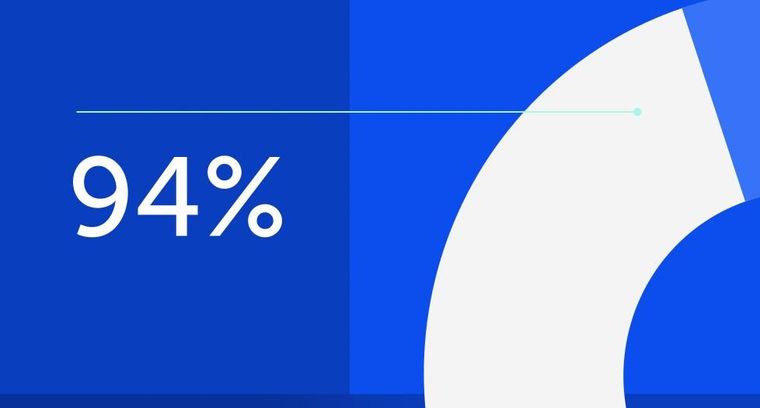
94% of researchers rate our articles as excellent or good
Learn more about the work of our research integrity team to safeguard the quality of each article we publish.
Find out more
BRIEF RESEARCH REPORT article
Front. Dent. Med.
Sec. Endodontics
Volume 6 - 2025 | doi: 10.3389/fdmed.2025.1540038
The final, formatted version of the article will be published soon.
Select one of your emails
You have multiple emails registered with Frontiers:
Notify me on publication
Please enter your email address:
If you already have an account, please login
You don't have a Frontiers account ? You can register here
Background: Failure of root canal treatment (RCT) significantly affects patient outcomes and dental practice. Understanding the association between root canal morphology and RCT outcomes can help predict treatment success. This study aimed to analyze the predictive role of root canal morphology in RCT failure.Methods: This retrospective study included 224 patients who underwent RCT. Demographic data, tooth type, and root canal morphology were also recorded. Univariate and multivariate logistic regression analyses were performed to identify predictors of RCT failure. Additionally, machine learning algorithms were employed to develop a predictive model that was evaluated using receiver operating characteristic (ROC) curves.Results: Of the 224 RCTs, 112 (50%) were classified as successful and 112 (50%) as failure.Severe canal curvature (p < 0.001) and presence of accessory canals (p = 0.002) were significant predictors of failure. The final predictive model demonstrated an area under the ROC curve (AUC) of 0.83, indicating good accuracy in distinguishing between successful and failed RCTs.These findings underscore the importance of root canal morphology in predicting RCT outcomes. Machine learning approaches can enhance clinical decision making, enabling better treatment planning for patients at a higher risk of RCT failure.
Keywords: Root canal treatment, Treatment Failure, root canal morphology, Predictive Modeling, machine learning, Logistic Regression enhancing treatment outcomes [7]
Received: 05 Dec 2024; Accepted: 08 Apr 2025.
Copyright: © 2025 Karobari, Veeraraghavan, P J, Varma, Kodangattil Narayanan and Patil. This is an open-access article distributed under the terms of the Creative Commons Attribution License (CC BY). The use, distribution or reproduction in other forums is permitted, provided the original author(s) or licensor are credited and that the original publication in this journal is cited, in accordance with accepted academic practice. No use, distribution or reproduction is permitted which does not comply with these terms.
* Correspondence:
Vishnu Priya Veeraraghavan, Saveetha Dental College And Hospitals, Chennai, 600077, Tamil Nadu, India
Prof (Dr) Santosh R Patil, Saveetha Medical College, Chennai, Tamil Nadu, India
Disclaimer: All claims expressed in this article are solely those of the authors and do not necessarily represent those of their affiliated organizations, or those of the publisher, the editors and the reviewers. Any product that may be evaluated in this article or claim that may be made by its manufacturer is not guaranteed or endorsed by the publisher.
Supplementary Material
Research integrity at Frontiers
Learn more about the work of our research integrity team to safeguard the quality of each article we publish.