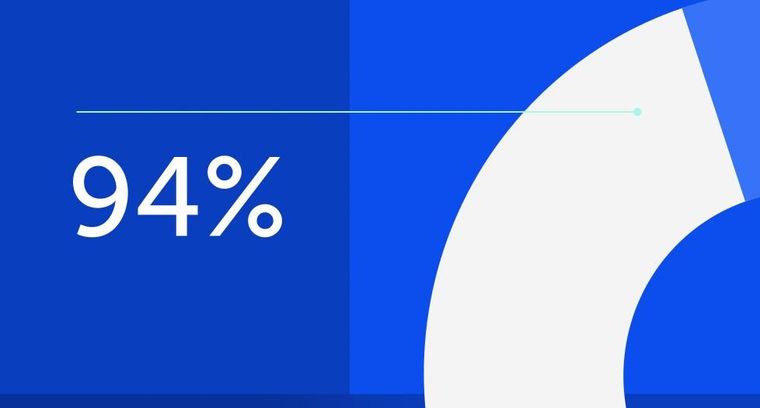
94% of researchers rate our articles as excellent or good
Learn more about the work of our research integrity team to safeguard the quality of each article we publish.
Find out more
EDITORIAL article
Front. Control Eng., 21 February 2024
Sec. Networked Control
Volume 5 - 2024 | https://doi.org/10.3389/fcteg.2024.1380005
This article is part of the Research TopicCooperative Control and Team Behaviors in Adversarial EnvironmentsView all 5 articles
Editorial on the Research Topic
Cooperative control and team behaviors in adversarial environments
Competitions against adversarial opponents often lead to complex and interesting cooperative behaviors exemplified by many biological collectives as well as strategies in team sports. Furthermore, as the deployment of a large number of robots becomes increasingly practical with current advances in technology, control and decision-making strategies for autonomous teams of agents against adversarial effects have become particularly important in various civilian and military applications. The complexity associated with the control and decision-making in multi-agent systems is further exacerbated when they must operate in adversarial environments.
To account for the complexity of the problem, the integration of diverse techniques at the intersection of several fields including multi-agent systems, game theory, machine learning, and control theory is imperative. This special topic highlights works that push the Frontier of this research domain at different levels: i.e., problem formulation, methodology, and/or applications. Specifically, this Research Topic includes two review articles discussing the frontiers in the field and the associated challenges, as well as two articles that push those frontiers in the context of specific multi-agent control problems in the presence of adversaries.
The mini-review article titled “Teaming behavior in adversarial scenarios” by Shishika et al. presents a broad overview of the types of teaming behavior that emerge in the presence of non-cooperative and/or competing teams of agents. From the viewpoint of a multi-agent task-allocation problem, the authors provide a categorization of existing works with a particular emphasis on two critical aspects: i) the extent of coupling or interdependence between the tasks; and ii) the dynamic and strategic nature of assignments that arise in the presence of an adversary. The authors propose a categorization of tasks, assignments, and the resultant team behavior that arise in various scenarios such as patrolling, routing, tracking, differential games (e.g., reach-avoid games), and resource allocation. Finally, the paper argues that one of the most important and challenging frontiers is the combination of first, incorporating specialized roles (heterogeneity) with strong coupling; and, second, achieving scalability in an adversarial setting that is not a one-sided optimization.
In the context of the aforementioned research Frontier, the article “Competitive perimeter defense with a turret and a mobile vehicle” by Bajaj et al. pushes on the aspect of heterogeneity. The paper studies the problem of intercepting incoming threats using two types of defenders: a turret that can neutralize the incoming threats by pointing to them, and a mobile vehicle that can intercept the threats by getting sufficiently close to them. The authors propose four classes of algorithms to coordinate the heterogeneous pair of agents, with associated performance guarantees in terms of the concept of competitive ratio. The results provide bounds on how well an online algorithm with local sensing performs in comparison to an offline one that has full knowledge of the timing and the location of the incoming threats, under the assumption of worst-case adversarial inputs.
Focusing more on a game-theoretic setting, the review paper titled “Multiplayer reach-avoid differential games with simple motions: A review”, by Yan et al. examines recent developments in multiplayer reach-avoid (M-RA) differential games. The review focuses on a particular variant of a pursuit-evasion game where the evader seeks to reach a target region without being intercepted by the pursuer, while the pursuer tries to prevent the evader from reaching the target. Although the solution to the one vs. one version of the problem has been known for some time now, the multi-agent (team vs. team) version is known to be challenging due to the curse of dimensionality, even with the simplified assumption of point mass, and holonomic agents. The authors discuss the existing approaches to mitigate this complexity and point out their limitations to identify directions for future research.
To address the scalability issue for multi-agent differential games, the article “Graph neural networks for decentralized multi-agent perimeter defense” by Lee et al. proposes a learning-based approach. In particular, the authors use an imitation learning framework with graph neural networks (GNNs) to learn how the defenders should be assigned to incoming attackers using only local perception and communication, whereas an expert policy relies on full state information. Essentially, scalability is achieved by learning a decentralized version of a centralized expert policy. Although heterogeneity was not considered in this work, the use of imitation learning to extend strategies built based on a game-theoretic approach seems to be a useful technique that applies to many other differential game settings.
The contributions in this Research Topic offer a broad perspective on the complexity of multi-agent systems operating in adversarial settings, showcasing various strategies and algorithms that address those challenges. These findings encourage further research in this direction and suggest several extensions to broader application fields.
DS: Writing–original draft. MD: Writing–review and editing. DM: Writing–original draft. JM: Writing–review and editing. PT: Writing–review and editing.
The authors declare that the research was conducted in the absence of any commercial or financial relationships that could be construed as a potential conflict of interest.
The author(s) declared that they were an editorial board member of Frontiers, at the time of submission. This had no impact on the peer review process and the final decision.
All claims expressed in this article are solely those of the authors and do not necessarily represent those of their affiliated organizations, or those of the publisher, the editors and the reviewers. Any product that may be evaluated in this article, or claim that may be made by its manufacturer, is not guaranteed or endorsed by the publisher.
Keywords: multi-agent systems, cooperative control, game theory, task assignment, resource allocation, perimeter defense, graph neural network
Citation: Shishika D, Dorothy M, Macharet DG, Marden JR and Tsiotras P (2024) Editorial: Cooperative control and team behaviors in adversarial environments. Front. Control. Eng. 5:1380005. doi: 10.3389/fcteg.2024.1380005
Received: 31 January 2024; Accepted: 06 February 2024;
Published: 21 February 2024.
Edited and reviewed by:
Jie Chen, City University of Hong Kong, Hong Kong SAR, ChinaCopyright © 2024 Shishika, Dorothy, Macharet, Marden and Tsiotras. This is an open-access article distributed under the terms of the Creative Commons Attribution License (CC BY). The use, distribution or reproduction in other forums is permitted, provided the original author(s) and the copyright owner(s) are credited and that the original publication in this journal is cited, in accordance with accepted academic practice. No use, distribution or reproduction is permitted which does not comply with these terms.
*Correspondence: Daigo Shishika, ZHNoaXNoaWtAZ211LmVkdQ==
Disclaimer: All claims expressed in this article are solely those of the authors and do not necessarily represent those of their affiliated organizations, or those of the publisher, the editors and the reviewers. Any product that may be evaluated in this article or claim that may be made by its manufacturer is not guaranteed or endorsed by the publisher.
Research integrity at Frontiers
Learn more about the work of our research integrity team to safeguard the quality of each article we publish.