- 1Department of Psychology, Queen Mary University of London, London, United Kingdom
- 2NeuroCognitive Imaging Lab, Department of Psychology and Neuroscience, Dalhousie University, Halifax, NS, Canada
- 3Département Sciences humaines, Lettres et Communication, Université TÉLUQ, Québec, QC, Canada
- 4School of Psychology and Life Sciences, Canterbury Christ Church University, Canterbury, United Kingdom
- 5Language and Literacy Lab, Department of Psychology and Neuroscience, Dalhousie University, Halifax, NS, Canada
Introduction: As children become independent readers, they regularly encounter new words whose meanings they must infer from context, and whose spellings must be learned for future recognition. The self-teaching hypothesis proposes orthographic learning skills are critical in the transition to fluent reading, while the lexical quality hypothesis further emphasizes the importance of semantics. Event-related potential (ERP) studies of reading development have focused on effects related to the N170 component—print tuning (letters vs. symbols) and lexical tuning (real words vs. consonant strings)—as well as the N400 reflecting semantic processing, but have not investigated the relationship of these components to word learning during independent reading.
Methods: In this study, children in grade 3 independently read short stories that introduced novel words, then completed a lexical decision task from which ERPs were derived.
Results: Like real words, newly-learned novel words evoked a lexical tuning effect, indicating rapid establishment of orthographic representations. Both real and novel words elicited significantly smaller N400s than pseudowords, suggesting that semantic representations of the novel words were established. Further, N170 print tuning predicted accuracy on identifying the spellings of the novel words, while the N400 effect for novel words was associated with reading comprehension.
Discussion: Exposure to novel words during self-directed reading rapidly establishes neural markers of orthographic and semantic processing. Furthermore, the ability to rapidly filter letter strings from symbols is predictive of orthographic learning, while rapid establishment of semantic representations of novel words is associated with stronger reading comprehension.
1 Introduction
Reading is an essential prerequisite for learning in school and ultimately in the working world. In becoming skilled readers, children transition from decoding letters into sounds (phonemes) to efficiently mapping printed words to their stored representations of sounds and meanings (Chall, 1996; Fitzgerald and Shanahan, 2000; Dyer et al., 2003). The extent to which children have acquired representations of the spellings for the novel words encountered through reading experience is termed orthographic knowledge, and the process by which orthographic knowledge is developed is termed orthographic learning (Share, 1999; Bowey and Miller, 2007). While phonemic skills—such as awareness and decoding—are critical at the earliest stages of reading development, orthographic learning is thought to be more predictive of reading ability once children start to become skilled readers (Share, 2008; Wang et al., 2013; Nation and Castles, 2017). Orthographic learning reflects skill in acquiring representations of the spellings of individual words, which builds more orthographic knowledge (Share, 1999; Bowey and Miller, 2007). By building orthographic knowledge, orthographic learning is hypothesized to be a mechanism by which this transition to skilled reading occurs at the level of individual words (Share, 2008). Indeed, it has been suggested that orthographic learning skills can serve as a measure of effective transition from the novice to skilled reading (Wang et al., 2013). The self-teaching hypothesis suggests that orthographic learning is critical in supporting children's transition to skilled reading (Share, 2008, 2011; Wang et al., 2011; Nation and Castles, 2017; Mimeau et al., 2018; Deacon et al., 2019).
Much of children's written vocabulary development occurs during independent reading, during which they regularly encounter unfamiliar words, and need to figure out how these map onto existing lexical representations—or infer their meanings from context—as well as establishing memory traces for the spellings and meanings that will facilitate reading when the words are encountered later. Behavioral paradigms used to study this process typically involve only a very few (e.g., four) exposures to novel words, and assess recognition shortly after exposure. This begs the question of when these newly-introduced letter strings actually become “words” in children's brains, and whether these items are on their way to becoming new lexical representations.
One approach to gain deeper understanding into the word-learning process is using event-related potentials (ERPs)—signals recorded using electroencephalographic (EEG) neuroimaging and time-locked to the presentation of specific stimuli. ERPs provide exquisite temporal resolution, which can reveal a sequence of different neurocognitive operations occurring in the first few hundred milliseconds after a word is read. However, little developmental ERP work has focused on the process of word learning, and none to our knowledge has looked at novel word learning via independent reading, which has been the focus of insightful behavioral work. In the present study our goal was to determine whether ERPs provide evidence supporting the assumption that a few brief exposures to novel words during independent reading is sufficient to establish neural responses reflecting wordform recognition and meaning integration. We also explored possible relationships between ERPs elicited in this context, orthographic and semantic learning performance, and standardized measures of reading ability.
1.1 Behavioral studies of word learning in reading development
The self-teaching hypothesis has emphasized the role of orthographic learning over existing orthographic or semantic knowledge, or the phonological skills critical in earlier reading development (Share, 2008, 2011; Nation and Castles, 2017; Mimeau et al., 2018). The lexical quality hypothesis, on the other hand, focuses on the importance of high-quality lexical representations (in particular semantics) in the development of reading comprehension skills (Perfetti and Hart, 2002; Perfetti, 2017). The term “lexical quality” refers to the distinctiveness of the representations of individual words at the phonological, orthographic, and semantic levels in an individual child's mind. Reduced lexical quality can result in slowed processing times and poorer reading skills (Perfetti and Hart, 2002; Perfetti et al., 2008; Richter et al., 2013; Perfetti, 2017; Swart et al., 2017; O'Connor et al., 2019; Andrews et al., 2020).
While semantic and orthographic learning have traditionally been studied separately in behavioral studies of reading (Cain et al., 2004; Cunningham, 2006; Graves, 2006; Bowey and Miller, 2007; Ricketts et al., 2008, 2011; Ouellette and Fraser, 2009; Wang et al., 2011; Tucker et al., 2016), recent empirical work has merged these two theoretical ideas to examine ortho-semantic learning (Wang et al., 2013; Tamura et al., 2017; Mimeau et al., 2018; Deacon et al., 2019). For example, Mimeau et al. (2018) conducted a behavioral study using an ortho-semantic learning task in which children in grade 3 read (aloud) paragraphs describing new inventions. Each paragraph introduced a novel word (the name of the invention, e.g., veap) which was repeated several times in the paragraph, and the meaning of the word could be inferred through the context of the story (e.g., in which a veap is used to clean a fish tank). Children were then tested on their recognition of both the spellings and the meanings of the novel words, reflecting orthographic and semantic learning, respectively. Structural equation modeling suggested that children's ability to learn orthographic representations over this short exposure—orthographic learning—was directly predictive of their word reading fluency, and through word reading fluency predicted reading comprehension. Children's ability to learn the meanings of words (semantic learning) was directly predictive of children's reading comprehension.
Similar patterns of results emerged in a study of younger readers, in grades 1 and 2 (Deacon et al., 2019), and a new longitudinal study demonstrates that orthographic learning mediates children's gains in word reading across three years. Over the same time period, their semantic learning mediates gains in reading comprehension (Deacon et al., under review). These latter results suggest that these effects cannot be explained by a common reading factor, but that there are separable skills in learning the spellings and meanings of words, which support word reading and reading comprehension development, respectively. Together, these studies provide empirical support for the integration of the self-teaching hypothesis and the lexical quality hypothesis (Mimeau et al., 2018).
1.2 Event-related potential studies of reading development
1.2.1 The N170 reflects acquired orthographic knowledge
The N170 ERP component (sometimes labeled the N1) has been of particular interest in studies of word reading (Schendan et al., 1998; Bentin et al., 1999; Rossion et al., 2003; Maurer et al., 2005; Proverbio et al., 2008; Xue et al., 2008). The N170 is thought to reflect the earliest stages of identifying a visual object as a word, and mapping it to phonological and orthographic knowledge. In skilled adult readers, the N170 is a negative-going potential with bilateral foci largest over lateral/inferior temporal-occipital areas of the scalp, typically peaking 150–200 ms after the appearance of a printed word form (Bentin et al., 1999). It is typically left-lateralized, and is thought to reflect activity in the ventral occipito-temporal cortex, including the visual word form area. The magnitude and lateralization of the N170 show characteristic changes throughout reading development, which seem to reflect the development of visual expertise for printed words (Maurer et al., 2005, 2008; Brem et al., 2013; Zhao et al., 2014; Eberhard-Moscicka et al., 2015). Two particular N170 effects have been of particular interest, in characterizing sensitivity to print (print tuning), and sensitivity to lexical structure (lexical tuning).
1.2.1.1 Print tuning
Print tuning (or coarse tuning) is thought to reflect the brain's ability to filter plausibly word-like stimuli from non-alphabetic symbols, for further lexical processing. The emergence of print tuning has been associated with children's acquiring knowledge of the mappings between graphemes and their corresponding phonemes. For example, it is not present in kindergartners who cannot read, but emerges following grapheme-to-phoneme correspondence training (Maurer et al., 2006; Brem et al., 2010, 2013). The amplitude of the print tuning effect continues to increase in size from kindergarten to at least second grade (Maurer et al., 2006; Brem et al., 2013), if not fourth grade (Coch and Meade, 2016). It then decreases by grade 5 and further by adulthood (Brem et al., 2009; Maurer et al., 2011; Coch and Meade, 2016; Fraga-González et al., 2021). Among younger children (at least up to and including grade 2), the magnitude of the print tuning effect is associated with reading skills (including letter knowledge, fluency, and vocabulary) (Maurer et al., 2005, 2006, 2011; Bach et al., 2013; Brem et al., 2013; Eberhard-Moscicka et al., 2015; Coch and Meade, 2016). Some studies have shown changes in lateralization, such that the print tuning effect becomes relatively larger over the left, and smaller over the right, hemisphere, with increasing reading proficiency (Maurer et al., 2011; Brem et al., 2013; Zhao et al., 2014).
1.2.1.2 Lexical tuning
Lexical (or fine) tuning is thought to index sensitivity to orthographic patterns characteristic of the language. Lexical tuning refers to N170 amplitudes that are larger for real words relative to orthotactically illegal sequences (e.g., consonant strings) and/or orthotactically legal pseudowords (Araújo et al., 2015; Coch and Meade, 2016). As such, lexical tuning is sensitive to the statistical regularities of letter strings a child has encountered. Lexical tuning has been reported in grades 1 and 5 (Zhao et al., 2014; Eberhard-Moscicka et al., 2015), and even preschoolers who were trained on the pronunciations and meanings of a small list of sight words (pseudowords; Zhao et al., 2018). Lexical tuning appears to develop with reading skills, with older children and better readers showing larger, and more left-lateralized, effects than younger and/or less skilled readers (Zhao et al., 2014; Eberhard-Moscicka et al., 2015). Given the sensitivity of lexical tuning to both orthographic regularities and reading speed, its presence may reflect a transition from phonemic decoding to fluent sight word processing.
1.2.2 The N400
A second ERP component relevant to reading development is the N400, which is broadly associated with semantic processing. The N400 has been hypothesized to reflect the activation of long-term memory by incoming stimuli—including accessing the meanings of words, integrating them into current semantic contexts, and also distinguishing real words from orthotactically plausible pseudowords (Kutas and Federmeier, 2011). Typically, greater demands in accessing the meaning of a word (including identifying it as a non-word), are associated with larger N400 amplitudes. Among other factors, the size of the N400 seems to be proportionate to the ease with which a word can be identified and/or classified as a non-word. For example, words that occur with high frequency in a language elicit smaller N400s than low-frequency words (Petten and Kutas, 1990; Rugg, 1990; Barber et al., 2004; Hauk et al., 2006; Payne et al., 2015; Vergara-Martínez et al., 2017), and pseudowords that differ only in one or a few letters elicit larger N400s than real words (Chwilla et al., 1995; Holcomb et al., 2002; Braun et al., 2006), whereas consonant strings elicit smaller N400s than real words (Rugg and Nagy, 1987; Laszlo et al., 2012).
While we are not aware of novel word learning studies in children using ERPs, in adults newly-learned words have been shown to elicit N400 effects similar to real words, suggesting that new form-meaning pairings are rapidly established in memory (McLaughlin et al., 2004; Usai et al., 2017). Further evidence emphasizes that the N400 is specific to form-meaning pairings; when novel words are taught without associated meanings, they do not modulate N400 amplitudes (Balass et al., 2010; Frishkoff et al., 2010).
1.3 The present study
Together, both prominent theories of reading development and empirical studies highlight the importance of orthographic and semantic learning in the transition to skilled reading—i.e., fluent word reading and comprehension (Cunningham, 2006; Ricketts et al., 2011). The novel word learning paradigm employed by Mimeau et al. (2018) and others is a well-established behavioral approach to studying orthographic learning in the context of independent reading, while the N170 and N400 ERPs provide insight into the sequence of processes involved in recognizing words, accessing their meanings, and integrating them for comprehension. It thus seems natural to apply ERPs in the context of novel word learning during independent reading. This can help us understand the extent to which the novel words are processed similar to already-known words, as well as to better connect our understanding of the behavioral and neurophysiological markers of reading.
In the present study we investigated whether a small number of exposures to novel words during independent reading is enough to establish neural responses typical of known words. The N170 component has been shown to index visual identification and the mapping of words to orthographic knowledge, as well as tracking the development of visual expertise for printed words (Maurer et al., 2005, 2008; Brem et al., 2013; Zhao et al., 2014; Eberhard-Moscicka et al., 2015). If novel words elicit N170 print and (especially) lexical tuning effects, this would provide support that the paradigm establishes orthographic representations of the novel words.
Likewise, the presence of an N400 effect for novel words, relative to unfamiliar pseudowords, could be taken as evidence of semantic learning, since previous research has associated the N400 with lexical access and semantic integration (Petten and Kutas, 1990; Rugg, 1990; Barber et al., 2004; Hauk et al., 2006; Payne et al., 2015). This pattern of N170-N400 results would both strengthen our understanding and the validity of the novel word learning paradigm as a model of orthographic and semantic learning, and provide novel evidence that the N170 and N400 effects can be established in children learning new vocabulary through independent reading.
The primary goal of the present study was to determine how orthographic and semantic learning abilities relate to established neurophysiological markers of visual word expertise (N170 print and lexical tuning effects). We recruited children in grade 3, as this is a transitional period characterized by high variability in reading ability among children, when self-teaching, sight word reading, and lexical tuning are all developing. It is also a pivotal stage of learning to read; children who do not read at grade level at the end of grade 3 are at higher risk for a later school dropout (Hernandez, 2011). We adapted the novel word learning paradigm of Mimeau et al. (2018). The learning task was broken into several blocks, and between each block EEG data was collected while children performed a lexical decision task (LDT) which involved the newly-taught words (henceforth novel words), as well as real English words, orthotactically legal non-words (pseudowords; comparable to the novel words but not presented in the learning task), consonant strings, and false fonts.
1.4 Hypotheses
Hypothesis 1: We predicted that we would replicate behavioral findings of prior novel word learning studies, with children showing above-chance performance on the orthographic and semantic choice tasks used in the ortho-semantic learning task, as well as on accuracy for novel words in the LDT.
Hypothesis 2: We predicted that we would replicate past findings of the print and lexical tuning N170 effects (false fonts vs consonant strings, and consonant strings vs. real words, respectively), and the N400 effect (pseudowords vs. real words). We further predicted that greater reading proficiency would be associated with more left-lateralized and larger-amplitude print and lexical tuning N170 effects.
Hypothesis 3: On the basis of the self-teaching and lexical quality hypotheses we predicted that independent reading would be effective in establishing orthographic representations of the novel words, which would be reflected by an enhanced N170 relative to consonant strings. We did not predict an N170 difference between consonant strings and pseudowords—which had similar orthographic structures to the novel words, but for which no prior exposure or association with meaning had occurred. We further predicted that better novel word learning performance would be associated with larger N170 print (consonant string vs. false font) and lexical (real words vs. consonant strings) tuning effects, on the premise that the magnitude of these N170 effects reflect skilled word recognition.
Hypothesis 4: We predicted that if the independent reading task was sufficient to establish semantic representations for the novel words, then they should pattern similarly to real words in eliciting smaller N400s than pseudowords. We further predicted that the N400 effect for novel words relative to pseudowords would be largest in children who performed best on the semantic components of the ortho-semantic learning task, and would be correlated with semantic abilities as measured by reading comprehension and vocabulary.
2 Materials and methods
2.1 Participants
Thirty-eight native English-speaking children were recruited from grade 3 programs in local schools. Data from four children (three female) were excluded due to excessively noisy EEG, defined as >25% of trials marked as unusable by the automated artifact correction/exclusion procedure described later in the section ERP Preprocessing. The final sample of 34 children consisted of 21 males and 13 females (chronological age range = 7.5–9.4, mean = 8.7, SD = 0.5; 30 right-handed). Although English was the native and dominant language for all children, five children had some exposure to other languages. All participants had normal hearing and normal or corrected-to-normal vision, with no reported developmental, neurological, or psychiatric disorders—including reading or other language disorders. Children and their parents/guardians provided informed assent and consent, respectively, before participating in the study. They were compensated monetarily, as well as with a certificate of completion. The research protocol was approved by the Dalhousie University Social Sciences and Humanities Research Ethics Board.
2.2 Behavioral measures and procedures
The following assessments were administered in the order described, for all participants: The Sight Word Efficiency and Phonemic Decoding sub-tests from the Test of Word Reading Efficiency (TOWRE-2; Torgesen et al., 1999); Word Identification and Passage Comprehension subtests from the Woodcock Reading Mastery Test-Revised (WRMT-R; Woodcock, 1998); Elision subtest of the Comprehensive Test of Phonological Processing (CTOPP-2; Wagner et al., 2013); orthographic and semantic knowledge tests (adapted from Olson et al., 1985); a shortened version of the Peabody Picture Vocabulary Test (M-PPVT-3; Dunn and Dunn, 2007; Wang et al., 2009; Pasquarella et al., 2011); the Matrix Reasoning subtest of the Wechsler Abbreviated Scale of Intelligence (WASI; Wechsler, 1999); the Digit Span subtest of the Wechsler Abbreviated Scale of Intelligence (WISC-4; Wechsler, 2011). Details of each assessment are included in the Supplementary material.
2.3 Ortho-semantic learning task
2.3.1 Stimuli
The ortho-semantic learning task was originally developed by Mimeau et al. (2018), adapted from Wang et al. (2011) and used previously by several authors (Cunningham, 2006; Bowey and Miller, 2007; Ricketts et al., 2011; Tucker et al., 2016). The task comprises 24 short stories, each consisting of five sentences with a consistent structure. In each story a novel word is introduced as an “invention” (e.g., a mechanism to remove juice from oranges), with the word repeated a total of four times. In each story, the first sentence introduced a problem; the second sentence introduced an initial action between the inventor and the invention; the third sentence described the invention's function; the fourth sentence described the use of the invention; and the fifth sentence described the resolution of the problem when the invention was used. An example paragraph is shown in the Supplementary Methods.
2.3.2 Procedure
Prior to reading the passages, children were instructed to read each passage aloud, and told that they would be asked later about the spellings and meanings of the inventions described in the stories. The exact instructions are provided in the Supplementary Methods. The 24 stories were divided into three blocks of eight each, with one block of stories presented prior to each ERP/LDT block. Each story was printed on a separate piece of paper, and children read all eight stories in the block aloud in sequence, and then were given an orthographic choice task for each of the eight novel words from that block, followed by a semantic choice task for each word. The experimenter provided help and corrective feedback if a child had trouble reading, or mispronounced, any word. Following each block of stories, prior to the ERP task, children were tested on their recognition of the spellings and meanings of the novel words, each using a four-alternative forced-choice task. In the spelling task, the correct spelling was presented along with a homophone of the same word, and two other words that shared the same vowel patterns as the target and its homophone. In the semantic task, a picture of the correct invention was shown along with an alternative involving similar objects, as well as two more distinct objects. Examples of these tasks, and further details, are provided in the Supplementary Methods.
2.4 Lexical decision task
2.4.1 Stimuli
The LDT consisted of five experimental conditions: real words, pseudowords, novel words (words presented as target items in the ortho-semantic learning task), consonant strings, and false fonts. The experiment contained a total of 198 stimuli. This included 100 real words, 50 novel non-words, 48 novel words, 50 consonant strings, and 50 false fonts. The number of real words was double that of other categories so that there were approximately equal numbers of items in the LDT requiring “YES” (real word) and “NO” responses. These stimuli were pseudo-randomly distributed into the three experimental blocks, such that equal numbers of items from each condition appeared in each block, and the novel words learned prior to that block were shown in the corresponding LDT block. Further details of each stimulus type are provided in the Supplementary Methods.
2.4.2 Procedure
All stimuli were presented on a computer screen (ViewSonic XG2401) 24” positioned 70 cm from the bridge of the child's nose, using software written with PsychoPy (Peirce et al., 2019). Children were given a USB numeric keypad (Nexxtech; Barrie, Canada) with two keys marked as “YES” and “NO” Children were presented with the following instructions in written form (over a series of four slides), and asked to read each slide aloud before progressing to the next one. Instructions are reprinted in the Supplementary Methods.
Once the child pressed the space bar, a short practice block began. This comprised 10 items, including three false font strings, four real words, two pseudowords, and one consonant string. The unbalanced distribution of stimuli across conditions was based on pilot testing to keep the practice as short as possible, while ensuring children gained some familiarity with each stimulus type. An experimenter observed the child's responses and provided verbal feedback as to whether each was correct or not, and coaching on how to perform the task as needed. After the practice, the first block of experimental trials began.
Each trial consisted of a 2.5 s gray screen, followed for 0.5 s by a fixation cross in the center of the screen. After this, the target stimulus was presented for 1 s. The order of presentation of items was randomized within each block. Following the stimulus item, the screen went blank gray, and the program waited until a response key was pressed before advancing to the next trial. Because the response window was unlimited, the duration of each block varied; however each block comprised 66 trials with a total duration of ~5 min, plus response time.
2.5 EEG recording
After completing the behavioral assessments, participants were fitted with a 128-channel Hydrocel Geodesic Sensor EEG net (HCGSN; Electrical Geodesics Inc., Eugene, Oregon) and seated in an electrically shielded, sound attenuating booth (Eckel Noise Control Technologies, Morrisburg, ON). EEG was recorded continuously with a sampling rate of 500 Hz, with a low pass filter of 100 Hz and high pass filter of 0.01 Hz, referenced to the vertex electrode Cz. Prior to recording, all electrode impedances lowered below 100 kΩ; impedances were checked again, and lowered as necessary, prior to each subsequent block of EEG recording.
During EEG recording, participants completed three blocks of a LDT (LDT; Ratcliff et al., 2004), each preceded by a block of the ortho-semantic learning task in which children were taught the meanings of novel words through exposure to written text. The EEG net was kept on the child's head for both the ortho-semantic learning task and LDT tasks, however EEG data were recorded only during the LDT.
2.6 ERP preprocessing
After recording, the EEG data were exported in binary format and preprocessed using the MNE-python software package (v1.4.2; Gramfort, 2013; Gramfort et al., 2014). Details of each preprocessing step are provided in the Supplementary Methods. In brief, preprocessing steps included: 0.1–30 Hz bandpass filtering; semi-automated artifact correction using independent components analysis (ICA; Hyvarinen, 1999; Delorme et al., 2007); automated residual artifact correction and/or removal, and bad channel interpolation (v0.4.0; Jas et al., 2017); and re-referencing to the average of all channels (for N170 analyses) or to the mastoid channels (for N400 analyses). Finally, the timing of the event onsets was corrected to account for the measured delay between when the stimulus computer sent the event markers to the EEG system, and when the words appeared on the screen.
2.7 ERP component measurement
2.7.1 Regions of Interest
Each ERP component of interest was analyzed within regions of interest (ROIs) that were defined a priori, based on previous research. For the N170 analyses, we used left and right temporal-parietal-occipital ROIs adapted from past studies of the N170 in children of similar ages (Brem et al., 2009, 2013; Maurer et al., 2011; Bach et al., 2013). These earlier papers typically used one or more of the following pairs: T5/6, O1/2 (or O1′/O2′, shifted slightly from the International 10–10 System locations of O1/2), PO9/10, and P7/8; since the EGI HCGSN montage does not include all positions specified in the 10–10 system, we included those that do correspond (58/96, corresponding to T5/6; and 70/83, corresponding to O1/2), as well as the electrodes in between/adjacent to those electrodes. Specifically, the left ROI included electrodes 58 (T5), 59, 64, 65, 66, 69, and 70 (O1), and the right ROI included the corresponding electrodes 96 (T6), 91, 95, 90, 84, 89, and 83 (O2). For the N400, we chose the set of electrodes centered around the vertex (Cz, as recommended by Šoškić et al., 2022), including 6, 112, 105, 87, 79, 54, 37, 30, 13, 106, 80, 55, 31, and 7.
2.7.2 Time windows
For the N170, each trial was baseline-corrected by subtracting the mean amplitude in the 100 ms preceding stimulus onset from each time point, for each channel. Then, we averaged across all trials for each channel within each of the two ROIs and then used MNE's get_peak function to find the most negative value in a 100 ms window centered around the peak of the N170 in the group average (190 ms), which we took as that individual's N170 peak. Finally, we computed the mean amplitude over a 50 ms time window centered on this peak, at each channel and for each trial within that child/condition/ROI.
For the N400, a priori we planned to compute mean amplitude over the 300–500 ms window, as is common in N400 studies (Šoškić et al., 2022). However, visual inspection of the group averages suggested that there were distinct patterns of effects across conditions between 300–400 and 400–500 ms. Therefore, as described in the Results we computed and analyzed mean amplitudes over these two time windows separately, as well as over the originally-planned 300–500 ms window. Furthermore, as discussed below rather than using a conventional pre-stimulus baseline we used baseline regression (Alday, 2019) to control for the differences in amplitude associated with the N170 component preceding the N400 analysis time window.
2.7.3 Outlier removal
Particularly since statistical analyses were to be performed on individual trials, we identified and removed outliers from the mean amplitude measurement data. The ERP component measurements for each participant were separately standardized using a z transform, and values ±2.5 standard deviations were removed. For the N400, the same procedure was also applied to the baseline measurements. This resulted in removal of 2.16% of the data for the N170, 3.71% for the early N400 window, and 3.82% for the late N400 window.
2.8 Statistical analysis
2.8.1 Behavioral data
Both accuracy and reaction time (RT) for the LDT were analyzed. For accuracy, we submitted single-trial data (correct/incorrect) to linear mixed effects modeling with a binomial family, using the glmer function in the lme4 (Bates et al., 2015) package for R (v.4.1.2; R Core Team, 2023). Condition was treated as a fixed effect, and a family of random effects were tested for inclusion, including random intercepts for participant, random intercepts for item, and random slopes for condition by participant. The best model was selected on the basis of Akaike's information criterion (AIC), and more specifically AIC weights (Wagenmakers and Farrell, 2004). For RT, we also used glmer and the same family of candidate models, but with an inverse Gaussian family as recommended by Lo and Andrews (2015) to account for the non-normal distribution of RTs.
2.8.2 ERP data
Linear mixed-effects (LME) modeling as implemented by the function bam, from the mgcv package (v. 1.8-42 Wood, 2011) in R was used to investigate the influence of predictor variables (Baayen et al., 2008; Newman et al., 2012; Tremblay and Newman, 2015). As fixed effects we considered Condition (false fonts, consonant strings, pseudowords, novel words, and real words) and, for the N170, ROI (left and right; for N400 only one ROI was analyzed). For random effects we considered random intercepts for participants, random slopes for condition by participant, and random slopes for channel by participant. AIC weights were used to select the best model. The best model was then explored using the emmeans package (v. 1.8.5 Lenth, 2023) and a set of planned, pairwise contrasts. For the N170, these were false fonts—consonant strings (print tuning); consonant strings—real words (lexical tuning); consonant strings—novel words “lexical tuning” for newly-learned words; consonant strings—pseudowords; real words—novel words; and novel words—pseudowords. For the N400, contrasts were print tuning; lexical tuning; pseudowords—real words; novel—real words; and pseudowords—novel words. The resulting p values were corrected for the number of contrasts using Tukey's method.
To test relationships between ERP amplitude and behavioral measures, we computed additional LME models, each extending the best model for that component with an additional fixed effect representing scores on one of the behavioral tests specified in the hypothesis. The slopes of N170 amplitude by the behavioral measure were tested with respect to zero (no relationship) for each of the planned contrasts described above. To control for Type I error, we did not consider all of the behavioral test scores that were obtained. Rather, we selected those that we believed were most relevant to the question of ortho-semantic learning: orthographic choice; semantic choice; and accuracy for novel words in the LDT. As well, we considered a set of measures previously shown to be related to performance on the ortho-semantic learning task (Mimeau et al., 2018): orthographic knowledge; semantic knowledge; reading fluency; and reading comprehension.
3 Results
3.1 Demographics and standardized tests
A summary of key demographic and behavioral tests are shown in Table 1. Scores on a small number of tests were not available for all participants, due to decisions by children or guardians to discontinue participation prior to all data being collected. However, only four participants had missing data, and no more than one test score was missing from any individual (one each on Digit Span, CTOPP Elision, PPVT, and Woodcock Word ID). A summary of standard test scores for all participants included in the analyses are provided in the Supplementary Results. This sample of children tended to score at or above their age-equivalent peers in the normative samples, with a few exceptions. On the other hand, the percentile scores were relatively uniformly distributed across the full range.
3.2 Ortho-semantic tasks
These tasks comprise the four measures developed by Mimeau et al. (2018), including orthographic choice, semantic choice, orthographic knowledge, and semantic knowledge with the “choice” tasks reflecting learning scores for the novel words in the ortho-semantic learning task. For three participants, data from the orthographic or semantic knowledge tests was lost due to technical errors, and the semantic knowledge task data was lost for one additional child. Descriptive statistics for each subtest are provided in tabular form in the Supplementary Results, and plotted—along with the scores for each individual child—in Figure 1. Given that each item on each test involved a choice among four possible responses, chance accuracy would be 25%. All participants thus responded at rates better than chance. There was, however, considerable variability between individuals in scores on these tests. This was desirable from the perspective of analyses presented below which investigate this variability in relation to ERP measures.
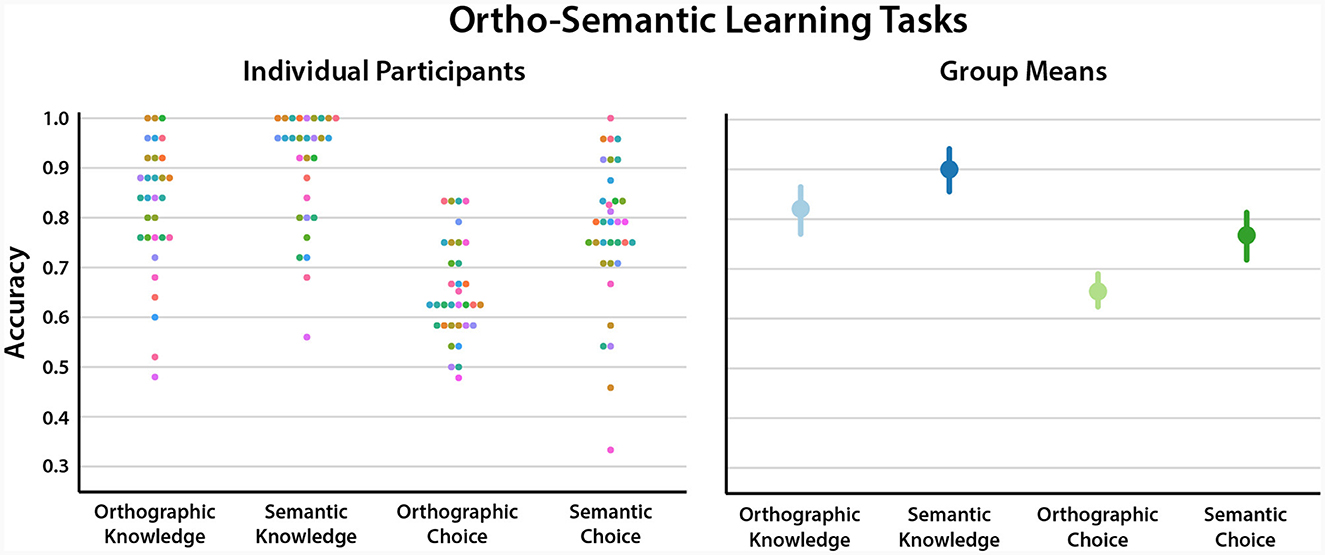
Figure 1. Swarm plots of orthographic and semantic knowledge and learning scores across participants. Each point represents average accuracy for one participant; with color coding participant ID. The horizontal axis represents chance performance (25%); no participants scored below this level.
3.3 Lexical decision task
Data from the LDT (during which the ERP data were collected) were trimmed prior to analysis to remove outliers. Children did not have a time limit to make a response to this task, and in some cases children chose this time period in which to take a break. Therefore, reaction times (RTs) on some trials extended to hundreds of seconds. We first removed any RTs shorter than 150 ms, or longer than 8 s (visual examination individual participant box plots showed that the interquartile range never exceeded 7.5 s). This step removed 101 trials, or 1.2% of the original data. We then converted RTs to z scores separately for each participant, and removed any trials with RTs ±2 standard deviations from the individual's mean RT. This removed an additional 319 trials, or 4.0% of the original data. Thus in total 5.2% of trials were removed as outliers. The trimmed data were used to analyze both accuracy and RT.
3.3.1 Accuracy
Accuracy rates across all participants are shown in the top panels of Figure 2, and in tabular form in the Supplementary Results. Generally speaking, children showed very high accuracy and little inter-individual variation for the false fonts (M = 97.2%), consonant strings (M = 93.9%), and real words (M = 93.3%). In contrast, performance was on average lower and more variable across children for pseudowords (M = 65.8%) and novel words (M = 63.8%).
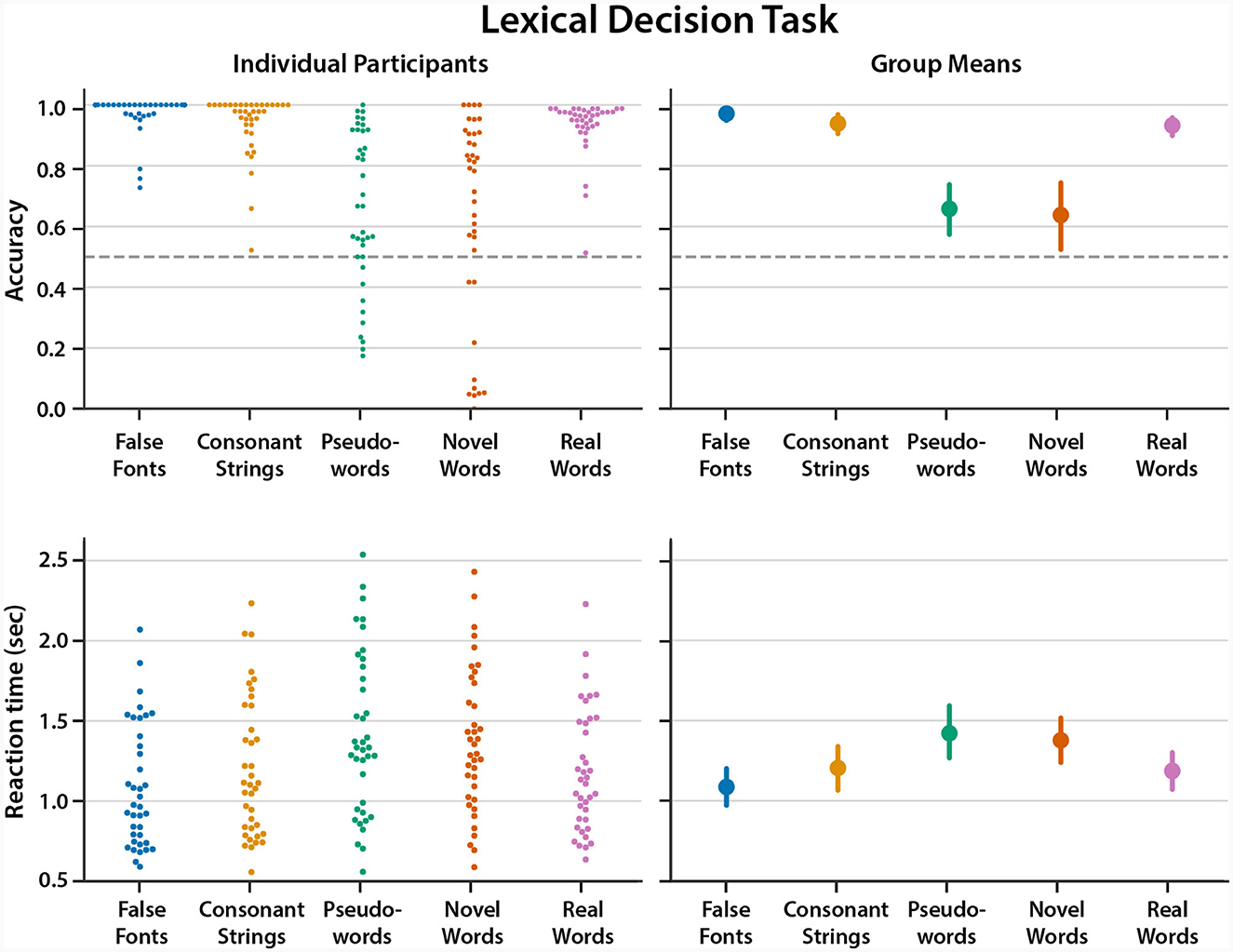
Figure 2. (Top panels) Mean accuracy (proportion correct) for each condition in the LDT. Left panel shows mean accuracy for each individual participant; right panel shows means across participants, with error bars representing 95% confidence intervals (CIs). (Bottom panels) Left panel shows mean RT for each individual participant; right panel shows mean RT across participants, with error bars representing 95% confidence intervals (CIs).
The best LME model included a fixed effect of condition, and random intercepts for participants and words, as well as random condition-by-participant slopes. Pairwise contrasts showed significantly greater accuracy for real words, consonant strings, and false fonts than for either novel words or pseudowords. Accuracy was also higher for false fonts than real words, but there was no difference between false fonts and consonant strings, nor between consonant strings and real words; accuracy was not significantly different between pseudowords and novel words. Statistical results are detailed in the Supplementary Results.
3.3.1.1 Sensitivity and response bias
During visual inspection of individual participants' data, we noted variability between children for both pseudowords and novel words, and in some cases what appeared to be a negative correlation between the two—suggesting that some children may have been biased to either treat both pseudowords and novel words consistently as either “words,” or “nonwords,” rather than discriminating between them. We performed an exploratory signal detection analysis (Donaldson, 1992) to quantify each child's sensitivity (A′) and response bias (B′′) using the psycho package in R (Makowski, 2018). Correctly responding to real or novel words with “yes” were counted as hits, whereas correctly responding to false fonts, consonant strings, and pseudowords as “no” were considered correct rejections. Given the overall high accuracy for false fonts, consonant strings, and real words, these metrics should be largely sensitive to responses to pseudowords and novel words. Plots of these metrics are shown in Supplementary Results. Most children showed good discriminability, and they were relatively evenly distributed between showing conservative and liberal biases in responding, with the majority of children (20/34) clustered around the zero line (B′′ values ± 0.25)—representing unbiased performance. We thus saw no evidence that there was a systematic bias in children's tendency to treat non-words as real words, or vice-versa; while some individual children demonstrated biases one way or the other, they did so in relatively equal proportions.
3.3.2 Reaction time
For analyzing RTs, we included trials on which incorrect responses were made (these comprised 1,026 trials or 13.5% of the data set after trimming). This was done (and likewise, ERPs were analyzed across correct and incorrect trials) because we were interested in analyzing the duration of the process by which children made a lexical decision—and also to keep the number of trials per condition more consistent across children, given the variance in accuracy rates and response biases reported in the previous section.
Mean RTs for each condition are shown in Figure 2, with details in the Supplementary Results. Children were on average fastest to respond to false fonts, and slowest to pseudowords. A linear mixed effects analysis was performed on RTs, with the best model including a fixed effect of condition, random intercepts for participants, and random condition-by-participant slopes. Responses to false fonts were significantly faster than for any other condition, and responses to consonant strings and real words were also significantly faster than to novel or pseudowords. RTs were not significantly different between consonant strings and real words, nor between pseudowords and novel words.
We re-ran the same analysis using only correct trials, since this approach is often used in RT analyses. These results, reported in Supplementary Table S6, were effectively identical to those with all trials, in terms of which contrasts were significant. The one difference is that whereas the RTs were significantly faster for real than novel words when all trials were considered, this contrast was not significant when only correct trials were considered.
3.4 Event-related potentials
All conditions elicited a largely similar pattern of ERPs, including bilateral positive peaks over parietal-occipital electrodes at 106 ms (corresponding to the visual P1 component), followed by bilateral negative peaks over slightly more lateral electrode sites (including locations T5/T6 and O1/O2 in the International 10–10 system) peaking 190 ms (corresponding to the N170). Following the N170, there were two bilateral, positive parietal-occipital peaks, at 306 and 428 ms respectively, which appeared largest for false fonts. Waveforms and topographic maps across all channels are shown in the Supplementary Results.
3.4.1 N170
The scalp distributions of the N170 component were quite consistent across conditions, with bilateral foci over the a priori ROIs consistent with previous studies. Waveforms and topographic maps are shown in Figure 3. Examination of the ERP waveforms over these ROIs shows apparent differences across conditions in the amplitude of the N170, but with highly consistent peak latency. In particular, N170 amplitude appeared to show a graded response with respect to “word-likeness,” being largest (most negative) for real and novel words, smaller for pseudowords (especially over the right ROI), smaller for consonant strings (especially over the left ROI), and smallest for false fonts. The N170 for consonant strings in particular appeared to be right-lateralized, resulting in a greater difference relative to real words (i.e., larger lexical tuning effect) over the left than right ROI. The best-fitting LME model included a fixed effect of condition; inclusion of the ROI factor was not warranted by the AIC weights. In other words, laterality did not explain sufficient variance to be warranted in the model. The model also included random intercepts for participants, and random slopes for both channels by participants and conditions by participants. Model-estimated means for each condition are plotted in Figure 4.
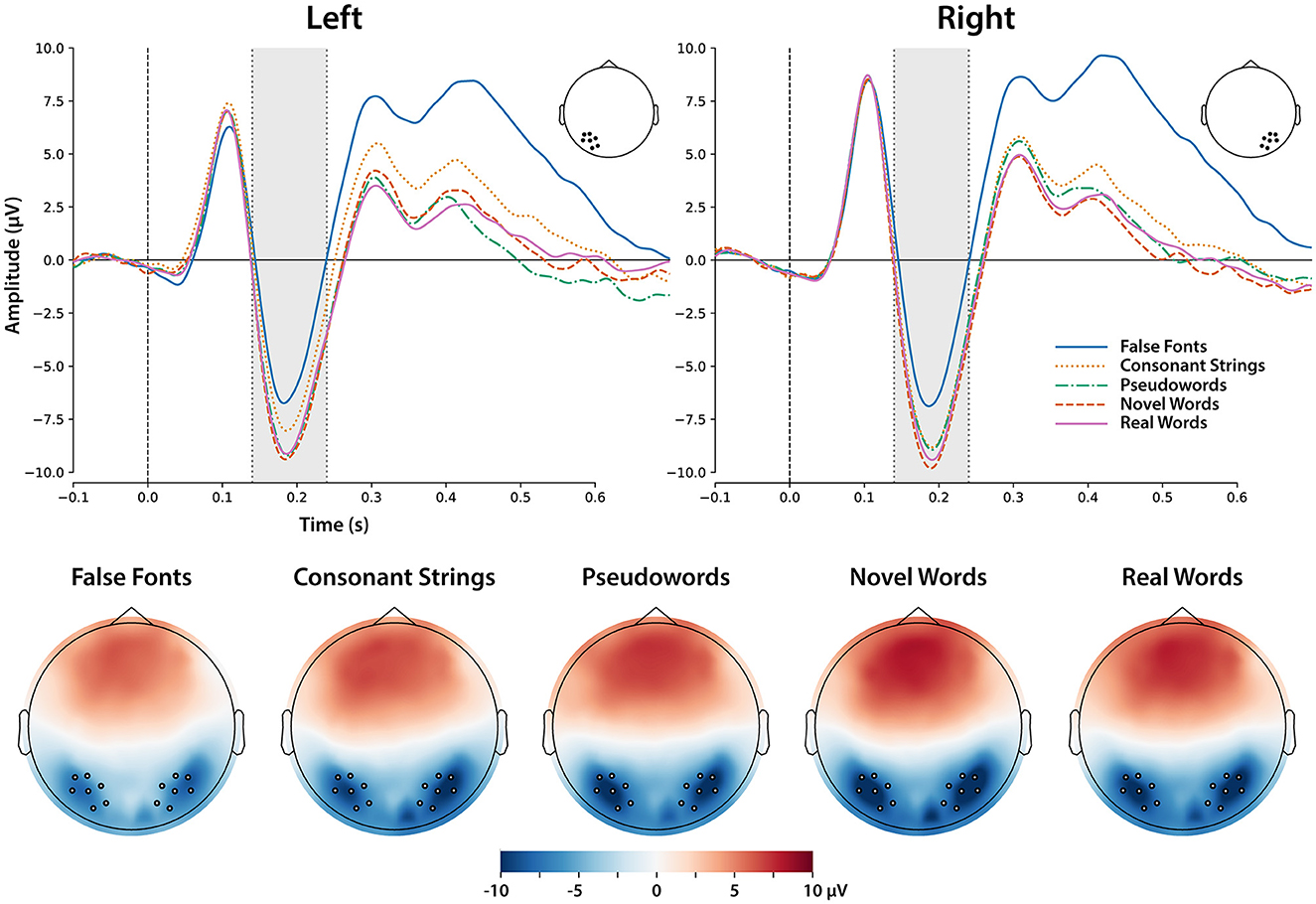
Figure 3. (Top) ERP waveforms for the left and right parietal-occipital ROIs analyzed for the N170 effect. Data are referenced to the average of all electrodes. Gray dotted lines show the time window used for statistical analysis. The head images show the clusters of electrodes in each ROI that were averaged to generate the waveforms. (Bottom) Scalp topographic maps showing the distribution of the N170 component in each condition. The maps reflect an average over 50 ms, centered at 190 ms post-word onset, corresponding to the peak of the N170. White dots indicate positions of channels included in the regions of interest used in waveform plots and statistical analyses.
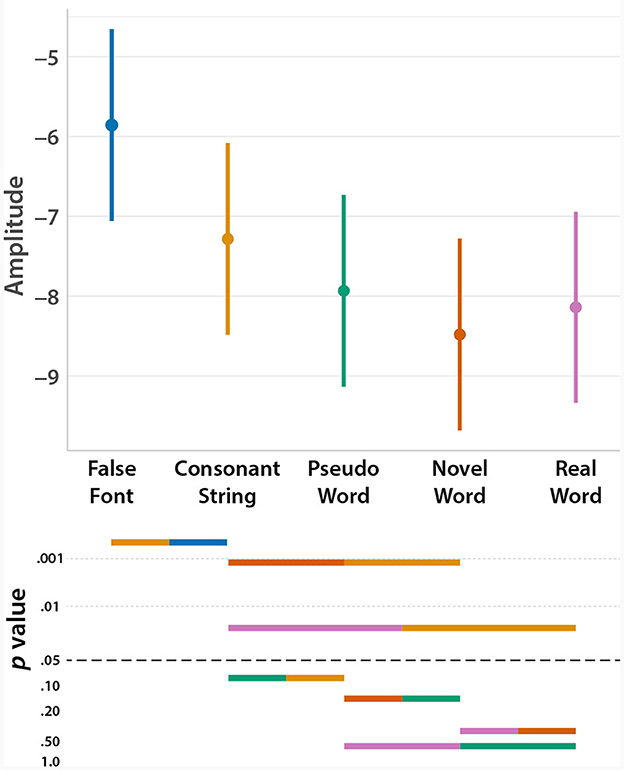
Figure 4. Top: Model-derived plot showing the estimated marginal mean amplitude of the N170 component for each condition, based on the linear mixed effects analysis. Error bars represent 95% CIs. Bottom: statistical significance of a priori pairwise contrasts between conditions.
The results of the planned statistical contrasts are shown in Table 2. In support of Hypothesis 2, we found significant print and lexical tuning effects. With respect to the novel words learned in the ortho-semantic learning task, Hypothesis 3 predicted a significant lexical tuning effect for novel words (i.e., a larger N170 than for consonant strings), but not for pseudowords. This hypothesis was supported: there was a significant lexical tuning effect for novel words but not pseudowords. However, there were no significant differences in the direct comparisons between real, novel, and pseudowords.
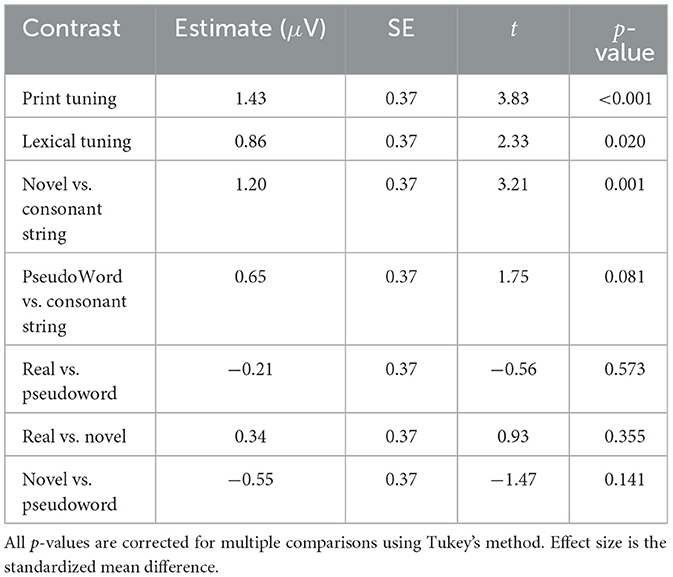
Table 2. Between-condition contrasts for the N170 component, from the linear mixed effects analysis.
3.4.1.1 Relationship to behavioral data
We further extended the linear mixed effects modeling to consider whether the print and lexical tuning N170 effects were modulated by ortho-semantic learning ability or reading ability. The only behavioral variable that showed a significant interaction with condition was accuracy on novel words in the LDT—a direct measure of children's recognition of the newly-learned words in the task performed during EEG data collection. The magnitude of the print tuning effect was significantly related to novel word accuracy, t = 2.15, p = 0.032, as shown in Figure 5. However, the lexical tuning contrast was not significantly related to any measure of ortho-semantic learning. To further understand the nature of this interaction, we examined the slopes of the relationship between novel word accuracy and N170 amplitude separately for each condition. The slopes were significant for consonant strings, t = −2.04, p = 0.041 (and also real words, t = 2.02, p = 0.043), but not for false fonts. The significant effect of print tuning was thus driven by increasing N170 amplitude for consonant strings in children with higher novel word accuracy, not by the response to false fonts; this can be seen in Figure 5.
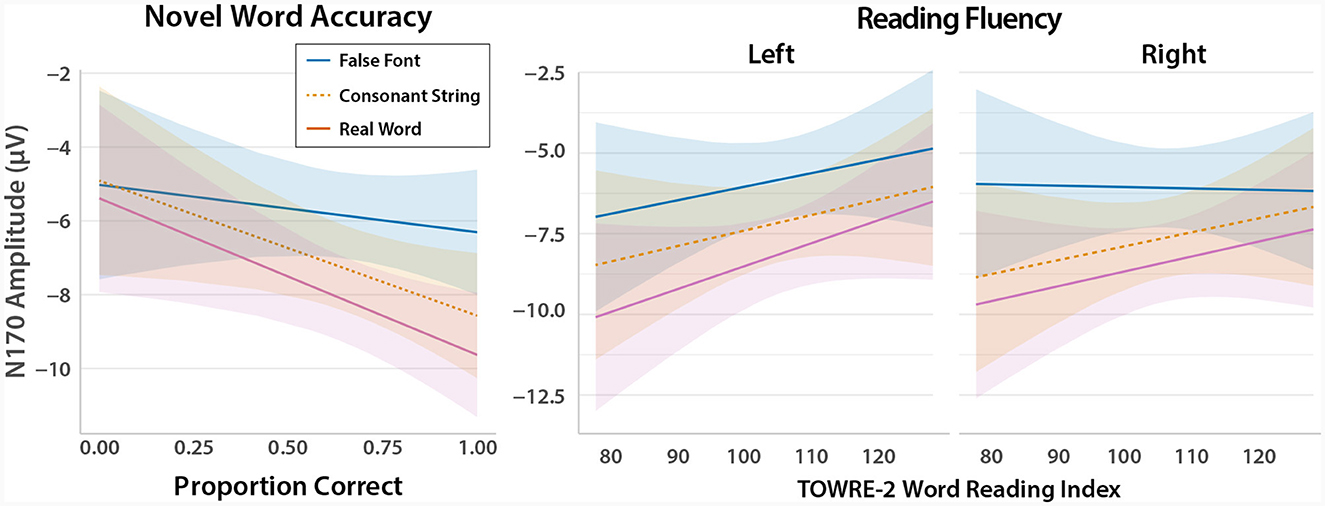
Figure 5. Relationships between N170 print tuning effects and behavioral measures. (Left panel) Model-derived plot showing the relationship between accuracy on novel words in the LDT, and N170 print tuning (false fonts—consonant strings) and lexical tuning (consonant strings—real words) effects. The relationship was significant for print, but not lexical, tuning. (Right panel) Model-derived plot showing the relationship between reading fluency, ROI, and N170 print and lexical tuning effects. The slope of the print tuning effect was significant over the right ROI only. Shaded areas represent 95% CIs.
Previous findings suggested that the lateralization of print and lexical tuning effects was modulated by grade level and/or reading proficiency. We thus explored whether ROI might be warranted in models that also contained a covariate representing reading ability, even though it was not warranted when only condition was considered. To test this, we compared (using AIC) the best model from above (i.e., a fixed effect of condition and the full random effects structure described above) with models that included condition interacting with TOWRE-2 index or WRMT-2 Passage Comprehension, and also models that included condition, one of those two reading proficiency measures, and ROI. The model including condition, ROI, and TOWRE-2 index was ~ 6.7× more likely than the next-best model. This model included a significant interaction between print tuning, ROI, and reading fluency, t = 2.27, p = 0.023. This interaction, plotted in Figure 5, suggests that as reading fluency increases, the size of the print tuning effect over the right ROI decreases.
3.4.2 N400
The grand averaged waveforms over the N400 vertex ROI, for each condition are shown in Figure 6. This figure includes two panels: on the left are the waveforms relative to a conventional baseline of the 100 ms preceding stimulus onset. Notably in this panel, there are clear differences in amplitude between conditions immediately prior to the N400 time window, and corresponding to the N170 time window (note that the peak in this time window is positive because it is over the vertex ROI, in contrast to the lateral posterior ROIs used for N170 analysis). To control for these preceding differences, and isolate differences in amplitude subsequent to the N170 window, we employed baseline regression (Alday, 2019) to control for the mean amplitude over a 50 ms window centered on the peak of the group-averaged N170 (165–215 ms) for each trial and channel. The right panel of Figure 6 shows the waveforms after applying baseline regression. Hereafter we focus on the N170 baseline-regressed data for description and statistical analyses.
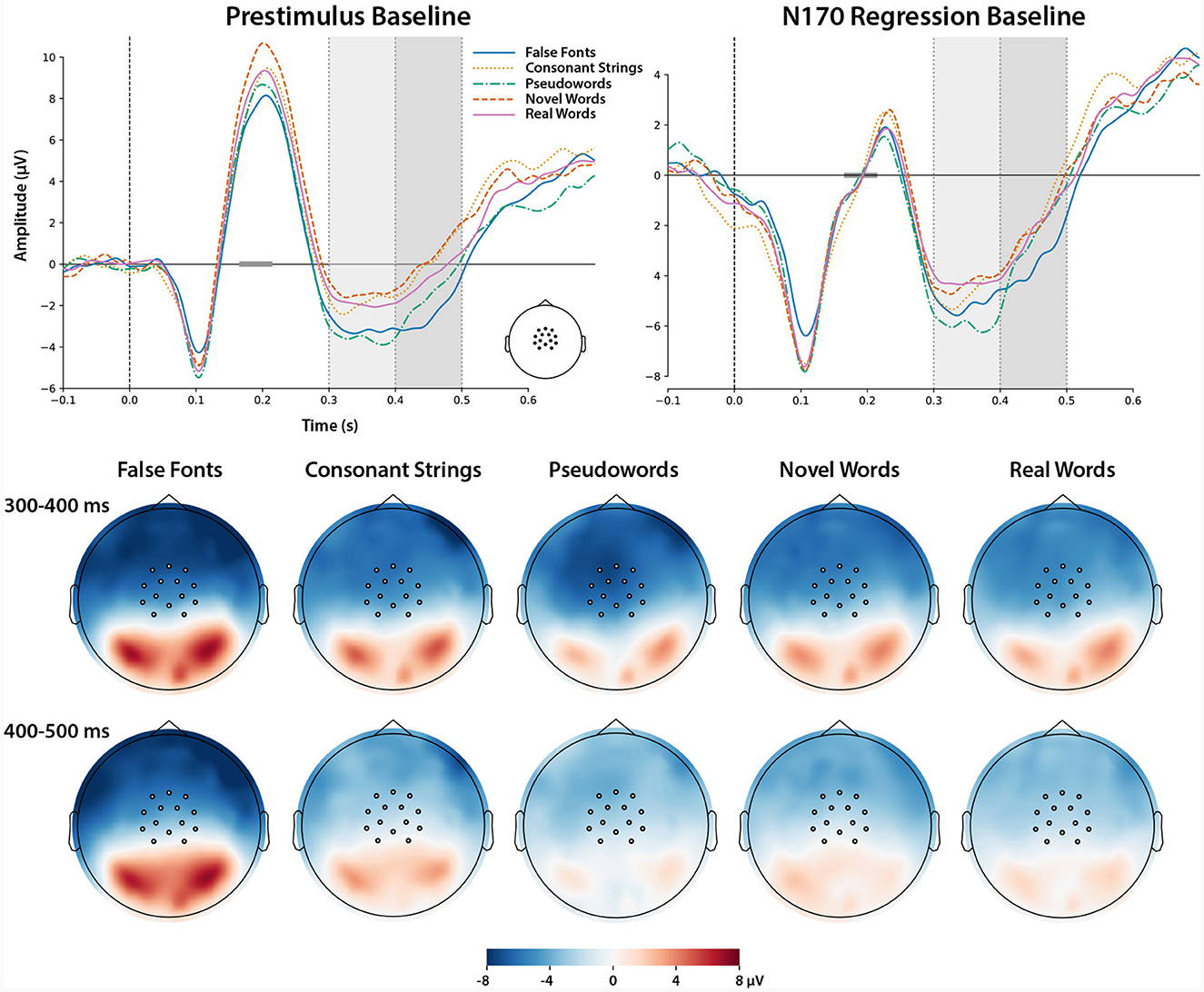
Figure 6. (Left panel) ERP waveform averaged over vertex ROI electrodes to show the N400 component. Data are referenced to the average of the mastoid electrodes. Gray dotted lines and shading show the two time windows used for statistical analysis. (Right panel) the same data, but with the mean amplitude in the N170 time window (165–215 ms) regressed from the waveform for each condition. This procedure serves to isolate any differences in amplitude that occurred in the N400 time window from those potentially attributable to between-condition differences in the preceding N170 component. (Bottom panel) Scalp topographic maps showing the baseline-regressed data, averaged over the two time windows analyzed. Circles indicate channels included in vertex ROI.
A second observation from the waveform plots was that, with both the conventional prestimulus baseline and the N170 regression baseline, different patterns of differences between conditions were apparent from 300 to 400 and 400 to 500 ms. Thus we chose to analyze the early (300–400 ms) and late (400–500 ms) segments of this time window separately, since analyzing the 300–500 ms window would conflate two apparently different patterns of effects. We did also perform the LME analysis on the a priori planned 300–500 ms time window; these results are included in the Supplementary material.
3.4.2.1 Early N400 time window (300–400 ms)
Focusing on the baseline-regressed data, in the early time window the N400 appeared largest (most negative) for pseudowords, followed by false fonts, and smallest for real and novel words. Consonant strings elicited a negativity comparable to false fonts early in the time window (~300–350 ms), but more similar to real and novel words in the later part of the window. The best linear mixed effects model included fixed effects of condition and baseline (but no interaction between them; Alday, 2019), random intercepts for each participant, and random channel-by-participant and condition-by-participant slopes. The model-estimated means for each condition are shown in Figure 7, and the results of pairwise between-condition contrasts are shown in Table 3. As predicted, the N400 for pseudowords was significantly larger (more negative) than for real words. As well, the pseudoword N400 was significantly larger than for novel words. No other contrasts were significant.
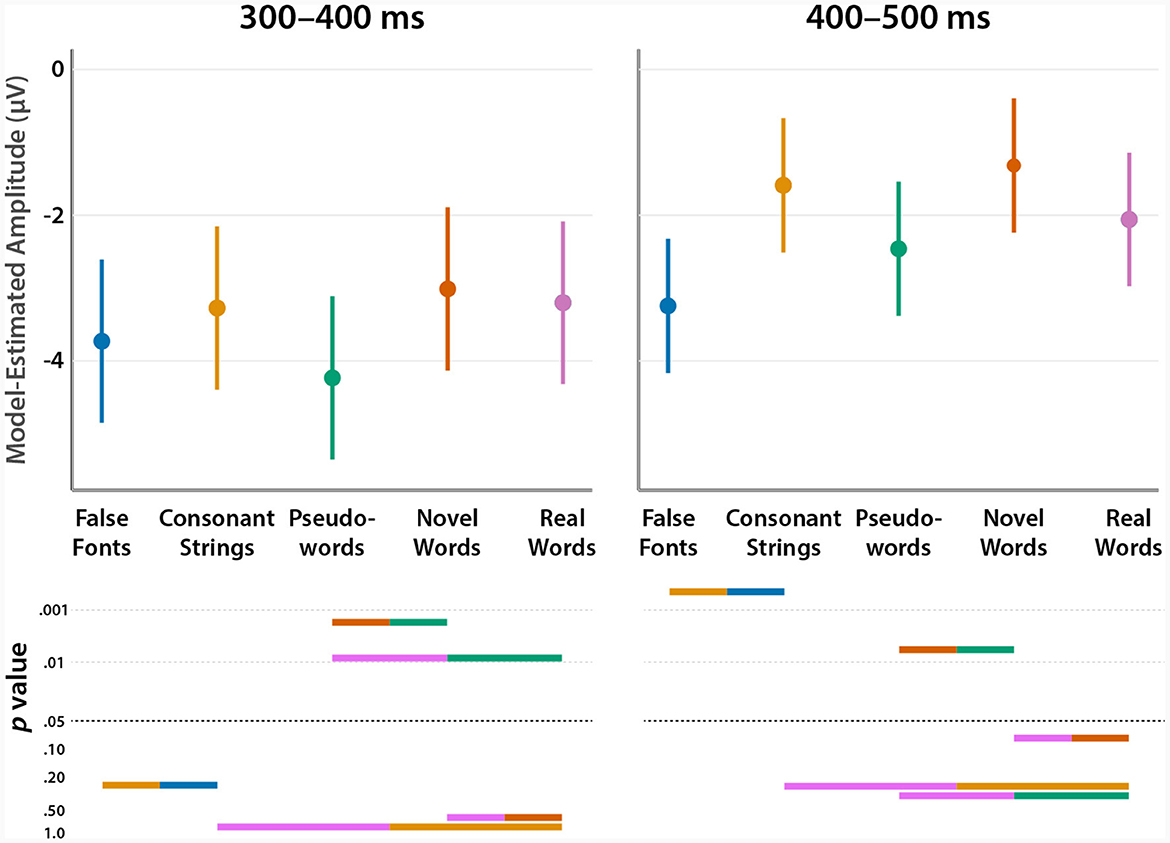
Figure 7. Top: Model-derived plots from the linear mixed effects analysis of the N400; left panel shows the 300–400 ms time window, right panel shows 400–500 ms. Points represent estimated means and error bars show 95% confidence intervals for each condition. Bottom: statistical significance of a priori pairwise contrasts between conditions.
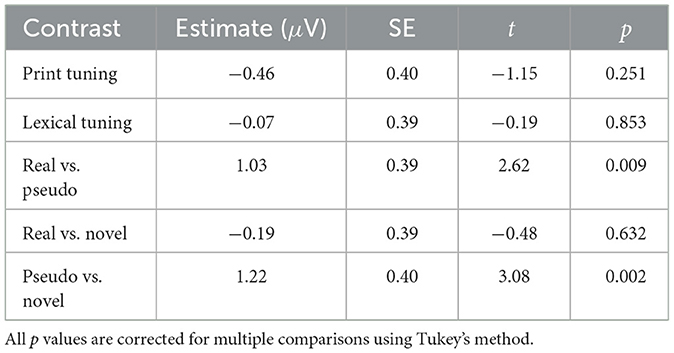
Table 3. Between-conditions contrasts for each condition from the linear mixed effects analysis of the N400 component from 300 to 400 ms.
The planned regressions of N400 amplitude for pseudowords vs. novel words against semantic components of the ortho-semantic learning task, reading comprehension (passage comprehension), and vocabulary (PPVT, Word ID) yielded one significant result, for passage comprehension (t = 2.28, p = 0.0225). Specifically, as shown in Figure 8, good comprehenders showed a larger N400 for pseudowords relative to novel words, but poor comprehenders did not; poor comprehenders showed similar N400 amplitudes for pseudowords and novel words. Examination of Figure 8 also shows that the N400 amplitude was flat with respect to reading comprehension scores; the significant difference between these conditions was driven by low comprehenders having equivalent N400 amplitudes for pseudowords and novel words, while high comprehenders had a reduced N400 amplitude (more similar to real words). Notably, this effect occurred even though the planned regressions of N400 amplitude with semantic learning scores (semantic choice on the ortho-semantic learning task, and novel word accuracy on the LDT) were not significant.
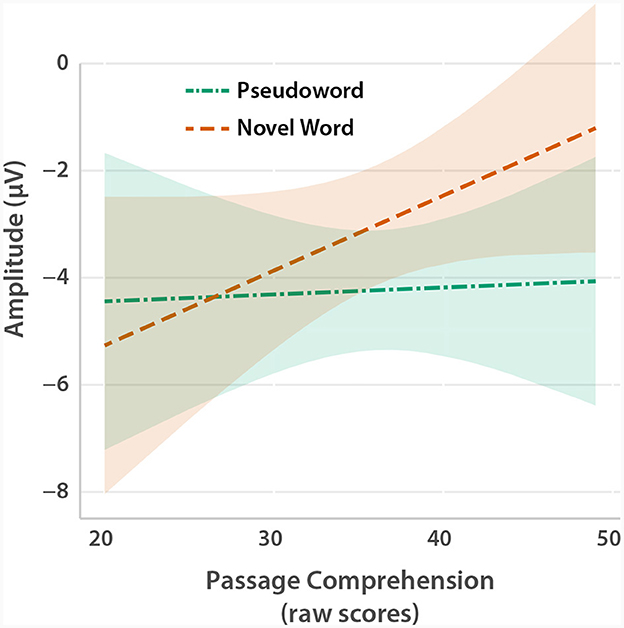
Figure 8. Model-derived plot showing the relationship between passage comprehension (from the WRMT-R), and N400 amplitude (300-400 ms) for the contrast between novel words and pseudowords. Shaded areas represent 95% CIs.
3.4.2.2 Late N400 time window (400–500 ms)
Detailed results of the LME analysis of this time window are presented in the Supplementary Results. In short, false fonts elicited a significantly larger N400 than consonant strings. Additionally, as in the preceding time window, the N400 was significantly larger for pseudowords than novel words. No other contrasts were significant, nor were any of the modals including behavioral predictors.
4 Discussion
4.1 Behavioral findings
Consistent with Hypothesis 1, children showed consistent evidence of learning the spellings and meanings of the novel words during independent reading. All children performed at rates better than chance on the orthographic and semantic choice tasks used in prior studies. This finding replicates prior studies and confirms the validity of our self-teaching task. In the LDT, children were also above chance levels in correctly classifying novel words that they had just been exposed to as “words,” and in classifying pseudowords as nonwords. Interestingly, RTs to real and novel words were not significantly different when only correct trials were considered, but were when all trials were considered. Since very few errors were made for real words, the difference between the two analyses must be driven by slower RTs for novel words on incorrect trials. In other words, when novel words were correctly recognized, this happened at a speed similar to real words, but the decision to (incorrectly) reject a novel word required more time.
Together these results suggest that indeed children recognized these letter strings on the basis of brief exposure through their independent reading, and support both the self-teaching and lexical quality hypotheses (Share, 1995; Perfetti and Hart, 2002; Mimeau et al., 2018). Critically, as advocated in other recent work (Deacon et al., under review), these findings provide additional evidence that the relevance of orthographic and semantic dimensions—emphasized within the lexical quality hypotheses Perfetti and Hart (2002)—need to be integrated with the self-teaching hypothesis Share (1995). Children are learning both the spellings and meanings of new words through their reading, and theories need to capture both dimensions. Further, these empirical findings give us confidence that the self-teaching task implemented here is capturing classic effects (see also Shakory et al., 2021), enabling us to examine relationships between novel word learning and the N170 and N400.
4.2 Event-related potentials
4.2.1 N170
4.2.1.1 Print and lexical tuning
Hypothesis 2 was also confirmed, in that we observed both print and lexical tuning effects, i.e., a larger N170 for consonant strings than false fonts, and real words than consonant strings, respectively. The print tuning effect has been consistently demonstrated to be established by grade 2, and is largely associated with children's familiarity with mappings between letters and sounds (Maurer et al., 2005, 2006; Brem et al., 2013; Zhao et al., 2014; Eberhard-Moscicka et al., 2015; Varga et al., 2020); as our children were all within the normal range of grade 3 reading ability these mappings can be expected to be well established.
Left-lateralized print tuning effects had been reported in some prior studies, but we found no evidence for significant lateralization at the group level. However, some studies have reported that left-lateralization increases with age and/or reading ability (Maurer et al., 2011; Brem et al., 2013; Zhao et al., 2014). Indeed, in the present data the print tuning effect over the right ROI decreased with higher reading fluency (TOWRE-2) scores. In other words, the print tuning effect was, in relative terms, larger over the left hemisphere in children with higher reading fluency. The fact that this effect was driven by a reduction in the print tuning effect over the right ROI is also consistent with previous studies (Maurer et al., 2011; Brem et al., 2013; Zhao et al., 2014).
We also found a significant lexical tuning effect—i.e., a larger N170 for real words relative to consonant strings. The presence of lexical tuning indicates that the children in our sample have established an ability to rapidly distinguish plausible strings of letters (orthotactically legal combinations of consonants and vowels) from those that never form words in English (consonant strings). While some previous studies have found a relationship between the size of the lexical tuning effect and reading proficiency, in the present sample we found no significant relationships with the standardized measures of proficiency we administered. However, this could be a ceiling effect whereby all participants had high enough reading levels to show the lexical tuning effect.
4.2.1.2 Novel words
Having established that we replicated the standard print and lexical tuning effects, we now turn to the focus of this study: orthographic and semantic learning of novel words. Our results supported Hypothesis 3 in showing a significant “lexical tuning” effect for novel words (relative to consonant strings), but not for pseudowords. This finding shows that not only did children rapidly learn to recognize the spellings and meanings of the novel words learned in the context of a paragraph, but this learning was associated with the emergence of lexical tuning for these novel words. Critically, this effect was not observed for the pseudowords, which were comparable in orthographic structure to the novel words but were not learned through independent reading. This provides novel support for the self-teaching hypothesis, indicating that brief exposure to new words in an independent reading context is sufficient to establish neural responses associated with word recognition that are similar to that for previously-known words.
In further support of Hypothesis 3, we found that greater accuracy in identifying novel words in the LDT was associated with a larger print tuning effect. This indicates that children whose brains are more tuned to print (i.e., show a greater differential N170 response to letters relative to letter-like symbols) are able to more reliably identify recently-learned novel words. Previous behavioral work using structural equation modeling showed that orthographic learning predicted reading fluency (Mimeau et al., 2018); our results suggest that children's ability to rapidly identify and filter letters from competing stimuli is important for their ability to quickly learn novel words while reading.
It is interesting that this finding did not extend to the lexical tuning effect, as we found no associations between lexical tuning and ortho-semantic learning scores—even though novel words elicited a lexical tuning effect. Given that the magnitude of the lexical tuning effect is smaller than print tuning, this may simply be an issue of sensitivity. On the other hand, it may suggest that the neural tuning to letters generally (rather than the ability to distinguish plausible from implausible letter strings) is most relevant to the recognition of recently-learned words. The relationship between novel word recognition and print tuning aligns well with the self-teaching hypothesis, which suggests that phonological decoding is central to word learning during independent reading because it promotes letter-by-letter processing, drawing attention to the specific sequence of letters which enables cementing that pattern into long-term memory (Share, 1995, 2008).
4.2.2 N400
We also explored the N400 component, which reflects processes involved in accessing the meanings of words and integrating them into an ongoing semantic context in memory. Thus while the N170 reflects processes more closely related to orthographic processing, the N400 is sensitive to the semantic properties of words. Hypothesis 2 predicted a significantly larger N400 for pseudowords than for real words, replicating past findings. Hypothesis 4 similarly predicted a larger N400 for pseudowords than for novel words, based on the prediction that novel words would be recognizable word forms, and associated with meanings, while pseudowords would not. Hypothesis 4 further predicted that the magnitude of this N400 effect for novel words would be larger in children who showed better performance on behavioral measures of semantic knowledge and learning ability.
These predictions were generally borne out. Most importantly, the N400 to pseudowords was significantly larger than for both real and novel words. The presence of the same effect for novel as for real words indicates that the ortho-semantic learning task was effective in establishing memory traces for the words. We speculate that the N400 reduction for novel words relative to pseudowords is attributable to learning meanings for the words, rather than simply the familiarity of the wordform. This is because the N400 has been associated specifically with the integration of incoming stimuli with semantic information in long-term memory (Kutas and Federmeier, 2011). Indeed, past studies of novel word learning in adults linked N400s specifically to novel words with associated semantic representations but not to repeated exposure without associated meaning (Balass et al., 2010; Frishkoff et al., 2010).
It is also notable that the one significant relationship between a behavioral variable and the N400 obtained in this study was between passage comprehension and the N400 difference between novel and pseudowords. That is, children who showed better passage comprehension showed a greater neural distinction between the newly-learned novel words and orthographically similar pseudowords. This finding suggests that stronger passage comprehension skills (including knowledge of word meanings and the ability to integrate them within and across sentences) allow children to better learn novel words from a passage context, and then rapidly (within 300–400 ms) recognize those words as distinct from orthotactically plausible words with which meanings have not been associated.
This result is consistent with several prior studies associating larger N400 amplitudes to better comprehension abilities in adults (Landi and Perfetti, 2007; Perfetti et al., 2008) and children (Henderson et al., 2011). As well, previous behavioral studies have found positive associations between learning the meanings of novel words through independent reading, and comprehension abilities (Ricketts et al., 2011; Mimeau et al., 2018). Notably across our finding and others, the N400 is associated with a relatively complex skill: passage comprehension. In contrast, neither we nor Henderson et al. (2011) found a relationship between the N400 amplitude and measures of vocabulary knowledge, which tap lexical semantics but not the more complex task of integrating the meanings of individual words with prior context and long-term memory. This suggests that learning novel word meanings from the context of a story relies more heavily on the ability to mentally form and understand that context—which is necessary for inferring the meaning of the novel words—than simply on knowledge of individual word meanings. Again, these ideas push the integration of a learning component into the lexical quality hypothesis (Perfetti and Hart, 2002), suggesting that we need to consider how these high-quality lexical representations are acquired (Deacon et al., under review), as well as their functional impacts on reading comprehension. Certainly, these ideas are supported by the finding here that when children later encountered the novel words in a LDT, they showed more efficient semantic integration of these words, reflected in a smaller N400.
It is worth comment that while we predicted an N400 effect from 300 to 500 ms (in line with previous N400 studies) in the present data set we observed different patterns across conditions in the 300–400 and 400–500 ms time windows, and so analyzed them separately. This is admittedly a post hoc decision that could be criticized on the basis of circularity and exploitation of “researcher degrees of freedom.” On the other hand, we did not change the time window used for analysis from what was planned, but merely analyzed it in a more fine-grained way. We did also perform the planned analysis across the 300–500 ms window (reported in the Supplementary material), and the results were not very different. Most importantly, the greater N400 for pseudowords than real words was also obtained in the 300–500 ms time window. Given the lack of previous studies of the N400 in children using a single word reading LDT (let alone an ortho-semantic learning task), we felt it was reasonable to titrate our analysis time windows based on the data itself. We encourage other researchers doing similar work to consider analyzing the N400 component in the future using similar time windows a priori, to if this finding is replicable.
5 Conclusion
We found that children learned new words' meanings and spellings from a short independent reading task, and these words triggered brain responses related to word recognition and meaning integration that were similar to real words, and different from unlearned pseudowords. N170 print tuning was significantly associated with accurate recognition of the novel words, suggesting that low-level sensitivity to print is important orthographic learning—even more than the ability to distinguish real words from consonant strings (lexical tuning). Differences in N400 amplitude between newly-learned words and (control) pseudowords were significantly related to levels of reading comprehension, but not vocabulary knowledge. This suggests that passage comprehension is related to the ability to infer the meanings of new words from context, and establish those meanings in memory so they can later be efficiently recalled. Future research should investigate these relationships across a larger age and ability range to replicate and extend the findings, including in a longitudinal design (e.g., Deacon et al., under review). Our results demonstrate the value of the N170 and N400 as biomarkers of reading abilities in developing readers; these markers in fact reflect the acquisition of key aspects of high-quality representations, providing empirical validation of integration of self-teaching with lexical quality hypotheses (e.g., Share, 1995; Perfetti and Hart, 2002). These findings also move us closer to an integration of the rich reading development literatures using behavioral and neurophysiological measures.
Data availability statement
The analysis code used to generate the results presented in this study can be found at: https://doi.org/10.17605/OSF.IO/54D8F.
Ethics statement
The studies involving humans were approved by Dalhousie University Social Sciences and Humanities Research Ethics Board. The studies were conducted in accordance with the local legislation and institutional requirements. Written informed consent for participation in this study was provided by the participants' legal guardians/next.
Author contributions
AG: Conceptualization, Data curation, Formal analysis, Investigation, Methodology, Project administration, Supervision, Validation, Writing – original draft, Writing – review & editing. LB: Formal analysis, Investigation, Writing – original draft. CL: Investigation, Writing – review & editing. JV: Investigation, Writing – review & editing. CM: Methodology, Writing – review & editing. TD: Writing – review & editing. LE: Validation, Writing – review & editing. SD: Conceptualization, Funding acquisition, Methodology, Writing – review & editing. AN: Conceptualization, Data curation, Formal analysis, Funding acquisition, Methodology, Project administration, Resources, Software, Supervision, Validation, Visualization, Writing – original draft, Writing – review & editing.
Funding
The author(s) declare financial support was received for the research, authorship, and/or publication of this article. This research was supported by an Insight Development Grant (430-2016-01097) and an Explore Grant from the Social Sciences and Humanities Research Council of Canada (SSHRC) to AN. LB was supported by a Nova Scotia Research and Innovation Graduate Scholarship.
Acknowledgments
We are grateful to Cindy Hamon-Hill and Morgan Johnson for assistance with the project, and to all of the children and families who participated.
Conflict of interest
The authors declare that the research was conducted in the absence of any commercial or financial relationships that could be construed as a potential conflict of interest.
The author(s) declared that they were an editorial board member of Frontiers, at the time of submission. This had no impact on the peer review process and the final decision.
Publisher's note
All claims expressed in this article are solely those of the authors and do not necessarily represent those of their affiliated organizations, or those of the publisher, the editors and the reviewers. Any product that may be evaluated in this article, or claim that may be made by its manufacturer, is not guaranteed or endorsed by the publisher.
Supplementary material
The Supplementary Material for this article can be found online at: https://www.frontiersin.org/articles/10.3389/fdpys.2024.1340383/full#supplementary-material
References
Alday, P. M. (2019). How much baseline correction do we need in ERP research? Extended GLM model can replace baseline correction while lifting its limits. Psychophysiology 56:e13451. doi: 10.1111/psyp.13451
Andrews, S., Veldre, A., and Clarke, I. E. (2020). Measuring lexical quality: the role of spelling ability. Behav. Res. Methods 52, 2257–2282. doi: 10.3758/s13428-020-01387-3
Araújo, S., Faísca, L., Bramão, I., Reis, A., and Petersson, K.-M. (2015). Lexical and sublexical orthographic processing: an ERP study with skilled and dyslexic adult readers. Brain Lang. 141(C), 16–27. doi: 10.1016/j.bandl.2014.11.007
Baayen, R. H., Davidson, D. J., and Bates, D. M. (2008). Mixed-effects modeling with crossed random effects for subjects and items. J. Mem. Lang. 59, 390–412. doi: 10.1016/j.jml.2007.12.005
Bach, S., Richardson, U., Brandeis, D., Martin, E., and Brem, S. (2013). Print-specific multimodal brain activation in kindergarten improves prediction of reading skills in second grade. Neuroimage 82, 605–615. doi: 10.1016/j.neuroimage.2013.05.062
Balass, M., Nelson, J. R., and Perfetti, C. A. (2010). Word learning: an ERP investigation of word experience effects on recognition and word processing. Contemp. Educ. Psychol. 35, 126–140. doi: 10.1016/j.cedpsych.2010.04.001
Barber, H., Vergara, M., and Carreiras, M. (2004). Syllable-frequency effects in visual word recognition: evidence from ERPs. Neuroreport 15, 545–548. doi: 10.1097/00001756-200403010-00032
Bates, D., Mächler, M., Bolker, B., and Walker, S. (2015). Fitting linear mixed-effects models using lme4. J. Stat. Softw. 67, 1–48. doi: 10.18637/jss.v067.i01
Bentin, S., Mouchetant-Rostaing, Y., Giard, M. H., Echallier, J. F., and Pernier, J. (1999). ERP manifestations of processing printed words at different psycholinguistic levels: time course and scalp distribution. J. Cogn. Neurosci. 11, 235–260. doi: 10.1162/089892999563373
Bowey, J. A., and Miller, R. (2007). Correlates of orthographic learning in third-grade children's silent reading. J. Res. Read. 30, 115–128. doi: 10.1111/j.1467-9817.2007.00335.x
Braun, M., Jacobs, A. M., Hahne, A., Ricker, B., Hofmann, M., Hutzler, F., et al. (2006). Model-generated lexical activity predicts graded ERP amplitudes in lexical decision. Brain Res. 1073, 431–439. doi: 10.1016/j.brainres.2005.12.078
Brem, S., Bach, S., Kucian, K., Kujala, J. V., Guttorm, T. K., Martin, E., et al. (2010). Brain sensitivity to print emerges when children learn letter speech sound correspondences. Proc. Nat. Acad. Sci. 107, 7939–7944. doi: 10.1073/pnas.0904402107
Brem, S., Bach, S., Kujala, J. V., Maurer, U., Lyytinen, H., Richardson, U., et al. (2013). An electrophysiological study of print processing in kindergarten: the contribution of the visual N1 as a predictor of reading outcome. Dev. Neuropsychol. 38, 567–594. doi: 10.1080/87565641.2013.828729
Brem, S., Halder, P., Bucher, K., Summers, P., Martin, E., Brandeis, D., et al. (2009). Tuning of the visual word processing system: distinct developmental ERP and fMRI effects. Hum. Brain Mapp. 30, 1833–1844. doi: 10.1002/hbm.20751
Cain, K., Oakhill, J., and Lemmon, K. (2004). Individual differences in the inference of word meanings from context: the influence of reading comprehension, vocabulary knowledge, and memory capacity. J. Educ. Psychol. 96, 671–681. doi: 10.1037/0022-0663.96.4.671
Chwilla, D. J., Brown, C. M., and Hagoort, P. (1995). The N400 as a function of the level of processing. Psychophysiology 32, 274–85. doi: 10.1111/j.1469-8986.1995.tb02956.x
Coch, D., and Meade, G. (2016). N1 and P2 to words and wordlike stimuli in late elementary school children and adults. Psychophysiology 53, 115–128. doi: 10.1111/psyp.12567
Cunningham, A. E. (2006). Accounting for children's orthographic learning while reading text: do children self-teach? J. Exp. Child Psychol. 95, 56–77. doi: 10.1016/j.jecp.2006.03.008
Deacon, S. H., Mimeau, C., Chung, S. C., and Chen, X. (2019). Young readers' skill in learning spellings and meanings of words during independent reading. J. Exp. Child Psychol. 181, 56–74. doi: 10.1016/j.jecp.2018.12.007
Deacon, S. H., Mimeau, C., Levesque, K., and Ricketts, J. (under review). Testing Mechanisms Underlying Children's Reading Development: The Power of Learning Lexical Representations.
Delorme, A., Sejnowski, T., and Makeig, S. (2007). Enhanced detection of artifacts in EEG data using higher-order statistics and independent component analysis. Neuroimage 34, 1443–1449. doi: 10.1016/j.neuroimage.2006.11.004
Donaldson, W. (1992). Measuring recognition memory. J. Exp. Psychol.: Gen. 121, 275–277. doi: 10.1037/0096-3445.121.3.275
Dunn, D. M., and Dunn, L. M. (2007). Peabody Picture Vocabulary Test, Fourth Edition, Manual. Chicago, IL: NCS Pearson, Inc. doi: 10.1037/t15144-000
Dyer, A., MacSweeney, M., Szczerbinski, M., Green, L., and Campbell, R. (2003). Predictors of reading delay in deaf adolescents: the relative contributions of rapid automatized naming speed and phonological awareness and decoding. J. Deaf. Stud. Deaf. Educ. 8, 215–229. doi: 10.1093/deafed/eng012
Eberhard-Moscicka, A. K., Jost, L. B., Raith, M., and Maurer, U. (2015). Neurocognitive mechanisms of learning to read: print tuning in beginning readers related to word-reading fluency and semantics but not phonology. Dev. Sci. 18, 106–118. doi: 10.1111/desc.12189
Fitzgerald, J., and Shanahan, T. (2000). Reading and writing relations and their development. Educ. Psychol. 35, 39–50. doi: 10.1207/S15326985EP3501_5
Fraga-González, G., Pleisch, G., Pietro, S. V. D., Neuenschwander, J., Walitza, S., Brandeis, D., et al. (2021). The rise and fall of rapid occipito-temporal sensitivity to letters: transient specialization through elementary school. Dev. Cogn. Neurosci. 49:100958. doi: 10.1016/j.dcn.2021.100958
Frishkoff, G. A., Perfetti, C. A., and Collins-Thompson, K. (2010). Lexical quality in the brain: ERP evidence for robust word learning from context. Dev. Neuropsychol. 35, 376–403. doi: 10.1080/87565641.2010.480915
Gramfort, A. (2013). MEG and EEG data analysis with MNE-Python. Front. Neurosci. 7:267. doi: 10.3389/fnins.2013.00267
Gramfort, A., Luessi, M., Larson, E., Engemann, D. A., Strohmeier, D., Brodbeck, C., et al. (2014). MNE software for processing MEG and EEG data. Neuroimage 86, 446–460. doi: 10.1016/j.neuroimage.2013.10.027
Graves, M. F. (2006). The Vocabulary Book: Leanring and Instruction. New York, NY: Teachers College Press.
Hauk, O., Davis, M., Ford, M., Pulvermller, F., and Marslen-Wilson, W. (2006). The time course of visual word recognition as revealed by linear regression analysis of ERP data. Neuroimage 30, 1383–1400. doi: 10.1016/j.neuroimage.2005.11.048
Henderson, L. M., Baseler, H. A., Clarke, P. J., Watson, S., and Snowling, M. J. (2011). The N400 effect in children: relationships with comprehension, vocabulary and decoding. Brain Lang. 117, 88–99. doi: 10.1016/j.bandl.2010.12.003
Hernandez, D. J. (2011). Double Jeopardy: How Third-Grade Reading Skills and Poverty Influence High School Graduation. Technical report. Baltimore, MD: The Annie E. Casey Foundation.
Holcomb, P. J., Grainger, J., and O'Rourke, T. (2002). An electrophysiological study of the effects of orthographic neighborhood size on printed word perception. J. Cogn. Neurosci. 14, 938–950. doi: 10.1162/089892902760191153
Hyvarinen, A. (1999). Fast and robust fixed-point algorithms for independent component analysis. IEEE Trans. Neural Netw. 10, 626–634. doi: 10.1109/72.761722
Jas, M., Engemann, D. A., Bekhti, Y., Raimondo, F., and Gramfort, A. (2017). Autoreject: automated artifact rejection for MEG and EEG data. Neuroimage 159, 417–429. doi: 10.1016/j.neuroimage.2017.06.030
Kutas, M., and Federmeier, K. D. (2011). Thirty years and counting: finding meaning in the N400 component of the event-related brain potential (ERP). Annu. Rev. Psychol. 62, 621–647. doi: 10.1146/annurev.psych.093008.131123
Landi, N., and Perfetti, C. A. (2007). An electrophysiological investigation of semantic and phonological processing in skilled and less-skilled comprehenders. Brain Lang. 102, 30–45. doi: 10.1016/j.bandl.2006.11.001
Laszlo, S., Stites, M., and Federmeier, K. D. (2012). Won't get fooled again: an event-related potential study of task and repetition effects on the semantic processing of items without semantics. Lang. Cogn. Process. 27, 257–274. doi: 10.1080/01690965.2011.606667
Lenth, R. V. (2023). emmeans: Estimated Marginal Means, aka Least-Squares Means. Available online at: https://CRAN.R-project.org/package=emmeans
Lo, S., and Andrews, S. (2015). To transform or not to transform: using generalized linear mixed models to analyse reaction time data. Front. Psychol. 6:1171. doi: 10.3389/fpsyg.2015.01171
Makowski, D. (2018). The psycho package: an efficient and publishing-oriented workflow for psychological science. J. Open Source Softw. 3:470. doi: 10.21105/joss.00470
Maurer, U., Brem, S., Bucher, K., and Brandeis, D. (2005). Emerging neurophysiological specialization for letter strings. J. Cogn. Neurosci. 17, 1532–1552. doi: 10.1162/089892905774597218
Maurer, U., Brem, S., Kranz, F., Bucher, K., Benz, R., Halder, P., et al. (2006). Coarse neural tuning for print peaks when children learn to read. Neuroimage 33, 749–758. doi: 10.1016/j.neuroimage.2006.06.025
Maurer, U., Schulz, E., Brem, S., Mark, S. V. D., Bucher, K., Martin, E., et al. (2011). The development of print tuning in children with dyslexia: evidence from longitudinal ERP data supported by fMRI. Neuroimage 57, 714–722. doi: 10.1016/j.neuroimage.2010.10.055
Maurer, U., Zevin, J. D., and McCandliss, B. D. (2008). Left-lateralized N170 effects of visual expertise in reading: evidence from japanese syllabic and logographic scripts. J. Cogn. Neurosci. 20, 1878–1891. doi: 10.1162/jocn.2008.20125
McLaughlin, J., Osterhout, L., and Kim, A. (2004). Neural correlates of second-language word learning: minimal instruction produces rapid change. Nat. Neurosci. 7, 703–704. doi: 10.1038/nn1264
Mimeau, C., Ricketts, J., and Deacon, S. H. (2018). The role of orthographic and semantic learning in word reading and reading comprehension. Sci. Stud. Read. 22, 1–17. doi: 10.1080/10888438.2018.1464575
Nation, K., and Castles, A. (2017). “Putting the learning into orthographic learning,” in Theories of Reading Development. Volume 15 Studies in Written Language and Literacy, eds K. Cain, D. L. Compton, and R. K. Parrila (Amsterdam: John Benjamins Publishing Company), 147–168. doi: 10.1075/swll.15.09nat
Newman, A. J., Tremblay, A., Nichols, E. S., Neville, H. J., and Ullman, M. T. (2012). The influence of language proficiency on lexical semantic processing in native and late learners of English. J. Cogn. Neurosci. 24, 1205–1223. doi: 10.1162/jocn_a_00143
O'Connor, M., Geva, E., and Koh, P. W. (2019). Examining reading comprehension profiles of grade 5 monolinguals and english language learners through the lexical quality hypothesis lens. J. Learn. Disabil. 52, 232–246. doi: 10.1177/0022219418815646
Olson, R. K., Kliegl, R., Davidson, B. J., and Foltz, G. (1985). “Individual and developmental differences in reading disability,” in Reading Research: Advances in Theory and Practice, Volume 4, eds G. E. MacKinnon, and T. G. Waller (Cambridge: Academic Press), 1–64.
Ouellette, G., and Fraser, J. R. (2009). What exactly is a yait anyway: the role of semantics in orthographic learning. J. Exp. Child Psychol. 104, 239–251. doi: 10.1016/j.jecp.2009.05.001
Pasquarella, A., Chen, X., Lam, K., Luo, Y. C., and Ramirez, G. (2011). Cross-language transfer of morphological awareness in Chinese English bilinguals. J. Res. Read. 34, 23–42. doi: 10.1111/j.1467-9817.2010.01484.x
Payne, B. R., Lee, C.-L., and Federmeier, K. D. (2015). Revisiting the incremental effects of context on word processing: evidence from single-word event-related brain potentials: effects of context on world-level N400. Psychophysiology 52, 1456–1469. doi: 10.1111/psyp.12515
Peirce, J., Gray, J. R., Simpson, S., MacAskill, M., Höchenberger, R., Sogo, H., et al. (2019). PsychoPy2: experiments in behavior made easy. Behav. Res. Methods 51, 195–203. doi: 10.3758/s13428-018-01193-y
Perfetti, C., Yang, C., and Schmalhofer, F. (2008). Comprehension skill and word-to-text integration processes. Appl. Cogn. Psychol. 22, 303–318. doi: 10.1002/acp.1419
Perfetti, C. A. (2017). “Lexical quality revisited,” in Developmental Perspectives in Written Language and Literacy, Volume 8, eds E. Segers, and P. van den Broek (Amsterdam: John Benjamins Publishing Company), 237. doi: 10.1075/z.206.04per
Perfetti, C. A., and Hart, L. (2002). “The lexical quality hypothesis,” in Precursors of Functional Literacy, Volume 11, eds L. Verhoeven, C. Elbro, and P. Reitsma (Amsterdam: John Benjamins Publishing Company), 189–213. doi: 10.1075/swll.11.14per
Petten, C. V., and Kutas, M. (1990). Interactions between sentence context and word frequency in event-related brainpotentials. Mem. Cogn. 18, 380–393. doi: 10.3758/BF03197127
Proverbio, A. M., Zani, A., and Adorni, R. (2008). The left fusiform area is affected by written frequency of words. Neuropsychologia 46, 2292–2299. doi: 10.1016/j.neuropsychologia.2008.03.024
R Core Team. (2023). R: A Language and Environment for Statistical Computing. Vienna: R Foundation for Statistical Computing.
Ratcliff, R., Thapar, A., Gomez, P., and McKoon, G. (2004). A diffusion model analysis of the effects of aging in the lexical-decision task. Psychol. Aging 19, 278–289. doi: 10.1037/0882-7974.19.2.278
Richter, T., Isberner, M.-B., Naumann, J., and Neeb, Y. (2013). Lexical quality and reading comprehension in primary school children. Sci. Stud. Read. 17, 415–434. doi: 10.1080/10888438.2013.764879
Ricketts, J., Bishop, D. V., and Nation, K. (2008). Investigating orthographic and semantic aspects of word learning in poor comprehenders. J. Res. Read. 31, 117–135. doi: 10.1111/j.1467-9817.2007.00365.x
Ricketts, J., Bishop, D. V. M., Pimperton, H., and Nation, K. (2011). The role of self-teaching in learning orthographic and semantic aspects of new words. Sci. Stud. Read. 15, 47–70. doi: 10.1080/10888438.2011.536129
Rossion, B., Joyce, C. A., Cottrell, G. W., and Tarr, M. J. (2003). Early lateralization and orientation tuning for face, word, and object processing in the visual cortex. Neuroimage 20, 1609–1624. doi: 10.1016/j.neuroimage.2003.07.010
Rugg, M. D. (1990). Event-related brain potentials dissociate repetition effects of high-and low-frequency words. Mem. Cogn. 18, 367–379. doi: 10.3758/BF03197126
Rugg, M. D., and Nagy, M. E. (1987). Lexical contribution to nonword-repetition effects: evidence from event-related potentials. Mem. Cogn. 15, 473–481. doi: 10.3758/BF03198381
Schendan, H. E., Ganis, G., and Kutas, M. (1998). Neurophysiological evidence for visual perceptual categorization of words and faces within 150 ms. Psychophysiology 35, 240–251. doi: 10.1111/1469-8986.3530240
Shakory, S., Chen, X., and Deacon, S. H. (2021). Learning orthographic and semantic representations simultaneously during shared reading. J. Speech Lang. Hear. Res. 64, 909–921. doi: 10.1044/2020_JSLHR-20-00520
Share, D. L. (1995). Phonological recoding and self-teaching: sine qua non of reading acquisition. Cognition 55, 151–218. doi: 10.1016/0010-0277(94)00645-2
Share, D. L. (1999). Phonological recoding and orthographic learning: a direct test of the self-teaching hypothesis. J. Exp. Child Psychol. 72, 95–129. doi: 10.1006/jecp.1998.2481
Share, D. L. (2008). Orthographic learning, phonological recoding, and self-teaching. Adv. Child Dev. Behav. 36, 31–82. doi: 10.1016/S0065-2407(08)00002-5
Share, D. L. (2011). “On the role of phonology in reading acquisition: the self-teaching hypothesis,” in Explaining Individual Differences in Reading: Theory and Evidence, eds S. A. Brady, D. Braze, and C. A. Fowler (London: Psychology Press), 45–68.
ŠoŠkić, A., Jovanović, V., Styles, S. J., Kappenman, E. S., and Ković, V. (2022). How to do better N400 studies: reproducibility, consistency and adherence to research standards in the existing literature. Neuropsychol. Rev. 32, 577–600. doi: 10.1007/s11065-021-09513-4
Swart, N. M., Muijselaar, M. M. L., Steenbeek-Planting, E. G., Droop, M., Jong, P. F., and Verhoeven, L. (2017). Differential lexical predictors of reading comprehension in fourth graders. Read. Writ. 30, 489–507. doi: 10.1007/s11145-016-9686-0
Tamura, N., Castles, A., and Nation, K. (2017). Orthographic learning, fast and slow: lexical competition effects reveal the time course of word learning in developing readers. Cognition 163, 93–102. doi: 10.1016/j.cognition.2017.03.002
Torgesen, J. K., Wagner, R. K., Rashotte, C. A., Rose, E., Lindamood, P., Conway, T., et al. (1999). Preventing reading failure in young children with phonological processing disabilities: group and individual responses to instruction. J. Educ. Psychol. 91, 579–593. doi: 10.1037/0022-0663.91.4.579
Tremblay, A., and Newman, A. J. (2015). Modeling nonlinear relationships in ERP data using mixed-effects regression with R examples. Psychophysiology 52, 124–139. doi: 10.1111/psyp.12299
Tucker, R., Castles, A., Laroche, A., and Deacon, S. H. (2016). The nature of orthographic learning in self-teaching: testing the extent of transfer. J. Exp. Child Psychol. 145, 79–94. doi: 10.1016/j.jecp.2015.12.007
Usai, F., O'Neil, K. G. R., and Newman, A. J. (2017). Design and empirical validation of effectiveness of LANGA, an online game-based platform for second language learning. IEEE Trans. Learn. Technol. 11, 107–114. doi: 10.1109/TLT.2017.2762688
Varga, V., Tóth, D., and Csépe, V. (2020). Orthographic-phonological mapping and the emergence of visual expertise for print: a developmental event-related potential study. Child Dev. 91, e1–e13. doi: 10.1111/cdev.13159
Vergara-Martínez, M., Comesaña, M., and Perea, M. (2017). The ERP signature of the contextual diversity effect in visual word recognition. Cogn. Affect. Behav. Neurosci. 17, 461–474. doi: 10.3758/s13415-016-0491-7
Wagenmakers, E.-J., and Farrell, S. (2004). AIC model selection using Akaike weights. Psychon. Bull. Rev. 11, 192–196. doi: 10.3758/BF03206482
Wagner, R., Torgesen, J., Rashotte, C., and Pearson, N. (2013). Comprehensive Test of Phonological Processing 2nd ed. (CTOPP-2). Austin, TX: Pro-Ed. doi: 10.1037/t52630-000
Wang, H.-C., Castles, A., Nickels, L., and Nation, K. (2011). Context effects on orthographic learning of regular and irregular words. J. Exp. Child Psychol. 109, 39–57. doi: 10.1016/j.jecp.2010.11.005
Wang, H.-C., Nickels, L., Nation, K., and Castles, A. (2013). Predictors of orthographic learning of regular and irregular words. Sci. Stud. Read. 17, 369–384. doi: 10.1080/10888438.2012.749879
Wang, M., Yang, C., and Cheng, C. (2009). The contributions of phonology, orthography, and morphology in Chinese English biliteracy acquisition. Appl. Psycholinguist. 30, 291–314. doi: 10.1017/S0142716409090122
Wechsler, D. (1999). Wechsler Abbreviated Scale of Intelligence. Austin, TX: Pro-Ed. doi: 10.1037/t15170-000
Wechsler, D. (2011). Wechsler Abbreviated Scale of Intelligence-Second Edition. Washington DC: APA PsycTests. doi: 10.1037/t15171-000
Wood, S. N. (2011). Fast stable restricted maximum likelihood and marginal likelihood estimation of semiparametric generalized linear models. J. R. Stat. Soc. 73, 3–36. doi: 10.1111/j.1467-9868.2010.00749.x
Woodcock, R. W. (1998). Woodcock Reading Mastery Tests Revised Normative Update forms G and H Examiner's Manual. San Antonio, TX: Pearson Assessment.
Xue, G., Jiang, T., Chen, C., and Dong, Q. (2008). Language experience shapes early electrophysiological responses to visual stimuli: the effects of writing system, stimulus length, and presentation duration. Neuroimage 39, 2025–2037. doi: 10.1016/j.neuroimage.2007.10.021
Zhao, J., Kipp, K., Gaspar, C., Maurer, U., Weng, X., Mecklinger, A., et al. (2014). Fine neural tuning for orthographic properties of words emerges early in children reading alphabetic script. J. Cogn. Neurosci. 26, 2431–2442. doi: 10.1162/jocn_a_00660
Keywords: language, reading, orthography, development, fluency, brain, N170, N400
Citation: Galilee A, Beck LJ, Lownie CJ, Veinot J, Mimeau C, Dempster T, Elliott LM, Deacon SH and Newman AJ (2024) Ortho-semantic learning of novel words: an event-related potential study of grade 3 children. Front. Dev. Psychol. 2:1340383. doi: 10.3389/fdpys.2024.1340383
Received: 17 November 2023; Accepted: 02 February 2024;
Published: 28 February 2024.
Edited by:
Ann Dowker, University of Oxford, United KingdomReviewed by:
Xiaoping Fang, Beijing Language and Culture University, ChinaXuejun Bai, Tianjin Normal University, China
Copyright © 2024 Galilee, Beck, Lownie, Veinot, Mimeau, Dempster, Elliott, Deacon and Newman. This is an open-access article distributed under the terms of the Creative Commons Attribution License (CC BY). The use, distribution or reproduction in other forums is permitted, provided the original author(s) and the copyright owner(s) are credited and that the original publication in this journal is cited, in accordance with accepted academic practice. No use, distribution or reproduction is permitted which does not comply with these terms.
*Correspondence: Aaron J. Newman, QWFyb24uTmV3bWFuQGRhbC5jYQ==