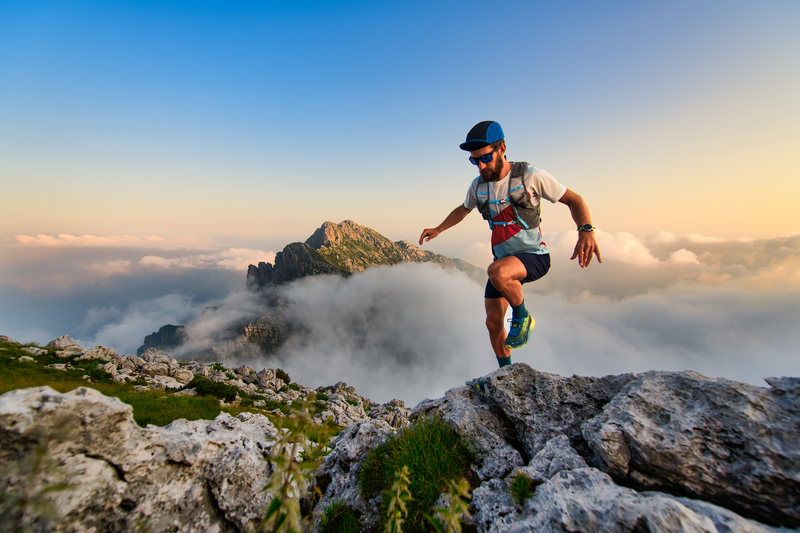
94% of researchers rate our articles as excellent or good
Learn more about the work of our research integrity team to safeguard the quality of each article we publish.
Find out more
REVIEW article
Front. Dent. Med , 19 August 2021
Sec. Dental Materials
Volume 2 - 2021 | https://doi.org/10.3389/fdmed.2021.689288
This article is part of the Research Topic Biofunctional Applications of Zirconia in Dental Devices View all 5 articles
Artificial intelligence (AI) is rapidly developed based on computer technology, which can perform tasks that customarily require human intelligence by building intelligent software or machines. As a subfield of AI, machine learning (ML) can learn from the intrinsic statistical patterns and structures in data through algorithms to predict invisible data. With the increasing interest in aesthetics in dentistry, zirconia has drawn lots of attention due to its superior biocompatibility, aesthetically pleasing, high corrosion resistance, good mechanical properties, and absence of reported allergic reactions. The evolution of AI and ML led to the development of novel approaches for the biomedical applications of zirconia in dental devices. AI techniques in zirconia-related research and clinical applications have attracted much attention due to their ability to analyze data and reveal correlations between complex phenomena. The AI applications in the field of zirconia science change according to the application direction of zirconia. Therefore, in this article, we focused on AI in biomedical applications of zirconia in dental devices and AI in zirconia-related applications in dentistry.
Digital technology is widely applied in dentistry, and it plays an essential role in many aspects, such as clinical treatment, laboratory procedures, student training, practice management, and dental research (1). Take clinical treatment, for example; the role of digitization in treatment included digital impressions, CAD/CAM, digital shade analysis, digital smile design, virtual articulators (2). Artificial intelligence (AI) is a technology coined in the 1950's, which is rapidly developed based on computer technology (3). As a branch of computer science, AI can perform tasks that customarily require human intelligence by building intelligent software or machines. With the development of artificial intelligence, modern machines can learn from experience to adapt to new requirements and perform tasks similar to humans (4).
AI technology has been used in many aspects of human society, including medicine, and it is also being used more and more widely in dentistry (Figure 1). Zirconia is an advanced ceramic material used in biomedical applications since the 1960's (5). Zirconia has drawn lots of attention in dentistry due to its excellent biocompatibility, aesthetically pleasing, high corrosion resistance, good mechanical properties, and absence of reported allergic reactions. In recent decades, AI techniques in zirconia-related research and clinical applications have attracted much attention due to their ability to analyze data and reveal correlations between complex phenomena. Therefore, dentists need to have a comprehensive understanding of artificial intelligence in the research of zirconia-related fields in order to use and study zirconia. In this paper, we summarize recent advances concerns about AI techniques in zirconia-related applications in dentistry.
AI application technology is improving dramatically in the dental field with the emergence of data calculation and the accumulation and analysis of many clinical patient data (4, 6). For instance, Hung et al. systematically investigated the literature related to the clinical application of AI in dentistry and maxillofacial radiology (7). The researchers summarized the latest and comprehensive information about the current diagnostic performance and diagnostic imaging of AI dental and maxillofacial radiology. Correspondingly, the rapid development and application of AI in stomatology will guide the development direction of dentistry to a certain extent. For dental professionals, it is essential to understand, learn and master AI, keep pace with the new era of medicine, and apply it to clinical practice and scientific research.
As a subfield of AI, Machine learning (ML) can learn from the intrinsic statistical patterns and structures in data through algorithms to predict invisible data (8). ML can learn and self-improve by using many data and then sum up experience and methods (9). ML is currently one of the fastest-growing technical fields. The development of AI and ML has also brought new opportunities and challenges to medicine, including dentistry. In many areas of dentistry, such as maxillofacial surgery, orthodontics, and prosthodontics, correct diagnosis is the basis of a proper treatment plan. The introduction of ML helps diagnose and predict diseases and compare their performance because ML can detect relationships between banking operation records and patterns in big data (10–13). Kositbowornchai et al. developed an artificial neural network for the diagnosis of vertical tooth fracture (14). Jung et al. constructed neural network machine learning models using a back-propagation algorithm to diagnose extractions to help orthodontists determine the treatment plan (15). There is no doubt that ML can assist the dentist and provide much convenience. It cannot be assumed that ML is capable of human-level performance. The development and application of AI and ML in dentistry are generally not intended to replace dentists but assist dentists in clinical diagnosis and treatment based on mathematical decisions and predictions.
With the development of technology, a new era of “artificial intelligence” is coming. The evolution of AI and ML led to the development of novel approaches for the biomedical applications of zirconia in dental devices. The AI applications in the field of zirconia science change according to the application direction of zirconia. This article will focus on AI in biomedical applications of zirconia in dental devices and AI in zirconia-related applications in dentistry.
Nowadays, with the increasing interest in aesthetics and attention to the cytotoxicity and allergic reactions of certain metals, researchers and dentists have been working to find a metal-free aesthetic restorative material (Figure 2) (16, 17). Zirconia can be utilized as the implant, abutment, post, core, crown (full and partial), bridges, inlay and onlay, veneer in the field of restorative dentistry (18–20). Previous clinical studies report that zirconia has an abrasive effect on dentition and leads to excessive wear of the tooth structure (21–23). Pjetursson et al. investigated the survival rates and technical, biologic, and aesthetic complication rates of implant—supported zirconia crowns and implant—supported metal—ceramic crowns systemically in 2018 (24). They reported that the 5-year sur-vival rate of zirconia-based implant-supported single crowns was 97.6% (95% CI: 94.3–99.0), and it showed a similar incidence of biological complications and fewer aesthetic problems.
Further, Zhang et al. reviewed the development history of current dental zirconia ceramics and try to improve the translucency of zirconia materials without excessively compromising their mechanical integrity (25). Besides, as a non-metallic biomaterial, zirconia can be used as a substitute for titanium implants, and its flexural strength and fracture toughness are better than other ceramics (26, 27). Hashim et al. reported that after 1 year of function, the survival rates of one- and two-piece zirconia implants reached 92% (95% CI: 87–95) (28).
As reported, monolithic zirconia without veneer has better fracture resistance than traditional zirconia and is expected to reduce the incidence of porcelain fracture in the posterior tooth area (29). Shen et al. performed a retrospective clinical study of 1–5 years which included 224 patients treated with 327 implants and restored with metal-ceramic or monolithic zirconia single crowns to evaluate the clinical properties of implant-supported monolithic zirconia prostheses (30). They studied the marginal bone level (MBL) by taking panoramic radiographs at different stages during the treatment and follow-up visit. The results show that the MBL changes for the monolithic zirconia group were 0.25 mm during the healing period and 0.43 mm during the prosthetic loading period. There are no significant differences (P > 0.5) compared to the metal-ceramic group, indicated that monolithic zirconia has a similar peri-implant bone resorption rate with metal-ceramic prostheses.
One recent retrospective study by Lerner et al. included 90 patients restored with 106 implant-supported monolithic zirconia crowns (MZCs) placed in the posterior area (31). They investigated the quality of interproximal contact points, occlusal contacts, and chromatic integration and evaluated the chromatic integration, survival, and success rates of MZCs. Their study captured the first optical impression using the CS 3600® intraoral scanner and modeled the individual zirconia abutment in CAD software (Valletta®, Exocad, Darmstadt, Germany). Noticeably, during the production of the zirconia crown, the researchers utilized a fully digital protocol that employing AI to automated margin line design and successfully fabricated MZCs cemented on customized hybrid abutments. As reported, the 3-year survival rate and success rate of the MZCs made via a complete digital workflow were 99.0 and 91.3%, respectively.
The lifespan of dental restorations is limited, and the longevity of dental restorations is essentially affected by the material used (31, 32). Zhang et al. lists the successive generations of commercial dental zirconia and summarizes their composition and mechanical properties (25). The first-generation 3Y-TZPs, included Lava Frame (3M ESPE), KaVo Everest ZH (KaVo Dental), Zenostar MO (Wieland Dental), contains 0.25 wt% alumina (Al2O3) sintering aid, and its bending strength exceeds 1 GPa in flexure. The next stage is monolithic zirconia, which was developed to comprehensively consider zirconia's mechanical property and aesthetics. This goal is achieved to reduce opacity with a higher yttria content to construct partially stabilized zirconias, 4Y- PSZ (4 mol%) or 5Y-PSZ (5 mol%), an increased amount of non-birefringent c-phase. The introduction of translucent zirconia brought advantages such as good mechanical properties, high strength, less tooth preparation, and minor antagonist wear (33). Therefore, many different brands and different specifications of zirconia materials are available for dentists and patients to choose from on the market. However, patients' treatments may vary according to the property of restorative material. It is difficult for them to choose the best and longest-lasting material.
Fortunately, AI plays a vital role in this situation. For instance, Aliaga et al. collected data from the notes, graphs, and radiographic information of restorative treatments performed by Dr. Vera and utilized AI to analyze the collected information to determine the most suitable material and then promote the development of the dental restoration (32). Besides, AI also could be used to predict the longevity of CAD/CAM crowns. Aliaga et al. developed a case-based reasoning (CBR) system to model and predict the longevity of tooth restorations. Another study conducted by Yamaguchi et al. used a similar approach, a convolution neural network (CNN) AI model, to predict CAD/CAM crowns (34). The authors collected a total of 24 cases (50% of the crowns had debonding problems) and 8,640 two-dimensional images of the virtual tooth-prepared three-dimensional models. The results indicate that AI technology, the CNN method, shows superior performance in predicting the debonding possibility of CAD/CAM crowns.
In addition to the longevity and functional recovery of zirconia restorations, patients pay more attention to aesthetics when making choices. In aesthetic dentistry, shade reproducing and color matching play an essential role (35). Recently, various zirconia ceramics with different optical properties are commercially available. It is difficult for patients and dentists to choose the exact shade, appropriate material, and suitable configuration to make the restoration consistent with natural tooth color. A backpropagation neural network (BPNN) has already been utilized for computer color matching in the dental clinic (36). However, BPNN has disadvantages like unstable and low accuracy. Li et al. introduced a genetic algorithm (GA) to optimize initial weight and thresholds in BPNN to enhance the matching accuracy. The experiment results demonstrate that the proposed method plays a positive role in improving the precision and predictability of color matching in selecting restoration materials. Also, AI was used to predict the color of the teeth after the tooth bleaching procedure. Thanathornwong et al. developed a clinical decision support system with a regression model as the intelligent part. The results showed that this system could predict the color change using colorimetric values effectively (37).
In addition, zirconia abutments are recommended as an alternative to alloy abutments, providing better aesthetic results. A systematic review and meta-analysis studied by Vechiato-Filho et al. showed the 5-year success rate of zirconia abutments was 99.3% for fixed implant single crowns in posterior areas, which was no statistical difference compared to 99.57% for titanium abutments (P = 0.26) (38). Therefore, customized abutment made of zirconia is a suitable option in an implant-supported digital fixed prosthesis because it can obtain high aesthetics, superior emergence profile, and high compatibility with surrounded soft tissues. Generally, the custom abutment is designed by computer-aided design (CAD) and then milled and sintered with zirconia (39). However, there is tolerance between zirconia abutment and boding base during the extraoral cementation, which may cause cementing errors (40). These errors, although very small, can cause positional problems for monolithic zirconia when delivering custom abutment and temporary restorations to patients (41). Fortunately, the problems mentioned above can be overcome using AI, which reduced errors the cost of prosthetic therapy (42, 43).
In addition to AI application in clinical aspects, many studies focused on applying AI in zirconia-related research (44). Arif et al. used a statistical and artificial neural network (ANN) approach to investigate the tribological performance of aluminum hybrid composites reinforced with nano zirconia (0, 3, 6, and 9 wt%). Artificial intelligence was effectively applied to study various control factors on hybrid composite wear behavior (45). Artificial muscle plays a vital role in accelerating the development of robotics, automation, and AI-embedded system. Zirconia shape-memory ceramics can significantly enhance shape-memory properties (8%) because of their sizeable free surface area and fewer grain boundaries. Therefore, Du et al. used AI to develop highly aligned shape-memory zirconia-based yarns and springs, which could be used as artificial muscles at elevated temperatures (46).
Furthermore, zirconia is a vital transition metal oxide and has been extensively used to develop high-performance computing devices. Wang et al. apply artificial intelligence to build a Behler–Parrinello Neural Network (BPNN), which can calculate energies and forces of zirconia bulk structures. The BPNN has similar accuracy to density functional theory (DFT) calculations and can be applied in the molecular dynamics simulation of the oxygen vacancy diffusion (47).
At present, the application and research of AI in dentistry mainly include the following aspects, AI appointments, AI medical guidance, AI oral disease diagnosis, AI oral monitoring. Studies investigated the application of AI in digital dental radiography analysis, periodontitis, aesthetic dentistry, orthodontics, oral surgery, or future oral healthcare concepts in general (48). For instance, Li et al. applied AI techniques to diagnose gum inflammation (49). In their study, a deep neural network, Deeplabv3+ network with Xception and MobileNetV2, was used to diagnose patients as healthy, questionable healthy, questionable diseased, and diseased by pixel semantic segmentation of patient images. The results suggest that this system may be suitable for the self-examination of teeth using mobile apps.
Also, AI and ML play a more critical role in teaching, scientific research, oral health management, and oral disease management. Concerning biomedical applications of zirconia in dental devices, AI and ML have shown an irreplaceable role in zirconia material selection, colorimetry, scheme design, guide plate production, tooth preparation, customization of aesthetic standards, teaching, and scientific research. At present, artificial intelligence and machine learning technology have changed from the fantasy of the past to today's reality. Researchers in various fields conducted in-depth discussions on its impact on society, the economy, healthcare, and politics. The dental field is also one of them. It is believed that artificial intelligence will significantly promote dentistry's progress and development in the future.
The production of zirconia restorations requires a series of steps. Preparing a tooth for crowns and bridges is daily work for a dentist. Even the dentist is experienced, it is still challenging work (50). The main challenge is how to ensure that there is enough space for the restoration with the least amount of tooth preparation. The idea of using a robotic arm to assist dentists in preparing teeth is attractive and wise. The mechatronics system that can assists dentists in tooth preparation has been tested in vitro and has shown encouraging results.
Ortiz Simon et al. developed the first electromechanical system to assist dentists in handling the dental drill (51). The robotic arm can help the dentist operate the tool smoothly and precisely during preparing teeth. The mechatronic system can minimize handshakes caused by fatigue and reduces the risk of iatrogenic dental injuries. Because the mechatronic system can provide support and stability when handling dental drills, the dentist's position accuracy was improved by 53% using this mechatronic system. Precision medicine is the consensus and direction of the international medical community. Therefore, the robot's high precision, dexterity, and speed can finally overcome the shortcomings of manual operation and improve the accuracy and efficiency of clinical operation (52). In order to overcome the limitations of traditional manual methods, Yuan et al. developed a robotic oral tooth preparation system to improve its quality, precision, and clinical efficacy (53).
Wang et al. introduce a micro-robotic device called LaserBot, which can handle decayed teeth by manipulating femtosecond laser beams to complete tooth preparation (54). Experiment results show that the laser beam point's movement range and resolution can meet typical dental operations requirements. Researchers performed ablation experiments on wax-resin material and tooth to confirm femtosecond laser can be applied for tooth preparation. The developed robotic device realizes the precise 3D motion control of the laser focus and is small enough to be applied in the oral cavity. Li et al. proposed a novel robotic manipulator system specifically for dental applications equipped with a tendon sheath transmission system. The electric-motor actuators of this robot can be placed away from the manipulator. The proposed dental robot system with soft bracer has a compact size, excellent tool interchangeability, and fully customized dental surgery specifications. Thus, it can be applied in different fields, like caries removal and crown treatment (55).
Moreover, other intelligent dental equipment for tooth preparation has been tested for accuracy. For instance, a system with a micro-robot that controls a picosecond laser has shown good preparation accuracy to meet the clinical requirement (53). Otani et al. evaluated the accuracy and precision of an automated robotic tooth preparation system for porcelain laminate veneers and took traditional freehand tooth preparation as a control group (56). The results indicated that the automated robotic group has better accuracy and precision at the finish line. Besides, another tooth preparation system for veneers, a robotic arm with a rotating diamond instrument, was developed and compared to freehand tooth preparation. The results show that the accuracy of this AI system is better than the dentist (57). The average repeatability of this AI system is ~40 μm. However, the tooth preparation robot has not been validated well in a clinical setting. Furthermore, when talking about safety, the current safety strategy of this robotic tooth preparation system is not yet complete, and it is unable to achieve visualization and intelligent control. Therefore, further research should be focused on those limitations.
Artificial intelligence is mainly used in dental restorations to obtain digital impressions of the patient's teeth and soft and hard tissues, obtain colorimetry similar to natural teeth, and use artificial intelligence to design restorations to generate readable and transmitted three-dimensional data (58). The dental prostheses were obtained under computer-aided manufacturing. At present, newer research uses tooth preparation robots with reasonable intelligence and accuracy, which have become the development direction of digital dental prostheses. CAD/CAM-based systems are used in the dental field to make high-precision restorations (Figure 3). In addition, AI-based systems are applied to design and manufacture inlays, inlays, crowns, and bridges (59). These systems replace the traditional method of making restorations, which can shorten the production time and reduce possible errors.
Currently, dentists have higher requirements for the convenience of operation. The patients become more demanding in terms of aesthetics and hope to shorten the time for consultation in the dental clinic. In modern dentistry, CAD/CAM technology has become an indispensable method for manufacturing zirconia restorations, and digital intraoral impressions technology is also considered an efficient impression technique (60, 61). Gao et al. launched an in-vitro comparison to evaluate the accuracy of digital scanning to prepare complete-arch. According to their research work, the precision of impression scanning is better than intraoral scanning and cast scanning for a completely prepared arch (62). Besides, Oh et al. evaluate the accuracy of three digitization methods, direct scanning, cast scanning, and impression scanning, for the maxillary dental arch (63). Their findings also indicate that impression scanning is a better choice for constructing a virtual dental model.
Digital information and communication technologies are increasingly applied in the dental field (6, 59). The computer is an effective means for interactive communication, which plays a crucial role in the dental clinic and dental laboratories (64, 65). Parmar et al. conducted a large-scale online survey with 588 patients and 532 dentists to evaluate the attitude of patients and dentists on social media usage (Facebook) and their online behaviors in the current context (66). They studied the potential challenges and opportunities for the dentist to adopt social media practices. They evaluated the attitudes, thoughts, and social media behaviors from the perspective of patients and dentists. The results indicate that the more active dentist on social media, the more interaction, and participation of patients can be increased. That is, when patients have bad teeth that need to be repaired or missing teeth need to be restored, they can communicate more conveniently and effectively with dentists through social media. Correspondingly, dentists can also utilize social media to communicate with patients and use CAD/CAM technology to make restorations (67), making the dentist's clinical work more convenient and efficient.
In the future, AI and ML will automate many steps during the aesthetic treatment process, such as aesthetic evaluation, digital smile design, and treatment process. The development and application of AI technology provide patients with patient-centered, natural-looking, customized dental care services consistent with patients' own facial and other characteristics (68).
Dental laboratories also utilize AI technology, which can learn from millions of cases to make better designs for prosthetics in restoration design software (69). For instance, AI technology can be given an image training data set, where one network focuses on synthesizing a new image, while the other network tries to distinguish which images are fake or real. This technology can make restorations that are the same as the patient's natural anatomy. For older patients, library-based systems tend to build more detailed anatomical structures similar to surrounding dentitions that wear out during utilization. The design generated by GAN software replicates the natural wear patterns and appropriate levels of detail that present during the use of dentures.
Tsoi et al. adopt a new method that uses AI technology to directly measure the initial bacterial adhesion on a scanning electron microscope (SEM) image (70). In their work, Porphyromonas gingivalis (P.g.) and Fusobacterium nucleatum (F.n.) were cultured on the surface of zirconia specimens individually. Then the initial bacterial attachment was studied at different time points by taking three random areas of the SEM image of the bacterial attachment surface on zirconia specimens. The research results show that direct SEM image analysis using AI technology can be used for morphological and quantitative analysis of initial bacterial adhesion on the surface of zirconia.
As an essential ion-conducting material, yttria-stabilized zirconia (YSZ) is widely applied in solid oxide fuel cells, oxygen sensors, and solid oxide electrolysis cells. Guan et al. evaluated the microstructure of YSZ under different Y concentrations through utilizing the recently developed ML-based PES exploration technique, namely stochastic surface walking global exploration with global neural network potential (71). They create the first Y–Zr–O ternary global neural network (G-NN) potential, construct the thermodynamic convex hull diagram for YSZ with different Y concentrations, and determine the most stable phase atom at each Y concentration, the so-called global minimum (GM). They demonstrated that 8 YSZ is a cubic crystal phase YSZ with the lowest Y concentrations, and O anion diffusion in YSZ is affected by O vacancies and moves along the (100) direction. Their results show that the G-NN has excellent potential in solving different problems in materials science, especially for those who need an in-depth understanding of complex PES. Besides, in the past 50 years, the temperature and composition dependence of YSZ ion conductivity has been intensively studied. Guan et al. used their recently developed Y–Zr–O G-NN potential. They performed a series of long-term molecular dynamics simulations on YSZ with different concentrations (6.7, 8, 10, and 14.3 mol%) in a specific wide temperature range (800–2,000 K) (72). Their work finally solved the influence of temperature and composition on YSZ ion conductivity. Further, the results show that the application of ML in G-NN potential can achieve the high precision and speed requirements for material simulation.
However, the current research on AI and ML still has many limitations, which affect their promotion and application in dentistry (73). Schwendicke et al. established a consented checklist related to the planning, conducting, and reporting of AI research to overcome those limitations and got support from 27 team members through the e-Delphi process (74). According to their suggestion, this checklist can overcome those shortcomings, an advance AI research, and promote the development of standards in the dental field.
In summary, zirconia has drawn lots of attention in dentistry due to its excellent biocompatibility, pleasing aesthetics, high corrosion resistance, and the absence of allergic reactions. Dentistry is moving forward toward a new era under the promotion of artificial intelligence technology. The evolution of AI and ML led to the development of novel approaches for the biomedical applications of zirconia in dental devices. Therefore, a clear understanding of AI technology and concepts will be an advantage in the coming future. We sincerely hope that AI will be applied entirely to various fields of dentistry soon.
All authors listed have made a substantial, direct and intellectual contribution to the work, and approved it for publication.
This study was supported by the CSA Clinical Research Foundation for Young Scholars-All Ceramic Material Research Project (CSA-P2019-01).
The authors declare that the research was conducted in the absence of any commercial or financial relationships that could be construed as a potential conflict of interest.
All claims expressed in this article are solely those of the authors and do not necessarily represent those of their affiliated organizations, or those of the publisher, the editors and the reviewers. Any product that may be evaluated in this article, or claim that may be made by its manufacturer, is not guaranteed or endorsed by the publisher.
1. Joda T, Ferrari M, Gallucci GO, Wittneben J-G, Brägger U, et al. Digital technology in fixed implant prosthodontics. Periodontology. (2017) 73:178–92. doi: 10.1111/prd.12164
2. Jain P, Wynne C. Artificial intelligence and big data in dentistry. Digitizat Dentistry Clin Appl. (2021) 2021:1–28. doi: 10.1007/978-3-030-65169-5_1
3. Helm JM, Swiergosz AM, Haeberle HS, Karnuta JM, Schaffer JL, Krebs VE, et al. Machine learning and artificial intelligence: definitions, applications, and future directions. Curr Rev Musculoskelet Med. (2020) 13:69–76. doi: 10.1007/s12178-020-09600-8
4. Deshmukh SV. Artificial intelligence in dentistry. J Int Clin Dental Res Org. (2018) 10:47 doi: 10.4103/jicdro.jicdro_17_18
5. Chen YW, Moussi J, Drury JL, Wataha JC. Zirconia in biomedical applications. Expert Rev Med Devic. (2016) 13:945–63. doi: 10.1080/17434440.2016.1230017
6. Chwendicke F, Samek W, Krois J. Artificial intelligence in dentistry: chances and challenges. J Dental Res. (2020) 99:769–74. doi: 10.1177/0022034520915714
7. Hung K, Montalvao C, Tanaka R, Kawai T, Bornstein MM. The use and performance of artificial intelligence applications in dental and maxillofacial radiology: a systematic review. Dentomaxillofacial Radiol. (2020) 49:20190107 doi: 10.1259/dmfr.20190107
8. Gilvary C, Madhukar N, Elkhader J, Elemento O. The missing pieces of artificial intelligence in medicine. Trends Pharmacol Sci. (2019) 40:555–64. doi: 10.1016/j.tips.2019.06.001
9. Ignatyev VI, Privalov AV. Artificial intelligence as the technosubject of hybrid society. Human Soc Sci. (2019) 2019:47–51. doi: 10.2991/hssnpp-19.2019.9
10. Sarkar K, Saha S, Basu S. A review on artificial intelligence technologies. Int J Emerg Trends Electr Commun Eng. (2018) 2.
11. González-Carrasco I, Jiménez-Márquez JL, López-Cuadrado JL, Ruiz-Mezcua B. Automatic detection of relationships between banking operations using machine learning. Inform Sci. (2019) 485:319–46. doi: 10.1016/j.ins.2019.02.030
12. Yang L, MacEachren AM, Mitra P, Onorati T. Visually-enabled active deep learning for (geo) text and image classification: a review. ISPRS Int J Geo Inform. (2018) 7:65. doi: 10.3390/ijgi7020065
13. Mao WB, Lyu JY, Vaishnani DK, Lyu YM, Gong W, Xue XL, et al. Application of artificial neural networks in detection and diagnosis of gastrointestinal and liver tumors. World J Clin Cases. (2020) 8:3971. doi: 10.12998/wjcc.v8.i18.3971
14. Kositbowornchai S, Plermkamon S, Tangkosol T. Performance of an artificial neural network for vertical root fracture detection: an ex vivo study. Dental Traumatol. (2013) 29:151–5. doi: 10.1111/j.1600-9657.2012.01148.x
15. Jung SK, Kim TW. New approach for the diagnosis of extractions with neural network machine learning. Am J Orthodont Dentofac Orthoped. (2016) 149:127–33. doi: 10.1016/j.ajodo.2015.07.030
16. Smitha AJ, Savitha PN. Shade matching in aesthetic dentistry–from past to recent advances. J Dent Oral Care Med. (2017) 3:102. doi: 10.15744/2454-3276.3.102
17. Omar D, Duarte C. The application of parameters for comprehensive smile aesthetics by digital smile design programs: a review of literature. Saudi Dental J. (2018) 30:7–12. doi: 10.1016/j.sdentj.2017.09.001
18. Alfawaz Y. Zirconia crown as single unit tooth restoration: a literature review. J Contemp Dent Pract. (2016) 17:418–22. doi: 10.5005/jp-journals-10024-1865
19. Hanawa T. Zirconia versus titanium in dentistry: a review. Dental Mater J. (2020) 39:24–36. doi: 10.4012/dmj.2019-172
20. Vitti RP, Catelan A, Amaral M, Pacheco RR. Zirconium in dentistry. Adv Dental Biomater. (2019) 2019:317–45. doi: 10.1016/B978-0-08-102476-8.00014-1
21. Stober T, Bermejo JL, Rammelsberg P, Schmitter M. Enamel wear caused by monolithic zirconia crowns after 6 months of clinical use. J Oral Rehabil. (2014) 41:314–22. doi: 10.1111/joor.12139
22. Mundhe K, Jain V, Pruthi G, Shah N. Clinical study to evaluate the wear of natural enamel antagonist to zirconia and metal ceramic crowns. J Prosthetic Dentistry. (2015) 114:358–63. doi: 10.1016/j.prosdent.2015.03.001
23. Fathy SM, Swain MV. In-vitro wear of natural tooth surface opposed with zirconia reinforced lithium silicate glass ceramic after accelerated ageing. Dental Mater. (2018) 34:551–9. doi: 10.1016/j.dental.2017.12.010
24. Pjetursson BE, Valente NA, Strasding M, Zwahlen M, Liu S, Sailer I. A systematic review of the survival and complication rates of zirconia-ceramic and metal-ceramic single crowns. Clin Oral Implant Res. (2018) 29:199–214. doi: 10.1111/clr.13306
25. Zhang Y, Lawn BR. Novel zirconia materials in dentistry. J Dental Res. (2018) 97:140–7. doi: 10.1177/0022034517737483
26. Botelho MG, Dangay S, Shih K, Lam WYH. The effect of surface treatments on dental zirconia: an analysis of biaxial flexural strength, surface roughness and phase transformation. J Dentistry. (2018) 75:65–73. doi: 10.1016/j.jdent.2018.05.016
27. Wang Y, Lam WYH, Luk HWK, Øilo M, Shih K, Botelho MG. The adverse effects of tungsten carbide grinding on the strength of dental zirconia. Dental Mater. (2020) 36:560–9. doi: 10.1016/j.dental.2020.02.002
28. Hashim D, Cionca N, Courvoisier DS, Mombelli A. A systematic review of the clinical survival of zirconia implants. Clin Oral Investig. (2016) 20:1403–17. doi: 10.1007/s00784-016-1853-9
29. Porojan L, Topală F, Porojan S. Assessment of all-ceramic dental restorations behavior by development of simulation-based experimental methods. Insights Various Aspects Oral Health. (2017) 2017:173. doi: 10.5772/intechopen.69162
30. Shen X, Li J, Luo X, Feng Y, Gai L-T, He F-M. Peri-implant marginal bone changes with implant-supported metal-ceramic or monolithic zirconia single crowns: a retrospective clinical study of 1 to 5 years. J Prosthetic Dentistry. (2021) 12:10. doi: 10.1016/j.prosdent.2020.12.010
31. Lerner H, Mouhyi J, Admakin O, Mangano F. Artificial intelligence in fixed implant prosthodontics: a retrospective study of 106 implant-supported monolithic zirconia crowns inserted in the posterior jaws of 90 patients. BMC Oral Health. (2020) 20:1–16. doi: 10.1186/s12903-020-1062-4
32. Aliaga IJ, Vera V, De JF, García AE, Mohamad MS. Modelling the longevity of dental restorations by means of a CBR System. BioMed Res Int. (2015) 2015:540306. doi: 10.1155/2015/540306
33. Ghodsi S, Jafarian Z. A review on translucent zirconia. Eur J Prosthodont Restorat Dentistry. (2018) 26:62–74. doi: 10.1922/EJPRD_01759Ghodsi13
34. Yamaguchi S, Lee C, Karaer O, Ban S, Mine A, Imazato S. Predicting the debonding of CAD/CAM composite resin crowns with AI. J Dental Res. (2019) 98:1234–8. doi: 10.1177/0022034519867641
35. Tabatabaian F. Color in zirconia-based restorations and related factors: a literature review. J Prosthodont. (2018) 27:201–11. doi: 10.1111/jopr.12740
36. Li H, Lai L, Chen L, Lu C, Cai Q. The prediction in computer color matching of dentistry based on GA+ BP neural network. Computat Math Methods Med. (2015) 2015:816719. doi: 10.1155/2015/816719
37. Thanathornwong B, Suebnukarn S, Ouivirach K. Decision support system for predicting color change after tooth whitening. Comput Methods Progr Biomed. (2016) 125:88–93. doi: 10.1016/j.cmpb.2015.11.004
38. Vechiato-Filho AJ, Pesqueira AA, De Souza GM, dos Santos DM, Pellizzer EP, Goiato MC. Are zirconia implant abutments safe and predictable in posterior regions? a systematic review and meta-analysis. Int J Prosthodont. (2016) 29:233–44. doi: 10.11607/ijp.4349
39. Schepke U, Gresnigt MMM, Browne WR, Abdolahzadeh S, Nijkamp J, Cune MS. Phase transformation and fracture load of stock and CAD/CAM- customized zirconia abutments after 1 year of clinical function. Clin Oral Implants Res. (2019) 30:559–69. doi: 10.1111/clr.13442
40. Mangano F, Mangano C, Margiani B, Admakin O. Combining intraoral and face scans for the design and fabrication of computer-assisted design/ computer-assisted manufacturing (CAD/CAM) polyether-ether-ketone (PEEK) implant-supported bars for maxillary overdentures. Scanning. (2019) 2019:4274715. doi: 10.1155/2019/4274715
41. Mangano F, Margiani B, Admakin O. A novel full-digital protocol (SCAN- PLAN-MAKE-DONE®) for the design and fabrication of implant-supported monolithic translucent zirconia crowns cemented on customized hybrid abutments: a retrospective clinical study on 25 patients. Int J Environ Res Public Health. (2019) 16:ijerph16030317. doi: 10.3390/ijerph16030317
42. Park ME, Shin SY. Three-dimensional comparative study on the accuracy and reproducibility of dental casts fabricated by 3D printers. J Prosthetic Dentistry. (2018) 119:861 e1–7. doi: 10.1016/j.prosdent.2017.08.020
43. Kulkarni S, Seneviratne N, Baig MS, Khan AHA. Artificial intelligence in medicine: where are we now? Acad Radiol. (2020) 27:62–70. doi: 10.1016/j.acra.2019.10.001
44. Serra A, Fratello M, Cattelani L, Liampa I, Melagraki G, Kohonen P, et al. Transcriptomics in toxicogenomics, part III: data modelling for risk assessment. Nanomaterials. (2020) 10:708. doi: 10.3390/nano10040708
45. Arif S, Alam MT, Ansari AH, Bilal Naim Shaikh M, Arif Siddiqui M. Analysis of tribological behaviour of zirconia reinforced Al-SiC hybrid composites using statistical and artificial neural network technique. Mater Res Expr. (2018) 5:056506. doi: 10.1088/2053-1591/aabec8
46. Du Z, Zhou X, Ye P, Zeng X, Gan CL. Shape-memory actuation in aligned zirconia nanofibers for artificial muscle applications at elevated temperatures. ACS Appl Nano Mater. (2020) 3:2156–66. doi: 10.1021/acsanm.9b02073
47. Wang C, Tharval A, Kitchin JR. A density functional theory parameterised neural network model of zirconia. Mol Simul. (2018) 44:623–30. doi: 10.1080/08927022.2017.1420185
48. Chen Y, Stanley K, Att W. Artificial intelligence in dentistry: current applications and future perspectives. Quintessence Int. (2020) 51:248–57. doi: 10.3290/j.qi.a43952
49. Li GH, Hsung TC, Ling WK, Lam WY-H, Pelekos G, McGrath C. Automatic site-specific multiple level gum disease detection based on deep neural network. In: 15th International Symposium on Medical Information and Communication Technology (ISMICT). Xiamen: IEEE (2021) p. 201–5. doi: 10.1109/ISMICT51748.2021.9434936
50. Yu Z, Yu S, Han J. The processing of dental medical devices. Metal Biomater Process Med Device Manufact. (2020) 2020:341–55. doi: 10.1016/B978-0-08-102965-7.00010-2
51. Ortiz Simon JL, Martinez AM, Espinoza DL, Romero Velazquez JG. Mechatronic assistant system for dental drill handling. Int J Med Robot Comp Assist Surg. (2011) 7:22–6. doi: 10.1002/rcs.363
52. Grischke J, Johannsmeier L, Eich L, Griga L, Haddadin S. Dentronics: towards robotics and artificial intelligence in dentistry. Dental Mater. (2020) 36:765–78. doi: 10.1016/j.dental.2020.03.021
53. Yuan F, Lyu P. A preliminary study on a tooth preparation robot. Adv Appl Ceram. (2020) 119:332–7. doi: 10.1080/17436753.2019.1666555
54. Wang D, Wang L, Zhang Y, Lv P, Sun Y, Xiao J. Preliminary study on a miniature laser manipulation robotic device for tooth crown preparation. Int J Med Robot Comput Assist Surg. (2014) 10:482–94. doi: 10.1002/rcs.1560
55. Li J, Shen Z, Xu WYT, Lam WYH, Hsung RTC, Pow EHN, et al. A compact dental robotic system using soft bracing technique. IEEE Robot Automat Lett. (2019) 4:1271–8. doi: 10.1109/LRA.2019.2894864
56. Otani T, Raigrodski AJ, Mancl L, Kanuma I, Rosen J. In vitro evaluation of accuracy and precision of automated robotic tooth preparation system for porcelain laminate veneers. J Prosthetic Dentistry. (2015) 114:229–35. doi: 10.1016/j.prosdent.2015.02.021
57. Geminiani A, Abdel-Azim T, Ercoli C, Feng C, Meirelles L, Massironi D. Influence of oscillating and rotary cutting instruments with electric and turbine handpieces on tooth preparation surfaces. J Prosthetic Dentistry. (2014) 112:51–8. doi: 10.1016/j.prosdent.2014.02.007
58. Korzynski P, Haenlein M, Rautiainen M. Impression management techniques in crowdfunding: an analysis of Kickstarter videos using artificial intelligence. Eur Manag J. (in press). doi: 10.1016/j.emj.2021.01.001
59. Tandon D, Rajawat J. Present and future of artificial intelligence in dentistry. J Oral Biol Craniofac Res. (2020) 7:15. doi: 10.1016/j.jobcr.2020.07.015
60. Rutkunas V, Gečiauskaite A, Jegelevičius D, Vaitiekunas M. Accuracy of digital implant impressions with intraoral scanners. A systematic review. Eur J Oral Implantol. (2017) 10(Suppl.1):101–20. Available online at: https://www.for.org/sites/default/files/consensus/European-Journal-of-Oral-Implantology-vol-1-supplement-1-autumn-2017_FOR_Supplement.pdf#page=101
61. Hack G, Liberman L, Vach K, Tchorz JP, Kohal RJ, Patzelt SBM. Computerized optical impression making of edentulous jaws–an in vivo feasibility study. J Prosthodontic Res. (2020) 64:444–53. doi: 10.1016/j.jpor.2019.12.003
62. Gao H, Liu X, Liu M, Yang X, Tan J. Accuracy of three digital scanning methods for complete-arch tooth preparation: an in vitro.comparison. J Prosthetic Dentistry. (2021) 1:29. doi: 10.1016/j.prosdent.2021.01.029
63. Oh KC, Lee B, Park YB, Moon HS. Accuracy of three digitization methods for the dental arch with various tooth preparation designs: an in vitro study. J Prosthodontics. (2019) 28:195–201. doi: 10.1111/jopr.12998
64. Touati R, Richert R, Millet C, Farges JC, Sailer I, Ducret M. Comparison of two innovative strategies using augmented reality for communication in aesthetic dentistry: a pilot study. J Healthcare Eng. (2019) 2019:7019046. doi: 10.1155/2019/7019046
65. Rekow ED. Digital dentistry: the new state of the art—Is it disruptive or destructive? Dental Mater. (2020) 36:9–24. doi: 10.1016/j.dental.2019.08.103
66. Parmar N, Dong L, Eisingerich AB. Connecting with your dentist on facebook: patients' and dentists' attitudes towards social media usage in dentistry. J Med Internet Res. (2018) 20:e10109. doi: 10.2196/10109
67. Susic I, Travar M, Susic M. The application of CAD/CAM technology in dentistry. IOP Conf Ser Mater Sci Eng. (2017) 200:012020. doi: 10.1088/1757-899X/200/1/012020
68. Blatz MB, Chiche G, Bahat O, Roblee R, Coachman C, Heymann HO. Evolution of aesthetic dentistry. J Dental Res. (2019) 98:1294–304. doi: 10.1177/0022034519875450
69. Leeson D. The digital factory in both the modern dental lab and clinic. Dental Mater. (2020) 36:43–52. doi: 10.1016/j.dental.2019.10.010
70. Tsoi J, Ding H, Yang JY, Matinlinna J. Evaluation of initial bacterial adhesion on zirconia using artificial-intelligence. Dental Mater. (2018) 34:e122. doi: 10.1016/j.dental.2018.08.255
71. Guan SH, Shang C, Liu ZP. Resolving the temperature and composition dependence of ion conductivity for Yttria-stabilized zirconia from machine learning simulation. J Phys Chem C. (2020) 124:15085–93. doi: 10.1021/acs.jpcc.0c04331
72. Guan SH, Zhang KX, Shang C, Liu ZP. Stability and anion diffusion kinetics of Yttria-stabilized zirconia resolved from machine learning global potential energy surface exploration. J Chem Phys. (2020) 152:094703. doi: 10.1063/1.5142591
73. Shan T, Tay FR, Gu L. Application of artificial intelligence in dentistry. J Dental Res. (2021) 100:232–44. doi: 10.1177/0022034520969115
Keywords: artificial intelligence, machine learning, zirconia, esthetics, biomedical applications
Citation: Luo F, Hong G and Wan Q (2021) Artificial Intelligence in Biomedical Applications of Zirconia. Front. Dent. Med. 2:689288. doi: 10.3389/fdmed.2021.689288
Received: 31 March 2021; Accepted: 21 May 2021;
Published: 19 August 2021.
Edited by:
James K. H. Tsoi, The University of Hong Kong, Hong Kong, SAR ChinaReviewed by:
Walter Y. H. Lam, The University of Hong Kong, Hong Kong, SAR ChinaCopyright © 2021 Luo, Hong and Wan. This is an open-access article distributed under the terms of the Creative Commons Attribution License (CC BY). The use, distribution or reproduction in other forums is permitted, provided the original author(s) and the copyright owner(s) are credited and that the original publication in this journal is cited, in accordance with accepted academic practice. No use, distribution or reproduction is permitted which does not comply with these terms.
*Correspondence: Qianbing Wan, d2FucWlhbmJpbmdAMTI2LmNvbQ==
Disclaimer: All claims expressed in this article are solely those of the authors and do not necessarily represent those of their affiliated organizations, or those of the publisher, the editors and the reviewers. Any product that may be evaluated in this article or claim that may be made by its manufacturer is not guaranteed or endorsed by the publisher.
Research integrity at Frontiers
Learn more about the work of our research integrity team to safeguard the quality of each article we publish.