- 1School of Natural Sciences, University of Hull, Hull, United Kingdom
- 2Institute of Science and Environment, University of Cumbria, Ambleside, United Kingdom
- 3Lake Ecosystems Group, UK Centre for Ecology and Hydrology, Lancaster Environment Centre, Bailrigg, Lancaster, United Kingdom
Introduction: Rewilding, the facilitation of self-sustaining and resilient ecosystems by restoring natural processes, is an increasingly popular conservation approach and potential solution to the biodiversity and climate crises. Outcomes of rewilding can be unpredictable, and monitoring is essential to determine whether ecosystems are recovering. Metabarcoding, particularly of environmental DNA (eDNA), is revolutionizing biodiversity monitoring and could play an important role in understanding the impacts of rewilding but has mostly been applied within aquatic systems.
Methods: This systematic review focuses on the applications of eDNA metabarcoding in terrestrial monitoring, with additional insights from metabarcoding of bulk and ingested DNA. We examine publication trends, choice of sampling substrate and focal taxa, and investigate how well metabarcoding performs compared to other monitoring methods (e.g. camera trapping).
Results: Terrestrial ecosystems represented a small proportion of total papers, with forests the most studied system, soil and water the most popular substrates, and vertebrates the most targeted taxa. Most studies focused on measuring species richness, and few included analyzes of functional diversity. Greater species richness was found when using multiple substrates, but few studies took this approach. Metabarcoding did not consistently outperform other methods in terms of the number of vertebrate taxa detected, and this was likely influenced by choice of marker, sampling substrate and habitat.
Discussion: Our findings indicate that metabarcoding, particularly of eDNA, has the potential to play a key role in the monitoring of terrestrial rewilding, but that further ground- truthing is needed to establish the most appropriate sampling and experimental pipelines for the target taxa and terrestrial system of interest.
Systematic Review Registration: https://osf.io/38w9q/?view_only=47fdab224a7a43d298eccbe578f1fcf0, identifier 38w9q.
Introduction
Rewilding has become an increasingly popular approach to the large-scale recovery of nature, with an aim to restore ecosystems to the point that they are self-sustaining and resilient, with increased trophic and functional complexity (Fernández et al., 2017; Carver et al., 2021; Pettorelli and Bullock, 2023). Rewilding can include species translocations, land abandonment (‘spontaneous rewilding’), and actions which actively kick-start ecological processes, followed by minimal intervention (Perino et al., 2019; Carver et al., 2021). These approaches can be applied across a range of ecological, spatial, temporal, and societal contexts, though are applied mainly to terrestrial ecosystems (Prior and Brady, 2017; Carver et al., 2021).
Literature surrounding rewilding is often disjointed, sometimes contradictory, frequently dominated by opinion pieces, and there is a lack of empirical, quantitative data and research (Pettorelli et al., 2018). A key goal of rewilding is to restore ecosystem processes and functioning, therefore monitoring strategies need to focus on the ecological integrity of whole ecosystems, in particular measuring trophic complexity, disturbance regimes and landscape connectivity (Torres et al., 2018). In comparison to more established restoration approaches, rewilding impacts can be unpredictable, forming potentially novel ecosystems over unknown timescales (Pettorelli and Bullock, 2023). For the purposes of this paper, we view rewilding and restoration as related but distinct conservation approaches. As both Mutillod et al. (2024) and Nelson (2022) note, whilst they may share the same ultimate goal, the ways to get there and the visions of what recovery looks like are different. While both seek to restore damaged ecosystems, rewilding generally focuses more on letting nature take its course once initial interventions are made. Rewilding also tends to lack historical benchmarks with which to measure a project’s success, unlike restoration (du Toit and Pettorelli, 2019). This inherent indeterminacy necessitates continuous monitoring strategies to understand impacts over long timescales, ideally with an adaptive management approach to help determine the level of any ongoing interventions or management decisions (Perino et al., 2019; Carver et al., 2021). Comprehensive and cost-effective monitoring methods, which maximize taxonomic coverage across different groups of organisms and can be applied at multiple spatial and temporal scales, are essential to understand whether rewilding practices are effective. Monitoring can also minimize any associated risks such as uncertainty around reintroductions, particularly for taxonomic substitutions of extinct native species, and uncertain timeframes, helping to enable the wider implementation of rewilding into legislation and policy (Pettorelli et al., 2018). However, questions remain regarding the best monitoring approach to cover a breadth of taxonomic diversity across temporal and spatial scales, and whether a holistic approach, integrating multiple methods may be most suitable in the context of rewilding.
In the last decade, biodiversity monitoring has experienced a molecular revolution, largely as a result of advances in high-throughput sequencing of PCR amplified gene regions (‘barcodes’) of interest across multiple taxa; a method known as ‘metabarcoding’ (Lawson Handley, 2015; Creer et al., 2016; Deiner et al., 2017; Takahashi et al., 2023). Metabarcoding has been applied to a number of mixed sample types, including bulk samples of invertebrates (often referred to as invertebrate “soup” e.g. Yu et al., 2012), ingested DNA from feces of predators or herbivores (sometimes described as ‘biodiversity capsules’ (Boyer et al., 2015; Nørgaard et al., 2021) or invertebrate-derived DNA (‘iDNA’, i.e. samples of blood meals of leeches or biting insects (Schnell et al., 2012, 2015; Abrams et al., 2019; Siegenthaler et al., 2019; Drinkwater et al., 2021a, 2021b). However, arguably the greatest sea change in metabarcoding of biodiversity over the last decade has been the analysis of environmental DNA or ‘eDNA’ (Taberlet et al., 2012; Creer et al., 2016; Deiner et al., 2017; Pawlowski et al., 2021; Takahashi et al., 2023), catalyzed by publication of a seminal paper describing how eDNA metabarcoding of seawater can effectively recover information on marine fish communities (Thomsen et al., 2012). Environmental DNA is DNA released by organisms into their environments via shed cells, mucus, gametes, and waste or decaying material, in addition to DNA from whole microbial and meiofaunal taxa present in environmental samples (Taberlet et al., 2012; Pawlowski et al., 2021). Although water and soil are the most common substrate types for sampling eDNA, eDNA metabarcoding studies have also been successfully performed on diverse sample substrates including snow (Kinoshita et al., 2019), air (Clare et al., 2022; Lynggaard et al., 2022) saltlicks and drinking water vessels (Ishige et al., 2017), spider webs (Gregorič et al., 2022), swabs and tree-roller samples (Allen et al., 2023). eDNA metabarcoding is particularly promising as a tool for non-invasive monitoring of rewilding across ecosystems and different spatial scales, as it has potential to generate whole-community datasets across the tree of life, estimate standard community and functional diversity metrics and perform trophic network analysis (Yan et al., 2018; Meyer et al., 2020; Blackman et al., 2022; Condachou et al., 2023; Hassan et al., 2023). To date though, there has been a much greater emphasis on eDNA metabarcoding for monitoring of aquatic than terrestrial ecosystems (van der Heyde et al., 2022).
Guidelines are needed for translating DNA metabarcoding into practice, and improving its uses in biodiversity monitoring (Blackman et al., 2024), particularly in terrestrial contexts (van der Heyde et al., 2022), which are generally more diverse and heterogeneous over smaller spatial scales than aquatic environments (Grosberg et al., 2012). Firstly, choice of sampling substrates can strongly influence the detection of different taxa (van der Heyde et al., 2020), but little guidance exists on the best substrate choice for different taxonomic groups, and it is unclear whether sampling multiple substrates is necessary or worth the increased effort and resources (van der Heyde et al., 2020, 2022). Secondly, a meta-analysis has demonstrated that eDNA metabarcoding often outperforms traditional methods in terms of cost, sensitivity and number of species detected (Fediajevaite et al., 2021), but this might reflect a bias towards aquatic systems and substrates, as well as a publication bias (failures may be less likely to be published; Beng and Corlett, 2020). The limited comparisons of metabarcoding and traditional methods in terrestrial settings so far indicate performance is mixed, both in terms of sensitivity and species detected (Fediajevaite et al., 2021), and questions therefore remain about the most suitable approach.
Here, we systematically review applications of metabarcoding to monitoring biodiversity in terrestrial ecosystems, focusing on eDNA, but also including relevant studies that have used bulk invertebrate or ingested DNA samples (i.e. fecal samples or iDNA). Our aim is to help inform how metabarcoding, in particular of eDNA, can be integrated into monitoring of terrestrial rewilding as well as other terrestrial conservation practices. We address the following research questions: 1) what are the trends in publications of terrestrial metabarcoding in relation to geographic location, target organisms, sample substrates used, and types of analyses performed to understand community or functional diversity?; 2) which sample substrate(s) are the most appropriate for the different target organisms and how does the sampling of multiple substrates affect the number of species detected and taxonomic coverage?; 3) how does metabarcoding perform when compared to traditional monitoring methods for terrestrial target taxa, and is this comparison dependent on choice of sampling substrate, the choice of target region and primer pairs for metabarcoding, and/or taxonomic focus?; and 4) what are the research gaps we need to fill for eDNA metabarcoding to be routinely used for effective monitoring of rewilding?
Materials and methods
Literature search
We performed a systematic review, using the Preferred Reporting Items for Systematic Reviews and Meta-Analyses (PRISMA) guidelines (https://www.prisma-statement.org/, Supplementary Figure S1). A literature search was conducted in Scopus and Web of Science to ensure the greatest coverage of journals relating to Natural Sciences (Mongeon and Paul-Hus, 2016) on 3rd January 2024. Initially, a search of the terms ‘eDNA OR Environmental DNA OR metabarcoding’ AND ‘rewild*’ was performed. This only returned 12 relevant studies, the majority of which focused on herbivore diet composition, indicating the so far limited application of DNA metabarcoding to monitor rewilding. To supplement these papers we performed a second, broader search to identify published scientific studies which used metabarcoding of environmental DNA to monitor terrestrial communities, with the aim that this would better show the potential applications for rewilding monitoring. This second search consisted of the following terms: ‘eDNA OR Environmental DNA OR metabarcoding’ AND ‘trophic OR function* OR network’ OR ‘monitor* OR survey*’. Results were then refined to include terrestrial studies only, using the addition ‘AND (terrestrial)’ term. The trophic OR function OR network search term was included to capture studies that have performed additional functional analyses beyond species richness or community comparisons.
Studies that sampled bulk DNA from invertebrates, were also included, as well as DNA from feces of predators or herbivores or iDNA. Fecal and iDNA samples were combined in the present study in the category ‘ingested’ DNA. Substrates that appeared less than three times in the database (e.g. spider webs, saltlicks, snow) were categorized as ‘Other’. Definitions of all substrates included are provided in Supplementary Table S1.
The automated refinement tools within Scopus and Web of Science were used to remove irrelevant studies (e.g. those from other disciplines such as Psychology, Physics etc.) and reviews or perspectives. After removing duplicates, this output was manually screened so that it included studies focusing on contemporary terrestrial ecosystems, at least in part (i.e. not wholly aquatic-focused, ancient DNA, or lab/desk-based studies where samples were not collected directly from the field). Of the remaining studies, further refinement removed papers which only used a single-species approach (quantitative PCR, droplet digital PCR etc.), leaving 164 papers analyzed in this review. This final refinement process enabled us to focus on papers with a community or ecosystem approach, which is important in the context of monitoring impacts of rewilding.
To understand the publication trends of these results in the context of the wider field of eDNA and DNA metabarcoding, we collected a list of papers from a search using only ‘eDNA OR Environmental DNA OR metabarcoding’ and a second search adding ‘AND soil’ from both Web of Science and Scopus. We were then able to look at the proportion of papers and overlap of those included in the current study compared to those with potentially more aquatic or below-ground focuses.
Data extraction and analysis
The following details were recorded from each publication: the study’s target taxa; primers used; substrates sampled; the ecosystem from which samples were taken; and analyses performed (see Supplementary Tables S1–S3 for definitions). Data analysis and visualization were undertaken using R version 4.3.1 (R Core Team, 2023) in RStudio version 2024.04.0 (Posit team, 2024), with the packages dplyr (Wickham et al., 2023a), tidyr (Wickham et al., 2023b) and tidyverse (Wickham et al., 2019) for data handling and ggplot2 (Wickham, 2016); hrbrthemes (Rudis, 2020) and viridis (Garnier et al., 2023) for visualization. To investigate publication trends (research question 1), a Sankey diagram was created using ggsankey (Sjoberg, 2023), and geographical trends plotted using ggplot2 (Wickham, 2016) and sf (Pebesma and Bivand, 2023) packages.
To investigate which substrate(s) are appropriate for chosen taxa and whether sampling multiple substrates improves taxonomic coverage (research question 2), information on taxonomic richness detected for each substrate was collected from articles which used multiple substrates for sampling. UpSet plots were created using UpSetR (Gehlenborg, 2019) to visualize the preference for multiple substrate and taxa combinations across studies. Pairwise comparisons for different substrate combinations were then analyzed to determine relative performance. The proportion of unique taxa detected only with one substrate, and the proportion of taxa shared between both substrates was calculated to allow comparisons across studies and visualized using stacked barcharts. Where a study sampled different sites that were treated separately in their own analyses, these were treated as independent comparisons. Similarly, where a study used more than two substrates, each pairwise comparison between substrates was treated independently. For each study, data was collected for the lowest taxonomic rank available across both methods so that data remained comparable.
A comparison of metabarcoding and traditional methods (research question 3) was carried out for vertebrates only, since just two studies compared metabarcoding with traditional methods from non-vertebrate taxa. Fifteen studies compared metabarcoding and traditional monitoring for detection of vertebrates. Our analysis focused on the influence of three variables: substrate choice, amplification/primer region, vertebrate focus. The data from these studies were expanded by treating discrete sites within each study separately, resulting in a dataset of 32 comparisons. We also treated individual substrates, primer regions or vertebrate focuses separately, providing a total of 33, 36 and 46 pairwise comparisons for analysis. Here ‘traditional’ monitoring methods, included camera traps or field surveys [e.g. line transects (Coutant et al., 2021), trapping (Mena et al., 2021) or vegetation surveys (Edwards et al., 2018)]. The proportion of unique taxa compared to total taxonomic richness was plotted for each comparison of metabarcoding vs traditional methods. Wilcoxon signed-rank tests were used to compare the median proportion of taxonomic richness between metabarcoding and traditional methods for the comparisons of different substrate, primer region and taxa in addition to overall comparisons of metabarcoding with cameras, and metabarcoding with field surveys.
Results
Database and publication trends
After refinement, 164 of the 299 studies identified were retained for analysis. The number of metabarcoding studies increased markedly between 2013 and 2023, from 54 to 962 (Figure 1A). The number of terrestrial metabarcoding studies has increased over time (Figure 1B) but they made up a small proportion (<20%) of the total, and this proportion has not increased over time (Figure 1A). Soil-focused studies ranged from 10-16% of all metabarcoding studies over the last 10 years (Figures 1A, B), while other terrestrial metabarcoding studies remained between 2-4% of the total. Of the studies included in our further analyses, 69% were based on eDNA metabarcoding, with the remaining 31% sampling ingested DNA or bulk invertebrates.
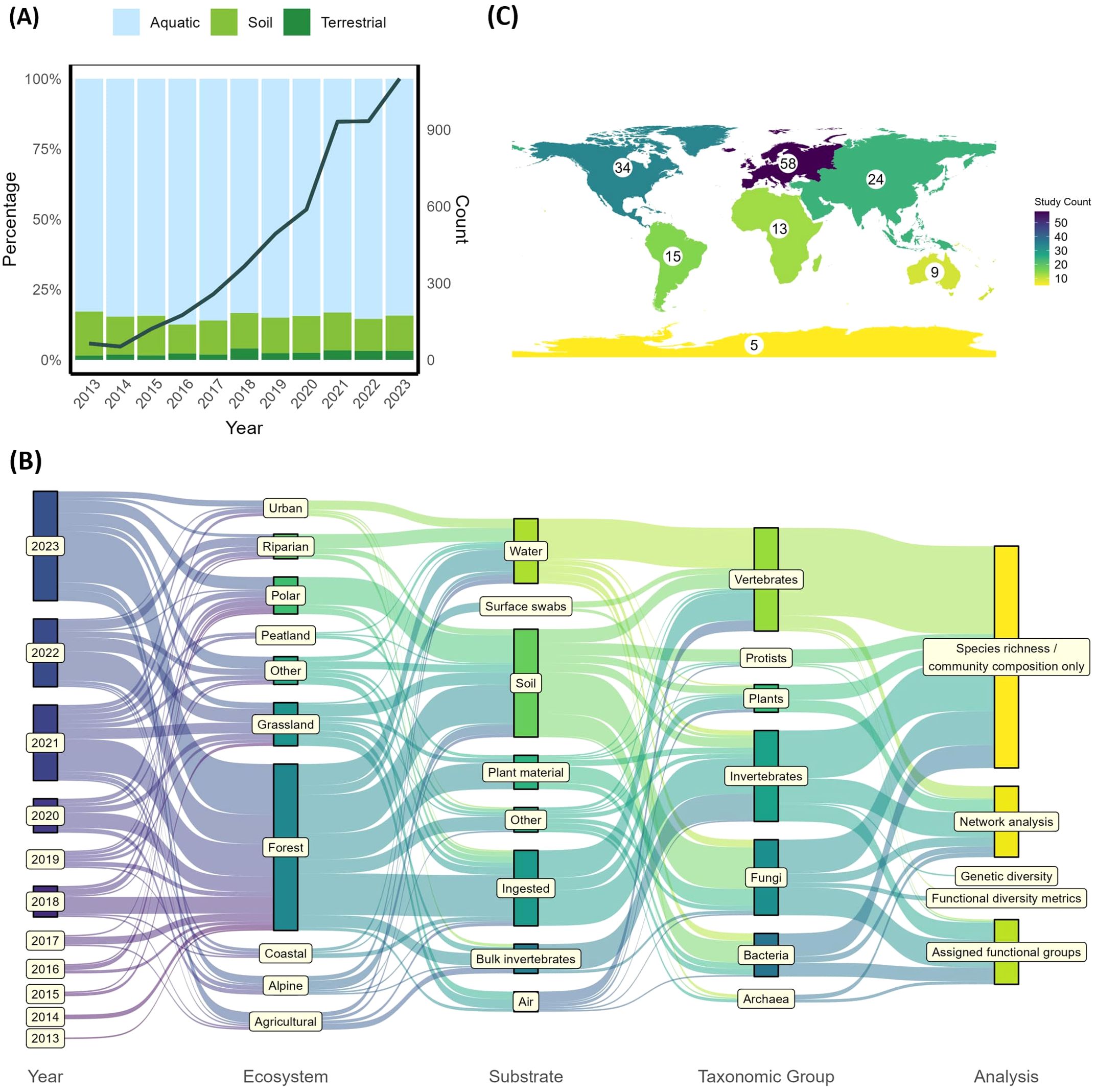
Figure 1. Publication trends: (A) the number of studies published each year and proportion for terrestrial-focused studies (those included in this review), soil-focused studies and all other eDNA focused-studies (aquatic) according to the Scopus and Web of Science outputs. (B) The number of studies by Continent – where samples were taken rather than research institutions. (C) Sankey diagram showing publication year, ecosystem sampled, substrate sampled, taxonomic focus and type of downstream analysis for each study. Where a study used multiple substrates or taxonomic groups, each substrate/taxa was counted independently. Substrates with < 3 occurrences were assigned ‘Other’. Where a study had multiple focuses per category (e.g. 2 different substrates), these were treated independently to show all interactions.
Terrestrial metabarcoding studies have been conducted on all continents, but with a bias towards Europe (n= 58 studies) and North America (n=34 studies) (Figure 1C). By country, most studies have been conducted in the USA (n=19), Denmark (n=13), China (n=9 and Germany (n=8) while no studies have been conducted in most African countries or in the Middle East (Supplementary Figure S2). Studies spanned diverse ecosystems, including urban, riparian, polar, peatland, grassland, coastal, alpine and agricultural habitats, but the most targeted ecosystem was forests, with over one third of papers (66 studies) sampling only in forests or woodlands. Only 21 studies (13%) sampled multiple ecosystems, and all these included forest ecosystems.
Soil, water and ingested material were the most common substrate choices for metabarcoding analysis in terrestrial ecosystems, with 34% of studies sampling soil (n=39 only soil, plus 16 using soil alongside other substrates), 24% sampling ingested material (n=39 only ingested material, plus one with other substrates, and 21% sampling water (n=27 only water, plus seven using water alongside other substrates), Figure 1B; Supplementary Figure S3A). Sampling of all substrates has increased over time, with the notable addition of air and surface swabs since 2022 (Supplementary Figure S3A). Only 10% of studies sampled multiple substrates (n=17, Figure 2A), and 5 of these studies were in 2023. Of these studies, six (35%) sampled both water and soil, four (24%) sampled soil and plant material, and two (12%) sampled soil and surface swabs (Figure 2A). The maximum number of substrates was four (soil, ingested material, plant material and bulk invertebrates), sampled in a single study (Figure 2A).
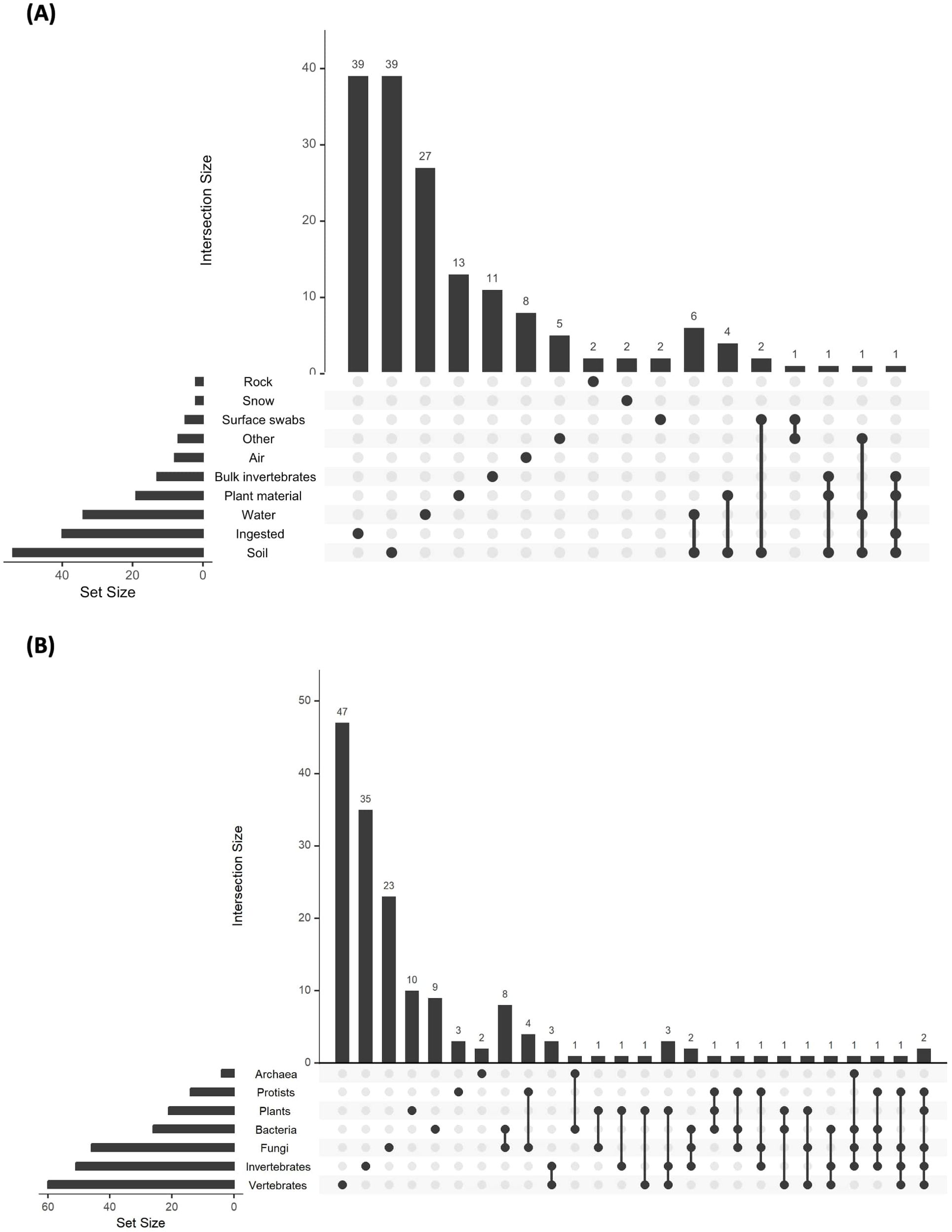
Figure 2. Upset plots showing the combinations of (A) multiple substrates and (B) multiple taxonomic focuses across studies. Intersection size represents the number of studies which focused on the combination represented below each bar. Set size indicates the total number of studies which included a focus on the corresponding substrate or taxonomic group to the right of each bar.
Vertebrates, invertebrates and fungi were frequently studied, with vertebrates studied in 37% of studies (n=47 vertebrate only, plus 13 with a multi-taxa focus), invertebrates in 33% (n=35 single plus 16 multi-taxa) and fungi in 28% (n=23 single, plus 23 multi-taxa, Figures 1C, 2B). Only ten studies focused just on plants, though a further 11 included plants as part of broader taxonomic surveys. Overall, 21% of studies (n=35) had a multi-taxa focus, with eight of those studies carried out between 2022-2023. Across these 35 studies there were 19 different taxonomic combinations, (Figure 2B), with no clear consensus over choice of combination.
The majority (57%) of studies investigated species richness and community composition only (n=93, Figure 1C). Twenty-four percent of studies carried out network analyses (n=32 one analysis type, plus n=8 multiple analyses), and a further 21% assigned functional groups (n=26 one analysis type, plus n=8 multiple) (Figure 1C). Three studies estimated functional diversity metrics, and just a single study on invertebrates investigated genetic diversity (Figure 1C; Supplementary Figure S3E). Network analyses were carried out on all taxa, though functional group assignment was mainly carried out for fungi, invertebrates, bacteria and archaea, rarely for vertebrates and protists, and not for plants (Supplementary Figure S3E). Three studies performed network analyses across multiple taxonomic groups, though just one included vertebrates, invertebrates and plants in this analysis.
Which substrate(s) are appropriate for chosen taxa and does sampling multiple different substrates improve taxonomic coverage?
Soil samples were used for analyzing all taxonomic groups while water samples targeted all taxa apart from protists, and plant material and air DNA targeted all taxa apart from archaea (Figure 1C; Supplementary Figure S3D). Surface swabs and ingested DNA were used to target invertebrates and vertebrates only. Invertebrates were targeted with all sample types. Vertebrates were targeted with all sample types apart from bulk samples (though iDNA from invertebrates was used, categorized under ‘ingested’), with water, ingested DNA, soil, and airDNA being popular (Figure 1C; Supplementary Figure S3D). Fungi and bacteria were sampled mainly via soil, but also commonly through plant material and water. There are few clear trends in terms of which substrates are targeted for certain taxa, apart from a slight trend for vertebrate studies to sample water and fungi studies to target soil (Figure 1C; Supplementary Figure S3D).
Seventeen studies (10%) sampled more than one type of substrate, with the most common substrates used together being soil and water (7 studies), and soil and plant material (4 studies; Figure 2A). Most studies that utilized more than one substrate for sampling found a greater species richness overall. All but five of the 29 comparisons found additional unique taxa with the addition of a second substrate (Figure 3). Soil performed poorly compared to bulk invertebrates, scats, water, plant material and roller swabs in terms of the number of unique taxa identified (Figure 3). For vertebrates, scat sampling performed best overall, with water and roller swabbing also outperforming soil (Figure 3). For invertebrates, scats, plant material and bulk invertebrates all outperformed soil, whereas bulk invertebrates also outperformed plant material and scats, and spray aggregations detected more unique taxa than roller swabs (Figure 3). For bacteria and fungi, there were more shared and fewer unique taxa overall, but slightly more unique taxa were detected with water compared to soil, and with soil compared to plant matter (Figure 3).
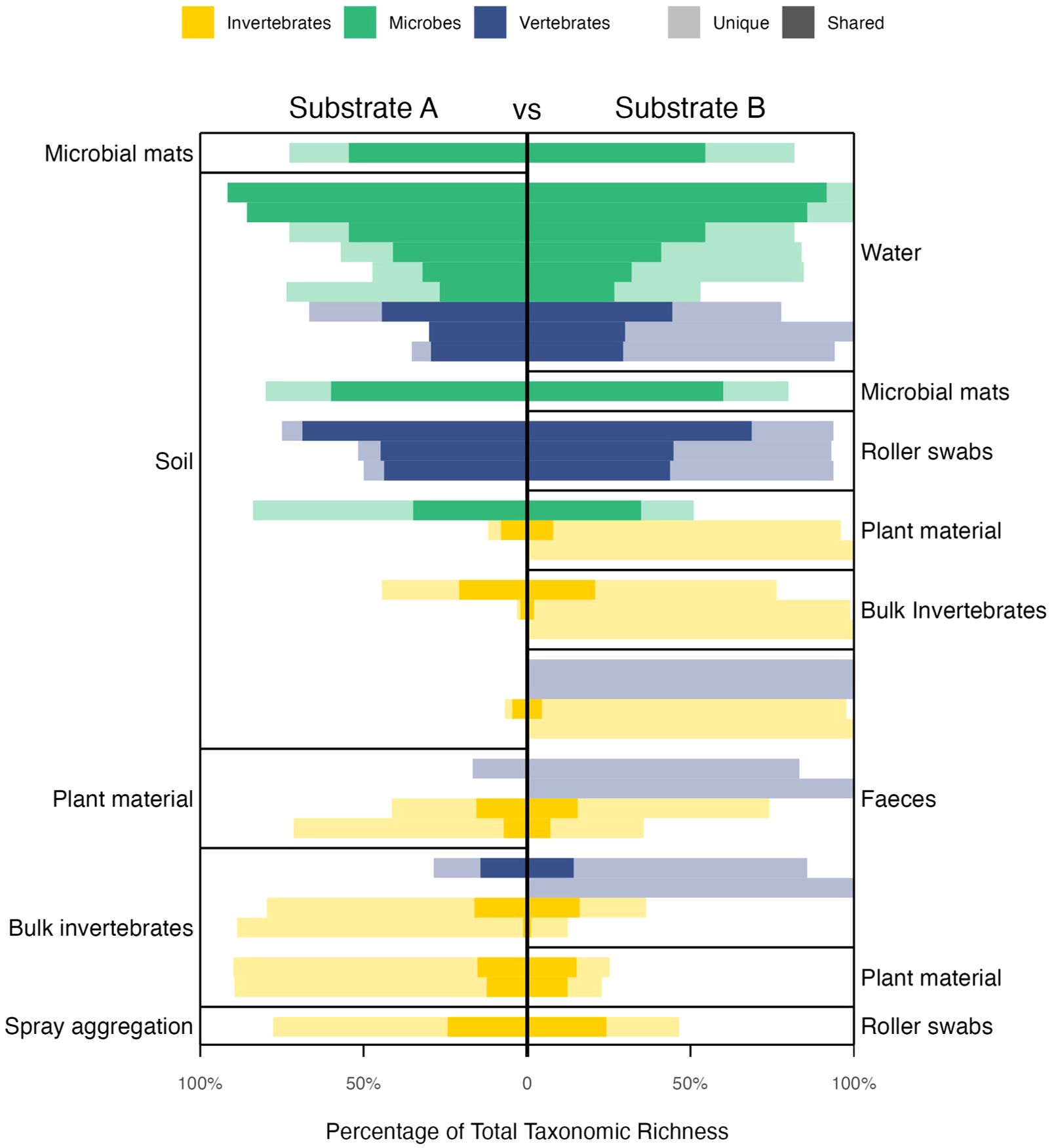
Figure 3. Pairwise comparisons of different eDNA substrates, showing the percentage of unique taxa found in each substrate (lighter shades) and the percentage of taxa shared by both substrates (darker shades). Percentages are of the total taxonomic richness when both substrates were combined within each study. Bars are colored according to the taxa detected.
How does metabarcoding perform when used alongside traditional methods for terrestrial vertebrate taxa?
Of the 33 comparisons of DNA-based and traditional monitoring, 13 found a greater proportion of vertebrate taxonomic richness (i.e. more unique taxa) with metabarcoding compared to the traditional method, 15 studies found higher taxonomic richness with the traditional method, and five studies found no clear difference (a difference in proportions of < 10%, Figure 4A). Three quarters of these comparisons were made between metabarcoding and cameras. Eight of these found a higher proportion of unique taxa with metabarcoding, 12 found a higher proportion with cameras, and four found similar proportions between the two. Of the eight comparisons between eDNA and field surveys, four found more unique taxa with eDNA, two found more unique taxa with field surveys, and one study found all the targeted taxa with both methods. No significant differences were found between the proportion of taxa uniquely detected with metabarcoding and either camera trapping or field surveys (Supplementary Table S4).
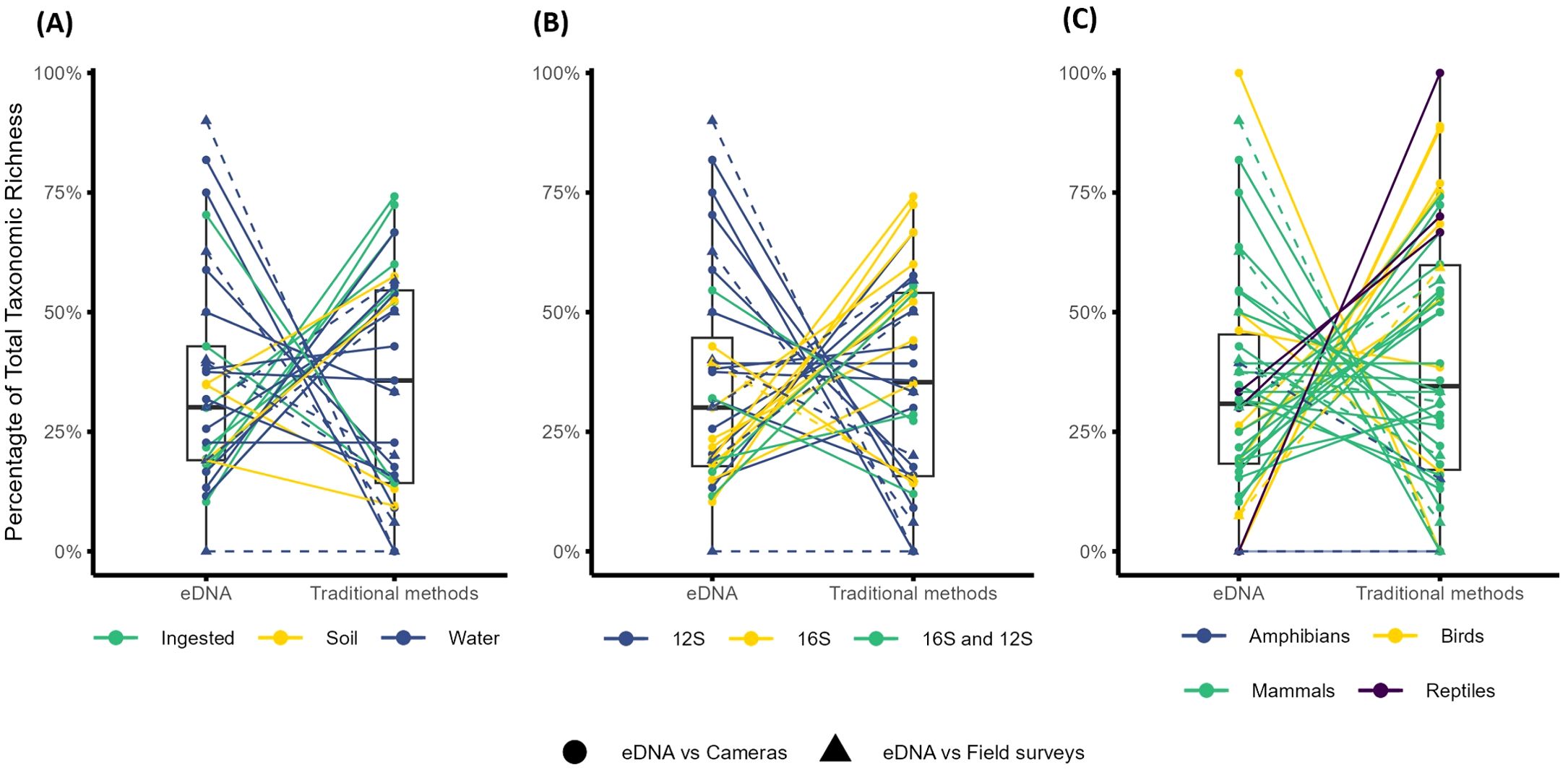
Figure 4. The proportion of unique taxa detected with either eDNA or traditional methods, compared to the total taxonomic richness recorded by both methods. Data are only from vertebrate-focused studies. Lines connecting the points indicate the comparable method for each study. Where the traditional method was camera traps, points are circles and lines are solid, other field surveys (e.g. field signs, line transects) have triangular points and dashed connecting lines. The remaining proportion of taxonomic richness which is not plotted is taxa shared across both methods. Where a study sampled multiple different sites, these were treated independently. (A) Studies and sites within studies, colored by eDNA sampling substrate (n=32). (B) Studies and sites within studies, colored by primer region, with additional data expansion for different amplification regions (n=35). (C) Studies and sites within studies colored by the vertebrate taxa detected, with additional expansion of data to separate different taxa (n=45).
Half of the comparisons between water-derived eDNA and traditional methods detected more unique taxa with eDNA, while five detected more unique taxa with traditional methods, though no statistical difference was found (Figure 4A; Supplementary Table S4). With iDNA and soil, two of nine studies and one of three studies detected more unique taxa with metabarcoding respectively (Figure 4A), though again, no statistical difference was detected (Supplementary Table S4).
Of the 20 comparisons that used only the 12S rRNA region for vertebrate metabarcoding, 10 detected more unique taxa with metabarcoding, six detected more unique taxa with traditional methods and four studies reported similar taxonomic richness (Figure 4B). By contrast, only two of the 11 comparisons using only 16S reported higher taxonomic richness with metabarcoding (Figure 4B), with this being the only statistically significant difference we detected across all comparisons (n=11, v=7, p=0.019). Five studies included both 12S and 16S, and of these only two comparisons reported higher taxonomic richness with metabarcoding (Figure 4B).
Thirty of 45 pairwise comparisons targeted mammals, and of these, 13 found a higher number of unique taxa with metabarcoding, 11 detected more taxa with traditional methods, and six found no difference (Figure 4C). Two of the 10 studies that detected birds found more unique taxa with metabarcoding, while seven detected more taxa with traditional methods. The two reptile studies both found higher taxonomic richness for traditional methods. Of the three amphibian studies, one found higher richness with eDNA and the others found all detected taxa with both methods (Figure 4C). Statistical tests showed no significant differences between these comparisons (Supplementary Table S4).
Discussion
Our systematic review demonstrated how terrestrial systems have been relatively neglected in relation to eDNA metabarcoding research overall. Sixty-nine percent of the 164 studies in our review were based on eDNA metabarcoding, reflecting research emphasis on eDNA as opposed to bulk samples or iDNA. We show that there are current biases towards research effort in the global north, forest habitats and single taxonomic groups (particularly vertebrates), soil as a substrate choice, and descriptive rather than functional analyses. Choice of sampling substrate is highly context-dependent for terrestrial ecosystems, something which has previously been suggested in more specific terrestrial habitats (Kestel et al., 2022; van der Heyde et al., 2022). We found that using multiple substrates in combination improves taxonomic coverage, though substrate choice should be informed by the target taxa. The recent review by van der Heyde et al. (2022) concluded that there is no ‘one size fits all’ approach to ecological monitoring with eDNA, and we draw similar conclusions, suggesting that multi-method ‘toolkit’ approaches, which integrate eDNA with established methods, could be most appropriate approach for future monitoring of terrestrial rewilding.
Publication trends
Although terrestrial metabarcoding studies have increased in number over time, they make up less than 20% of all eDNA metabarcoding studies, and this proportion has remained stable over the last decade, suggesting terrestrial research is lagging behind the field in terms of research effort. In contrast, aquatic eDNA research is maturing from a developmental-focus to more application-focused for ecological monitoring (Schenekar, 2023; Takahashi et al., 2023).
There is also a geographic bias in research coverage, with over 70% of terrestrial eDNA metabarcoding studies conducted in Europe, North America and Asia; a slightly higher proportion than the 61% reported in van der Heyde et al. (2022) which included both targeted and metabarcoding terrestrial studies. A northern hemisphere bias has also been reported for aquatic eDNA studies (DiBattista et al., 2022; Rishan et al., 2023; Takahashi et al., 2023), and highlights the need to promote and support eDNA research in the global south.
Terrestrial metabarcoding studies have been carried out in diverse ecosystems, but by far the greatest emphasis has been on forests and woodlands, with one third of all studies included here sampling these habitats; a similar trend to that noted previously (van der Heyde et al., 2022). This emphasis may be due to the global importance of forest habitats for biodiversity and ecosystem services such as carbon sequestration, as well as their economic importance (Brockerhoff et al., 2017; Coble et al., 2019).
Soil was by far the most sampled terrestrial substrate in our review, featuring in one third of included studies, similar to previous reviews (van der Heyde et al., 2022). Water and ingested material were also popular choices, while more novel methods such as airDNA metabarcoding and surface swabs are increasing in popularity. So far, the use of multiple substrates in terrestrial eDNA studies is limited, with only 11% of the papers combining two substrates and a single study combining four. Combining substrates obviously incurs additional time and resources, so a key question is whether this is worth the effort, which we address in the following section.
Vertebrates, invertebrates and fungi were the most frequently studied taxa in terrestrial systems. Only 20% of studies sequenced multiple taxonomic groups, despite the great potential of metabarcoding to survey across the tree of life (Smart et al., 2016; Stat et al., 2017). This could partly relate to the absence of a ‘one size fits all’ universal primer combination and the expense of carrying out multiple metabarcoding assays. Functional analyses (beyond species richness and community composition) were often overlooked, as previously documented (van der Heyde et al., 2022), with only ~40% of studies in our review carrying out any network or functional group/diversity analyses. A multi-marker approach, to cover different taxonomic groups, is becoming more feasible and gaining traction, and will create more opportunities for analyses of ecological networks and ecosystem function (Donald et al., 2021; Keck et al., 2022). Nonetheless, only 32% of studies that included multiple taxonomic groups undertook any functional analyses, and only 22% performed network analyzes.
Which substrate(s) are appropriate for chosen taxa and does sampling multiple substrates improve taxonomic coverage?
Environmental DNA from soil and water and ingested DNA (iDNA) were the most common substrates, as mentioned above, and ‘soil + water’ was the most common substrate combination (n=6). However, the popularity of a substrate does not necessarily reflect its suitability for detecting different taxa. We first reviewed which substrates have been used to target particular taxa, then, based on the small sample of studies (n=17) that have directly compared substrates in terrestrial systems (n=29 comparisons), we asked if certain substrates perform better for particular taxa and whether combining substrates improves taxonomic coverage. As expected, there is no clear ‘one size fits all’ substrate. Greater species richness was found in the majority (83%) of cases when two substrates were used, compared to the respective substrates in isolation, but whether this is worth the additional cost and effort will depend on the study question, feasibility and taxa of interest.
Soil samples were used for surveying all taxonomic groups, with approximately half of these studies targeting fungi and bacteria, nine (25.7%) targeting vertebrates and three (8.6%) targeting plants. Despite its popularity, soil performed poorly compared to other substrates (water, bulk invertebrates, scats, plant material and roller swabs) in multiple-substrate comparisons, in terms of the number of unique taxa identified, particularly for vertebrates and invertebrates. Performance was however more comparable to other substrates (water, plant material and microbial mats) for microbial taxa. Distribution of eDNA is known to be highly heterogeneous in soil (Hermans et al., 2022), with vertebrate eDNA particularly patchy (Seeber and Epp, 2022; Li et al., 2023). Detection of species of interest can therefore require more extensive sampling and replication, in addition to more costly and/or laborious methods to process sufficient material and overcome inhibitors (such as humic acid) and locate the ‘needle in the haystack’ (Valentin et al., 2020; Hermans et al., 2022). In addition, eDNA is generally considered to have greater persistence time in soil compared to water and therefore a less contemporary signal, although this is strongly governed by soil properties and where the sample is taken from (Sirois and Buckley, 2019); sampling at the soil surface provides a more contemporary signal of above-ground diversity compared to sampling 20 cm underground (Yasashimoto et al., 2021). Sampling soil is of particular interest for monitoring changes in the communities of below-ground taxa, for example during reforestation. Sampling the upper 10 cm of soil proved effective for detecting changes in soil fungi and bacterial composition over a 30-year chronosequence of reforestation in New Caledonia, for example (Fernandez Nuñez et al., 2021). Soil is arguably less suitable for vertebrates, particularly at landscape scales. For example, soil eDNA (and eDNA more generally) has limited application to terrestrial reptiles (reviewed in Nordstrom et al., 2022), although their detection can be improved by targeted sampling (e.g. under cover objects) and increasing soil volume (Kyle et al., 2022). However, it should be noted that soil eDNA metabarcoding can outperform camera-trapping for mammal detection over relatively local scales (Leempoel et al., 2020), highlighting the importance of considering both scale and taxa of interest.
Water samples were used for all taxonomic groups apart from protists, and over half of the studies that sampled water targeted vertebrates. eDNA detected within a waterbody reflects not only the aquatic and semi-aquatic species living within it, but also the terrestrial species that interact directly with it (through drinking, urinating etc (Harper et al., 2019), or inhabit the surrounding environment. DNA is transported via groundwater run-off and other running water, making it possible to detect species several hundred meters or even kilometers from their location, particularly in lotic environments (Deiner et al., 2016). eDNA metabarcoding of samples from a waterbody is therefore a convenient and effective way to describe the terrestrial biodiversity in a given area (Sales et al., 2020; Broadhurst et al., 2021; Lyet et al., 2021; Mena et al., 2021) and can provide catchment-scale biodiversity measures (Lyet et al., 2021). Sampling water during rainy seasons, when there is increased run-off from soil, can gather data that would otherwise require sampling two substrates (Yang et al., 2021). Water outperformed soil for detection of vertebrates and (to a lesser extent) microbes in our multi-substrate comparisons. Water has also been recommended for terrestrial invertebrate detections (Deiner et al., 2016; Sacco et al., 2022), and better targeted detection of ants was found by sampling water compared to soil with quantitative PCR (Villacorta-Rath et al., 2022) though no studies have yet compared eDNA metabarcoding of water with other substrates for terrestrial invertebrates. Choice of water as a substrate for sampling terrestrial environments of course depends on its accessibility. If permanent water bodies are not present in the site of interest, ponds, puddles, tree rot-holes (Newton et al., 2022), bromeliads (Torresdal et al., 2017) or other ephemeral sources may be appropriate for sampling water.
It is difficult to draw conclusions on the efficacy of other substrates because the number of comparisons is very small. However, roller swabs and feces were effective for sampling vertebrates compared to soil, plant material and bulk invertebrates in pairwise comparisons, while plant material, feces, bulk invertebrates, spray aggregation and (to a lesser extent) roller swabs worked well for invertebrates. Swabbing or rolling tree bark (‘tree rolling’), is particularly well-suited to forested habitats for detecting arboreal species, when avian data is a priority (Newton et al., 2022) and/or if water is not readily available for sampling. Fecal samples are also effective for detection of both vertebrate and invertebrate predator and prey (e.g. Harper et al., 2020), but sampling relies on being able to easily locate scats (van der Heyde et al., 2020). Tree-rolling and aggregated water from sprayed leaves (Allen et al., 2022) are promising, non-destructive alternatives to bulk sampling for invertebrates (Roger et al., 2022), but more research is needed to understand their efficacy as so few comparisons have been made. Although airDNA has not yet been included in multi-substrate comparisons, it has great potential to address the ‘needle in a haystack’ limitation of soil, but conversely may suffer from too great a dilution effect if applied in open spaces (an ‘everything is everywhere’ problem (Clare et al., 2021, 2022; Lynggaard et al., 2022). Further research is needed to understand the spatial and temporal distribution and dispersion of airborne DNA particles (Clare et al., 2021), but airDNA could be particularly informative when combined with other substrates, to survey different taxa, temporal and spatial scales. Ji et al. (2022) also note the potential for iDNA to enable direct measurements of biodiversity conservation outcomes across protected areas and with broad taxonomic coverage. It is important to stress that choice of substrate(s) depends on the research question, ecosystem(s), and taxonomic group(s) of interest. In the context of rewilding, it may be of interest to detect relatively short-term temporal changes in biodiversity, which requires sampling contemporary eDNA, and it is important to consider that eDNA degrades faster in water (Barnes and Turner, 2016) and on tree bark (depending on weather conditions; Allen et al., 2023).
How does metabarcoding perform when used alongside traditional methods for terrestrial target taxa?
There was large variation in the relative performance of metabarcoding in comparison to traditional methods across studies, which suggests it is highly context dependent, as has been suggested for aquatic sampling (Keck et al., 2022). This is equally apparent when looking closer at substrate choice, though the outcome for eDNA metabarcoding was slightly better when restricted to water versus traditional methods (53% found more taxa with eDNA). Terrestrial vertebrate eDNA can be highly localized in both water (Harper et al., 2019) and soil samples (Andersen et al., 2012) and the detectability of species is dependent on their level of interaction with the substrate and the local environment (Andersen et al., 2012; Ryan et al., 2022). Water samples are more likely to detect species with a high affinity to water, compared to traditional methods that may be better suited to detect fully terrestrial or arboreal species (Coutant et al., 2021; Mena et al., 2021). This is particularly important for amphibian monitoring, as species may become less detectable with aquatic eDNA after shifting to their terrestrial life-stage, highlighting the importance of the timing of sampling (Moss et al., 2022).
We found that the 12S region performed better than the 16S region in terms of unique taxa detected when compared to traditional methods. Seventy percent of 20 studies employing 12S found similar or higher taxonomic richness compared to traditional methods, whereas only 18% of 11 studies found higher richness with 16S. Targeting both these regions in combination has been widely recommended to increase taxonomic coverage (Kumar et al., 2022; Siziba and Willows-Munro, 2024). Despite this, only two of the five comparisons that employed both markers found higher taxonomic richness with eDNA. Our findings might therefore suggest that 12S is a better choice for maximizing taxonomic coverage of vertebrates, and that there is little to gain from also including 16S. However, this result is likely influenced by the choice of different primers for the two regions, amplicon length, reference database coverage, and other features of the study design. In a recent direct comparison, newly designed vertebrate primers for 16S outperformed 12S and COI primers in terms of detection, amplifying 98% of vertebrate species included in in silico tests (Wang et al., 2023). This study also found improved species detection with multiple markers and highlighted the complementary nature of the three regions (Wang et al., 2023), therefore we caution against dismissing 16S based on the small number of comparisons included here.
Finally, we found mixed results in the relative performance of methods in terms of the vertebrate taxa they detected, though there is some suggestion that traditional methods may remain a more favorable choice for bird and reptile monitoring. Low DNA shedding rates due to the keratinized exterior of reptiles may reduce their detectability in environmental samples (Adams et al., 2019; Andruszkiewicz Allan et al., 2021). Likewise, the diversity and life-histories of taxa may influence detectability. Flying species, in addition to solitary, large-ranging species such as carnivores, may have limited interactions with terrestrial sampling substrates and therefore tend to be better detected with camera traps or field surveys (Leempoel et al., 2020; Sales et al., 2020; Mena et al., 2021; Kim et al., 2022; Mas-Carrió et al., 2022). Comparatively, smaller, more cryptic mammals are generally detected better with eDNA (Harper et al., 2019; Mena et al., 2021; Ryan et al., 2022). However, as previously discussed, sampling substrate can also influence detectability, as ingested DNA derived from flies is well suited to detecting arboreal species (Gogarten et al., 2020; Massey et al., 2022), which are difficult to detect with standard camera trapping methods (Moore et al., 2021).
Obtaining perfect congruence between metabarcoding-based methods and traditional methods is impossible because the character of the data is completely different, and this should not impede the application of eDNA-based tools (Pawlowski et al., 2021). Instead, eDNA metabarcoding should be considered an important addition to the ecological monitoring ‘toolkit’ and continue to be used to complement more established monitoring techniques within terrestrial contexts. Within the context of rewilding monitoring, if the aim is to consistently monitor a wide scope across the tree of life, a multi-method ‘toolkit’ sampling design would likely be the best approach. For its wider implementation, this ‘multi-tool’ approach will likely need to be balanced with the relative costs of different methods. eDNA metabarcoding in particular has been shown to be comparatively lower cost to more traditional methods across different contexts (Fediajevaite et al., 2021), though this can vary, particularly for sites with lower taxonomic diversity (Bálint et al., 2018).
Limitations and research gaps
Our meta-analysis is based on studies that display a high degree of heterogeneity in terms of study design, and approach to data collection and analysis. To overcome this caveat requires large-scale individual studies that compare methods in the same way across diverse systems, and the adoption of method standards across studies; something that the global eDNA research community is starting to address (Hirsch et al., 2024).
Questions still remain regarding the best eDNA substrate choice for terrestrial monitoring. Notably, airDNA has not yet been ground-truthed against other survey methods, and there is much to learn about how it compares to other eDNA approaches. Improved understanding of the ‘ecology of eDNA’ and its persistence in terrestrial settings would also enable a more informed choice of sampling substrate (Leandro et al., 2024). Additionally, despite suggestions that sampling multiple substrates can ensure more comprehensive ecological monitoring (Hassan et al., 2022), only a small proportion of the terrestrial eDNA studies reviewed here did this, and many studies failed to justify their substrate choice or acknowledge alternatives. Improving transparency and reproducibility in metabarcoding workflows is a priority across all studies to facilitate better sampling strategies and uptake of eDNA for terrestrial monitoring.
Questions also remain around the best combination of survey methods to use for the rewilding monitoring toolkit. Our results suggest a multi-method strategy, using a combination of metabarcoding and established survey methods, increases the number of taxa detected. However, comparisons between metabarcoding and acoustic monitoring, which is emerging as a highly effective survey tool in terrestrial settings, were a notable omission from our reviewed papers. Acoustic monitoring has so far only been compared to eDNA in aquatic (Easson et al., 2020; Sato et al., 2021) or species-specific contexts (Takahara et al., 2020), though results indicate similar, patterns to our general findings, with detectability of taxa for either method depending on the ecological characteristics of the respective target species. The level of disturbance created by different methods and how this could impact the nature-driven ethos of rewilding should also be considered. For example, while eDNA sampling can offer a detailed snapshot of a community, sampling may still cause disturbances, and this level of data may only be necessary at key milestones during a project’s trajectory. More continuous monitoring methods, such as camera or acoustic tools, may provide sufficient data between surveys, whilst providing additional data regarding population sizes and behavior (O’Connell et al., 2010; Marques et al., 2013). Understanding the efficacy of metabarcoding at different stages during a project’s progression may be crucial for its effective integration into rewilding research and practice.
Rewilding aims to restore the functional ecology of ecosystems (Torres et al., 2018) but our review highlights that few terrestrial metabarcoding studies perform functional diversity and/or network analyzes. The assignment of functional groups is possible from DNA data, by using functional trait databases that exist for certain taxonomic groups (e.g. fungi FunTraits, Põlme et al., 2020), though reference database gaps can create uncertainty and bias in functional diversity estimates (Condachou et al., 2023). Ancillary information is often required to associate taxa with functional traits or trophic levels and the availability and reliability of this information may limit the uptake of functional analyzes with DNA metabarcoding data (Evans et al., 2016; Pereira et al., 2023). Trophic networks can be readily constructed, and network parameters estimated via DNA metabarcoding of feces or iDNA, or, from swabs of plants and pollinators (Evans and Kitson, 2020). Networks can also be constructed just from community composition data, by assigning functional feeding groups from literature or databases, but few eDNA studies have yet adopted this approach (but see Blackman et al., 2022) but our review highlights that few terrestrial metabarcoding studies perform functional diversity and/or network analyzes. The assignment of functional groups is possible from DNA data, by using functional trait databases that exist for certain taxonomic groups (e.g. fungi FunTraits, Põlme et al., 2020), though reference database gaps can create uncertainty and bias in functional diversity estimates (Condachou et al., 2023). Ancillary information is often required to associate taxa with functional traits or trophic levels and the availability and reliability of this information may limit the uptake of functional analyzes with DNA metabarcoding data (Evans et al., 2016; Pereira et al., 2023). Trophic networks can be readily constructed, and network parameters estimated via DNA metabarcoding of feces or iDNA, or, from swabs of plants and pollinators (Evans and Kitson, 2020). Networks can also be constructed just from community composition data, by assigning functional feeding groups from literature or databases, but few eDNA studies have yet adopted this approach (but see Blackman et al., 2022).
Metabarcoding of eRNA is gaining traction for biodiversity monitoring because of its greater lability and potential for distinguishing live from dead sources, compared to eDNA (e.g. Cristescu, 2019; Littlefair et al., 2022). eRNA is also arguably more suited than eDNA to studying ecosystem function, as it allows the detection of changes in expression of single or multiple genes or whole metatranscriptomes in response to environmental change (Yates et al., 2021; Hechler et al., 2023). However, eRNA analysis is still in its infancy relative to eDNA metabarcoding, so was not included in our review. Studies that ground-truth eDNA and eRNA analyzes against traditional monitoring in terrestrial contexts would be useful to understand the relative pros and cons of the different approaches.
Finally, it should be noted that although metabarcoding is currently the most widely used approach for community analyzes of eDNA, bulk or iDNA samples, it is not the only molecular approach to biodiversity monitoring, and it has its limitations, particularly in relation to amplification bias during PCR (see e.g. Nichols et al., 2018). Hybridization capture (or target enrichment metabarcoding), which utilizes oligonucleotide baits complementary to barcodes or other regions of interest, for example, is an emerging PCR-free alternative to traditional metabarcoding, but not without its own biases and limitations (Giebner et al., 2020; Nota et al., 2024). These emerging molecular technologies hold promise for the monitoring of rewilding projects, but further research is required to establish their uses and limitations.
Conclusion
Adaptive ecological monitoring plays a pivotal role in understanding ecosystem dynamics and informing management strategies for terrestrial rewilding projects. Although underutilized in terrestrial contexts, eDNA metabarcoding offers promise in striking the balance between minimizing disturbance and maximizing data collection efficacy across different ecosystems and taxa. However, it is imperative that sampling design, substrate(s) and assay choice are carefully considered as these choices are highly context dependent. Monitoring strategies for rewilding need to be designed to encompass spatial and temporal variability of ecosystems and distributions of taxa. A combination of eDNA and other survey methods will maximize taxonomic coverage, but eDNA has a clear role to play as a complementary tool in rewilding and other terrestrial monitoring schemes.
Data availability statement
The datasets presented in this study can be found in online repositories. The names of the repository/repositories and accession number(s) can be found in the article/Supplementary Material.
Author contributions
CC: Conceptualization, Writing – original draft, Writing – review & editing, Data curation, Formal analysis, Investigation, Methodology, Project administration, Resources, Software, Validation, Visualization. JG: Conceptualization, Funding acquisition, Project administration, Supervision, Writing – review & editing. IC: Conceptualization, Project administration, Supervision, Writing – original draft, Writing – review & editing. LLH: Conceptualization, Funding acquisition, Investigation, Methodology, Project Administration, Supervision, Validation, Writing – original draft, Writing – review & editing.
Funding
The author(s) declare financial support was received for the research, authorship, and/or publication of this article. This study was supported by the University of Hull Rewild Cluster studentship.
Acknowledgments
Thanks to Matthew Morgan and Declan Judge for comments on previous drafts and to Clare Collins, Graham Sellers and Matthew Morgan for help and advice in creating the data visualizations.
Conflict of interest
The authors declare that the research was conducted in the absence of any commercial or financial relationships that could be construed as a potential conflict of interest.
Publisher’s note
All claims expressed in this article are solely those of the authors and do not necessarily represent those of their affiliated organizations, or those of the publisher, the editors and the reviewers. Any product that may be evaluated in this article, or claim that may be made by its manufacturer, is not guaranteed or endorsed by the publisher.
Supplementary material
The Supplementary Material for this article can be found online at: https://www.frontiersin.org/articles/10.3389/fcosc.2024.1473957/full#supplementary-material
References
Abrams J. F., Hörig L. A., Brozovic R., Axtner J., Crampton-Platt A., Mohamed A., et al. (2019). Shifting up a gear with iDNA: From mammal detection events to standardised surveys. J. Appl. Ecol. 56, 1637–1648. doi: 10.1111/1365-2664.13411
Adams C. I. M., Hoekstra L. A., Muell M. R., Janzen F. J. (2019). A Brief Review of Non-Avian Reptile Environmental DNA (eDNA), with a Case Study of Painted Turtle (Chrysemys picta) eDNA Under Field Conditions. Diversity 11, 50. doi: 10.3390/d11040050
Allen M., Kwait R., Vastano A., Kisurin A., Zoccolo I., Jaffe B. D., et al. (2023). Sampling environmental DNA from trees and soil to detect cryptic arboreal mammals. Sci. Rep. 13, 180. doi: 10.1038/s41598-023-27512-8
Allen M., Lockwood J., Kwait R., Vastano A., Peterson D., Tkacenko L., et al. (2022). Using surface environmental DNA to assess arthropod biodiversity within a forested ecosystem. Authorea Preprints 5, 1652-1666. doi: 10.22541/au.166723594.46081923/v1
Andersen K., Bird K. L., Rasmussen M., Haile J., Breuning-Madsen H., Kjaer K. H., et al. (2012). Meta-barcoding of “dirt” DNA from soil reflects vertebrate biodiversity. Mol. Ecol. 21, 1966–1979. doi: 10.1111/j.1365-294X.2011.05261.x
Andruszkiewicz Allan E., Zhang W. G., C. Lavery A., XXXF. Govindarajan A. (2021). Environmental DNA shedding and decay rates from diverse animal forms and thermal regimes. Environ. DNA 3, 492–514. doi: 10.1002/edn3.141
Bálint M., Nowak C., Márton O., Pauls S. U., Wittwer C., Aramayo J. L., et al. (2018). Accuracy, limitations and cost efficiency of eDNA-based community survey in tropical frogs. Mol. Ecol. Resour. 18, 1415–1426. doi: 10.1111/1755-0998.12934
Barnes M. A., Turner C. R. (2016). The ecology of environmental DNA and implications for conservation genetics. Conserv. Genet. 17, 1–17. doi: 10.1007/s10592-015-0775-4
Beng K. C., Corlett R. T. (2020). Applications of environmental DNA (eDNA) in ecology and conservation: opportunities, challenges and prospects. Biodivers. Conserv. 29, 2089–2121. doi: 10.1007/s10531-020-01980-0
Blackman R., Couton M., Keck F., Kirschner D., Carraro L., Cereghetti E., et al. (2024). Environmental DNA: The next chapter. Mol. Ecol. 33, e17355. doi: 10.1111/mec.17355
Blackman R. C., Ho H.-C., Walser J.-C., Altermatt F. (2022). Spatio-temporal patterns of multi-trophic biodiversity and food-web characteristics uncovered across a river catchment using environmental DNA. Commun. Biol. 5, 259. doi: 10.1038/s42003-022-03216-z
Boyer S., Cruickshank R. H., Wratten S. D. (2015). Feces of generalist predators as “biodiversity capsules”: A new tool for biodiversity assessment in remote and inaccessible habitats. Food Webs 3, 1–6. doi: 10.1016/j.fooweb.2015.02.001
Broadhurst H. A., Gregory L. M., Bleakley E. K., Perkins J. C., Lavin J. V., Bolton P., et al. (2021). Mapping differences in mammalian distributions and diversity using environmental DNA from rivers. Sci. Total Environ. 801, 149724. doi: 10.1016/j.scitotenv.2021.149724
Brockerhoff E. G., Barbaro L., Castagneyrol B., Forrester D. I., Gardiner B., González-Olabarria J. R., et al. (2017). Forest biodiversity, ecosystem functioning and the provision of ecosystem services. Biodivers. Conserv. 26, 3005–3035. doi: 10.1007/s10531-017-1453-2
Carver S., Convery I., Hawkins S., Beyers R., Eagle A., Kun Z., et al. (2021). Guiding principles for rewilding. Conserv. Biol. 35, 1882–1893. doi: 10.1111/cobi.13730
Clare E. L., Economou C. K., Bennett F. J., Dyer C. E., Adams K., McRobie B., et al. (2022). Measuring biodiversity from DNA in the air. Curr. Biol. 32, 693–700.e5. doi: 10.1016/j.cub.2021.11.064
Clare E. L., Economou C. K., Faulkes C. G., Gilbert J. D., Bennett F., Drinkwater R., et al. (2021). eDNAir: proof of concept that animal DNA can be collected from air sampling. PeerJ 9, e11030. doi: 10.7717/peerj.11030
Coble A. A., Flinders C. A., Homyack J. A., Penaluna B. E., Cronn R. C., Weitemier K. (2019). eDNA as a tool for identifying freshwater species in sustainable forestry: A critical review and potential future applications. Sci. Total Environ. 649, 1157–1170. doi: 10.1016/j.scitotenv.2018.08.370
Condachou C., Milhau T., Murienne J., Brosse S., Villéger S., Valentini A., et al. (2023). Inferring functional diversity from environmental DNA metabarcoding. Environ. DNA 5, 934–944. doi: 10.1002/edn3.391
Coutant O., Richard-Hansen C., de Thoisy B., Decotte J.-B., Valentini A., Dejean T., et al. (2021). Amazonian mammal monitoring using aquatic environmental DNA. Mol. Ecol. Resour. 21, 1875–1888. doi: 10.1111/1755-0998.13393
Creer S., Deiner K., Frey S., Porazinska D., Taberlet P., Thomas W. K., et al. (2016). The ecologist’s field guide to sequence-based identification of biodiversity. Methods Ecol. Evol. 7, 1008–1018. doi: 10.1111/2041-210x.12574
Cristescu M. E. (2019). Can environmental RNA revolutionize biodiversity science? Trends Ecol. Evol. 34, 694–697. doi: 10.1016/j.tree.2019.05.003
Deiner K., Bik H. M., Mächler E., Seymour M., Lacoursière-Roussel A., Altermatt F., et al. (2017). Environmental DNA metabarcoding: Transforming how we survey animal and plant communities. Mol. Ecol. 26, 5872–5895. doi: 10.1111/mec.14350
Deiner K., Fronhofer E. A., Mächler E., Walser J.-C., Altermatt F. (2016). Environmental DNA reveals that rivers are conveyer belts of biodiversity information. Nat. Commun. 7, 12544. doi: 10.1038/ncomms12544
DiBattista J. D., Fowler A. M., Riley I. J., Reader S., Hay A., Parkinson K., et al. (2022). The use of environmental DNA to monitor impacted coastal estuaries. Mar. pollut. Bull. 181, 113860. doi: 10.1016/j.marpolbul.2022.113860
Donald J., Murienne J., Chave J., Iribar A., Louisanna E., Manzi S., et al. (2021). Multi-taxa environmental DNA inventories reveal distinct taxonomic and functional diversity in urban tropical forest fragments. Global Ecol. Conserv. 29, e01724. doi: 10.1016/j.gecco.2021.e01724
Drinkwater R., Jucker T., Potter J. H. T., Swinfield T., Coomes D. A., Slade E. M., et al. (2021a). Leech blood-meal invertebrate-derived DNA reveals differences in Bornean mammal diversity across habitats. Mol. Ecol. 30, 3299–3312. doi: 10.1111/mec.15724
Drinkwater R., Williamson J., Clare E. L., Chung A. Y. C., Rossiter S. J., Slade E. (2021b). Dung beetles as samplers of mammals in Malaysian Borneo—a test of high throughput metabarcoding of iDNA. PeerJ 9, e11897. doi: 10.7717/peerj.11897
du Toit J. T., Pettorelli N. (2019). The differences between rewilding and restoring an ecologically degraded landscape. J. Appl. Ecol. 56, 2467–2715. doi: 10.1111/1365-2664.13487
Easson C. G., Boswell K. M., Tucker N., Warren J. D., Lopez J. V. (2020). Combined eDNA and acoustic analysis reflects diel vertical migration of mixed consortia in the gulf of Mexico. Front. Mar. Sci. 7. doi: 10.3389/fmars.2020.00552
Edwards M. E., Alsos I. G., Yoccoz N., Coissac E., Goslar T., Gielly L., et al. (2018). Metabarcoding of modern soil DNA gives a highly local vegetation signal in Svalbard tundra. Holocene 28, 2006–2016. doi: 10.1177/0959683618798095
Evans D. M., Kitson J. J. N. (2020). Molecular ecology as a tool for understanding pollination and other plant–insect interactions. Curr. Opin. Insect Sci. 38, 26–33. doi: 10.1016/j.cois.2020.01.005
Evans D. M., Kitson J. J. N., Lunt D. H., Straw N. A., Pocock M. J. O. (2016). Merging DNA metabarcoding and ecological network analysis to understand and build resilient terrestrial ecosystems. Funct. Ecol. 30, 1904–1916. doi: 10.1111/1365-2435.12659
Fediajevaite J., Priestley V., Arnold R., Savolainen V. (2021). Meta-analysis shows that environmental DNA outperforms traditional surveys, but warrants better reporting standards. Ecol. Evol. 11, 4803–4815. doi: 10.1002/ece3.7382
Fernández N., Navarro L. M., Pereira H. M. (2017). Rewilding: A call for boosting ecological complexity in conservation. Conserv. Lett. 10, 276–278. doi: 10.1111/conl.12374
Fernandez Nuñez N., Maggia L., Stenger P.-L., Lelievre M., Letellier K., Gigante S., et al. (2021). Potential of high-throughput eDNA sequencing of soil fungi and bacteria for monitoring ecological restoration in ultramafic substrates: The case study of the New Caledonian biodiversity hotspot. Ecol. Eng. 173, 106416. doi: 10.1016/j.ecoleng.2021.106416
Garnier S., Ross N., Rudis R., Camargo A. P., Sciaini M., Scherer C. (2023). viridis(Lite) - Colorblind-Friendly Color Maps for R. viridis package version 0.6.4. Available at: https://rdrr.io/cran/viridisLite/ (Accessed July 26, 2024).
Gehlenborg N. (2019). UpSetR: A More Scalable Alternative to Venn and Euler Diagrams for Visualizing Intersecting Sets (R package version 1.4.0). Available at: https://CRAN.R-project.org/package=UpSetR (Accessed July 26, 2024).
Giebner H., Langen K., Bourlat S. J., Kukowka S., Mayer C., Astrin J. J., et al. (2020). Comparing diversity levels in environmental samples: DNA sequence capture and metabarcoding approaches using 18S and COI genes. Mol. Ecol. Resour. 20, 1333–1345. doi: 10.1111/1755-0998.13201
Gogarten J. F., Hoffmann C., Arandjelovic M., Sachse A., Merkel K., Dieguez P., et al. (2020). Fly-derived DNA and camera traps are complementary tools for assessing mammalian biodiversity. Environ. DNA 2, 63–76. doi: 10.1002/edn3.46
Gregorič M., Kutnjak D., Bačnik K., Gostinčar C., Pecman A., Ravnikar M., et al. (2022). Spider webs as eDNA samplers: Biodiversity assessment across the tree of life. Mol. Ecol. Resour. 22, 2534–2545. doi: 10.1111/1755-0998.13629
Grosberg R. K., Vermeij G. J., Wainwright P. C. (2012). Biodiversity in water and on land. Curr. Biol. 22, R900–R903. doi: 10.1016/j.cub.2012.09.050
Harper L. R., Lawson Handley L., Carpenter A. I., Ghazali M., Di Muri C., Macgregor C. J., et al. (2019). Environmental DNA (eDNA) metabarcoding of pond water as a tool to survey conservation and management priority mammals. Biol. Conserv. 238, 108225. doi: 10.1016/j.biocon.2019.108225
Harper L. R., Watson H. V., Donnelly R., Hampshire R., Sayer C. D., Breithaupt T., et al. (2020). Using DNA metabarcoding to investigate diet and niche partitioning in the native European otter (Lutra lutra) and invasive American mink (Neovison vison). bioRxiv 2020, 7.03.186346. doi: 10.1101/2020.07.03.186346
Hassan S., Ganiee S. A., Yaseen A., Zaman M., Shah A. J., Ganai B. A. (2023). Unraveling the potential of environmental DNA for deciphering recent advances in plant–animal interactions: a systematic review. Planta 258, 117. doi: 10.1007/s00425-023-04267-0
Hassan S., Poczai P., Ganai B. A., Almalki W. H., Gafur A., Sayyed R. Z. (2022). Environmental DNA metabarcoding: A novel contrivance for documenting terrestrial biodiversity. Biology 11, 1297. doi: 10.3390/biology11091297
Hechler R. M., Yates M. C., Chain F. J. J., Cristescu M. E. (2023). Environmental transcriptomics under heat stress: Can environmental RNA reveal changes in gene expression of aquatic organisms?. Molecular Ecology 00,1–15. doi: 10.1111/mec.17152
Hermans S. M., Lear G., Buckley T. R., Buckley H. L. (2022). Environmental DNA sampling detects between-habitat variation in soil arthropod communities, but is a poor indicator of fine-scale spatial and seasonal variation. Ecol. Indic. 140, 109040. doi: 10.1016/j.ecolind.2022.109040
Hirsch S., Acharya-Patel N., Amamoo P. A., Borrero-Pérez G. H., Cahyani N. K. D., Ginigini J. G. M., et al. (2024). Centering accessibility, increasing capacity, and fostering innovation in the development of international eDNA standards. Metabarcoding Metagenomics 8, e126058. doi: 10.3897/mbmg.8.126058
Ishige T., Miya M., Ushio M., Sado T., Ushioda M., Maebashi K., et al. (2017). Tropical-forest mammals as detected by environmental DNA at natural saltlicks in Borneo. Biol. Conserv. 210, 281–285. doi: 10.1016/j.biocon.2017.04.023
Ji Y., Baker C. C. M., Popescu V. D., Wang J., Wu C., Wang Z., et al. (2022). Measuring protected-area effectiveness using vertebrate distributions from leech iDNA. Nat. Commun. 13, 1555. doi: 10.1038/s41467-022-28778-8
Keck F., Blackman R. C., Bossart R., Brantschen J., Couton M., Hürlemann S., et al. (2022). Meta-analysis shows both congruence and complementarity of DNA and eDNA metabarcoding to traditional methods for biological community assessment. Mol. Ecol. 31, 1820–1835. doi: 10.1111/mec.16364
Kestel J. H., Field D. L., Bateman P. W., White N. E., Allentoft M. E., Hopkins A. J. M., et al. (2022). Applications of environmental DNA (eDNA) in agricultural systems: Current uses, limitations and future prospects. Sci. Total Environ. 847, 157556. doi: 10.1016/j.scitotenv.2022.157556
Kim W.-M., Lee H.-J., Song W. (2022). Environmental DNA metabarcoding effectively monitors terrestrial species by using urban green spaces. Urban For. Urban Greening 78, 127782. doi: 10.1016/j.ufug.2022.127782
Kinoshita G., Yonezawa S., Murakami S., Isagi Y. (2019). Environmental DNA collected from snow tracks is useful for identification of mammalian species. Zoolog. Sci. 36, 198–207. doi: 10.2108/zs180172
Kumar G., Reaume A. M., Farrell E., Gaither M. R. (2022). Comparing eDNA metabarcoding primers for assessing fish communities in a biodiverse estuary. PloS One 17, e0266720. doi: 10.1371/journal.pone.0266720
Kyle K. E., Allen M. C., Dragon J., Bunnell J. F., Reinert H. K., Zappalorti R., et al. (2022). Combining surface and soil environmental DNA with artificial cover objects to improve terrestrial reptile survey detection. Conserv. Biol. 36, e13939. doi: 10.1111/cobi.13939
Lawson Handley L. (2015). How will the “molecular revolution” contribute to biological recording?: The Molecular Revolution in Biological Recording. Biol. J. Linn. Soc Lond. 115, 750–766. doi: 10.1111/bij.12516
Leandro C., Jay-Robert P., Pétillon J. (2024). eDNA for monitoring and conserving terrestrial arthropods: Insights from a systematic map and barcode repositories assessments. Insect Conserv. Divers. 17, 565-678. doi: 10.1111/icad.12726
Leempoel K., Hebert T., Hadly E. A. (2020). A comparison of eDNA to camera trapping for assessment of terrestrial mammal diversity. Proc. Biol. Sci. 287, 20192353. doi: 10.1098/rspb.2019.2353
Li J., Seeber P., Axtner J., Crouthers R., Groenenberg M., Koehncke A., et al. (2023). Monitoring terrestrial wildlife by combining hybridization capture and metabarcoding data from waterhole environmental DNA. Biol. Conserv. 284, 110168. doi: 10.1016/j.biocon.2023.110168
Littlefair J. E., Rennie M. D., Cristescu M. E. (2022). Environmental nucleic acids: A field-based comparison for monitoring freshwater habitats using eDNA and eRNA. Mol. Ecol. Resour. 22, 2928–2940. doi: 10.1111/1755-0998.13671
Lyet A., Pellissier L., Valentini A., Dejean T., Hehmeyer A., Naidoo R. (2021). eDNA sampled from stream networks correlates with camera trap detection rates of terrestrial mammals. Sci. Rep. 11, 11362. doi: 10.1038/s41598-021-90598-5
Lynggaard C., Bertelsen M. F., Jensen C. V., Johnson M. S., Frøslev T. G., Olsen M. T., et al. (2022). Airborne environmental DNA for terrestrial vertebrate community monitoring. Curr. Biol. 32, 701–707.e5. doi: 10.1016/j.cub.2021.12.014
Marques T. A., Thomas L., Martin S. W., Mellinger D. K., Ward J. A., Moretti D. J., et al. (2013). Estimating animal population density using passive acoustics. Biol. Rev. Camb. Philos. Soc 88, 287–309. doi: 10.1111/brv.12001
Mas-Carrió E., Schneider J., Nasanbat B., Ravchig S., Buxton M., Nyamukondiwa C., et al. (2022). Assessing environmental DNA metabarcoding and camera trap surveys as complementary tools for biomonitoring of remote desert water bodies. Environ. DNA 4, 580–595. doi: 10.1002/edn3.274
Massey A. L., Bronzoni R. V., de M., da Silva D. J. F., Allen J. M., de Lázari P. R., et al. (2022). Invertebrates for vertebrate biodiversity monitoring: Comparisons using three insect taxa as iDNA samplers. Mol. Ecol. Resour. 22, 962–977. doi: 10.1111/1755-0998.13525
Mena J. L., Yagui H., Tejeda V., Bonifaz E., Bellemain E., Valentini A., et al. (2021). Environmental DNA metabarcoding as a useful tool for evaluating terrestrial mammal diversity in tropical forests. Ecol. Appl. 31, e02335. doi: 10.1002/eap.2335
Meyer J. M., Leempoel K., Losapio G., Hadly E. A. (2020). Molecular ecological network analyzes: An effective conservation tool for the assessment of biodiversity, trophic interactions, and community structure. Front. Ecol. Evol. 8. doi: 10.3389/fevo.2020.588430
Mongeon P., Paul-Hus A. (2016). The journal coverage of Web of Science and Scopus: a comparative analysis. Scientometrics 106, 213–228. doi: 10.1007/s11192-015-1765-5
Moore J. F., Soanes K., Balbuena D., Beirne C., Bowler M., Carrasco-Rueda F., et al. (2021). The potential and practice of arboreal camera trapping. Methods Ecol. Evol. 12, 1768–1779. doi: 10.1111/2041-210x.13666
Moss W. E., Harper L. R., Davis M. A., Goldberg C. S., Smith M. M., Johnson P. T. J. (2022). Navigating the trade-offs between environmental DNA and conventional field surveys for improved amphibian monitoring. Ecosphere 13, e3941. doi: 10.1002/ecs2.3941
Mutillod C., Buisson É., Mahy G., Jaunatre R., Bullock J. M., Tatin L., et al. (2024). Ecological restoration and rewilding: two approaches with complementary goals? Biol. Rev. 99, 820–836. doi: 10.1111/brv.13046
Nelson C. R. (2022). “Ecological restoration and rewilding: Integrating communities of practice to achieve common goals,” in Routledge Handbook of Rewilding (Abingdon, UK: Routledge), 31–41.
Newton J. P., Bateman P. W., Heydenrych M. J., Mousavi-Derazmahalleh M., Nevill P. (2022). Home is where the hollow is: Revealing vertebrate tree hollow user biodiversity with eDNA metabarcoding. Environ. DNA 4, 1078–1091. doi: 10.1002/edn3.313
Nichols R. V., Vollmers C., Newsom L. A., Wang Y., Heintzman P. D., Leighton M., et al. (2018). Minimizing polymerase biases in metabarcoding. Mol. Ecol. Resour. 18, 927–939. doi: 10.1111/1755-0998.12895
Nordstrom B., Mitchell N., Byrne M., Jarman S. (2022). A review of applications of environmental DNA for reptile conservation and management. Ecol. Evol. 12, e8995. doi: 10.1002/ece3.8995
Nørgaard L., Olesen C. R., Trøjelsgaard K., Pertoldi C., Nielsen J. L., Taberlet P., et al. (2021). eDNA metabarcoding for biodiversity assessment, generalist predators as sampling assistants. Sci. Rep. 11, 6820. doi: 10.1038/s41598-021-85488-9
Nota K., Orlando L., Marchesini A., Girardi M., Bertilsson S., Vernesi C., et al. (2024). Enriching barcoding markers in environmental samples utilizing a phylogenetic probe design: Insights from mock communities. Environ. DNA (Hoboken N.J.) 6, e593. doi: 10.1002/edn3.593
O’Connell A. F., Nichols J. D., Ullas Karanth K. (2010). Camera Traps in Animal Ecology: Methods and Analyses (Tokyo: Springer Science & Business Media). Available at: https://play.google.com/store/books/details?id=onO2967GdPwC (Accessed July 02, 2024).
Pawlowski J., Bonin A., Boyer F., Cordier T., Taberlet P. (2021). Environmental DNA for biomonitoring. Mol. Ecol. 30, 2931–2936. doi: 10.1111/mec.16023
Pebesma E., Bivand R. (2023). Spatial Data Science: With Applications in R (New York: Chapman and Hall/CRC). doi: 10.1201/9780429459016
Perino A., Pereira H. M., Navarro L. M., Fernández N., Bullock J. M., Ceauşu S., et al. (2019). Rewilding complex ecosystems. Science 364, eaav5570. doi: 10.1126/science.aav5570
Pereira C. L., Ersoy Z., Gilbert M. T. P., Gravel D., Araujo M. B., Matias M. G. (2023). Future-proofing environmental DNA and trait-based predictions of food webs. BioScience 73, 862–878. doi: 10.1093/biosci/biad089
Pettorelli N., Barlow J., Stephens P. A., Durant S. M., Connor B., Bühne H., et al. (2018). Making rewilding fit for policy. J. Appl. Ecol. 55, 1114–1125. doi: 10.1111/1365-2664.13082
Pettorelli N., Bullock J. M. (2023). Restore or rewild? Implementing complementary approaches to bend the curve on biodiversity loss. Ecol. Sol. Evid. 4, e12244. doi: 10.1002/2688-8319.12244
Põlme S., Abarenkov K., Henrik Nilsson R., Lindahl B. D., Clemmensen K. E., Kauserud H., et al. (2020). FungalTraits: a user-friendly traits database of fungi and fungus-like stramenopiles. Fungal Divers. 105, 1–16. doi: 10.1007/s13225-020-00466-2
Posit team (2024). RStudio: Integrated Development Environment for R (Boston, MA: Posit Software, PBC). Available at: http://www.posit.co/ (Accessed July 26, 2024).
Prior J., Brady E. (2017). Environmental aesthetics and rewilding. Environ. Values 26, 31–51. doi: 10.3197/096327117X14809634978519
R Core Team (2023). R: A Language and Environment for Statistical Computing (Vienna, Austria: R Foundation for Statistical Computing). Available at: https://www.R-project.org/ (Accessed July 26, 2024).
Rishan S. T., Kline R. J., Rahman M. S. (2023). Applications of environmental DNA (eDNA) to detect subterranean and aquatic invasive species: A critical review on the challenges and limitations of eDNA metabarcoding. Environ. Adv. 12, 100370. doi: 10.1016/j.envadv.2023.100370
Roger F., Ghanavi H. R., Danielsson N., Wahlberg N., Löndahl J., Pettersson L. B., et al. (2022). Airborne environmental DNA metabarcoding for the monitoring of terrestrial insects—A proof of concept from the field. Environ. DNA 4, 790–807. doi: 10.1002/edn3.290
Rudis B. (2020). hrbrthemes: Additional Themes, Theme Components and Utilities for ‘ggplot2’ (R package version 0.8.0). Available at: https://CRAN.R-project.org/package=hrbrthemes (Accessed July 02, 2024).
Ryan E., Bateman P., Fernandes K., van der Heyde M., Nevill P. (2022). eDNA metabarcoding of log hollow sediments and soils highlights the importance of substrate type, frequency of sampling and animal size, for vertebrate species detection. Environ. DNA 4, 940–953. doi: 10.1002/edn3.306
Sacco M., Guzik M. T., van der Heyde M., Nevill P., Cooper S. J. B., Austin A. D., et al. (2022). eDNA in subterranean ecosystems: Applications, technical aspects, and future prospects. Sci. Total Environ. 820, 153223. doi: 10.1016/j.scitotenv.2022.153223
Sales N. G., McKenzie M. B., Drake J., Harper L. R., Browett S. S., Coscia I., et al. (2020). Fishing for mammals: Landscape-level monitoring of terrestrial and semi-aquatic communities using eDNA from riverine systems. J. Appl. Ecol. 57, 707–716. doi: 10.1111/1365-2664.13592
Sato M., Inoue N., Nambu R., Furuichi N., Imaizumi T., Ushio M. (2021). Quantitative assessment of multiple fish species around artificial reefs combining environmental DNA metabarcoding and acoustic survey. Sci. Rep. 11, 19477. doi: 10.1038/s41598-021-98926-5
Schenekar T. (2023). The current state of eDNA research in freshwater ecosystems: are we shifting from the developmental phase to standard application in biomonitoring? Hydrobiologia 850, 1263–1282. doi: 10.1007/s10750-022-04891-z
Schnell I. B., Sollmann R., Calvignac-Spencer S., Siddall M. E., Douglas W. Y., Wilting A., et al. (2015). iDNA from terrestrial haematophagous leeches as a wildlife surveying and monitoring tool–prospects, pitfalls and avenues to be developed. Front. Zool. 12, 24. doi: 10.1186/s12983-015-0115-z
Schnell I. B., Thomsen P. F., Wilkinson N., Rasmussen M., Jensen L. R. D., Willerslev E., et al. (2012). Screening mammal biodiversity using DNA from leeches. Curr. Biol. 22, R262–R263. doi: 10.1016/j.cub.2012.02.058
Seeber P. A., Epp L. S. (2022). Environmental DNA and metagenomics of terrestrial mammals as keystone taxa of recent and past ecosystems. Mamm. Rev. 52, 538–553. doi: 10.1111/mam.12302
Siegenthaler A., Wangensteen O. S., Soto A. Z., Benvenuto C., Corrigan L., Mariani S. (2019). Metabarcoding of shrimp stomach content: Harnessing a natural sampler for fish biodiversity monitoring. Mol. Ecol. Resour. 19, 206–220. doi: 10.1111/1755-0998.12956
Sirois S. H., Buckley D. H. (2019). Factors governing extracellular DNA degradation dynamics in soil. Environ. Microbiol. Rep. 11, 173–184. doi: 10.1111/1758-2229.12725
Siziba V. I., Willows-Munro S. (2024). An assessment of South African small mammal barcode sequence libraries: Implications for future carnivore diet analyzes by DNA. Afr. J. Ecol. 62, e13233. doi: 10.1111/aje.13233
Sjoberg D. (2023). ggsankey: Sankey, Alluvial and Sankey Bump Plots (R package version 0.0.99999). https://github.com/davidsjoberg/ggsankey (Accessed July 26, 2024)
Smart A. S., Weeks A. R., van Rooyen A. R., Moore A., McCarthy M. A., Tingley R. (2016). Assessing the cost-efficiency of environmental DNA sampling. Methods Ecol. Evol. 7, 1291–1298. doi: 10.1111/2041-210x.12598
Stat M., Huggett M. J., Bernasconi R., DiBattista J. D., Berry T. E., Newman S. J., et al. (2017). Ecosystem biomonitoring with eDNA: metabarcoding across the tree of life in a tropical marine environment. Sci. Rep. 7, 12240. doi: 10.1038/s41598-017-12501-5
Taberlet P., Coissac E., Hajibabaei M., Rieseberg L. H. (2012). Environmental DNA. Mol. Ecol. 21, 1789–1793. doi: 10.1111/j.1365-294X.2012.05542.x
Takahara T., Iwai N., Yasumiba K., Igawa T. (2020). Comparison of the detection of 3 endangered frog species by eDNA and acoustic surveys across 3 seasons. Freshw. Sci. 39, 18–27. doi: 10.1086/707365
Takahashi M., Saccò M., Kestel J. H., Nester G., Campbell M. A., van der Heyde M., et al. (2023). Aquatic environmental DNA: A review of the macro-organismal biomonitoring revolution. Sci. Total Environ. 873, 162322. doi: 10.1016/j.scitotenv.2023.162322
Thomsen P. F., Kielgast J., Iversen L. L., Møller P. R., Rasmussen M., Willerslev E. (2012). Detection of a diverse marine fish fauna using environmental DNA from seawater samples. PloS One 7, e41732. doi: 10.1371/journal.pone.0041732
Torres A., Fernández N., Zu Ermgassen S., Helmer W., Revilla E., Saavedra D., et al. (2018). Measuring rewilding progress. Philos. Trans. R. Soc Lond. B. Biol. Sci. 373, 20170433. doi: 10.1098/rstb.2017.0433
Torresdal J. D., Farrell A. D., Goldberg C. S. (2017). Environmental DNA detection of the golden tree frog (Phytotriades auratus) in bromeliads. PloS One 12, e0168787. doi: 10.1371/journal.pone.0168787
Valentin R. E., Fonseca D. M., Gable S., Kyle K. E., Hamilton G. C., Nielsen A. L., et al. (2020). Moving eDNA surveys onto land: Strategies for active eDNA aggregation to detect invasive forest insects. Mol. Ecol. Resour. 20, 746-755. doi: 10.1111/1755-0998.13151
van der Heyde M., Bunce M., Nevill P. (2022). Key factors to consider in the use of environmental DNA metabarcoding to monitor terrestrial ecological restoration. Sci. Total Environ. 848, 157617. doi: 10.1016/j.scitotenv.2022.157617
van der Heyde M., Bunce M., Wardell-Johnson G., Fernandes K., White N. E., Nevill P. (2020). Testing multiple substrates for terrestrial biodiversity monitoring using environmental DNA metabarcoding. Mol. Ecol. Resour. 20, 732-745. doi: 10.1111/1755-0998.13148
Villacorta-Rath C., Lach L., Andrade-Rodriguez N., Burrows D., Gleeson D., Trujillo-González A. (2022). Invasive terrestrial invertebrate detection in water and soil using a targeted eDNA approach. NeoBiota 83, 71–89. doi: 10.1101/2022.11.29.518289
Wang Z., Liu X., Liang D., Wang Q., Zhang L., Zhang P. (2023). VertU: universal multilocus primer sets for eDNA metabarcoding of vertebrate diversity, evaluated by both artificial and natural cases. Front. Ecol. Evol. 11. doi: 10.3389/fevo.2023.1164206
Wickham H. (2016). ggplot2: Elegant Graphics for Data Analysis (Switzerland: Springer-Verlag New York).
Wickham H., Averick M., Bryan J., Chang W., McGowan L. D., François R., et al. (2019). Welcome to the tidyverse. J. Open Source Software 4, 1686. doi: 10.21105/joss.01686
Wickham H., François R., Henry L., Müller K., Vaughan D. (2023a). dplyr: A Grammar of Data Manipulation (R package version 1.1.2). Available at: https://CRAN.R-project.org/package=dplyr (Accessed July 26, 2024).
Wickham H., Vaughan D., Girlich M. (2023b). tidyr: Tidy Messy Data (R package version 1.3.0). Available at: https://CRAN.R-project.org/package=tidyr (Accessed July 26, 2024).
Yan D., Mills J. G., Gellie N. J. C., Bissett A., Lowe A. J., Breed M. F. (2018). High-throughput eDNA monitoring of fungi to track functional recovery in ecological restoration. Biol. Conserv. 217, 113–120. doi: 10.1016/j.biocon.2017.10.035
Yang H., Du H., Qi H., Yu L., Hou X., Zhang H., et al. (2021). Effectiveness assessment of using riverine water eDNA to simultaneously monitor the riverine and riparian biodiversity information. Sci. Rep. 11, 24241. doi: 10.1038/s41598-021-03733-7
Yasashimoto T., Sakata M. K., Sakita T., Nakajima S., Ozaki M., Minamoto T. (2021). Environmental DNA detection of an invasive ant species (Linepithema humile) from soil samples. Sci. Rep. 11, 10712. doi: 10.1038/s41598-021-89993-9
Yates M. C., Derry A. M., Cristescu M. E. (2021). Environmental RNA: A Revolution in Ecological Resolution? Trends in Ecology and Evolution 36, 601–609. doi: 10.1016/j.tree.2021.03.001
Keywords: environmental DNA, eDNA, biodiversity monitoring, terrestrial, rewilding, DNA-based monitoring
Citation: Cowgill C, Gilbert JDJ, Convery I and Lawson Handley L (2025) Monitoring terrestrial rewilding with environmental DNA metabarcoding: a systematic review of current trends and recommendations. Front. Conserv. Sci. 5:1473957. doi: 10.3389/fcosc.2024.1473957
Received: 02 August 2024; Accepted: 27 November 2024;
Published: 02 January 2025.
Edited by:
Cristiano Vernesi, Fondazione Edmund Mach, ItalyReviewed by:
Daniel Garcia-Souto, University of Vigo, SpainAlexis Marchesini, National Research Council (CNR), Italy
Till-Hendrik Macher, University of Trier, Germany
Copyright © 2025 Cowgill, Gilbert, Convery and Lawson Handley. This is an open-access article distributed under the terms of the Creative Commons Attribution License (CC BY). The use, distribution or reproduction in other forums is permitted, provided the original author(s) and the copyright owner(s) are credited and that the original publication in this journal is cited, in accordance with accepted academic practice. No use, distribution or reproduction is permitted which does not comply with these terms.
*Correspondence: Clare Cowgill, Yy5jb3dnaWxsLTIwMjFAaHVsbC5hYy51aw==
†ORCID: James D. Gilbert, orcid.org/0000-0001-7014-2803
Ian Convery, orcid.org/0000-0003-2527-5660
Lori Lawson Handley, orcid.org/0000-0002-8153-5511
Clare Cowgill, orcid.org/0009-0005-1382-998X