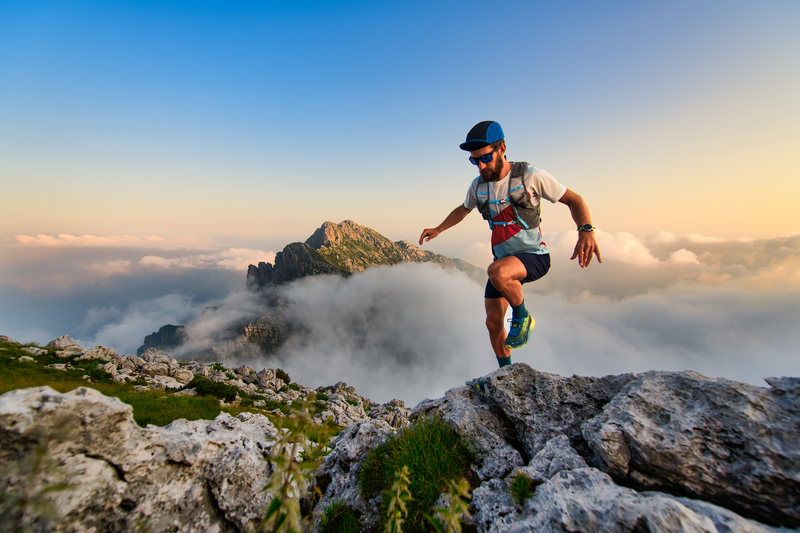
95% of researchers rate our articles as excellent or good
Learn more about the work of our research integrity team to safeguard the quality of each article we publish.
Find out more
REVIEW article
Front. Dent. Med. , 23 December 2024
Sec. Reconstructive Dentistry
Volume 5 - 2024 | https://doi.org/10.3389/fdmed.2024.1525505
This article is part of the Research Topic Revolutionizing Dentistry: The Impact of AI and new Algorithms on Diagnostics, Treatment, and Patient Engagement View all articles
Artificial intelligence (AI) technology is being used in various fields and its use is increasingly expanding in dentistry. The key aspects of AI include machine learning (ML), deep learning (DL), and neural networks (NNs). The aim of this review is to present an overview of AI, its various aspects, and its application in biomedicine, dentistry, and dental biomaterials focusing on restorative dentistry and prosthodontics. AI-based systems can be a complementary tool in diagnosis and treatment planning, result prediction, and patient-centered care. AI software can be used to detect restorations, prosthetic crowns, periodontal bone loss, and root canal segmentation from the periapical radiographs. The integration of AI, digital imaging, and 3D printing can provide more precise, durable, and patient-oriented outcomes. AI can be also used for the automatic segmentation of panoramic radiographs showing normal anatomy of the oral and maxillofacial area. Recent advancement in AI in medical and dental sciences includes multimodal deep learning fusion, speech data detection, and neuromorphic computing. Hence, AI has helped dentists in diagnosis, planning, and aid in providing high-quality dental treatments in less time.
Artificial intelligence (AI) refers to the ability of machines to exhibit a form of intelligence (1). AI is described as “a branch of science and engineering concerned with the computational understanding of what is often referred to as intelligent behavior and the development of artifacts that display such behavior” (2). At present, AI has brought a new paradigm that affects various disciplines, including science and technology, and affects everyday life (3). AI uses machines to mimic human intellectual behavior and cognitive skills like problem-solving (1, 4). The key aspects of AI include machine learning (ML), neural networks (NNs), and deep learning (DL), as illustrated in Figure 1 (1). The wide applications of these materials include information, construction, biomedicine, and biomaterials (3). AI has made it possible to analyze large amounts of data (big data) in real time and provides forecasts that can support the clinician's decisions (5).
Figure 1. Key aspects of artificial intelligence; machine learning, neural network, and deep learning.
ML is part of AI, and it depends on algorithms that can predict outcomes from datasets. It is based on algorithms trained for decision-making that robotically learn and recognize patterns from data. ML facilitates machine learning from data, and it can resolve issues/problems without people's involvement (1). DL is a constituent of ML that involves algorithms that are inspired by the structure and function of the human brain's NNs (6). DL uses deep NNs, which are composed of multiple layers of interconnected nodes, and DL constructs NNs to identify patterns to improve feature detection (1, 6). DL uses convolutional neural networks (CNNs), which is an automated feature discovery from raw data, resulting in better generalization and real-time decision-making. Hence, DL is increasingly important in medical and dental research, particularly in areas such as radiological image classification and segmentation, brain mapping with fMRI data, and diagnostic prognostication using various data types. The goals of AI research are reasoning, knowledge, planning, learning, natural language processing, perception, and moving and manipulating objects (7). Such technologies require good medical image processing of digital data, effective interpreting of diagnostic images, and applying mathematical operations for calculation and interpretation (8).
AI has led to wide applications in the medical sciences and education (9–11). The use of digital dentistry and dental computer-aided design (CAD) and computer-aided manufacturing (CAM) technologies are being used in dental education in dental education curriculum (12). Nassani et al. (13) assessed the dental students' perception of digital technologies and CAD/CAM technologies integrating the dental students in scanning, designing, and manufacturing CAD provisional fixed dental restorations. They concluded that the presence of digital technology in practice and in educational academic environments significantly improved students' interest and perception of their knowledge and skills.
AI has been used in medical science. AI in imaging is one of the most developed areas for detection, classification, and splitting tasks in computer vision (14). AI is extensively utilized in medicine, where it serves a crucial function across various domains, including diagnosis and treatment planning, clinical care, laboratory processes, virtual assistant, treatment prognosis, educational training, administrative tasks, and electronic data record (EDR) (15). Recent advancements in AI in medical sciences include multimodal deep learning fusion (MDLF), speech data detection, and neuromorphic computing. At present, MDLF techniques are used in disease detection and diagnosis (16). The MDLF techniques can enhance the capabilities of machine learning models which can result in improved accuracy (17). These techniques provide adequate complementary information from multi-modal medical images and aid in disease diagnosis.
There is an increasing tendency of aging society with more elderly populations globally with more neurological and psychiatric disorders. Hence, there is a challenge to provide adequate care for many affected individuals, their families, and caregivers. AI has helped to address these problems to some extent. The interaction of neuromorphic computing and neuroscience results in understanding the human brain's complexities and addressing neurological challenges in elderly people. The body images are fused by using Siamese convolutional neural network structure and the entropy of the images (16). Therefore, the DL models can emphasize discerning complex patterns and offer advantages over conventional machine learning approaches. Recently, speech data have also been considered valuable clinical data for the detection of various diseases (18). AI-based techniques can help to categorize the data based on underlying algorithms. Such data helps to provide information on the association with the progressive degeneration of brain cells and successive impacts on cognition, memory, and language abilities. Various neurological diseases can be prevented, which can ultimately improve oral health. Finally, the development of neuromorphic computing has led to a transformative framework for modeling neurological disorders in drug development and therapeutic interventions (6, 19).
With the rising need for health care services, the needs of the hospital are evolving from traditional service-based to internet and smart hospitals (Figure 2) (3). AI can be implemented in public health services in various ways. One good example is the digital health Quick Response (QR) code, which uses a color-coding system to detect COVID-19 and show the person's health conditions using mobile data (20). This QR code was adopted by various countries to prevent and control pandemic diseases (especially COVID-19) worldwide.
Figure 2. Applications of AI in medicine and dentistry. Adapted with permission from (3).
The aim of this review is to present an overview of AI, its various aspects, and its application in biomedicine, dentistry, and dental biomaterials focusing on restorative dentistry and prosthodontics. Articles on AI and its applications in medicine, dentistry and biomaterials were searched on PubMed, Google Scholar, Scopus, and ScienceDirect. Additional sources were also searched for additional articles and relevant articles were included in this review.
In dentistry, AI is used in various specialties, i.e., maxillofacial radiology, orthodontics, prosthodontics, dental implantology, etc. (14, 21, 22). Modern dentistry is progressing into AI-assisted data-driven and robot-assisted. Various AI technologies have been applied such as robot dental assistants in oral surgery, tooth arrangement, computer-assisted orthodontics, and material testing (23). Diagnosis of diseases mainly relies on radiologists' subjective assessment and it varies according to clinical experience (8). AI models can be used to diagnose dental caries, root fractures, maxillofacial cysts, salivary gland diseases, maxillary sinusitis, osteoporosis, cancerous lesions, lymph node metastasis, and alveolar bone loss (1, 24). AI computer algorithms have also been applied for computer-assisted diagnosis and treatment using computed tomography (CT) and electrocardiography (ECG) repeatedly without fatigue (3, 22). The AI program anticipates pathology or prognosis by prioritizing risk factors (25). The first AI-based medical product permitted by the Food and Drug Administration (FDA) is IDx-DR, which uses an AI-based model based on patient images to predict diabetic retinopathy (26). Furthermore, an AI-based medical smartphone application called SkinVision can precisely identify melanomas (27). AI can be used to detect various anatomical landmarks, such as the maxillary sinus, nasal cavity, and condyles, as shown in Figure 3.
Figure 3. AI detection of various anatomical landmarks in the axial view of CBCT; the maxillary sinus, nasal cavity, and condyles.
In orthodontics, AI models can be used to detect the need for orthodontic treatments, predict orthodontic extractions, and perform cephalometric analysis. In endodontics, AI models can be used to locate the apical foramen, assess root morphologies, predict retreatment, predict periapical pathologies, and detect root fractures (24). The different applications of AI in dentistry are illustrated in Figure 4 (25). AI is also useful in biological age and sex identification (25, 28).
Figure 4. Applications of AI in dentistry. Adapted with permission from (25).
Furthermore, AI can be used to evaluate occlusal contacts and predict mandibular morphology (1). AI can also be used for the automatic segmentation of panoramic radiographs showing normal anatomy of the oral and maxillofacial area (Figure 5). In addition, AI is also used for tooth presentation in 3D view by AI systems and automatic crown and caries detection on periapical radiographs (Figure 6). Hence, dentists are increasingly relying on computer applications and artificial intelligence models for clinical decision-making (29, 30). This approach not only supports clinical decisions but also increases the precision, efficiency, and accuracy of treatment planning and oral rehabilitation (31).
Figure 5. Automatic segmentation of a panoramic radiograph showing normal anatomy of oral and maxillofacial area.
Figure 6. AI for tooth presentation in 3D view by an AI system (A) and automatic crown and caries detection on the periapical radiograph (B) (courtesy—cranioCatch AI software).
Digital technologies and AI-based applications have streamlined dental care, simplified laborious routine tasks, increased health at lower cost, and enabled personalized and predictive dentistry (32, 33). AI functions like machines and follows a basic hierarchy; input, processing, and output, as shown in Figure 7 (25). In dentistry, data (experimental parameters and medical records) or pictures (photos and radiographic images). The results can be used for disease prediction, diagnosis, treatment, or prognosis. AI can predict treatment from the input by distinguishing normal structures, stimulating, and evaluating the outcomes (31, 34).
Figure 7. Hierarchy of AI in dentistry. Adapted with permission from (25).
AI software can be used to detect restorations, prosthetic crowns, periodontal bone loss, and root canal segmentation from the periapical radiographs as shown in Figure 8. In addition, AI software can be used in the identification and segmentation of a prosthetic crown and periapical radiolucency on a periapical radiograph (Figure 9).
Figure 8. Restoration, crown, periodontal bone loss, and root canal segmentation as observed in periapical radiographs. (Courtesy—CranioCatch AI software).
Figure 9. Identification and segmentation of a prosthetic crown and periapical radiolucency on a periapical radiograph. (Courtesy—CranioCatch AI software).
AI technology helps examine a patient's oral structure and customize treatment based on the patient's condition (35). AI can also recommend the most suitable biomaterials, designs, and technologies for prosthesis fabrication (36). Such customized prostheses have the advantages of good fit, comfort, and function (37). Furthermore, the incorporation of AI into 3D printing permits rapid fabrication of dental prostheses rapidly (36). Zirconia prostheses can be produced in-office on the same day using a speed sintering technique and delivered to the patient, providing rapid and cost-effective solutions for their patients (38–41). These use intraoral scanners and computer-aided design/computer-aided manufacturing software programs for prosthesis fabrication (40).
Furthermore, the computer-based prosthesis design uses the knowledge of bioengineering biomechanics and expert systems. A design-assisted computer application for removable partial dentures (RPD), RaPid, links knowledge-based systems, databases, and CAD systems (42). The patients' data are interpreted as transactions set on a database of design components. This application can be used in RPD and it has combined various parameters such as ethnicity, anthropological calculations, face proportions, and patient preferences for optimal esthetics. RaPiD uses design rules; that are implemented instantly when the design components are generated which describe characteristics such as the size and orientation of rests (the component that transfers force during mastication) and the connection between clasp arms and rests (retaining and reciprocating parts). The RPD design applications often require the manipulation of various component types, constraints, geometrical properties, and related computational methods. This software is useful in designing RPD.
Finally, with developments in NN, dental laboratories are using AI to create advanced dental restorations with high standards of fit, function, and esthetics (25, 43). AI is also being used for maxillofacial prostheses. With AI, a bone graft model can be created for surgical planning, and bone grafts can subsequently be created from the iliac crest. These bone grafts with reconstruction plates are used to reconstruct the mandibular defects (Figure 10).
Figure 10. AI for creating a model of bone graft from the iliac bone for reconstructing mandibular defect (courtesy of Dr. Wichuda Kongsong).
AI has been used in dental implant prosthodontics. In dental implantology, AI is used in diagnostics, treatment planning, and patient outcomes (44). In addition, AI can enhance the precision of treatment planning, enable differentiation of implant brands through ML, help in designing the implants via finite element analysis, and predict treatment outcomes (44, 45). In addition, AI is an important tool for detecting anatomical landmarks that are important for implant placement. AI helps detection of the mandibular canal using a deep convolutional neural network model (46–48). This factor plays an important role in implant planning and helps prevent per- and postoperative neurovascular complications. Morgan et al. (49) used a convolutional NN for automatic segmentation of the maxillary sinus, which allowed for the exact reproduction of 3D models for planning.
For prosthetically driven implants, precise three-dimensional (3D) placement is necessary (44). AI helps in treatment planning for dental implants by helping in decision-making. Mangano et al. (50) combined AI and augmented reality for guided implant planning in a partially edentulous patient, and their protocol was time-saving and efficient. AI can be also used to predict the primary stability of dental implants (accuracy of 93.7%) based on drilling protocols during implant surgery, which is useful for young clinicians (51). Furthermore, AI can also help identify dental implants through the convolutional neural network of deep learning by forming an identification algorithm to detect spatial features such as shape, texture, and edges (52, 53).
Finally, AI can help predict implant success and implant loss using neural networks (54–56). Dental implant failure is characterized by the features of insufficient bone volume, bone loss, and unfavorable bone quality (57). Zhang et al. (55) developed an effective implant success prediction model by studying the peri-implant alveolar bone pattern on dental periapical and panoramic films (Figure 11). They obtained features using a deep convolutional NN and built a hybrid model to combine panoramic and periapical images. Both the periapical and panoramic images showed a prediction accuracy of 87%.
Figure 11. AI and deep learning in implant success. Adapted with permission from (55).
Similarly, Liu et al. (58) studied the accuracy of an AI application for the detection of peri-implant marginal bone loss on periapical radiographs (Figure 12). For this, a Faster region-based convolutional NN (R-CNN) was trained. They found that the evaluation metrics of the AI system were equal to resident dentist. Hence, R-CNN analysis of periapical radiographs is a promising auxiliary diagnostic tool for peri-implant bone loss detection.
Figure 12. Periapical radiographs showing areas of bone loss detected by neural networks in platform-matched implants (A) and platform-switched implants (B). Adapted with permission from (58).
The orthodontic diagnosis relies on various analyses such as dental analysis, cephalometric analysis, facial analysis, skeletal analysis, and upper-airway assessment to evaluate the patient's overall profile including facial profile and dental and skeletal relationship (59). Clinical orthodontics requires a significant time for the analysis of each case. With the advancement of computing capabilities and AI algorithms, AI applications are expanding in orthodontics and pediatric dentistry. At present, AI can help in the analysis, diagnosis, and treatment planning, and significantly enhance clinical practice (59, 60). Various AI-driven software such as 3Shape Dental System, Mastro 3D, Uceph, etc. are used widely in orthodontics. The software is undergoing continuous upgrades and developments.
Currently, there is no standardized formula to do extraction for orthodontic alignment and the decision depends on the orthodontists' experience (61). Incorrect decisions can cause irreversible problems such as difficulty closing the extraction space, improper occlusion, and unfavorable profile. AI can assist orthodontists in making decisions and reducing incorrect extractions.
Predicting treatment outcomes in orthodontics and pediatric dentistry is important. Currently, AI helps in predicting dental, skeletal, and facial changes thereby guiding treatment planning (62–65). Furthermore, AI to predict skeletal and facial changes after orthodontic treatments. For patients with severe dentofacial deformities, combined orthodontic and orthognathic surgical treatment is required to correct skeletal deformity (66, 67). Lateral cephalograms are being used to assess sagittal skeletal deformities and there is high accuracy in predicting orthognathic surgery diagnosis (68). Tanikawa and Yamashiro (63) developed AI systems that predict the 3D facial profile and morphology after orthognathic surgery and orthodontic treatment based on the results of previous treatment (Figure 13). The success rates of error of <1 mm were 54% and 98% for systems of orthognathic surgery and orthodontic treatment, respectively. The total success rate of error of <2 mm was 100%. Hence, AI systems to predict facial profile and morphology following treatment were clinically acceptable.
Figure 13. Use of AI in surgical planning in maxillo-mandibular skeletal deformity. (A) The actual facial changes in the surgery group for pre-treatment, superimposition, and post-treatment. (B) The average actual facial changes in the extraction group for pre-treatment, and the superimposition post-treatment. Adapted with permission from Ref. (63).
Over recent decades, a novel discipline within materials science has emerged, focusing on biomaterials. Biomaterials are substances, whether synthetic or natural in origin, that are used to enhance, treat, substitute, or regenerate tissues (69). Currently, biomaterials have extensive applications in medicine and dentistry (70). The trend is shifting from replacement to regeneration (Figure 14) (71). Biomaterials can be broadly classified into metals, polymers, ceramics (including carbon-based materials, ceramics, and glass), and composites (72). Ceramic materials include carbon-based materials, ceramics, and glasses. In the last half-decade, there has been increasing interest in biomaterials for dental applications, and numerous new biomaterials have been introduced for these applications (73–75).
Figure 14. The history and shift of biomaterials: from replacement to regeneration. Adapted with permission from (71).
With the wide applications of materials ranging from information, transportation, construction, and biomedicine (3), AI has been implemented in materials science. Recently, AI has made important improvements in rational design and has accelerated the discovery of different biomaterials (3).
With the rapid progress of data handling and algorithms, ML and DL are applied in the search for new biomaterials before actually producing them (76). By incorporating various biomaterial data (such as the integral element, atomic weight and radius, lattice symmetry, magnetism, binding energy, polarization, electronegativity, band energy, and structural property), the machines are trained to improve the design of new materials and predict properties cost-effectively (Figure 15) (3). Data-driven computing and algorithms can help in the simulation and ML of property prediction and material discovery.
Figure 15. AI-powered development in materials science. Adapted with permission from (3).
AI tools can be adapted for experimental testing of materials and use computing, automation, and ML to calculate material properties such as bulk, interface, and defects (77). In the future, AI techniques can be used to design metals, superconductors, glasses, alloys, magnetocaloric materials, thermoelectric materials, polymeric materials, composite materials, 2D materials, and topological (electronic and phonon) materials (3). A precise AI-based model needs to be developed to forecast the formation energy of a compound to achieve good predictive ability (78).
AI has helped to replace the costly trial and error by developing novel biomaterials. The use of AI methods, notably high-throughput experimentation, has significantly improved the design and production of biomaterials. The major reason for this is the increase in the scope of outcomes to encompass the FDA-endorsed excipient database. The implementation of AI techniques has demonstrated the potential to revolutionize biomaterial development by improving the efficiency and accuracy of research (79). To improve the functionality and application effectiveness of hydrogels, the use of AI has brought about unprecedented advancements in the fields of material design and the optimization of the preparation process. AI has been instrumental in enabling detailed material screening and analysis, performance monitoring, and control. Material characterization analysis has also significantly benefitted from the application of AI technology (80).
AI using 3D printing techniques has been extremely valuable for transforming various data and images to produce prostheses and devices (81). Data collected from 3D instruments from various sources are computed using dental CAD models designed using software, and prostheses are printed using 3D printing technology (82, 83). AI-supported 3D printing facilitates the manufacture of different types of implants and prostheses (3, 84). The prostheses reproduce the normal anatomy precisely and can be customized to help improve function and esthetics. AI can accelerate the fabrication process; hence, the reconstruction process is also quicker (85). Apart from printing the maxillofacial anatomical structures, limbs (i.e., hands and legs) can also be printed (86, 87). The printed limbs can also be supported with myoelectric stimulation support (88, 89). Recent studies have also tried to print bionic ears in models simulating artificial environments, but clinical trials are yet to be conducted soon (90). One advantage of AI is the fabrication of prostheses in children. In children, prostheses need to be changed frequently, which is time-consuming and costly. However, with 3D printing technology using light printing materials, the time and cost can be significantly reduced, and prostheses can be used when needed (3). 3D-printed prostheses in children have better functionality and movement (91).
Although AI holds great promise, it also faces several challenges and ethical considerations. AI in dentistry is rapidly exploring new uses of AI for electronic health records, image analysis, and prosthesis design (92). Atlas-based and statistical shape models are important in capturing the shape, appearance, and location of organs but still a challenge to capture inter-subject variability (8).
Despite a progressive improvement, automatic cephalometry cannot completely replace manual tracing (93) and AI has exceeded the recommended magnitude of error for most cephalometric landmarks (94). It was also found that the AI automatic landmarking on 3D CBCT is found to be less accurate compared to 2D x-rays. Hence, AI-driven radiographic landmarking tracing requires the final supervision and approval of an experienced orthodontist.
Furthermore, important considerations include instilling knowledge of the basic knowledge and application of AI, examining current and potential ethical practices, and discussing its limitations (92, 95). Dental professionals need to understand and acclimatize themselves to such advances for better oral healthcare delivery (96). Finally, AI requires careful governance similar to the governance of physician conduct. Regulatory guidelines are needed regarding safely implementing and assessing AI technology (97).
In dentistry, AI technologies can be used as an important tool for treatment planning, diagnosis, prediction of treatment outcomes, and patient-centered care. The integration and continuous improvement of AI has brought significant advancements in dentistry. Various AI-driven software are going through continuous upgrades and developments. The application of AI in dentistry has made promising progress and has great potential for wider clinical applications in the near future.
The future of AI in dentistry extends into dental education, where AI-powered tools offer interactive learning, virtual patient simulations, and personalized feedback (98). Such technologies can allow dental students to practice clinical skills in a controlled, digital environment, preparing them for real-world scenarios.
Although AI has wide applications in dentistry, dental procedures performed by machines without human interaction are not representative of clinical care (25). There is also a risk of fully applying AI in dentistry without human control. AI has shown various errors in analysis and diagnosis. Hence, to avoid such errors, the final evaluation should be done by an experienced dentist.
Human-to-human communication is very difficult to translate directly into computer language and coding (4). Finally, the computational complexity of generating and selecting an atlas is a big challenge. Data safety, security, and privacy are critical aspects of integrating AI into health care (36, 92). Further long-term studies are needed into the specific capabilities to fully integrate AI into clinical practice.
The application of AI-based systems in dentistry and dental biomaterials is continually increasing. Recent advancement in AI in medical and dental sciences includes multimodal deep learning fusion, speech data detection, and neuromorphic computing. In the future, with the help of generative AI, dentists can prepare patient-specific prostheses for specific oral conditions. The application of AI-based technologies in prosthodontics is desirable in various aspects from clinicians' and patients' points of view. In prosthodontics, they have a tangible influence on widening opportunities for clinicians as well as patients and can be used as an additional simple tool for assembling, handling, and establishing patient-related datasets to deliver individual, patient-centered, and personalized treatment. In orthodontics, AI has contributed to diagnosis, treatment planning, and clinical practice. AI has made important improvements in rational design and has accelerated the discovery of various biomaterials used in dentistry. At present, AI still cannot fully replace human experts and it can serve as an important component in clinical dentistry.
DR: Conceptualization, Data curation, Formal Analysis, Funding acquisition, Investigation, Methodology, Project administration, Resources, Software, Validation, Visualization, Writing – original draft, Writing – review & editing. AJ: Conceptualization, Data curation, Formal Analysis, Methodology, Project administration, Resources, Software, Supervision, Validation, Visualization, Writing – review & editing. RJ: Conceptualization, Data curation, Methodology, Resources, Software, Validation, Visualization, Writing – review & editing. VS: Conceptualization, Data curation, Formal Analysis, Funding acquisition, Investigation, Methodology, Project administration, Resources, Software, Supervision, Validation, Visualization, Writing – original draft, Writing – review & editing.
The author(s) declare financial support was received for the research, authorship, and/or publication of this article. This research work is supported by the Chulalongkorn University Visiting Scholar Grant (Grant No 2010041000).
The authors declare that the research was conducted in the absence of any commercial or financial relationships that could be construed as a potential conflict of interest.
The author(s) declared that Dinesh Rokaya is an editorial board member of Frontiers, at the time of submission. This had no impact on the peer review process and the final decision.
All claims expressed in this article are solely those of the authors and do not necessarily represent those of their affiliated organizations, or those of the publisher, the editors and the reviewers. Any product that may be evaluated in this article, or claim that may be made by its manufacturer, is not guaranteed or endorsed by the publisher.
1. Khanagar SB, Al-Ehaideb A, Maganur PC, Vishwanathaiah S, Patil S, Baeshen HA, et al. Developments, application, and performance of artificial intelligence in dentistry—a systematic review. J Dent Sci. (2021) 16(1):508–22. doi: 10.1016/j.jds.2020.06.019
3. Xu Y, Liu X, Cao X, Huang C, Liu E, Qian S, et al. Artificial intelligence: a powerful paradigm for scientific research. Innovation. (2021) 2(4):100179. doi: 10.1016/j.xinn.2021.100179
4. Shan T, Tay FR, Gu L. Application of artificial intelligence in dentistry. J Dent Res. (2021) 100(3):232–44. doi: 10.1177/0022034520969115
5. Bellini V, Cascella M, Cutugno F, Russo M, Lanza R, Compagnone C, et al. Understanding basic principles of artificial intelligence: a practical guide for intensivists. Acta Biomed. (2022) 93(5):e2022297. doi: 10.23750/abm.v93i5.13626
6. Raikar AS, Andrew J, Dessai PP, Prabhu SM, Jathar S, Prabhu A, et al. Neuromorphic computing for modeling neurological and psychiatric disorders: implications for drug development. Artif Intell Rev. (2024) 57(12):318. doi: 10.1007/s10462-024-10948-3
7. Sniecinski I, Seghatchian J. Artificial intelligence: a joint narrative on potential use in pediatric stem and immune cell therapies and regenerative medicine. Transfus Apher Sci. (2018) 57(3):422–4. doi: 10.1016/j.transci.2018.05.004
8. Jain S, Sikka G, Dhir R. A systematic literature review on pancreas segmentation from traditional to non-supervised techniques in abdominal medical images. Artif Intell Rev. (2024) 57(12):317. doi: 10.1007/s10462-024-10966-1
9. Vodanović M, Subašić M, Milošević D, Savić Pavičin I. artificial intelligence in medicine and dentistry. Acta Stomatol Croat. (2023) 57(1):70–84. doi: 10.15644/asc57/1/8
10. Sarfaraz S, Khurshid Z, Zafar MS. Use of artificial intelligence in medical education: a strength or an infirmity. J Taibah Univ Med Sci. (2023) 18(6):1553–4. doi: 10.1016/j.jtumed.2023.06.008
11. Bindra S, Jain R. Artificial intelligence in medical science: a review. Ir J Med Sci. (2024) 193(3):1419–29. doi: 10.1007/s11845-023-03570-9
12. Afshari FS, Sukotjo C, Alfaro MF, McCombs J, Campbell SD, Knoernschild KL, et al. Integration of digital dentistry into a predoctoral implant program: program description, rationale, and utilization trends. J Dent Educ. (2017) 81(8):986–94. doi: 10.21815/JDE.017.050
13. Nassani LM, Bencharit S, Schumacher F, Lu WE, Resende R, Fernandes GVO. The impact of technology teaching in the dental predoctoral curriculum on students’ perception of digital dentistry. Dent J. (2024) 12(3):75. doi: 10.3390/dj12030075
14. Dashti M, Londono J, Ghasemi S, Tabatabaei S, Hashemi S, Baghaei K, et al. Evaluation of accuracy of deep learning and conventional neural network algorithms in detection of dental implant type using intraoral radiographic images: a systematic review and meta-analysis. J Prosthet Dent. (2024) In Press. https://www.sciencedirect.com/science/article/pii/S0022391323008120
15. Nasarudin NA, Al Jasmi F, Sinnott RO, Zaki N, Al Ashwal H, Mohamed EA, et al. A review of deep learning models and online healthcare databases for electronic health records and their use for health prediction. Artif Intell Rev. (2024) 57(9):249. doi: 10.1007/s10462-024-10876-2
16. Sangeetha Francelin Vinnarasi F, Daniel J, Anita Rose JT, Pugalenthi R. Deep learning supported disease detection with multi-modality image fusion. J Xray Sci Technol. (2021) 29(3):411–34. doi: 10.3233/XST-210851
17. Nakach F-Z, Idri A, Goceri E. A comprehensive investigation of multimodal deep learning fusion strategies for breast cancer classification. Artif Intell Rev. (2024) 57(12):327. doi: 10.1007/s10462-024-10984-z
18. Ding K, Chetty M, Noori Hoshyar A, Bhattacharya T, Klein B. Speech based detection of Alzheimer’s disease: a survey of AI techniques, datasets and challenges. Artif Intell Rev. (2024) 57(12):325. doi: 10.1007/s10462-024-10961-6
19. Dzobo K, Adotey S, Thomford NE, Dzobo W. Integrating artificial and human intelligence: a partnership for responsible innovation in biomedical engineering and medicine. OMICS. (2020) 24(5):247–63. doi: 10.1089/omi.2019.0038
20. Huang Y, Wu Q, Wang P, Xu Y, Wang L, Zhao Y, et al. Measures undertaken in China to avoid COVID-19 infection: internet-based, cross-sectional survey study. J Med Internet Res. (2020) 22(5):e18718. doi: 10.2196/18718
21. Talesara V, Bennani V, Aarts J, Ratnayake J, Khurshid Z, Brunton P. Accuracy of digitally coded healing abutments: a systematic review. Saudi Dent J. (2023) 35(8):891–903. doi: 10.1016/j.sdentj.2023.08.006
22. Orhan K, Jagtap R. Introduction to artificial intelligence. In: Orhan K, Jagtap R, editors. Artificial Intelligence in Dentistry. Cham: Springer International Publishing (2023). p. 1–7.
23. Nassani LM, Javed K, Amer RS, Pun MHJ, Abdelkarim AZ, Fernandes GVO. Technology readiness level of robotic technology and artificial intelligence in dentistry: a comprehensive review. Surgeries. (2024) 5(2):273–87. doi: 10.3390/surgeries5020025
24. Boreak N. Effectiveness of artificial intelligence applications designed for endodontic diagnosis, decision-making, and prediction of prognosis: a systematic review. J Contemp Dent Pract. (2020) 21(8):926–34. doi: 10.5005/jp-journals-10024-2894
25. Agrawal P, Nikhade P. Artificial intelligence in dentistry: past, present, and future. Cureus. (2022) 14(7):e27405. doi: 10.7759/cureus.27405
26. Grzybowski A, Brona P. Analysis and comparison of two artificial intelligence diabetic retinopathy screening algorithms in a pilot study: IDx-DR and retinalyze. J Clin Med. (2021) 10(11):2352. doi: 10.3390/jcm10112352
27. Freeman K, Dinnes J, Chuchu N, Takwoingi Y, Bayliss SE, Matin RN, et al. Algorithm based smartphone apps to assess risk of skin cancer in adults: systematic review of diagnostic accuracy studies. BMJ. (2020) 368:m127. doi: 10.1136/bmj.m127
28. Mohammad N, Ahmad R, Kurniawan A, Mohd Yusof MYP. Applications of contemporary artificial intelligence technology in forensic odontology as primary forensic identifier: a scoping review. Front Artif Intell. (2022) 5:1049584. doi: 10.3389/frai.2022.1049584
29. Schleyer TK, Thyvalikakath TP, Spallek H, Torres-Urquidy MH, Hernandez P, Yuhaniak J. Clinical computing in general dentistry. J Am Med Inform Assoc. (2006) 13(3):344–52. doi: 10.1197/jamia.M1990
30. Chae YM, Yoo KB, Kim ES, Chae H. The adoption of electronic medical records and decision support systems in Korea. Healthc Inform Res. (2011) 17(3):172–7. doi: 10.4258/hir.2011.17.3.172
31. Khurshid Z. Digital dentistry: transformation of oral health and dental education with technology. Eur J Dent. (2023) 17(4):943–4. doi: 10.1055/s-0043-1772674
32. Schwendicke F, Samek W, Krois J. Artificial intelligence in dentistry: chances and challenges. J Dent Res. (2020) 99(7):769–74. doi: 10.1177/0022034520915714
33. Humagain M, Rokaya D. Integrating digital technologies in dentistry to enhance the clinical success. Kathmandu Univ Med J. (2019) 17(68):256–7. https://www.kumj.com.np/issue/68/256-257.pdf
34. Londono J, Ghasemi S, Hussain Shah A, Fahimipour A, Ghadimi N, Hashemi S, et al. Evaluation of deep learning and convolutional neural network algorithms accuracy for detecting and predicting anatomical landmarks on 2D lateral cephalometric images: a systematic review and meta-analysis. Saudi Dent J. (2023) 35(5):487–97. doi: 10.1016/j.sdentj.2023.05.014
35. Chadha U, Abrol A, Vora NP, Tiwari A, Shanker SK, Selvaraj SK. Performance evaluation of 3D printing technologies: a review, recent advances, current challenges, and future directions. Prog Addit Manuf. (2022) 7(5):853–86. doi: 10.1007/s40964-021-00257-4
36. Heboyan A, Yazdanie N, Ahmed N. Glimpse into the future of prosthodontics: the synergy of artificial intelligence. World J Clin Cases. (2023) 11(33):7940–2. doi: 10.12998/wjcc.v11.i33.7940
37. Alammar A, Kois JC, Revilla-León M, Att W. Additive manufacturing technologies: current Status and future perspectives. J Prosthodont. (2022) 31(S1):4–12. doi: 10.1111/jopr.13477
38. Liu H, Inokoshi M, Xu K, Tonprasong W, Minakuchi S, Van Meerbeek B, et al. Does speed-sintering affect the optical and mechanical properties of yttria-stabilized zirconia? A systematic review and meta-analysis of in vitro studies. Jpn Dent Sci Rev. (2023) 59:312–28. doi: 10.1016/j.jdsr.2023.08.007
39. Kongkiatkamon S, Peampring C. Comparison of regular and speed sintering on low-temperature degradation and fatigue resistance of translucent zirconia crowns for implants: an in vitro study. J Funct Biomater. (2022) 13(4):281. doi: 10.3390/jfb13040281
40. Perlea P, Stefanescu C, Al-Aloul OA, Ionita C, Petre AE. Digital workflow for producing hybrid posts and cores. Healthcare. (2023) 11(5):727. doi: 10.3390/healthcare11050727
41. Saini RS, Alshadidi AAF, Gurumurthy V, Okshah A, Vaddamanu SK, Binduhayyim RIH, et al. Quantum mechanical analysis of yttrium-stabilized zirconia and alumina: implications for mechanical performance of esthetic crowns. Eur J Med Res. (2024) 29(1):254. doi: 10.1186/s40001-024-01851-2
42. Hammond P, Davenport JC, Fitzpatrick FJ. Logic-based integrity constraints and the design of dental prostheses. Artif Intell Med. (1993) 5:431–46. doi: 10.1016/0933-3657(93)90035-2
43. Banerjee M. Artificial intelligence in dentistry: a ray of hope. CODS J Dent. (2021) 13(2):58–60. doi: 10.5005/jp-journals-10063-0121
44. Altalhi AM, Alharbi FS, Alhodaithy MA, Almarshedy BS, Al-Saaib MY, Al Jfshar RM, et al. The impact of artificial intelligence on dental implantology: a narrative review. Cureus. (2023) 15(10):e47941. doi: 10.7759/cureus.47941
45. Amornvit P, Rokaya D, Keawcharoen K, Thongpulsawasdi N. Stress distribution in implant retained finger prosthesis: a finite element study. J Clin Diagn Res. (2013) 7(12):2851–4. doi: 10.7860/JCDR/2013/7001.3775
46. Kwak GH, Kwak EJ, Song JM, Park HR, Jung YH, Cho BH, et al. Automatic mandibular canal detection using a deep convolutional neural network. Sci Rep. (2020) 10(1):5711. doi: 10.1038/s41598-020-62586-8
47. Jindanil T, Marinho-Vieira LE, de-Azevedo-Vaz SL, Jacobs R. A unique artificial intelligence-based tool for automated CBCT segmentation of mandibular incisive canal. Dentomaxillofac Radiol. (2023) 52(8):20230321. doi: 10.1259/dmfr.20230321
48. Lahoud P, Diels S, Niclaes L, Van Aelst S, Willems H, Van Gerven A, et al. Development and validation of a novel artificial intelligence driven tool for accurate mandibular canal segmentation on CBCT. J Dent. (2022) 116:103891. doi: 10.1016/j.jdent.2021.103891
49. Morgan N, Van Gerven A, Smolders A, de Faria Vasconcelos K, Willems H, Jacobs R. Convolutional neural network for automatic maxillary sinus segmentation on cone-beam computed tomographic images. Sci Rep. (2022) 12(1):7523. doi: 10.1038/s41598-022-11483-3
50. Mangano FG, Admakin O, Lerner H, Mangano C. Artificial intelligence and augmented reality for guided implant surgery planning: a proof of concept. J Dent. (2023) 133:104485. doi: 10.1016/j.jdent.2023.104485
51. Sakai T, Li H, Shimada T, Kita S, Iida M, Lee C, et al. Development of artificial intelligence model for supporting implant drilling protocol decision making. J Prosthodont Res. (2023) 67(3):360–5. doi: 10.2186/jpr.JPR_D_22_00053
52. da Mata Santos RP, Vieira Oliveira Prado HE, Soares Aranha Neto I, Alves de Oliveira GA, Vespasiano Silva AI, Zenóbio EG, et al. Automated identification of dental implants using artificial intelligence. Int J Oral Maxillofac Implants. (2021) 36(5):918–23. doi: 10.11607/jomi.8684
53. Revilla-León M, Gómez-Polo M, Vyas S, Barmak BA, Galluci GO, Att W, et al. Artificial intelligence applications in implant dentistry: a systematic review. J Prosthet Dent. (2023) 129(2):293–300. doi: 10.1016/j.prosdent.2021.05.008
54. Huang N, Liu P, Yan Y, Xu L, Huang Y, Fu G, et al. Predicting the risk of dental implant loss using deep learning. J Clin Periodontol. (2022) 49(9):872–83. doi: 10.1111/jcpe.13689
55. Zhang C, Fan L, Zhang S, Zhao J, Gu Y. Deep learning based dental implant failure prediction from periapical and panoramic films. Quant Imaging Med Surg. (2023) 13(2):935–45. doi: 10.21037/qims-22-457
56. Kohlakala A, Coetzer J, Bertels J, Vandermeulen D. Deep learning-based dental implant recognition using synthetic x-ray images. Med Biol Eng Comput. (2022) 60(10):2951–68. doi: 10.1007/s11517-022-02642-9
57. Rokaya D, Srimaneepong V, Wisitrasameewon W, Humagain M, Thunyakitpisal P. Peri-implantitis update: risk indicators, diagnosis, and treatment. Eur J Dent. (2020) 14(4):672–82. doi: 10.1055/s-0040-1715779
58. Liu M, Wang S, Chen H, Liu Y. A pilot study of a deep learning approach to detect marginal bone loss around implants. BMC Oral Health. (2022) 22(1):11. doi: 10.1186/s12903-021-02035-8
59. Liu J, Zhang C, Shan Z. Application of artificial intelligence in orthodontics: current state and future perspectives. Healthcare. (2023) 11(20):2760. doi: 10.3390/healthcare11202760
60. Albalawi F, Alamoud KA. Trends and application of artificial intelligence technology in orthodontic diagnosis and treatment planning—a review. Appl Sci. (2022) 12(22):11864. doi: 10.3390/app122211864
61. Ribarevski R, Vig P, Vig KD, Weyant R, O'Brien K. Consistency of orthodontic extraction decisions. Eur J Orthod. (1996) 18(1):77–80. doi: 10.1093/ejo/18.1.77
62. Lee H, Ahmad S, Frazier M, Dundar MM, Turkkahraman H. A novel machine learning model for class III surgery decision. J Orofac Orthop. (2024) 85(4):239–49. doi: 10.1007/s00056-022-00421-7
63. Tanikawa C, Yamashiro T. Development of novel artificial intelligence systems to predict facial morphology after orthognathic surgery and orthodontic treatment in Japanese patients. Sci Rep. (2021) 11(1):15853. doi: 10.1038/s41598-021-95002-w
64. Xu L, Mei L, Lu R, Li Y, Li H, Li Y. Predicting patient experience of Invisalign treatment: an analysis using artificial neural network. Korean J Orthod. (2022) 52(4):268–77. doi: 10.4041/kjod21.255
65. Chang Q, Bai Y, Wang S, Wang F, Wang Y, Zuo F, et al. Automatic soft-tissue analysis on orthodontic frontal and lateral facial photographs based on deep learning. Orthod Craniofac Res. (2024) 27(6):893–902. doi: 10.1111/ocr.12830
66. Duran GS, Gökmen Ş, Topsakal KG, Görgülü S. Evaluation of the accuracy of fully automatic cephalometric analysis software with artificial intelligence algorithm. Orthod Craniofac Res. (2023) 26(3):481–90. doi: 10.1111/ocr.12633
67. Kiełczykowski M, Kamiński K, Perkowski K, Zadurska M, Czochrowska E. Application of artificial intelligence (AI) in a cephalometric analysis: a narrative review. Diagnostics. (2023) 13(16):2640. doi: 10.3390/diagnostics13162640
68. Shin W, Yeom GH, Lee GH, Yun JP, Jeong SH, Lee JH, et al. Deep learning based prediction of necessity for orthognathic surgery of skeletal malocclusion using cephalogram in Korean individuals. BMC Oral Health. (2021) 21(1):130. doi: 10.1186/s12903-021-01513-3
69. Eldeeb AE, Salah S, Elkasabgy NA. Biomaterials for tissue engineering applications and current updates in the field: a comprehensive review. AAPS PharmSciTech. (2022) 23(7):267. doi: 10.1208/s12249-022-02419-1
70. Heboyan A, Bennardo F. New biomaterials for modern dentistry. BMC Oral Health. (2023) 23(1):817. doi: 10.1186/s12903-023-03531-9
71. Todros S, Todesco M, Bagno A. Biomaterials and their biomedical applications: from replacement to regeneration. Processes. (2021) 9(11):1949. doi: 10.3390/pr9111949
72. Vaiani L, Boccaccio A, Uva AE, Palumbo G, Piccininni A, Guglielmi P, et al. Ceramic materials for biomedical applications: an overview on properties and fabrication processes. J Funct Biomater. (2023) 14(3):146. doi: 10.3390/jfb14030146
73. Alqurashi H, Khurshid Z, Syed AUY, Rashid Habib S, Rokaya D, Zafar MS. Polyetherketoneketone (PEKK): an emerging biomaterial for oral implants and dental prostheses. J Adv Res. (2021) 28:87–95. doi: 10.1016/j.jare.2020.09.004
74. Heboyan A, Zafar MS, Rokaya D, Khurshid Z. Insights and advancements in biomaterials for prosthodontics and implant dentistry. Molecules. (2022) 27(16):5116. doi: 10.3390/molecules27165116
75. Tuna EB, Oshida Y, Ozen B, Gjorgievska E, Tuzuner T. Biomaterials for dental applications. Biomed Res Int. (2017) 2017:2520536. doi: 10.1155/2017/2520536
76. Mahmood A, Wang J-L. Machine learning for high performance organic solar cells: current scenario and future prospects. Energy Environ Sci. (2021) 14(1):90–105. doi: 10.1039/D0EE02838J
77. Correa-Baena JP, Hippalgaonkar K, van Duren J, Jaffer S, Chandrasekhar VR, Stevanovic V, et al. Accelerating materials development via automation, machine learning, and high-performance computing. Joule. (2018) 2(8):1410–20. doi: 10.1016/j.joule.2018.05.009
78. Liang Y, Chen M, Wang Y, Jia H, Lu T, Xie F, et al. A universal model for accurately predicting the formation energy of inorganic compounds. Sci China Mater. (2023) 66(1):343–51. doi: 10.1007/s40843-022-2134-3
79. Reker D, Rybakova Y, Kirtane AR, Cao R, Yang JW, Navamajiti N, et al. Computationally guided high-throughput design of self-assembling drug nanoparticles. Nat Nanotechnol. (2021) 16(6):725–33. doi: 10.1038/s41565-021-00870-y
80. Lashari N, Ganat T, Otchere D, Kalam S, Ali I. Navigating viscosity of GO-SiO2/HPAM composite using response surface methodology and supervised machine learning models. J Pet Sci Eng. (2021) 205:108800. doi: 10.1016/j.petrol.2021.108800
81. Qian C, Zheng B, Shen Y, Jing L, Li E, Shen L, et al. Deep-learning-enabled self-adaptive microwave cloak without human intervention. Nat Photonics. (2020) 14(6):383–90. doi: 10.1038/s41566-020-0604-2
82. Balestra D, Lowther M, Goracci C, Mandurino M, Cortili S, Paolone G, et al. 3D printed materials for permanent restorations in indirect restorative and prosthetic dentistry: a critical review of the literature. Materials (Basel). (2024) 17(6):1380. doi: 10.3390/ma17061380
83. Amornvit P, Rokaya D, Sanohkan S. Comparison of accuracy of current ten intraoral scanners. Biomed Res Int. (2021) 2021:2673040. doi: 10.1155/2021/2673040
84. Dimitrova M, Vlahova A, Kalachev Y, Zlatev S, Kazakova R, Capodiferro S. Recent advances in 3D printing of polymers for application in prosthodontics. Polymers (Basel). (2023) 15(23):4525. doi: 10.3390/polym15234525
85. Cortes C, Vapnik V. Support-vector networks. Mach Learn. (1995) 20(3):273–97. doi: 10.1007/BF00994018
86. Ten Kate J, Smit G, Breedveld P. 3D-printed upper limb prostheses: a review. Disabil Rehabil Assist Technol. (2017) 12(3):300–14. doi: 10.1080/17483107.2016.1253117
87. Vujaklija I, Farina D. 3D printed upper limb prosthetics. Expert Rev Med Devices. (2018) 15(7):505–12. doi: 10.1080/17434440.2018.1494568
88. Srimaneepong V, Heboyan A, Syed AUY, Trinh HA, Amornvit P, Rokaya D. Recent advances in myoelectric control for finger prostheses for multiple finger loss. Appl Sci. (2021) 11(10):4464. doi: 10.3390/app11104464
89. Kumar DK, Jelfs B, Sui X, Arjunan SP. Prosthetic hand control: a multidisciplinary review to identify strengths, shortcomings, and the future. Biomed Signal Process Control. (2019) 53:101588. doi: 10.1016/j.bspc.2019.101588
90. McCulloch WS, Pitts W. A logical calculus of the ideas immanent in nervous activity. Bull Math Biophys. (1943) 5(4):115–33. doi: 10.1007/BF02478259
91. Freund Y, Schapire RE. Experiments with a new boosting algorithm. Machine Learning: Proceedings of the Thirteenth International Conference: 1996 (1996), p. 148–56
92. Kim CS, Samaniego CS, Sousa Melo SL, Brachvogel WA, Baskaran K, Rulli D. Artificial intelligence (A.I.) in dental curricula: ethics and responsible integration. J Dent Educ. (2023) 87(11):1570–3. doi: 10.1002/jdd.13337
93. Bao H, Zhang K, Yu C, Li H, Cao D, Shu H, et al. Evaluating the accuracy of automated cephalometric analysis based on artificial intelligence. BMC Oral Health. (2023) 23(1):191. doi: 10.1186/s12903-023-02881-8
94. Polizzi A, Leonardi R. Automatic cephalometric landmark identification with artificial intelligence: an umbrella review of systematic reviews. J Dent. (2024) 146:105056. doi: 10.1016/j.jdent.2024.105056
95. Orhan K, Gülbeş MM, Jadhav A, Jagtap R. Medico-Legal problems of artificial intelligence. In: Orhan K, Jagtap R, editors. Artificial Intelligence in Dentistry. Cham: Springer International Publishing (2023). p. 259–82.
96. Amisha MP, Pathania M, Rathaur VK. Overview of artificial intelligence in medicine. J Family Med Prim Care. (2019) 8(7):2328–31. doi: 10.4103/jfmpc.jfmpc_440_19
97. Aung YYM, Wong DCS, Ting DSW. The promise of artificial intelligence: a review of the opportunities and challenges of artificial intelligence in healthcare. Br Med Bull. (2021) 139(1):4–15. doi: 10.1093/bmb/ldab016
Keywords: artificial intelligence, machine learning, deep learning, neural networks, medicine, dentistry, dental medicine, dental biomaterials
Citation: Rokaya D, Jaghsi AA, Jagtap R and Srimaneepong V (2024) Artificial intelligence in dentistry and dental biomaterials. Front. Dent. Med 5:1525505. doi: 10.3389/fdmed.2024.1525505
Received: 9 November 2024; Accepted: 6 December 2024;
Published: 23 December 2024.
Edited by:
Rodrigo Resende, Fluminense Federal University, BrazilReviewed by:
Valentino Natoli, European University of Madrid, SpainCopyright: © 2024 Rokaya, Jaghsi, Jagtap and Srimaneepong. This is an open-access article distributed under the terms of the Creative Commons Attribution License (CC BY). The use, distribution or reproduction in other forums is permitted, provided the original author(s) and the copyright owner(s) are credited and that the original publication in this journal is cited, in accordance with accepted academic practice. No use, distribution or reproduction is permitted which does not comply with these terms.
*Correspondence: Viritpon Srimaneepong, dmlyaXRwb24uc0BjaHVsYS5hYy50aA==
Disclaimer: All claims expressed in this article are solely those of the authors and do not necessarily represent those of their affiliated organizations, or those of the publisher, the editors and the reviewers. Any product that may be evaluated in this article or claim that may be made by its manufacturer is not guaranteed or endorsed by the publisher.
Research integrity at Frontiers
Learn more about the work of our research integrity team to safeguard the quality of each article we publish.