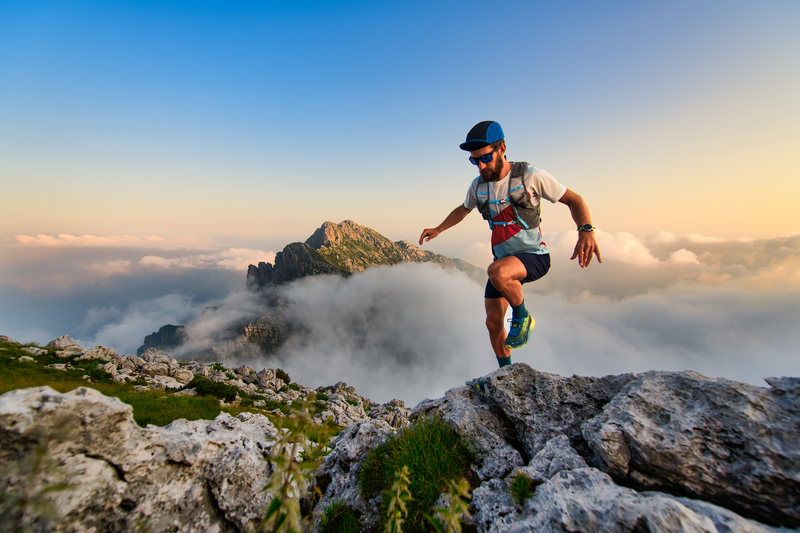
94% of researchers rate our articles as excellent or good
Learn more about the work of our research integrity team to safeguard the quality of each article we publish.
Find out more
ORIGINAL RESEARCH article
Front. Conserv. Sci. , 16 September 2024
Sec. Animal Conservation
Volume 5 - 2024 | https://doi.org/10.3389/fcosc.2024.1426646
This article is part of the Research Topic Linking Habitat Quality to Population Dynamics for Conservation Decision Making View all 10 articles
Uncertainty, complexity, and dynamic changes present challenges for conservation and natural resource management. Evidence-based approaches grounded in reliable information and rigorous analysis can enhance the navigation of the uncertainties and trade-offs inherent in conservation problems. This study highlights the importance of collaborative efforts and evidence-based decision-making, specifically implementing the Resilience-Experimentalist school of adaptive management (RE-ARM), which emphasizes stakeholder involvement, shared understanding, and experimentation. Our goal was to develop an adaptive management framework to reduce the uncertainty around the use of prescribed fire to manage the habitat for eastern black rails (Laterallus jamaicensis jamaicensis) and mottled ducks (Anas fulvigula) in saltmarshes of the Gulf of Mexico. Supported by discussions at a series of workshops, we used a value of information analysis to select a fire management hypothesis to test, developed an influence diagram to represent the system under fire management, used the influence diagram to develop a Bayesian decision network (BDN), and conducted a power analysis to guide management experiments and monitoring. Value of information analysis identified fire return interval as the critical uncertainty. Our BDN provided valuable insight into how managers believe prescribed fire influences vegetation characteristics and how vegetation influences both eastern black rail occupancy and mottled duck abundance. The results of the power analysis indicated that a standard occupancy modeling framework was more useful to compare 2- and 5-year fire return intervals for black rails than two alternative designs (removal and conditional). Our BDN can be used to predict the probability of achieving the desirable vegetative response to increase the occupancy probability of black rails and abundance of mottled ducks, and monitoring data can be used to update the BDN (learn) and improve best management practices for prescribed burns (adapt). Linking the value of information, BDNs, and power analysis enhances our understanding of the system, improves management decision-making, and builds trust among scientists, interested parties, and decision-makers. This approach lays the groundwork for knowledge co-production and adaptive management.
Conservation and natural resource management decisions are characterized by uncertainty, complexity, and dynamic change. A multitude of stressors, such as habitat loss and fragmentation, climate change, and disease, pose significant threats to wildlife. These threats are often interconnected, and their impacts can compound in unpredictable ways (NASEM, 2022), further exacerbating the challenges faced by wildlife populations (Polasky et al., 2011; Game et al., 2014). Evidence-based conservation approaches enable us to navigate the uncertainties, trade-offs, and conflicting interests inherent in these problems, ensuring that management efforts are grounded in reliable information and rigorous analysis (Nichols, 2012; Salafsky et al., 2019; Fuller et al., 2020).
Effectively tackling conservation and natural resource management issues is benefitted by collaborative efforts (Beier et al., 2017; Cundill and Fabricius, 2009; Dubois et al., 2020). Adaptive resource management (ARM; Lancia et al., 1996) is a collaborative, iterative approach to decision-making and resource management that engages scientists, interested parties, and decision-makers and aims to address complex and uncertain problems. There are two primary schools of adaptive management: Resilience-Experimentalist (RE-ARM) and Decision-Theoretic (DT-ARM; McFadden et al., 2011). The DT-ARM framework is grounded in decision-theory and focuses on defining the management problem, objectives, and alternatives with no explicit requirement for experimentation to test management actions (McFadden et al., 2011; Johnson et al., 2013). An important difference between DT-ARM and RE-ARM is that DT-ARM often frames management as a Markov decision process with a single decision-maker and uses an optimization algorithm (e.g., stochastic dynamic programming) to provide a sequence of optimal decisions (Williams, 2009), whereas RE-ARM emphasizes a general learning process that embraces uncertainty and uses the principles of experimental design as part of “learning-by-doing” (Walters and Holling, 1990; Walters, 1997). Within the RE-ARM framework, emphasis is placed on early involvement by interested parties, experimentation to test management hypotheses, and evaluation of management outcomes to create a shared understanding of system dynamics among interested parties. The collaborative learning process of the RE-ARM framework leads to improved communication and trust among participants and experiments offer distinct advantages in establishing causality and providing strong evidence for making informed decisions (Gerber et al., 2020; Ockendon et al., 2021; Caro et al., 2023).
In the context of RE-ARM and DT-ARM, value of information (VOI) can be an important tool to identify which uncertainty, if resolved, will result in greatest management benefits (Walters, 1986; Yokota and Thompson, 2004; Runge et al., 2011; Williams et al., 2011). VOI analyses compare the expected outcomes of different management actions under uncertainty with expected outcomes after resolving uncertainty. By considering the potential effects of different actions and the uncertainties associated with each, managers can evaluate the sensitivity of management outcomes to different hypotheses (Williams and Johnson, 2015; Bolam et al., 2019). This comparison helps prioritize which management actions to test experimentally within the RE-ARM framework.
Bayesian decision networks (BDNs) provide a framework for representing and reasoning about uncertainty and for explicitly representing decision alternatives and their utilities (Marcot et al., 2006; Chen and Pollino, 2012). BDNs are constructed based on a conceptual model (i.e., influence diagram) that represents causal, logical, or other relationships among variables and their dependencies. BDNs can be easily created in participatory settings, particularly with experts contributing their knowledge, insights, and diverse perspectives, thereby improving the development process and parameterization of the network (Drew and Collazo, 2012; Hassall et al., 2019; Marcot, 2019). As new data are collected, the BDN can be repeatedly updated, refined, and calibrated to improve its accuracy and relevance to decision-making (Nyberg et al., 2006). Finally, BDNs can incorporate multiple types of uncertainty relevant for adaptive management: environmental variation, structural uncertainty, partial management control, and partial observability (Williams, 1997; Lyons et al., 2008).
Power analysis assesses the potential for learning by estimating the statistical power of a monitoring design (Anderson, 1998; Steidl and Thomas, 2001; Di Stephano, 2003). Insufficient sample size can result in low power, increasing the risk of type II errors (false negatives) where true effects are not detected. Power analysis can identify the minimum sample size required to achieve a desired level of statistical power (i.e., to detect a meaningful effect) and is amenable to nearly any analytical framework including BDNs, allowing decision-makers to allocate necessary resources. Power analysis provides a quantitative measure of the ability to detect effects in adaptive management experiments—by considering statistical power, decision-makers can communicate the level of confidence in the reliability of monitoring outcomes and the effectiveness of strategies aimed at reducing structural uncertainty. Because experimentation is a fundamental aspect of the RE-ARM framework, conducting power analyses becomes an important step during experimental design to ensure an adequate amount of data is collected to facilitate learning and reduce critical uncertainties.
Here we present a case study of the setup phase for a RE-ARM framework to actively learn about and improve fire management strategies for eastern black rails (BLRA; Laterallus jamaicensis jamaicensis) and mottled ducks (MODU; Anas fulvigula) (hereafter, focal species) in U.S. Gulf of Mexico salt marshes. ARM frameworks include two distinct phases: a setup phase in which key components are developed and an iterative phase in which the components are linked together in a sequential learning and decision process (Williams et al., 2007). Our focus was on understanding and managing a dynamic ecosystem that involves interacting factors, nonlinear dynamics, and multiple decision-makers. Through experimentation, we can continuously improve our understanding of the system, refine our decision-making processes, and strengthen the ability to manage effectively. By coproducing the framework with experts, decision-makers, and other interested parties, we can harness their insights, generate shared ownership over the management strategies, and improve conservation for our focal species in the Gulf of Mexico. Throughout our case study, we show how coproduction tools from decision analysis, modeling, and monitoring can create a consortium for developing and improving evidence-based decision-advisory tools (Nichols et al., 2019).
The history of fire in salt marshes of the Gulf of Mexico has been shaped by both natural and anthropogenic causes (Nyman and Chabreck, 1995). Historically, fire was predominantly a natural occurrence, ignited by lightning strikes. These fires helped control the growth of woody vegetation, promote nutrient cycling, and create a mosaic of vegetation patches with varying stages of succession. Native Americans also had a long history of utilizing fire as a land management tool. However, during the 20th century, widespread fire suppression policies were implemented across the United States, including the Gulf of Mexico, leading to changes in ecosystem dynamics and altered natural fire regimes. Whereas there is increasing recognition of the importance of managing fire in salt marshes for conservation purposes, the effects of prescribed fire on focal species of concern, such as eastern black rails and mottled ducks, through changes to vegetation structure and composition, are unknown (Mitchell et al., 2006).
Eastern black rails are small, secretive birds that inhabit coastal wetlands, including salt marshes, in the Gulf of Mexico (Conway, 2011; Watts, 2016). They prefer dense, tall grasses, typically occupying areas with high marsh vegetation, especially where it transitions to low marsh or wet meadows, which provides cover and foraging opportunities (Roach and Barrett, 2015). Mottled ducks inhabit a variety of coastal wetland habitats in the Gulf of Mexico, including salt marshes, prairies, freshwater marshes, and estuaries. They prefer shallow marshes with dense emergent vegetation, such as cordgrass, sedges, and bulrushes, which provides important nesting and foraging areas (Bonczek and Ringelman, 2021; Haukos et al., 2010). Eastern black rails are federally threatened (USFWS, 2020), and mottled ducks are a regionally important game species (Krainyk and Ballard, 2016), with both species co-occurring in saltmarshes throughout the Gulf of Mexico. The response of eastern black rails and mottled ducks to prescribed fire in terms of habitat suitability is not fully understood.
Because co-produced science and adaptive management are collaborative- and partnership-driven, we first established a planning committee composed of decision analysts and research scientists to develop a framework that integrates diverse perspectives and expert knowledge into the decision-making process. The planning committee identified a larger multi-disciplinary team of interested parties and experts with a diversity of experiences and knowledge (prescribed fire, focal species, high marsh management, endangered species regulation, conservation, etc.) who were either decision-makers or who have an influential role within a decision-making organization. We invited these experts to an initial adaptive management virtual workshop (hosted in September 2020) where we framed the decision context and created influence diagrams to illustrate the relationships among management actions and ecological variables within the high marsh (Figure 1). Using influence diagrams allowed for communication of key uncertainties and development of multiple working hypotheses that specified a threat to the focal species, description of the demographic mechanism by which the focal species were affected, and identification of a management action that may reduce or eliminate the threat. We calculated the constructed value of information (CVOI; Runge et al., 2023), which prioritizes sources of uncertainty in management actions, such as regarding the use of prescribed fire to benefit the focal species (i.e., increase BLRA occupancy or MODU abundance). Grounded in the algebra of the expected value of perfect information (EVPI), CVOI uses ratio scales to decompose EVPI into a contribution representing the relevance of the uncertainty to the decision and a contribution representing the magnitude of uncertainty (Runge et al., 2023). CVOI scores elicited from experts were used to identify the hypothesis which, if tested, would best maximize management benefits (see Stantial et al., 2023 and Table 1).
Figure 1. The progression from the original conceptual model (A), developed during the initial adaptive management workshop, to a trimmed conceptual model (B), where node states are defined for each component, and finally to a Bayesian decision network (BDN; C) parameterized with expert-elicited conditional probabilities tables. The initial conceptual model (A) was conceived as an influence diagram during brainstorming sessions in the early stages of the project. Green rectangles represent management actions, yellow rounded rectangles represent ecological variables, red ovals represent chance events, and blue hexagons represent fundamental objectives. Arrows represent the direction of cause and effect. The conceptual model was refined and streamlined (B) through an interactive and consultative process between subject matter experts and decision analysts. Specific states were assigned to each node for clarity and precision. The streamlined conceptual model (B) was transformed into a BDN (C), incorporating expert knowledge through elicited conditional probabilities to quantify the relationships and uncertainties within the model. This progression highlights the evolution of the model from its conceptual inception to a fully specified BDN, enabling informed decision-making based on expert input and probabilistic reasoning.
Table 1. Hypotheses generated during workshop breakout group discussions about reducing uncertainty around prescribed fire decisions for eastern black rails, and mottled ducks in high marsh habitats along the Gulf of Mexico, USA.
We followed the recommendations from Marcot et al. (2006) to convert the influence diagrams from the initial workshop into a BDN. The conversion process involves three steps: the alpha-level model, initially created from influence diagrams and expert judgment; the beta-level model, revised after peer review and calibrated with case data; and the gamma-level model, finalized by updating and validating with new data for final application (Marcot et al., 2006). We developed the BLRA alpha model in a second virtual model-building workshop (hosted in 2021), where our multidisciplinary team refined the BLRA influence diagrams, removed linguistic uncertainty among node definitions, converted the influence diagram to a BDN, and contributed values for the conditional probability tables (CPT; a table that represents the hypothesized influence of each parent node on the outcomes of child nodes; Stantial and Lyons, 2024) in the network via a formal elicitation for expert judgment (Hemming et al., 2018). Following the workshop, we averaged the individual experts’ responses (Clemen and Winkler, 2007) and entered the CPT values into the alpha-level BDN in Netica® (Norsys Software Corp., www.norsys.com). Simple averaging has been shown to work well in many cases, and in this case, the experts’ opinions were closely aligned, making simple averaging an appropriate and effective method for aggregating the responses (Clemen and Winkler, 2007). In a subsequent meeting, we reviewed the alpha-level BLRA model with participants, each of the relationships were discussed, and the alpha-level BLRA model was finalized. We procured two independent reviews from BLRA experts who reviewed the model structure and CPT values and confirmed the model’s construction, which finalized the beta-level BLRA model. To create the gamma-level model, we conducted a one-way sensitivity analysis by varying one input node at a time while keeping the other input nodes constant (Conroy and Peterson, 2013). This allowed us to observe and measure the effect on the probability distribution for BLRA occupancy, evaluate the behavior of the BLRA BDN, and verify that the model’s predictions were reasonable and informed.
We followed the same process to develop the MODU BDN, again, using the guidelines from Marcot et al. (2006). We then combined the gamma-level BLRA and MODU models into a single BLRA + MODU BDN because they shared most of the same environmental forcing variables and so that we might most efficiently evaluate tradeoffs among various fire return intervals for the two species (Figure 1).
Once the gamma-level BLRA BDN was created, we hosted an interactive scenario modeling workshop (hosted in 2022) where our multidisciplinary team, other researchers, and other managers received firsthand experience running BDN scenarios of their choice in Netica with the gamma-level BLRA BDN (i.e., influence runs of Marcot, 2012). Scenario modeling involves using the BLRA BDN to simulate various management scenarios and predict their outcomes, allowing participants to explore and evaluate different strategies. Before the workshop, all participants received a copy of the model and downloaded the software to their computer so that they could conduct influence runs, which involve using the model to analyze the effects of different variables on the outcomes. We also reviewed the MODU + BLRA BDN, explored its functionalities, discussed model outputs in terms of causal and diagnostic reasoning, and discussed future steps for utilizing this model in adaptive management.
Finally, because reducing uncertainty and learning from experimental management are hallmarks of RE-ARM, we also conducted a learning simulation study to demonstrate methods for learning with a BDN. We simulated 1,000 observations of data from each node in the gamma-level BLRA model using the bnlearn package (Scutari, 2010) in R (R Core Team, 2023) under the assumption that the 2-year fire return interval would result in highest BLRA occupancy; we focused on fire return interval because it was prioritized as the critical uncertainty for managers in our value of information analysis (Stantial et al., 2023). We chose the 2-year fire return interval for demonstration purposes only; we do not draw inference about BLRA occupancy from this analysis. Rather, we consider this simulation to be one possible outcome for this study and use the analysis only to demonstrate model updating and Bayesian learning via RE-ARM. With the simulated data, we updated the gamma-level BLRA model in Netica using the expectation maximization algorithm (Do and Batzoglou, 2008). The simulated data were arranged in a spreadsheet with columns corresponding to each node in the Bayes Net and each row representing a simulated outcome (Netica refers to such a spreadsheet as “case data”). Updating the model was accomplished using Netica’s facilities for parameter learning from case data, which calculates a new conditional probability table at each node, given the model structure and the new data. To evaluate differences in the initial and updated model, we compared (1) Netica belief bars for the time since fire node, (2) the mean and SD (assuming Gaussian errors) of expected value for time since fire, and (3) the prior and posterior distributions for fire return interval, all conditional on BLRA being present.
We conducted power analyses to guide our sampling design and determine the number of sites and surveys at each site needed to ensure an acceptable level of variance by which to then confidently test the effects of various fire return intervals on MODU abundance and BLRA occupancy. Knowing that BLRA and MODU surveys would be completed simultaneously under the same study design, we focused our power analyses on BLRA, which is a rare and cryptic species and occupancy estimates are often inaccurate for rare and cryptic species (Tolliver et al., 2019; Guillera-Arroita et al., 2014, 2010). We simulated BLRA occupancy data assuming an average occupancy probability of 0.30 and detection probability of 0.10, which may be conservative. Mean occupancy probability for the Gulf coast region ranges from 0.09 to 0.75, and detection probability ranges from 0.11 to 0.28 (McGowan et al., 2020a; Butler et al., 2023). Using the framework from Specht et al. (2017), we evaluated various sampling designs (standard, conditional, and removal) and effect sizes (small, medium, or large; Cohen, 1988) to determine the power to detect a difference between the null (no difference between 2- and 5-year fire return intervals) and fire treatment (difference between 2- and 5-year fire return intervals) models. The standard design involves multiple visits to all sites, the removal design ceases visits after initial detection, and the conditional design focuses follow-up efforts only on sites where the species was detected initially, each optimizing resource use based on species detectability and rarity (Specht et al., 2017). According to Cohen (1988), small, medium, and large effect sizes correspond to standardized differences (d) of 0.2, 0.5, and 0.8, respectively, representing small detectable, moderate visible, and substantial noticeable differences between groups. These standardized differences, or effect sizes, measure the magnitude of difference between groups in a standardized way, allowing comparisons across different studies and variables (Cohen, 1988). Using the results of the power analysis, we finalized the sampling design through consensus among a small team of experts, who evaluated the implications of different study designs and power thresholds on our study’s objectives and resource constraints. The study design will be used to guide the iterative phase of adaptive management.
Using their influence diagrams, the participants generated nine management hypotheses at the initial workshop (Table 1; see also Table 1 from Stantial et al., 2023). The hypothesis with the greatest value of information for decision-making based on the results of the CVOI analysis was the Fire Return Interval hypothesis (Table 1, #8). This hypothesis was chosen for experimentation within a RE-ARM framework, aiming to expedite the learning process regarding the effects of fire frequency on the focal species.
During the second workshop, we finalized the BLRA conceptual model, converted the BLRA conceptual model into a BDN, and parameterized the BLRA BDN through expert elicitation to create the alpha-level BLRA BDN model (Figure 1). The alpha-level BLRA BDN comprised a burn decision node, six ecological variable nodes, one species occupancy node (Table 2), and the causal relationships among these components (as shown in Figure 1). The fully parameterized, alpha-level BLRA BDN was the average of the individual expert-elicited conditional probabilities (n = 20). The average probability of BLRA occupancy being present across all ecological states and management decisions from the expert-elicited conditional probabilities was 29.7%.
Table 2. Bayesian decision network (BDN) nodes, node states, and definitions for the adaptive management BDN used to evaluate the effects of varying intervals of prescribed fire on eastern black rails and mottled ducks in the Gulf of Mexico.
Through demonstrations and discussions during our interactive scenario modeling workshop, the attendees gained a thorough understanding of BDNs, the importance of various habitat variables on BLRA occupancy and MODU abundance, and the predictive capabilities of these networks in assessing the ecological impacts of varying fire frequencies on BLRA and MODU habitat. The participants also gained a thorough understanding of the practical application of BDNs in adaptive management.
During the final workshop, we focused on finalizing the MODU conceptual model, converted the MODU conceptual model into a BDN, and parameterized the MODU BDN through expert elicitation. The final MODU BDN was composed of a burn decision node, seven ecological variable nodes (six from the BLRA BDN and one specific to MODU), one species abundance node, and the causal relationships among these components (Table 2). The fully parameterized MODU BDN was the average (n = 19) of expert-elicited conditional probabilities. The mean probability of high MODU abundance was 25%, while low abundance had a mean probability of 40%, and none had a mean probability of 35%.
The results of our one-way sensitivity analysis with the gamma-level BLRA + MODU BDN suggested that the node most influential to BLRA occupancy was the herbaceous vegetation node whereas the annual precipitation node was the least influential (Figure 2A). The node most influential to MODU abundance was the proximity to brood rearing habitat whereas the annual precipitation node was the least influential (1.7%; Figure 2B). The learning simulation study explored a scenario in which BLRA are found most often in management units with a 2-year fire return interval. In the initial model parameterized with expert judgment, the belief bars for the time since fire node are nearly equal and reflect managers’ uncertainty about the effect of fire return interval (Figure 3A). The mean time since fire in this model was 4.8 growing seasons (SD = 2.4). In the updated model, the belief bars are unequal and reflect evidence, as expected, that BLRA are found more often in the 2-year return interval (Figure 3B). The mean time since fire in the updated model was 2.8 growing seasons (SD = 1.3). The posterior distribution for time since fire (updated model) shifted as expected and showed smaller variance compared to the prior distribution (initial model; Figure 3C).
Figure 2. One-way sensitivity analysis depicting the sensitivity of the eastern black rail (BLRA) occupancy (A) and mottled duck (MODU) abundance (B) nodes to changes in their respective parent nodes within the Bayesian decision network (BDN). This analysis illustrates the extent to which variations in the parent nodes influence the outcomes of BLRA occupancy and MODU abundance, providing insights into the relative sensitivity of these key nodes in the BDN. Vertical lines in both panels indicate average model probability for BLRA presence (0.297) and high MODU abundance (0.253). The horizontal bars represent the maximum and minimum values when manipulating the parent node (y-axis) of the BDN.
Figure 3. Learning (reducing uncertainty) about fire management with a Bayesian decision network (BDN) for eastern black rails. (A) The initial BDN parameterized with expert judgment shows high uncertainty about the effect of time since fire when eastern black rails are present (nearly equal belief bars for two, five, and 8+ growing seasons in time since fire node). (B) In the updated model after learning from simulated monitoring data, the belief bars reflect accumulating evidence that BLRAs prefer a fire return interval of two growing seasons (demonstration purposes only). (C) Prior (initial model) and posterior (updated) distributions for time since fire conditional on rails being present. The reduction in uncertainty (learning) is evident in the shift of the distribution and smaller standard deviation.
The results of the power analysis for BLRA occupancy revealed no differences between the standard, conditional, and removal sampling designs (Figure 4). We found that if the effect size is small, no matter the sampling design or number of surveys, we will have low power to detect a difference between the fire treatments. Our results suggest that if the effect size is medium or high, surveying 375 points (S) eight times (k) under the standard sampling design, we will have a high power (>75%) to detect a difference between the null and fire treatment models.
Figure 4. Power analysis for three different sampling designs for monitoring eastern black rail occupancy. The panels (gray bars at the top) represent total effort (number of surveys across all sites). Power to detect the fire treatment effect (y-axis) was estimated across different standardized effect sizes (x-axis) categorized as small (0.2), medium (0.5), and large (0.8) according to Cohen (1988). The shapes indicate the number of site visits (k) required. The top row represents the optimal number of surveys from Specht et al. (2017) (i.e., the number of survey repetitions at each site that will maximize the accuracy and precision of occupancy and detection probability estimates, based on the expected occupancy and detection probabilities), and the bottom row represents half the optimal number of site visits (half optimal = k/2). Additionally, sampling designs are represented by different colors.
Our value of information (VOI) analysis identified that reducing uncertainty about the effect of fire return interval will provide the greatest management benefit to BLRAs and MODUs in high marshes of the Gulf of Mexico. Addressing the remaining uncertainty could help managers better understand ecological processes, balance the trade-offs between species, allocate resources more efficiently, and prioritize areas for burning—for example, burning too frequently may be counterproductive because vegetation might not have time to recover, leading to increased rates of soil erosion; however, not burning frequently enough might reduce habitat quality by leading to woody vegetation encroachment that provides little structural cover for the focal species. Regular, well-timed prescribed fires can sustain the diverse vegetation relying on these fire-adapted ecosystems, especially in the high marsh (Allain and Grace, 2001; Lynch, 1941; Nyman and Chabreck, 1995). Relative to the other hypotheses, the Fire Return Interval hypothesis was considered the most pertinent because it addressed a fundamental aspect of habitat management with high potential for improving ecological understanding and practical management outcomes for the focal species in Gulf of Mexico high marshes.
BDNs offer multiple advantages as a modeling framework for adaptive management. In our case study, BDNs allowed us to intricately map and analyze the complex interactions between fire management (fire return intervals), habitat variables, and BLRA occupancy and MODU abundance. Their graphical nature, with boxes and arrows showing influence, is intuitive, improving communication during co-production because participants with a wide variety of knowledge, insights, and perspectives can more clearly define, visualize, and discuss variables and their dependencies (Carriger and Newman, 2012; Robinson and Fuller, 2017). In this case, the participants were able to gain a thorough understanding of the complex relationships between management actions and ecological components of Gulf of Mexico high marshes through a hands-on learning demonstration of the BDN. It is also possible to parameterize a BDN with formal elicitation of expert judgment when data are lacking (Drew and Collazo, 2012; Marcot, 2012; Hassall et al., 2019); the expert elicitation of conditional probability tables for the Gulf of Mexico BDN allowed a diverse set of experts to contribute their expertise, facilitating shared ownership of the modeling effort. As new data are collected, the BDN structure and probability parameters can be iteratively updated, refined, and calibrated to improve its accuracy and relevance to decision-making (Nyberg et al., 2006). Finally, resilience thinking places high value on understanding consequences of uncertainty for system behavior and management outcomes (Chapin et al., 2009; Polasky et al., 2011). Our BDN incorporates multiple types of uncertainty important for adaptive management (Williams, 2009; Lyons et al., 2008). First, the probabilistic relationships between the burn decision node and vegetation (child) nodes can help managers reduce uncertainty from partial management control; a burn decision does not always result in the same vegetation conditions, and as the CPTs for the vegetation nodes evolve over time based on field data, managers could gain insight about management control. Second, uncertainty related to environmental variation, while not reducible, is incorporated in the BDN with the precipitation node. Third, structural (model) uncertainty, including parametric uncertainty, can be understood and reduced over time via learning from case data and structural learning algorithms, e.g. those available in the R package bnlearn (Scutari, 2010).
Power analysis is a foundational component to any adaptive management program because it determines the sample size needed to detect statistically significant differences between models, guiding efficient resource allocation and ensuring that monitoring efforts yield reliable data (Williams et al., 2007). Our finding that there is no difference between standard, conditional, and removal sampling designs helps streamline the monitoring process by indicating that the typical, standard sampling design may be as effective as other designs. This is particularly advantageous because, at all of our survey locations, we will also be surveying for mottled ducks. Given the challenges of detecting BLRA, if one of the sampling designs had shown a substantial advantage in power to detect differences, we might have considered adapting our approach to accommodate that design. However, since none of the designs offer a significant advantage, it is more efficient and practical to use the standard sampling design for both species, allowing us to survey for mottled ducks and black rails at the same sites using the same sampling design. The identification of a threshold effect size for detecting meaningful differences underscores the necessity of designing studies with sufficient power. If the effect size is low, a much larger sample size will be necessary to reliably discern between the effects of 2- and 5-year fire return intervals. Therefore, power analysis has been critical to ensuring that the design of our experiment is robust enough to identify differences between fire return intervals that are meaningful for management decisions.
We parameterized the BDN for BLRAs and MODUs using expert elicitation, gathering conditional probability estimates from a panel of experts. The resulting parameterized BDN represented the mean expert-elicited conditional probabilities. Notably, the probability of BLRA being present, as derived from the expert data, was 30%. This finding closely aligns with published literature for Gulf of Mexico BLRA, where the average occupancy is reported to be around 30% (Tolliver et al., 2019). Such agreement between the expert-elicited data and the literature values is encouraging, indicating that the panel of experts provided reasonable and informed estimates for the conditional probability tables for each of the nodes in our BDN. This agreement not only validates the value of expert elicitation in parameterizing BDNs but also underscores the value of integrating expert judgment with empirical data to enhance the accuracy and reliability of modeling efforts (O’Hagan et al., 2006; Martin et al., 2012).
Implementation of adaptive management, whether RE-ARM or DT-ARM, includes two phases: a setup phase to identify key components and an iterative phase in which the components are linked together in a sequential learning and decision process (Williams et al., 2007). Our RE-ARM setup phase created the framework for collecting and analyzing data to reduce multiple types of uncertainty about the effect of prescribed fire in high marsh ecosystems. As our RE-ARM setup phase concludes, the iterative phase will allow for refining and adapting management strategies based on new knowledge, monitoring data, and interested parties’ input. Data collected during the iterative phase can be readily incorporated into the BDN (Nyberg et al., 2006), enabling us to improve the model outputs, gain insight into system dynamics, and evaluate the effects of different fire return intervals on the focal species (i.e., learn). In other words, the iterative phase involves updating the model based on the collected data, laying the foundation for evidence-based decision-making in the future. As new knowledge about system dynamics accrues over time, it may be helpful to revisit the objectives, actions, and BDN as part of a “double-loop learning” process (Williams and Brown, 2018; McGowan et al., 2020b). Through double-loop learning, continuous improvements can be made, contributing to the conservation of the focal species and restoration of ecological balance within Gulf of Mexico high marshes.
Collaborative model co-production with participants was critical to improving the overall understanding of our data collection and helped to identify additional state variables of interest in our model. Participatory modeling thus resulted in robust monitoring protocols, ensuring that our data collection methodologies aligned with the hypotheses they are meant to inform. Moreover, the model-building process identified additional monitoring endpoints for “umbrella learning,” where auxiliary data collected during the iterative phase could also be extended to test other hypotheses. This collaborative strategy, driven by the co-creation of models and monitoring (Nichols, 2012; Nichols et al., 2019), has allowed valuable insights for our conservation and resource management initiatives for the focal species in this study.
Protecting species and restoring ecosystems necessitates informed decision-making based on reliable scientific knowledge. This study underscores the value of evidence-based decision-making in addressing the biodiversity crisis and highlights the importance of tools such as VOI analysis, BDNs, and power analysis in a RE-ARM framework to produce reliable and actionable science for adaptive management.
The original contributions presented in the study are publicly available. This data can be found here: https://www.sciencebase.gov/catalog/item/66a14f72d34ec831f2c2b50c (Stantial and Lyons, 2024).
MS: Conceptualization, Data curation, Formal Analysis, Investigation, Methodology, Validation, Visualization, Writing – original draft, Writing – review & editing. AF: Funding acquisition, Investigation, Supervision, Writing – review & editing. AL: Conceptualization, Investigation, Methodology, Writing – review & editing. BM: Methodology, Validation, Writing – review & editing. MW: Funding acquisition, Investigation, Supervision, Writing – review & editing. JL: Conceptualization, Funding acquisition, Investigation, Methodology, Project administration, Resources, Supervision, Validation, Visualization, Writing – review & editing.
The author(s) declare financial support was received for the research, authorship, and/or publication of this article. Funding was provided by the National Oceanic and Atmospheric Administration’s RESTORE Science Program under award NA19NOS4510195 to the U.S. Geological Survey.
We are grateful to all workshop participants who contributed expertise and made co-production possible. We thank Harrison Goldspiel for assisting with workshops. We thank Alix Godar for helpful discussions during the model building phase.
The authors declare that the research was conducted in the absence of any commercial or financial relationships that could be construed as a potential conflict of interest.
All claims expressed in this article are solely those of the authors and do not necessarily represent those of their affiliated organizations, or those of the publisher, the editors and the reviewers. Any product that may be evaluated in this article, or claim that may be made by its manufacturer, is not guaranteed or endorsed by the publisher.
Any use of trade, firm, or product names is for descriptive purposes only and does not imply endorsement by the U.S. Government.
Allain L. K., Grace J. B. (2001). “Changes in density and height of the shrub baccharis halimifolia following burning in coastal tallgrass prairie,” in Proceedings of the 17th North American Prairie Conference, Mason City, IA. 66–72, North Iowa Area Community College.
Anderson J. L. (1998). “Errors of inference,” in Statistical Methods for Adaptive Management Studies Land Management Handbook #42. Eds. Sit V., Taylor B. (British Columbia, Ministry of Forests, Research Branch, Victoria, British Columbia, Canada).
Beier P., Hansen L. J., Helbrecht L., Behar D. (2017). A how-to guide for coproduction of actionable science: coproducing actionable science. Conserv. Lett. 10, 288–296. doi: 10.1111/conl.12300
Bolam F. C., Grainger M. J., Mengersen K. L., Stewart G. B., Sutherland W. J., Runge M. C., et al. (2019). Using the Value of Information to improve conservation decision making. Biol. Rev. 94, 629–647. doi: 10.1111/brv.12471
Bonczek E. S., Ringelman K. M. (2021). Breeding ecology of mottled ducks: A review. J. Wildlife Manage. 85, 825–837. doi: 10.1002/jwmg.22048
Butler C. J., Tibbits J. B., Wilson J. K. (2023). Black rail occupancy and detectability in the texas mid-coast national wildlife refuge. Waterbirds 46, 1–12. doi: 10.1675/063.046.0102
Caro T., Andrews J., Clark M., Borgerhoff Mulder M. (2023). Practical guide to coproduction in conservation science. Conserv. Biol. 37, e14011. doi: 10.1111/cobi.14011
Carriger J. F., Newman M. C. (2012). Evaluation of stressor interactions in aquatic systems using bayesian networks. Environ. Sci. Technol. 46, 1203–1210. doi: 10.1002/ieam.268
Chapin F. S., Kofinas G. P., Folke C. (Eds.) (2009). Principles of Ecosystem Stewardship: Resilience-Based Natural Resource Management in a Changing World. 1st ed (New York: Springer).
Chen S. H., Pollino C. A. (2012). Good practice in Bayesian network modelling. Environ. Model. Software 37, 134–145. doi: 10.1016/j.envsoft.2012.03.012
Clemen R. T., Winkler R. L. (2007). “Aggregating probability distributions,” in Advances in Decision Analysis: From Foundations to Applications. Eds. Edwards W., Miles R. F. Jr, von Winterfeldt D. (Cambridge University Press, Cambridge), 154–176. doi: 10.1017/CBO9780511611308.010
Cohen J. (1988). Statistical Power Analysis for the Behavioral Sciences. 2. ed (New York, NY: Psychology Press).
Conroy M. J., Peterson J. T. (2013). Decision Making in Natural Resource Management: A Structured, Adaptive Approach (Hoboken, NJ: Wiley).
Conway C. J. (2011). Standardized North American marsh bird monitoring protocol. Waterbirds 34, 319–346. doi: 10.1675/063.034.0307
Cundill G., Fabricius C. (2009). Monitoring in adaptive co-management: Toward a learning based approach. J. Environ. Manage. 90, 3205–3211. doi: 10.1016/j.jenvman.2009.05.012
Do C. B., Batzoglou S. (2008). What is the expectation maximization algorithm? Nat. Biotechnol. 26, 897–899. doi: 10.1038/nbt1406
Drew C. A., Collazo J. A. (2012). “Expert knowledge as a foundation for the management of secretive species and their habitat,” in Expert Knowledge and Its Application in Landscape Ecology. Eds. Perera A. H., Drew C. A., Johnson C. J. (Springer New York, New York, NY), 87–107. doi: 10.1007/978-1-4614-1034-8_5
Dubois N. S., Gomez A., Carlson S., Russell D. (2020). ). Bridging the research-implementation gap requires engagement from practitioners. Conserv. Sci. Pract. 2, e134. doi: 10.1111/csp2.134
Fuller A. K., Decker D. J., Schiavone M. V., Forstchen A. B. (2020). Ratcheting up rigor in wildlife management decision making. Wildlife Soc. Bull. 44, 29–41. doi: 10.1002/wsb.1064
Game E. T., Meijaard E., Sheil D., McDonald-Madden E. (2014). Conservation in a wicked complex world; challenges and solutions. Conserv. Lett. 7, 271–277. doi: 10.1111/conl.12050
Gerber L. R., Barton C. J., Cheng S. H., Anderson D. (2020). Producing actionable science in conservation: Best practices for organizations and individuals. Conserv. Sci. Pract. 2, e295. doi: 10.1111/csp2.295
Guillera-Arroita G., Lahoz-Monfort J. J., MacKenzie D. I., Wintle B. A., McCarthy. M. A. (2014). Ignoring imperfect detection in biological surveys is dangerous: A response to ‘Fitting and interpreting occupancy models’. PloS One 9, e99571. doi: 10.1371/journal.pone.0099571
Guillera-Arroita G., Ridout M. S., Morgan. B. J. T. (2010). Design of occupancy studies with imperfect detection. Methods Ecol. Evol. 1, 131–139. doi: 10.1111/j.2041-210X.2010.00017.x
Hassall K. L., Dailey G., Zawadzka J., Milne A. E., Harris J. A., Corstanje R., et al. (2019). Facilitating the elicitation of beliefs for use in Bayesian Belief modelling. Environ. Model. Software 122, 104539. doi: 10.1016/j.envsoft.2019.104539
Haukos D., Martinez S., Heltzel. J. (2010). Characteristics of ponds used by breeding mottled ducks on the chenier plain of the texas gulf coast. J. Fish Wildlife Manage. 1, 93–101. doi: 10.3996/032010JFWM-005
Hemming V., Burgman M. A., Hanea A. M., McBride M. F., Wintle. B. C. (2018). A practical guide to structured expert elicitation using the IDEA protocol. Methods Ecol. Evol. 9, 169–180. doi: 10.1111/2041-210X.12857
Johnson F. A., Williams B. K., Nichols J. D. (2013). Resilience thinking and a decision-analytic approach to conservation: strange bedfellows or essential partners? Ecol. Soc. 18, 27. doi: 10.5751/ES-05544-180227
Krainyk A., Ballard B. M. (2016). Guiding Mottled Duck Habitat Conservation in the 21st Century (Kingsville, TX: Caesar Kleberg Wildlife Research Institute). Available at: https://www.ckwri.tamuk.edu/sites/default/files/news/pdfs/2022/20160616_mottled_duck_twa_4.16.pdf.
Lancia R. A., Braun C. E., Collopy M. W., Dueser R. D., Kie J. G., Martinka C. J., et al. (1996). ARM! for the future: adaptive resource management in the wildlife profession. Wildlife Soc. Bull. 24, 436–442.
Lynch J. J. (1941). The place of burning in management of the gulf coast wildlife refuges. J. Wildlife Manage. 5, 454–457. doi: 10.2307/3795690
Lyons J. E., Runge M. C., Laskowski H. P., Kendall W. L. (2008). Monitoring in the context of structured decision-making and adaptive management. J. Wildlife Manage. 72, 1683–1692. doi: 10.2193/2008-141
Marcot B. G. (2012). Metrics for evaluating performance and uncertainty of Bayesian network models. Ecol. Model. 230, 50–62. doi: 10.1016/j.ecolmodel.2012.01.013
Marcot B. G. (2019).Causal modeling and the role of expert knowledge. In: Quantitative Analyses in Wildlife Science (Baltimore, MD: Johns Hopkins University Press). Available online at: http://www.plexusowls.com/PDFs/JHUP_Chap_16_Causal_Modeling.pdf (Accessed August 27, 2021).
Marcot B. G., Steventon J. D., Sutherland G. D., McCann R. K. (2006). Guidelines for developing and updating Bayesian belief networks applied to ecological modeling and conservation. Can. J. For. Res. 36, 3063–3074. doi: 10.1139/x06-135
Martin T. G., Burgman M. A., Fidler F., Kuhnert P. M., Low-Choy S., McBride M., et al. (2012). Eliciting expert knowledge in conservation science. Conserv. Biol. 26, 29–38. doi: 10.1111/j.1523-1739.2011.01806.x
McFadden J. E., Hiller T. L., Tyre A. J. (2011). Evaluating the efficacy of adaptive management approaches: Is there a formula for success? J. Environ. Manage. 92, 1354–1359. doi: 10.1016/j.jenvman.2010.10.038
McGowan C. P., Angeli N. F., Beisler W. A., Snyder C., Rankin N. M., Woodrow J. O., et al. (2020a). Linking monitoring and data analysis to predictions and decisions for the range-wide eastern black rail status assessment. Endangered Species Res. 43, 209–222. doi: 10.3354/esr01063
McGowan C. P., Lyons J. E., Smith D. R. (2020b). “Decision implementation and the double-loop process in adaptive management of horseshoe crab harvest in Delaware Bay,” in Structured Decision Making: Case Studies in Natural Resources Management. Eds. Runge M. C., Converse S. J., Lyons J. E., Smith D. R. (Johns Hopkins University Press, Baltimore, MD), 258–268.
Mitchell L., Gabrey S., Marra P., Erwin R. (2006). Impacts of marsh management on coastal-marsh bird habitats. Stud. Avian Biol. 32, 155–175.
NASEM (2022). Resilience for Compounding and Cascading Events (Washington, D.C: The National Academies Press). National Academies of Sciences, Engineering, and Medicine.
Nichols J., Kendall W., Boomer G. (2019). Accumulating evidence in ecology: Once is not enough. Ecol. Evol. 9, 13991–14004. doi: 10.1002/ece3.5836
Nichols J. D. (2012). Evidence, models, conservation programs and limits to management: Evidence, models, conservation programs and limits to management. Anim. Conserv. 15, 331–333. doi: 10.1111/j.1469-1795.2012.00574.x
Nyberg J. B., Marcot B. G., Sulyma R. (2006). Using Bayesian belief networks in adaptive management. Can. J. For. Res. 36, 3104–3116. doi: 10.1139/x06-108
Nyman J. A., Chabreck R. H. (1995). “Fire in coastal marshes: history and recent concerns,” in Fire in Wetlands: A Management Perspective (Tallahassee, FL: Tall Timbers Research Inc.) vol. 19.
O’Hagan A., Buck C. E., Daneshkhah A., Eiser J. R., Garthwaite P. H., Jenkinson D. J., et al. (2006). Uncertain Judgements: Eliciting Experts' Probabilities (Chichester, UK: John Wiley & Sons).
Ockendon N., Amano T., Cadotte M., Downey H., Hancock M. H., Thornton A., et al. (2021). Effectively integrating experiments into conservation practice. Ecol. Solutions Evidence 2, e12069. doi: 10.1002/2688-8319.12069
Polasky S., Carpenter S. R., Folke C., Keeler B. (2011). Decision-making under great uncertainty: environmental management in an era of global change. Trends Ecol. Evol. 26, 398–404. doi: 10.1016/j.tree.2011.04.007
R Core Team (2023). R: A Language and Environment for Statistical Computing (Vienna, Austria: R Foundation for Statistical Computing). Available at: https://www.R-project.org/.
Roach N. S., Barrett K. (2015). Managed habitats increase occupancy of black rails (Laterallus jamaicensis) and may buffer impacts from sea level rise. Wetlands 35, 1065–1076. doi: 10.1007/s13157-015-0695-6.greg
Robinson D. W., Fuller T. (2017). Human-centered design for complex systems: Co-evolution of problem-solving approaches and representation techniques. Syst. Eng. 20, 329–340. doi: 10.1007/978-3-319-25053-3_5
Runge M. C., Converse S. J., Lyons J. E. (2011). Which uncertainty? Using expert elicitation and expected value of information to design an adaptive program. Biol. Conserv. 144, 1214–1223. doi: 10.1016/j.biocon.2010.12.020
Runge M. C., Rushing C. S., Lyons J. E., Rubenstein M. A. (2023). A simplified method for value of information using constructed scales. Decision Anal. 20, 220–230. doi: 10.1287/deca.2023.0474
Salafsky N., Boshoven J., Burivalova Z., Dubois N. S., Gomez A., Johnson A., et al. (2019). Defining and using evidence in conservation practice. Conserv. Sci. Pract. 1, e27. doi: 10.1111/csp2.27
Scutari M. (2010). Learning Bayesian networks with the bnlearn R package. J. Stat. Soft 35, 1–22. doi: 10.18637/jss.v035.i03
Specht H. M., Reich H. T., Iannarilli F., Edwards M. R., Stapleton S. P., Weegman M. D., et al. (2017). Occupancy surveys with conditional replicates: an alternative sampling design for rare species. Methods Ecol. Evol. 8, 1725–1734. doi: 10.1111/2041-210X.12842
Stantial M. L., Lawson A. J., Fournier A. M. V., Kappes P. J., Kross C. S., Runge M. C., et al. (2023). Qualitative value of information provides a transparent and repeatable method for identifying critical uncertainty. Ecol. Appl. 33, e2824. doi: 10.1002/eap.2824
Stantial M. L., Lyons J. E. (2024). Expert Judgment Data for a Bayesian Decision Network of Prescribed Fire in Gulf of Mexico Marshes (Reston, VA: U.S. Geological Survey data release). doi: 10.5066/P13HKWUT
Steidl R. J., Thomas L. (2001).Power analysis and experimental design. In: Design and Analysis of Ecological Experiments (New York: Oxford University Press). Available online at: http://www.lenthomas.org/papers/SteidlDAEE2001.pdf (Accessed May 27, 2021).
Tolliver J. D.M., Moore A. A., Green M.C., Weckerly F. W. (2019). Coastal Texas black rail population states and survey effort. J. Wildlife Manage. 83, 312–324. doi: 10.1002/jwmg.21589
USFWS (2020). Endangered and Threatened Wildlife and Plants; Threatened Species Status for Eastern Black Rail with a Section 4(d) Rule. Available online at: https://www.govinfo.gov/content/pkg/FR-2020-10-08/pdf/2020-19661.pdf (Accessed February 28, 2021).
Walters C. (1997). Challenges in adaptive management of riparian and coastal ecosystems. Conserv. Ecol. 1, 1. doi: 10.5751/CE-00026-010201
Walters C. J., Holling C. S. (1990). Large-scale management experiments and learning by doing. Ecology 71, 2060–2068. doi: 10.2307/1938620
Watts B. D. (2016). Status and Distribution of the Eastern Black Rail along the Atlantic and Gulf Coasts of North America (Williamsburg, VA: William & Mary, Center for Conservation Biology). Available at: https://scholarworks.wm.edu/cgi/viewcontent.cgi?article=1314&context=ccb_reports.
Williams B. K. (1997). Approaches to the management of waterfowl under uncertainty. Wildlife Soc. Bull. 25, 714–720.
Williams B. K. (2009). Markov decision processes in natural resources management: Observability and uncertainty. Ecol. Model. 220, 830–840. doi: 10.1016/j.ecolmodel.2008.12.023
Williams B. K., Brown E. D. (2018). Double-loop learning in adaptive management: the need, the challenge, and the opportunity. Environ. Manage. 62, 995–1006. doi: 10.1007/s00267-018-1107-5
Williams B. K., Eaton M. J., Breininger. D. R. (2011). Adaptive resource management and the value of information. Ecol. Model. 222, 3429–3426. doi: 10.1016/j.ecolmodel.2011.07.003
Williams B. K., Johnson F. A. (2015). Value of information and natural resources decision-making. Wildlife Soc. Bull. 39, 488–496. doi: 10.1002/wsb.575
Williams B. K., Szaro R. C., Shapiro C. D. (2007). Adaptive Management: The U.S. Department of the Interior Technical Guide (Washington, D.C: U.S. Department of the Interior).
Keywords: adaptive management, Bayesian decision network, co-production, marsh birds, power analysis, structured decision making, value of information
Citation: Stantial ML, Fournier AMV, Lawson AJ, Marcot BG, Woodrey MS and Lyons JE (2024) RE-ARMing salt marshes: a resilience-experimentalist approach to prescribed fire and bird conservation in high marshes of the Gulf of Mexico. Front. Conserv. Sci. 5:1426646. doi: 10.3389/fcosc.2024.1426646
Received: 01 May 2024; Accepted: 19 August 2024;
Published: 16 September 2024.
Edited by:
David R. Breininger, University of Central Florida, United StatesReviewed by:
Ellie Brown, Science and Decisions Center (USGS), United States Department of the Interior, United StatesCopyright © 2024 Stantial, Fournier, Lawson, Marcot, Woodrey and Lyons. This is an open-access article distributed under the terms of the Creative Commons Attribution License (CC BY). The use, distribution or reproduction in other forums is permitted, provided the original author(s) and the copyright owner(s) are credited and that the original publication in this journal is cited, in accordance with accepted academic practice. No use, distribution or reproduction is permitted which does not comply with these terms.
*Correspondence: Michelle L. Stantial, bWljaGVsbGUuc3RhbnRpYWxAZ21haWwuY29t
Disclaimer: All claims expressed in this article are solely those of the authors and do not necessarily represent those of their affiliated organizations, or those of the publisher, the editors and the reviewers. Any product that may be evaluated in this article or claim that may be made by its manufacturer is not guaranteed or endorsed by the publisher.
Research integrity at Frontiers
Learn more about the work of our research integrity team to safeguard the quality of each article we publish.