007/s10212-013-0190-5
van Loon, M., Destan, N., Spiess, M. A., de Bruin, D, and Roebers, A. (2017). Developmental progression in performance evaluations: Effects of children's cue-utilization and self-protection. Learn. Instr. 51, 47–60. doi: 10.1016/j.learninstruc.2016.11.011
van Loon, M. H., and Roebers, C. M. (2020). Using feedback to improve monitoring judgment accuracy in kindergarten children. Early Childhood Res. Q. 53, 301–313. doi: 10.1016/j.ecresq.2020.05.007
van Loon, M. H., and Roebers, C. M. (2021). “Using feedback to support children when monitoring and controlling their learning,” in Trends and Prospects in Metacognition Research Across the Life Span, eds. D. Moraitou and P. Metallidou (Cham: Springer International Publishing), 161–184.
Veenman, M., and Elshout, J. J. (1999). Changes in the relation between cognitive and metacognitive skills during the acquisition of expertise. Eur. J. Psychol. Educ. 14, 509–523. doi: 10.1007/BF03172976
Veenman, M. V. J., and Beishuizen, J. J. (2004). Intellectual and metacognitive skills of novices while studying texts under conditions of text difficulty and time constraint. Learn. Instr. 14, 621–640. doi: 10.1016/j.learninstruc.2004.09.004
Veenman, M. V. J., and Spaans, M. A. (2005). Relation between intellectual and metacognitive skills: Age and task differences. Learn. Ind. Diff. 15, 159–176. doi: 10.1016/j.lindif.2004.12.001
Keywords: monitoring, memory, non-verbal intellectual ability, metacognition, discrimination, unskilled-and-unaware, intelligence
Citation: Kolloff K and Roebers CM (2024) The relationship between metacognitive monitoring, non-verbal intellectual ability, and memory performance in kindergarten children. Front. Dev. Psychol. 2:1417197. doi: 10.3389/fdpys.2024.1417197
Received: 14 April 2024; Accepted: 14 June 2024;
Published: 02 July 2024.
Edited by:
Kim P. Roberts, Wilfrid Laurier University, CanadaReviewed by:
Elena Escolano-Pérez, University of Zaragoza, SpainAna Clara Ventura, National Scientific and Technical Research Council (CONICET), Argentina
Copyright © 2024 Kolloff and Roebers. This is an open-access article distributed under the terms of the Creative Commons Attribution License (CC BY). The use, distribution or reproduction in other forums is permitted, provided the original author(s) and the copyright owner(s) are credited and that the original publication in this journal is cited, in accordance with accepted academic practice. No use, distribution or reproduction is permitted which does not comply with these terms.
*Correspondence: Claudia M. Roebers, Y2xhdWRpYS5yb2ViZXJzQHVuaWJlLmNo