- 1Department of Ecology and Evolutionary Biology, University of California Los Angeles, Los Angeles, CA, United States
- 2Stillwater Sciences, Los Angeles, CA, United States
- 3The Rocky Mountain Biological Laboratory, Crested Butte, CO, United States
Outdoor recreation benefits local economies, environmental education, and public health and wellbeing, but it can also adversely affect local ecosystems. Human presence in natural areas alters feeding and reproductive behaviors, physiology, and population structure in many wildlife species, often resulting in cascading effects through entire ecological communities. As outdoor recreation gains popularity, existing trails are becoming overcrowded and new trails are being built to accommodate increasing use. Many recreation impact studies have investigated effects of the presence or absence of humans while few have investigated recreation effects on wildlife using a gradient of disturbance intensity. We used camera traps to quantify trail use by humans and mid- to large-sized mammals in an area of intense outdoor recreation–the Upper East River Valley, Colorado, USA. We selected five trails with different types and intensities of human use and deployed six cameras on each trail for five weeks during a COVID-enhanced 2020 summer tourism season. We used occupancy models to estimate detectability and habitat use of the three most common mammal species in the study area and determined which human activities affect the habitat use patterns of each species. Human activities affected each species differently. Mule deer (Odocoileus hemionus) tended to use areas with more vehicles, more predators, and greater distances from the trailhead, and they were more likely to be detected where there were more bikers. Coyotes (Canis latrans) and red foxes (Vulpes vulpes) were most likely to use areas where their prey species occurred, and foxes were more likely to be detected where the vegetation was shorter. Humans and their recreational activities differentially influence different species. More generally, these results reinforce that it is unlikely that a single management policy is suitable for all species and management should thus be tailored for each target species.
1 Introduction
An essential question for biodiversity conservation is the effectiveness of different types of protected areas for conserving biodiversity (Sutherland et al., 2009). Protected areas are often multipurpose, with humans using them in many ways, including for recreation. Although likely beneficial for human health, the majority of outdoor recreation activities can have negative effects on wildlife, across taxa and environments (Larson et al., 2016; Baker and Leberg, 2018; Ladle et al., 2019). Human recreational activities can change animal behaviors, as well as the physical aspects of their habitats, which in turn can impact the population and community dynamics of entire ecosystems (Caravaggi et al., 2017; Suraci et al., 2019a). Protected areas globally receive billions of visits per year (Balmford et al., 2015), and as human population grows and recreation in natural areas around the globe becomes more popular, overcrowding can occur (Boué, 2019). For example, recreation visits increased by about 200 percent across Colorado, USA in early 2020 (Kwak-Hefferan, 2020), and this is projected to continue to increase in the United States (Bowker et al., 2012). This increased visitation has the potential to exacerbate the negative effects of recreation.
Trails are an especially impactful way in which human recreation leads to physical modification of habitat, with concomitant effects on wildlife. Trampling by humans can alter the physical properties of soil, leading to changes in the development and diversity of vegetation (Cole and Landres, 1995). Changes in habitat can alter community composition because some species are more resistant or resilient to change than others (Cole and Landres, 1995), and habitat fragmentation can disrupt some animals’ dispersal behaviors (Caravaggi et al., 2017). Human presence on trails has an even greater effect than habitat modification on biodiversity (Bötsch et al., 2018; Doherty et al., 2021). High human use on trails has been observed to deter some species, especially diurnal ones, and attract others that can become habituated to human presence (Erb et al., 2012). Off-trail travel and sometimes (but not always) anthropogenic noises are two of the main causes of negative impacts on wildlife (Larson et al., 2016; Bastone, 2019). Anthropogenic disturbance can have negative effects on animal behavior, such as increased vigilance (Ciuti et al., 2012). But animals may also take advantage of human activity to escape predators (Caravaggi et al., 2017). New trails are being built to accommodate for their increasing popularity (Bastone, 2019), which makes better understanding their effects on wildlife increasingly important.
Fear of humans as predators alters animals’ feeding behaviors (Frid and Dill, 2002), potentially leading to ecological cascades (Smith et al., 2017). Human presence and sounds create a “landscape of fear” in which prey animals perceive spatially varied risk across a landscape (Suraci et al., 2019a); large animals moved more cautiously and reduced their home range size in response to human sounds (Suraci et al., 2019a). Medium-sized mammals responded to human sounds by reducing their foraging time or increasing nocturnality, and small mammals increased their habitat use near human activity due to the new absence of larger mammals (Suraci et al., 2019a). Gaynor et al. (2018) found that human activity increased nocturnality in mammal species across continents, trophic levels, and body sizes, and that nocturnality increased similarly in response to both lethal and non-lethal human activities. Human presence can also lead to complex outcomes on species with multiple competing pressures and responses, such as near-trail areas acting as refugia for elk (Cervus canadensis) from wolf (Canis lupus) predators (Rogala et al., 2011).
Just as foot traffic on trails can disturb wildlife communities, so can mechanized recreation. There is substantial evidence that non-motorized vehicles, such as bicycles, have negative impacts on wildlife (Larson et al., 2016) and modify their behavior (Scholten et al., 2018; Wisdom et al., 2018). Fast, quiet bikers can trigger startle responses or aggression in wildlife (Quinn and Chernoff, 2010). New mountain bike technologies (fat tired bikes and e-bikes) are expanding biking seasons and distances, and mountain bikers are responsible for a substantial amount of new legal as well as illegal trail construction (Quinn and Chernoff, 2010; Monz and Kulmatiski, 2016). For example, between 2000 and 2019, over 3600 trail projects have received funding for new bike trail construction in the United States (US Department of Transportation, 2019). The greatest impacts of mountain biking trails occur during the development and early use period of the trails (Quinn and Chernoff, 2010). Many mountain bike organizations oppose designations of new wilderness areas that restrict trail use or creation, and the “outlaw mentality” in mountain bike culture leads to many bikers going off trails even though they know it is not allowed (Wuerthner, 2019). Additionally, in terms of risk perception, animals may perceive mountain bikes to be more similar to motorized vehicles than to other forms of recreation due to their high velocity (Naidoo and Burton, 2020).
Motorized vehicles are an important means for people to access nature (Jones et al., 2016), but they can also be a major disturbance to the ecosystems that people are traveling to see in the first place. Studies have found that compared to non-motorized recreation, motorized vehicles cause greater changes in species’ habitat use (Naidoo and Burton, 2020) as well as elk behavior (Ciuti et al., 2012). An increase in daily vehicular traffic correlated with decrease in moose and bear sightings (Knight and Cole, 1995). Yet the demand for outdoor recreation opportunities and access via motorized vehicles continues to grow (Jones et al., 2016).
Previous investigations into the relationship between human presence and wildlife habitat use have compared animal responses at sites with and without tourism, but it is also important to study a gradient of recreation intensity (Larson et al., 2016; Dertien et al., 2021) and a variety of different impact regimes based on different management protocols (Blumstein et al., 2017). More research is needed to examine the effectiveness of different types of protected areas with different human activities for conserving wildlife (Sutherland et al., 2009). To date, research on recreation impacts more often quantifies individual-level responses, while population- and community-level responses are less studied. Studies of terrestrial recreation impacts often focus on hiking and running, while fewer studies investigate impacts of biking, dog walking, and equestrian activities (Larson et al., 2016). To manage wildlands more effectively, we need to study many species to determine if they have similar or different responses to a variety of recreational activities and visitation rates.
We studied wildlife habitat use on a set of trails with a variety of recreational activities and visitation rates. We focused on a network of trails in the Upper East River Valley in and around the Rocky Mountain Biological Laboratory (RMBL) near Crested Butte, Colorado, a prominent outdoor recreation site for hikers and mountain bikers. Here we use single-species, single-season occupancy models to identify recreational and environmental factors that influence the habitat use of three common mammal species: mule deer (Odocoileus hemionus), coyotes (Canis latrans), and red foxes (Vulpes vulpes). We tested effects of recreation on both occupancy and detectability for each species. We used three separate variables representing different types of recreation (hiking, mountain biking, and motorized vehicle densities) to compare impacts of different human activities.
Given the evidence that animals perceive risk from human presence in natural areas (Frid and Dill, 2002; Suraci et al., 2019a), and that many wildlife species are known to avoid humans either temporally (Gaynor et al., 2018; Naidoo and Burton, 2020) or spatially (Suraci et al., 2019a), we predicted that wildlife detectability (visibility/activity) and occupancy (site presence) would be lower in areas with more recreational activity. We also predicted that mechanized and motorized recreation, including mountain biking and driving larger vehicles such as cars, would have greater impacts relative to hiking because the higher speed and noise levels of these vehicles are thought to cause greater disturbances to wildlife than foot traffic (Quinn and Chernoff, 2010; Naidoo and Burton, 2020).
2 Methods
We studied human and wildlife activity in and around the Rocky Mountain Biological Laboratory (RMBL; N 38.9585336, W 106.9899337) over a five-week period in June-August 2020. This data collection period captured the start of peak tourism season to examine varying levels of human visitation. We surveyed mammalian activity near hiking and biking trails by placing camera traps along trails. We quantified how humans influenced the habitat use of mid-sized and large mammals as a function of human visitation rate and activity type with occupancy models to estimate species occupancy while correcting for imperfect detection (MacKenzie et al., 2002; Bailey and Adams, 2005).
We included five hiking and biking trails in this study: Deer Creek (N 38.945534, W 106.981061), Trail 401 (N 38.964142, W 106.988587), Trail 403 (N 38.982264, W 107.007438), Kettle Ponds Road (N 38.953452, W 106.988142), and Avery Mountain (N 38.966412, W 106.993313) (Figure 1). This set includes trails that are frequently used for all activities, trails that are off limits to bikers, trails that are infrequently visited and only by hikers, and wide trails with motorized vehicle access. This site presents an excellent opportunity to study wildlife habitat use near trails with varying activities and heterogeneous impact intensities. The shortest trail, Kettle Ponds Road, is approximately 1500 m long, so we placed cameras along the first 1500 m of each trail to standardize the camera spacing among all the trails. Most, but not all, trails originated at a road. The mix of trails was intentional; we aimed to have trails with different degrees of human use.
We used a total of 30 cameras, placing six cameras on each of the five trails using a stratified random design to ensure every portion of each trail was studied throughout the study period (Figure 2). Each 1500 m trail segment was divided into six zones, each of which contained one camera. Each zone was divided into six subsections, and every week one subsection was randomly selected in each zone for the camera to be deployed for seven days. At the end of a seven-day deployment period, each camera was moved to a new, randomly selected subsection within its original zone for the next deployment. Each subsection was used only once during the entire study period.
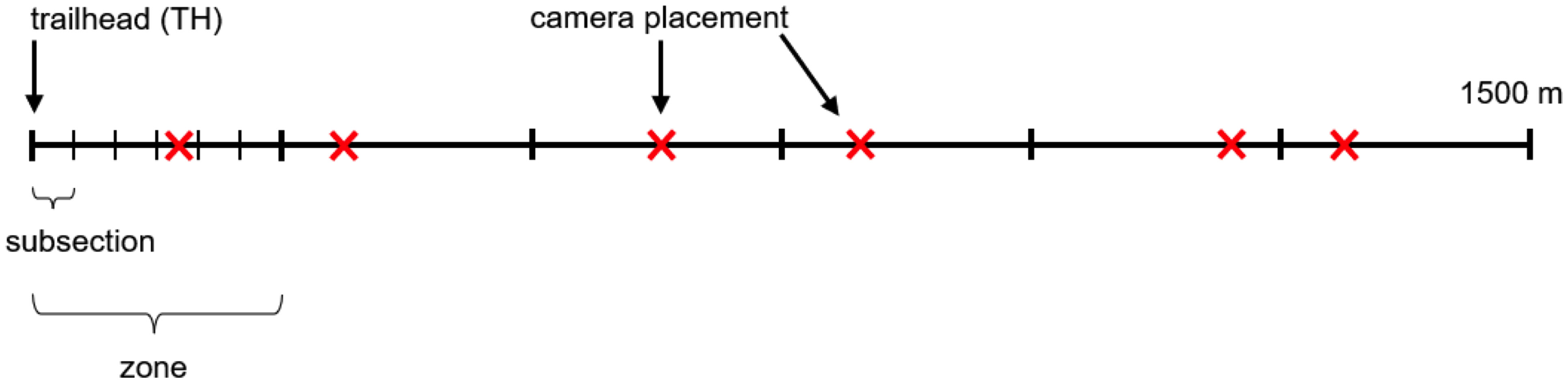
Figure 2 Camera placement schematic representing a 1500 m trail segment to be sampled, starting at the trailhead (TH), and divided into six zones with 6 subsections each. Each “X” represents one camera.
We used digital camera traps (Browning Strike Force Model BTC-5HDPX, Prometheus Group, LLC, Birmingham, Alabama, USA) to record human and wildlife activity on the trails in the study area. Cameras were placed next to the trail and pointed at the trail so as to capture movement along the trail and we focused on images of animals moving along the trails. The motion-activated cameras captured a single still image when triggered by movement from a human, animal, or vehicle, with a delay of one second between photos. The cameras also recorded the date and time that each photo was taken, and this information was later used to create species detection histories. For each deployment we recorded the height of the tallest vegetation between the camera and the trail in its view to account for the potential for tall vegetation to obscure small animals from the camera’s view.
Cameras were considered non-operational if the batteries died, the SD card became too full to store any additional photos, or the camera fell and was no longer facing the trail. We also discarded the camera-days where cattle were present as the impacts of cattle ranching on wildlife was beyond the scope of this study. Non-operational camera days were treated as missing (NA) data within our occupancy modeling framework and thus are incorporated into the uncertainty for each estimate (MacKenzie et al., 2006).
The short delay of one second between photos allowed for the collection of detailed photo sequences, often with multiple photos representing a single visit. We processed the resulting image dataset by saving a single image from a sequence to represent each “capture,” or one individual person, animal, or vehicle at one time. A capture starts when an individual entered the camera’s view, and it ends when that individual left the camera’s view and was not present in the next photo. Each sequence of photos was examined to determine the number of captures in the sequence. The number of captures of each species denotes the number of times the cameras were triggered by activity. This method counts individuals each separate time they pass in front of the camera, which represents the amount of activity at the site rather than the number of unique individuals. All wildlife sightings were identified to species using all available clues within the photo sequences, including body size, body features, color patterns, and fur textures.
Three wildlife species were sufficiently common to create occupancy models: mule deer, coyotes, and red foxes. The metadata of the scored photos was used to create presence-absence detection histories for each of the three focal species in the R programming language v4.2.0 (R Core Team, 2021) using the package camtrapR, version 2.1.1.01 (Niedballa et al., 2016). The detection histories were then used to fit single-species, single-season occupancy models for each of the three focal species (MacKenzie et al., 2006). We fitted occupancy models in the R package unmarked, version 1.2.3 (Fiske and Chandler, 2011).
The primary period, or “season,” (MacKenzie et al., 2006) for the occupancy framework was seven days long as each camera was deployed for seven days before being moved to start a new deployment. The secondary period, or “survey” (MacKenzie et al., 2006), was one day, meaning the presence or absence of a species or activity was measured on a daily basis. Detectability is represented on a daily scale and occupancy is represented on a seasonal (i.e., seven-day) scale.
We calculated the number of captures of each human activity per day and used these daily captures as predictor variables in the detection formulas of the models. Although it did not impact our results, since ‘unmarked’ models cannot tolerate missing covariate values (even when the primary observation data itself is missing), we replaced covariate missing values with deployment median values where necessary. We calculated the seven-day averages of daily captures of each activity at each site to use as seasonal predictor variables in the occupancy formulas of the models. We standardized all continuous predictor variables, which included survey-level covariates: pedestrians (ped), bikers (biker), and motorized vehicles (vehicle); seasonal covariates: site-averaged pedestrians (avg.ped), site-averaged bikers (avg.biker), and site-averaged motorized vehicles (avg.vehicle); as well as distance from the trailhead (scale.distance), understory vegetation height (scale.veg), and Day of Year of the start of each deployment (start.jday.scale).
We tested for multicollinearity among the human predictor variables and found that pedestrians and dogs were highly correlated (r > 0.9); therefore, we excluded dog data from the models. There were very few horseback riders in the data set, and since we did not expect them to be a major driver of habitat use patterns in this system, we excluded equestrian data to avoid over-fitting the models.
We included human activities as covariates of both detectability and occupancy in accordance with our hypotheses. We included human covariates in the detectability formula to test how human disturbance manifested itself on detection rates. We used daily count data for the detectability covariates and seasonal averages of the count data for the occupancy covariates.
We hypothesized that trailheads have more human activity than farther along the trails, and we expected that proximity to the trailhead could affect species occupancy. Therefore, we included a variable accounting for the distance from the trailhead in the occupancy formula, but not the detection formula because we expected this covariate to likely have longer-term effects on wildlife presence or absence rather than day-to-day effects on wildlife behaviors.
We expected that a focal species’ predators or prey could affect occupancy. We therefore included predators (coyotes, black bears, and mountain lions) in the deer model (avg.pred) and prey in the coyote and fox models (we discuss this assumption below). For coyotes, the “avg.prey” variable included all detections of deer, elk, and marmots combined. For foxes, the “avg.prey.fox” variable included only marmots. We recognize that both coyotes and foxes eat smaller mammals as well as birds at our study site, which we were unable to quantify.
We included the understory vegetation height variable in the detectability formula for its potential to decrease probability of detection, especially for smaller animals. We also included the Day of Year variable, which represents the Day of Year of the first day of each deployment, because animals may change their activity patterns throughout the year, especially in this highly seasonal study area where perceived risk may vary over time.
We included trail as a random effect in the occupancy formula to account for potential differences among the five trails studied. We included this random effect in every model we tested. We tested random effects in the occupancy models using the R package TMB, version 1.8.1 (Kristensen et al., 2016).
Consequently, we developed a full model with all a priori covariates for both the detectability and occupancy equations. For detectability, our full model included covariates for pedestrians (‘ped’), bikers (‘biker’), vehicles (‘vehicle’), vegetation height (‘scale.veg’), and the first calendar day of the season (‘start.jday.scale’). For occupancy, our full model included covariates for seasonally averaged pedestrians (‘avg.ped’), bikers (‘avg.biker’), vehicles (‘avg.vehicle’), distance from trailhead (‘scale.distance’), a measure of species-specific predators or prey (‘avg.pred’, ‘avg.prey’, or ‘avg.prey.fox’), and a random effect for trail.
We used a two-step model selection approach (MacKenzie et al., 2006): we first tested all possible combinations of covariates in the detectability formula using the full occupancy formula and found the detectability parameterization with the lowest Akaike Information Criterion (AIC). Then we used the lowest AIC detectability parameterization for each species to test all combinations of covariates in the occupancy formula. This approach involved testing a total of 64 models for each species (listed in Supplementary Materials). The lowest AIC model resulting from the second step was considered the “best” model for a given species.
Site occupancy of unmarked species can be estimated with an occupancy model framework, but the model assumptions are often violated in camera trap studies (MacKenzie et al., 2006). In this study, the model assumption that sites are “closed” (site occupancy does not change during the survey period) is likely violated because the home ranges of the species in this study are presumably larger than the distance between the cameras. Therefore, the model output is best interpreted as habitat use – the probability that a site is used by a species – rather than the strict probability of occupancy (MacKenzie et al., 2006; Burton et al., 2015; Gould et al., 2019). All cameras were deployed along trails; thus, the resulting models can be used to make inferences about species presence and detectability around these trails.
3 Results
Trails varied greatly in their exposure to human disturbance. Across the five trails, only two trails regularly experienced off-road vehicles (Avery: 21 average detections per week, per camera; Kettle Ponds: 10 average detections per week, per camera). While all trails experienced pedestrians and bikers, Trail 401 had the most bikers (78 average detections per week, per camera) while Avery had the most pedestrians (272 average detections per week, per camera). Deer Creek was the least impacted trail, with a weekly average of 17 pedestrians, 15 bikers, and 0 vehicles per camera. Considerable variation existed within trails as well. For example, all trails had cameras that detected 0 vehicles per week, and most (Trail 403, Avery, and Kettle Ponds) had cameras that detected 0 bikers per week. Even one trail (Kettle Ponds) had cameras that detected 0 pedestrians per week.
Mule deer were the most commonly detected species in our dataset, with a total of 777 detections. The final model for mule deer included bikers in the detectability formula, and vehicles, predators, and distance from the trailhead in the occupancy formula (Tables 1, S1). The sign of these covariate relationships indicated that mule deer were more likely to use areas with more vehicles, in areas with more predators, and in areas farther from the trailhead. At a finer scale, they were more likely to be detected where there were more bikers (Figure 3). Although all variables were included in the top model by AIC, none of the independent covariate effects showed significant relationships (all p > 0.05).
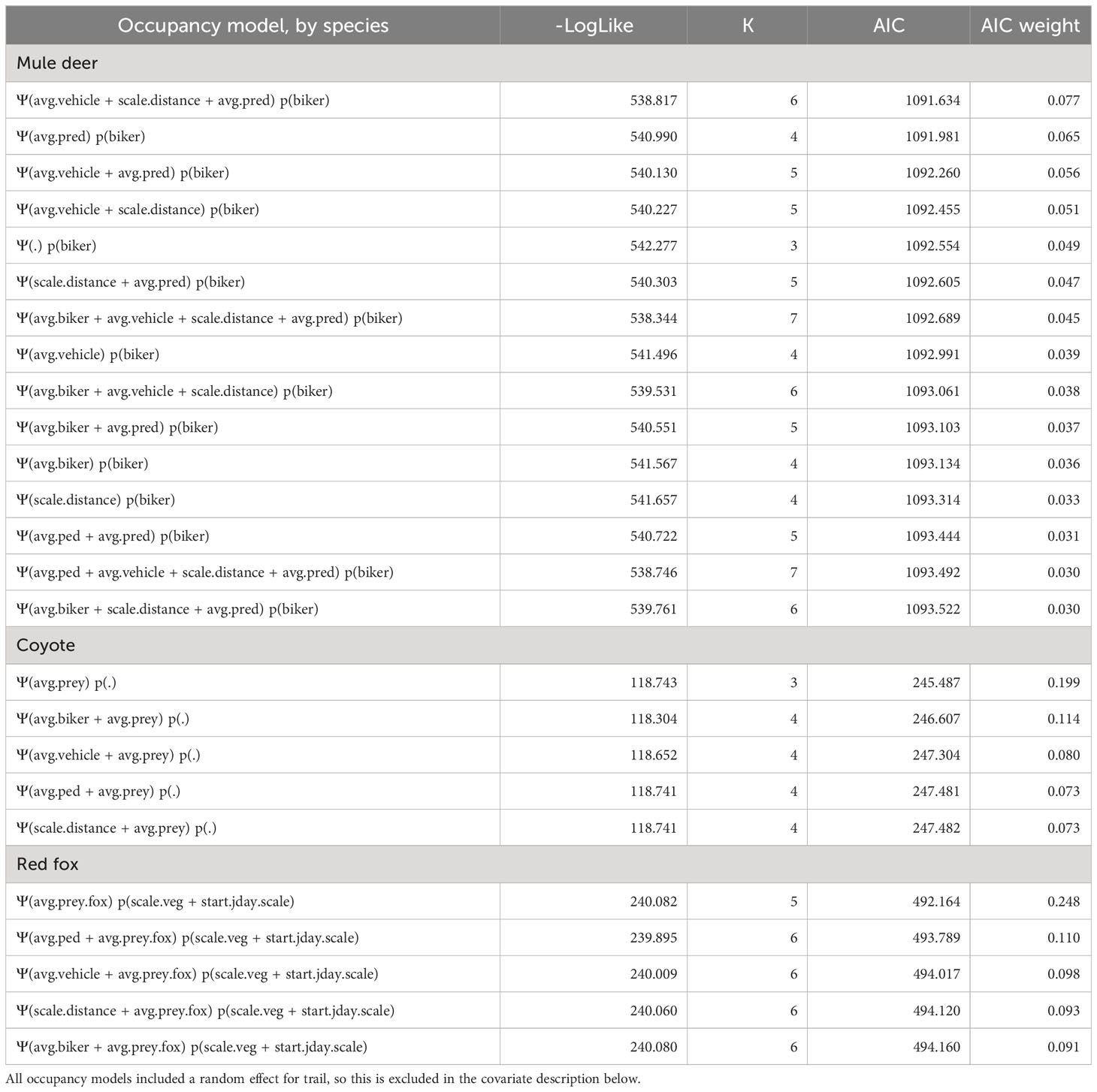
Table 1 Negative log likelihood (-LogLike), number of parameters (K), AIC, and AIC weight for the top occupancy models (ΔAIC < 2) for each species.
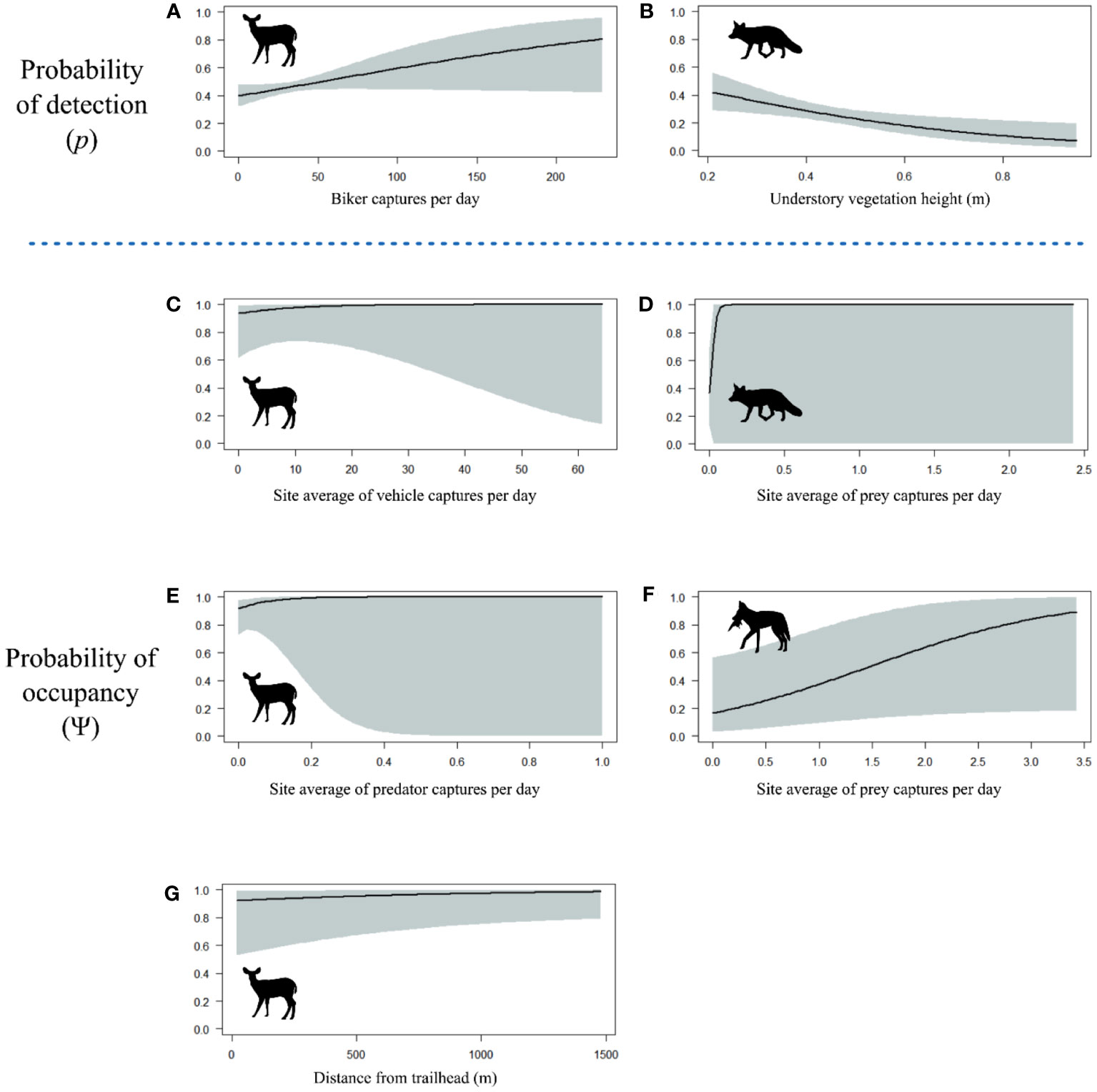
Figure 3 Predictor variable response curves for probability of detection of (A) mule deer versus biker captures per day, and (B) red foxes versus understory vegetation height in meters. Predictor variable response curves for probability of occupancy of (C) mule deer versus site average of vehicle captures per day, (D) red foxes versus site average of prey captures per day, (E) mule deer versus site average of predator captures per day, (F) coyote versus site average of prey captures per day, and (G) mule deer versus distance from trailhead in meters. Shaded areas correspond to 95% confidence intervals. Covariate effects are plotted only for those variables appearing in the top model for each species.
The top model for coyotes had no covariates in the detectability formula and prey in the occupancy formula (Tables 1, S2). Coyotes were most likely to use areas where their prey were also found (covariate effect p = 0.07). There was no evidence that their weekly habitat use patterns or diurnal activity patterns were affected by recreation (Table 1).
The top model for foxes included vegetation height and day of year in the detectability formula and fox-specific prey in the occupancy formula (Tables 1, S3). The sign of the covariate effect for occupancy indicated that foxes were most likely to use areas where their prey were also found, although this was highly uncertain (covariate effect p = 0.80). They had significantly lower detectability in taller vegetation (p < 0.001; Figure 3). We did not find evidence of recreation impacts in this species.
During the course of this study, we also detected several other mammal species, although not in sufficient numbers to allow for inclusion in the modeling framework. These included moose (Alces alces), badgers (Taxidea taxus), pine martens (Martes americana), porcupines (Erethizon dorsatum), yellow-bellied marmots (Marmota flaviventer), and elk and we summarize these results in Table S4.
4 Discussion
We used occupancy models to examine three species’ habitat use patterns near trails in a popular outdoor recreation area. We observed species-specific responses (or lack of responses) to the range of human recreation that occurs in the Upper East River Valley, Colorado during the summer tourist season. We predicted that because wildlife perceive risk from human presence (Frid and Dill, 2002; Suraci et al., 2019a), we would find lower wildlife detectability and occupancy in areas with more recreational activity. This prediction was not supported by the data as we did not find any negative relationships between species’ habitat use and human recreational activities. We also predicted that mountain biking and motorized vehicles would have a greater impact than hiking due to the speed and noise of these activities (Quinn and Chernoff, 2010; Naidoo and Burton, 2020). We did not find evidence to support this prediction; mule deer were more likely to use areas with more mountain bikers and motorized vehicles, and coyotes and foxes were apparently not affected by mechanized recreation.
Although our best model included substantial uncertainty, mule deer trended toward being more likely to use habitats farther from the start of the trails, but also in areas with more motorized vehicles, and locations where there were more predators. They were also more likely to be detected where there were more bikers. In some ways, these results – if indicating true relationships – appear counter-intuitive. However, Price et al. (2014) found that mule deer perceived less risk in areas of focused human activity. The deer in the study area could be more common and/or detectable in areas with more bikers and motorized vehicles due to habituation around the heavily-used trails. Interestingly, Lendrum et al. (2017) found that during the winter, mule deer became more nocturnal in areas around energy development and its associated impacts. The increased habitat use where there were more predators may be a consequence of predator species’ attraction to prey animals, as shown in the final coyote model.
We did not find evidence of recreation effects for coyotes. Previous studies have shown mixed results regarding coyotes’ tolerance of human disturbance. Several studies show that coyotes exhibit low same-day occurrence with humans (Patten and Burger, 2018) and decrease habitat use near human disturbance (George and Crooks, 2006; Larson et al., 2020). Other studies show that human trail use does not affect coyote habitat use (Townsend et al., 2020) and that coyotes often habituate to disturbances (Darrow and Shivik, 2009). While they may be sensitive to recreation impacts, coyotes are known to be very adaptable and somewhat tolerant of human presence (Larson et al., 2020). Coyotes at all our sites were detected mostly at night. McClennen et al. (2001) found that human activity drove coyotes to be more nocturnally active. Earlier in the spring, when there are few recreationists in the valley and very few researchers at the RMBL, coyotes are occasionally seen during the day (D.T.B. personal observations). It is likely that by the time we began our study, the impact of humans on their diurnal activity patterns was complete. But many mammals, including coyotes, are quite flexible in their diel activity patterns as a function of the intensity of human activity, and increased human activity drives them to be more nocturnal (Gallo et al., 2022). From a management perspective, the lack of behavioral changes in this study area may be a relatively favorable outcome than the alternative possibility of attraction to human activity or developments, which can lead to coyote-human conflicts (Poessel et al., 2017).
We also found no evidence of recreation effects for red foxes. A possible explanation for this result is that red foxes are generally either nocturnal or crepuscular (Figure S1; Ables, 1969), and nocturnal species are less likely to be affected by recreation than diurnal species (Erb et al., 2012). This mostly nocturnal behavior on the trails was somewhat surprising because during this study an exceptionally human-tolerant pair of foxes were raising their litter in the RMBL townsite and were seen throughout the day and night (D.T.B. personal observations). Additionally, Gallo et al. (2022) found a weaker effect of increased urbanization on red foxes than coyotes. Indeed, red foxes were positively associated with high trail use areas in a study by Erb et al. (2012), exemplifying their adaptability and tolerance of human disturbance. This result aligns with residents’ observations of red foxes in the East River Valley; they are commonly observed in towns and sometimes den near people’s houses (D.T.B. personal observations). Fox detectability was influenced by the day of year; detectability decreased later in the season. This could mean that foxes become more sensitive to human presence as the tourist season progressed, or this result could simply be due to natural changes in the species’ movements and/or abundance throughout the year (for instance, as their young grew and required more food).
Aside from methodological and power limitations, including the potential absence of important predictors like fine-scale habitat information, another possible reason for a lack of a detectable recreation effect on coyotes and red foxes is that recreation activities were predictable or were below some threshold activity level that could drive a change in behavior. In addition, as noted above (and in Figure S1), foxes and coyotes were mostly detected in the middle of the night, times that there was little if any recreational activity on the trails which was mostly concentrated during the day. Thus, the activity patterns of these species may already have been modified by humans. A lack of recreation effects could also result from habituation, whereby repeated exposure to humans have already increased their tolerance of humans. This is not likely to explain the negative effects of humans on foxes and coyotes since their detections were mostly at times when people were not active on trails. Future studies, including perhaps multi-state diel models (Rivera et al., 2022), will be required to evaluate these alternatives.
Our study neglected to consider topography or microhabitat. These factors might attract or funnel species into certain locations. Future studies could capitalize on recently developed LiDAR models of the area and add these potential covariates to determine their impact on activity.
Generally, wildlife species perceive a much greater threat from humans than from non-human predators (Clinchy et al., 2016; Zanette et al., 2023), including domestic dogs (Suraci et al., 2019b). However, humans and dogs together can have a greater impact on wildlife than humans or dogs separately (Banks and Bryant, 2007; Lenth et al., 2008; Parsons et al., 2016). Hikers in the study area often bring their dogs with them, which could increase their potential impact. However, the dog data were excluded from the analyses due to high correlation with the hiker data; thus, our results may somewhat underestimate potential negative impacts of hiking on wildlife. These effects are still incompletely understood; we advocate for additional studies designed specifically for gauging the effects of dogs and humans together on wildlife.
We grouped the captures of potential prey species, including mule deer, elk, and marmots, to test whether prey species detections influenced predator species habitat use. This makes an assumption that all are equally vulnerable and at all ages. However, this is certainly incorrect in that mountain lions may be a primary predator on adult deer, while deer fawns and elk calves may be preyed upon by coyotes and bears. More data are required to properly tease apart these fascinating ecological interactions.
We also acknowledge that the predator species in this study may also prey on other species (mice, voles, ground squirrels and chipmunks, and birds) and data for these species are being analyzed in a complementary study. Our results show a general positive relationship between prey density and predator habitat use, but further research should study prey effects further, including smaller mammals and birds, to increase the precision of the estimate of this relationship.
We studied three common species found at the study site, but this area is home to many more species, including other mid-sized and large mammals such as black bears, mountain lions, elk, moose, marmots, snowshoe hares (Lepus americanus), pine martens, badgers, and porcupines. However, we were unable to fit occupancy models for the other species found at our sites due to insufficient detection data. This is not to say that humans have no impact on their distribution and abundance as shown in studies by Suraci et al. (2019a); Suraci et al. (2019b); Naylor et al. (2009), and Ciuti et al. (2012). Future studies could use more survey sites (MacKenzie et al., 2006) and an extended data collection period to capture more detections and create models for these species with low detection rates.
Of the three focal species studied here, two apparently were not impacted by recreation, and the third showed evidence of potential attraction to areas with higher levels of recreation. The deer in our study area may be exhibiting habituation, which can lead to behavioral changes such as lower vigilance or increased boldness, potentially making them more vulnerable to predators and reducing their fitness (Geffroy et al., 2015). Because deer are declining in Colorado (Colorado Parks and Wildlife, 2020) and are of particular management concern, future research should more closely investigate fitness impacts for each species to determine whether mitigation is needed. Importantly, these results show that wildlife species are differentially affected by human recreation activities. These findings lend support to sustainable ecotourism recommendations founded on the knowledge that wildlife species each have unique sensitivities and management needs (Samia et al., 2017). Therefore management plans should be tailored to the species of interest; single interventions will likely have different impacts on different species and, depending upon the species, lead to different outcomes.
Data availability statement
The datasets presented in this study can be found in online repositories. The names of the repository/repositories and accession number(s) can be found below: Summarized detection histories and code are available at https://osf.io/4f9vc/.
Ethics statement
The animal study was approved by University of California Los Angeles Animal Research Committee. The study was conducted in accordance with the local legislation and institutional requirements.
Author contributions
MU and DB designed the study. JR, RS, and SS contributed to the design of the field experiment, and AB, DN, and VR executed the field experiment. MA, MT, and DB conducted the statistical analysis. MU wrote the manuscript, with input from all authors. All authors contributed to the article and approved the submitted version.
Funding
The author(s) declare financial support was received for the research, authorship, and/or publication of this article. AB, DN and VR were NSF-REU fellows at the RMBL (DBI 1755522).
Acknowledgments
We thank Kevin Blecha for logistical support and advice and the Blumstein lab for feedback on this project. We thank Graham Montgomery for assisting with the creation of the results figure. We are indebted to our team of photo scorers that included (alphabetically): Tracy Anyasi; Lynnea Doshi; Marni Kahn; Shyl Lamba; Isabel Luu; Silvi Lybbert; Meghety Manoyan; Madison Pfau; Hind Saif; Adrianna Schwaiger; and Emily Smetanka.
Conflict of interest
Author MU is employed by company Stillwater Sciences.
The remaining authors declare that the research was conducted in the absence of any commercial or financial relationships that could be construed as a potential conflict of interest.
Publisher’s note
All claims expressed in this article are solely those of the authors and do not necessarily represent those of their affiliated organizations, or those of the publisher, the editors and the reviewers. Any product that may be evaluated in this article, or claim that may be made by its manufacturer, is not guaranteed or endorsed by the publisher.
Supplementary material
The Supplementary Material for this article can be found online at: https://www.frontiersin.org/articles/10.3389/fcosc.2023.1234157/full#supplementary-material
References
Ables E. D. (1969). Studies of red foxes in Southern Wisconsin. J. Wildl. Mgmt. 33, 145–153. doi: 10.2307/3799662
Bailey L., Adams M. (2005)Occupancy models to study wildlife. Available at: https://www.nps.gov/olym/learn/nature/upload/OccupancyModelFactSheet.pdf (Accessed August 21, 2021).
Baker A. D., Leberg P. L. (2018). Impacts of human recreation on carnivores in protected areas. PloS One 13 (4), e0195436. doi: 10.1371/journal.pone.0195436
Balmford A., Green J. M. H., Anderson M., Beresford J., Huang C., Naidoo R., et al. (2015). Walk on the wild side: Estimating the global magnitude of visits to protected areas. PloS Biol. 13 (2), e1002074. doi: 10.1371/journal.pbio.1002074
Banks P. B., Bryant J. V. (2007). Four-legged friend or foe? Dog walking displaces native birds from natural areas. Biol. Lett. 3, 611–613. doi: 10.1098/rsbl.2007.0374
Bastone K. (2019) Are trails in Colorado harming wildlife? Available at: https://www.5280.com/2019/07/are-trails-in-colorado-harming-wildlife/.
Blumstein D. T., Geffroy B., Samia D. S. M., Bessa E. (2017). “Creating a research-based agenda to reduce ecotourism impacts on wildlife,” in Ecotourism’s Promise and Peril. Eds. Blumstein D. T., Geffroy B., Samia D. S. M., Bessa E. (Cham: Springer International Publishing), 179–185. doi: 10.1007/978-3-319-58331-0_11
Bötsch Y., Tablado Z., Scherl D., Kéry M., Graf R. F., Jenni L. (2018). Effect of recreational trails on forest birds: Human presence matters. Front. Ecol. Evol. 6. doi: 10.3389/fevo.2018.00175
Boué K. (2019). Geotagging and social media in our modern age of conservation. Uncommon Path. Available at: https://www.rei.com/blog/news/rei-presents-in-our-nature-is-photography-ruining-the-outdoors.
Bowker J. M., Askew A. E., Cordell H. K., Betz C. J., Zarnoch S. J., Seymour L. (2012). Outdoor recreation participation in the United States-projections to 2060: a technical document supporting the Forest Service 2010 RPA Assessment. General Technical Report-Southern Research Station, USDA Forest Service, (SRS-160). (Asheville, NC: US Forest Service).
Burton A. C., Neilson E., Moreira D., Ladle A., Steenweg R., Fisher J. T., et al. (2015). REVIEW: Wildlife camera trapping: a review and recommendations for linking surveys to ecological processes. J. Appl. Eco. 52, 675–685. doi: 10.1111/1365-2664.12432
Caravaggi A., Banks P. B., Burton A. C., Finlay C. M. V., Haswell P. M., Hayward M. W., et al. (2017). A review of camera trapping for conservation behaviour research. Remote Sens. Ecol. Conserv. 3, 109–122. doi: 10.1002/rse2.48
Ciuti S., Northrup J. M., Muhly T. B., Simi S., Musiani M., Pitt J. A., et al. (2012). Effects of humans on behaviour of wildlife exceed those of natural predators in a landscape of fear. PloS One 7, e50611. doi: 10.1371/journal.pone.0050611
Clinchy M., Zanette L. Y., Roberts D., Suraci J. P., Buesching C. D., Newman C., et al. (2016). Fear of the human “super predator” far exceeds the fear of large carnivores in a model mesocarnivore. Behav. Ecol. 27, 1826–1832. doi: 10.1093/beheco/arw117
Cole D., Landres P. (1995). “Indirect effects of recreation on wildlife,” in Wildlife and recreationists - coexistence through management and research. Eds. Knight R. L., Gutzwiller K. J. (Washington, D.C.: Island Press), 183–202.
Colorado Parks and Wildlife (2020) Status of Colorado’s deer, elk, and moose populations. Available at: https://cpw.state.co.us/Documents/Hunting/BigGame/Colorado_Big_Game_Population_Status_and_Management_Summary2_2020.pdf.
Darrow P. A., Shivik J. A. (2009). Bold, shy, and persistent: Variable coyote response to light and sound stimuli. Appl. Anim. Behav. Sci. 116, 82–87. doi: 10.1016/j.applanim.2008.06.013
Dertien J. S., Larson C. L., Reed S. E. (2021). Recreation effects on wildlife: a review of potential quantitative thresholds. Nat. Cons. 44, 51–68. doi: 10.3897/natureconservation.44.63270
Doherty T. S., Hays G. C., Driscoll D. A. (2021). Human disturbance causes widespread disruption of animal movement. Nat. Ecol. Evol. 5, 513–519. doi: 10.1038/s41559-020-01380-1
Erb P. L., McShea W. J., Guralnick R. P. (2012). Anthropogenic influences on macro-level mammal occupancy in the Appalachian trail corridor. PloS One 7, e42574. doi: 10.1371/journal.pone.0042574
Fiske I., Chandler R. (2011). unmarked: An R package for fitting hierarchical models of wildlife occurrence and abundance. J. Stat. Soft. 43, 1–23. doi: 10.18637/jss.v043.i10
Frid A., Dill L. (2002). Human-caused disturbance stimuli as a form of predation risk. Conserv. Ecol. 6, 11. doi: 10.5751/ES-00404-060111
Gallo T., Fidino M., Gerber B., Ahlers A. A., Angstmann J. L., Amaya M., et al. (2022). Mammals adjust diel activity across gradients of urbanization. eLife 11, e74756. doi: 10.7554/eLife.74756.sa2
Gaynor K. M., Hojnowski C. E., Carter N. H., Brashares J. S. (2018). The influence of human disturbance on wildlife nocturnality. Science 360, 1232–1235. doi: 10.1126/science.aar7121
Geffroy B., Samia D. S. M., Bessa E., Blumstein D. T. (2015). How nature-based tourism might increase prey Vulnerability to Predators. Trends Ecol. Evol. 30, 755–765. doi: 10.1016/j.tree.2015.09.010
George S. L., Crooks K. R. (2006). Recreation and large mammal activity in an urban nature reserve. Biol. Cons. 133, 107–117. doi: 10.1016/j.biocon.2006.05.024
Gould M. J., Gould W. R., Cain J. W., Roemer G. W. (2019). Validating the performance of occupancy models for estimating habitat use and predicting the distribution of highly-mobile species: A case study using the American black bear. Biol. Cons. 234, 28–36. doi: 10.1016/j.biocon.2019.03.010
Jones C., Newsome D., Macbeth J. (2016). Understanding the conflicting values associated with motorized recreation in protected areas. Ambio 45, 323–330. doi: 10.1007/s13280-015-0721-1
Knight R., Cole D. (1995). “Wildlife responses to recreationists,” in Wildlife and recreationists - coexistence through management and research. Eds. Knight R. L., Gutzwiller K. J. (Washington, D.C.: Island Press), 51–69.
Kristensen K., Nielsen A., Berg C. W., Skaug H., Bell B. M. (2016). TMB : Automatic differentiation and laplace approximation. J. Stat. Soft. 70, 1–21. doi: 10.18637/jss.v070.i05
Kwak-Hefferan E. (2020) 8 ways to ease overcrowding at our national parks. Available at: https://www.5280.com/2020/09/8-ways-to-ease-overcrowding-at-our-national-parks/.
Ladle A., Avgar T., Wheatley M., Stenhouse G. B., Nielsen S. E., Boyce M. S. (2019). Grizzly bear response to spatio-temporal variability in human recreational activity. J. Appl. Ecol. 56 (2), 375–386. doi: 10.1111/1365-2664.13277
Larson C. L., Reed S. E., Merenlender A. M., Crooks K. R. (2016). Effects of recreation on animals revealed as widespread through a global systematic review. PloS One 11, e0167259. doi: 10.1371/journal.pone.0167259
Larson C. L., Reed S. E., K. R. Crooks (2020). Increased hiking and mountain biking associated with declines in urban mammal activity. CFWJ 52–61.
Lendrum P. E., Crooks K. R., Wittemyer G. (2017). Changes in circadian activity patterns of a wildlife community post high-intensity energy development. J. Mammal. 98, 1265–1271. doi: 10.1093/jmammal/gyx097
Lenth B. E., Knight R. L., Brennan M. E. (2008). The effects of dogs on wildlife communities. Nat. Areas J. 28, 218–227. doi: 10.3375/0885-8608(2008)28[218:TEODOW]2.0.CO;2
MacKenzie D. I., Nichols J. D., Lachman G. B., Droege S., Andrew Royle J., Langtimm C. A. (2002). Estimating site occupancy rates when detection probabilities are less than one. Ecology 83, 2248–2255. doi: 10.1890/0012-9658(2002)083[2248:ESORWD]2.0.CO;2
MacKenzie D. I., Nichols J. D., Royle J. A., Pollock K. H., Bailey L. L., Hines J. E. (2006). Occupancy Estimation and Modeling: Inferring Patterns and Dynamics of Species Occurrence. (New York: Academic Press).
McClennen N., Wigglesworth R. R., Anderson S. H. (2001). The effects of suburban and agricultural development on the activity patterns of coyotes (Canis latrans). Am. Midl. Nat. 146, 27–36. doi: 10.1674/0003-0031(2001)146[0027:TEOSAA]2.0.CO;2
Monz C., Kulmatiski A. (2016). The emergence of “fat bikes’ in the USA: Trend, potential consequencdes and management implications. J. Outdoor Rec. Tour. 15, 20–25. doi: 10.1016/j.jort.2016.04.001
Naidoo R., Burton A. C. (2020). Relative effects of recreational activities on a temperate terrestrial wildlife assemblage. Cons. Sci. Pract. 2, e271. doi: 10.1111/csp2.271
Naylor L. M., Wisdom M. J., Anthony R. G. (2009). Behavioral responses of North American Elk to recreational activity. J. Wildl. Manage. 73, 328–338. doi: 10.2193/2008-102
Niedballa J., Sollmann R., Courtiol A., Wilting A. (2016). camtrapR: an R package for efficient camera trap data management. Methods Ecol. Evol. 7, 1457–1462. doi: 10.1111/2041-210X.12600
Parsons A. W., Bland C., Forrester T., Baker-Whatton M. C., Schuttler S. G., McShea W. J., et al. (2016). The ecological impact of humans and dogs on wildlife in protected areas in eastern North America. Biol. Cons. 203, 75–88. doi: 10.1016/j.biocon.2016.09.001
Patten M. A., Burger J. C. (2018). Reserves as double-edged sword: Avoidance behavior in an urban-adjacent wildland. Biol. Cons. 218, 233–239. doi: 10.1016/j.biocon.2017.12.033
Poessel S. A., Gese E. M., Young J. K. (2017). Environmental factors influencing the occurrence of coyotes and conflicts in urban areas. Landscape Urban Plan 157, 259–269. doi: 10.1016/j.landurbplan.2016.05.022
Price M. V., Strombom E. H., Blumstein D. T. (2014). Human activity affects the perception of risk by mule deer. Curr. Zool. 60, 693–699. doi: 10.1093/czoolo/60.6.693
Quinn M., Chernoff G. (2010) Mountain biking: A review of the ecological effects. Available at: https://www.lib.washington.edu/msd/norestriction/b67566091.pdf.
R Core Team (2021) R: A language and environment for statistical computing. Available at: https://www.r-project.org/.
Rivera K., Fidino M., Farris Z. J., Magle S. B., Murphy A., Gerber B. D. (2022). Rethinking habitat occupancy modeling and the role of diel activity in an Anthropogenic world. Amer. Nat. 200, 556–570. doi: 10.1086/720714
Rogala J. K., Hebblewhite M., Whittington J., White C. A., Coleshill J., Musiani M. (2011). Human activity differentially redistributes large mammals in the Canadian Rockies national parks. Ecol. Soc 16 (3), 16. doi: 10.5751/ES-04251-160316
Samia D. S. M., Angeloni L. M., Bearzi M., Bessa E., Crooks K. R., D’Amico M., et al. (2017). “Best practices toward sustainable ecotourism,” in Ecotourism’s Promise and Peril. Eds. Blumstein D. T., Geffroy B., Samia D. S. M., Bessa E. (Cham: Springer International Publishing), 153–178. doi: 10.1007/978-3-319-58331-0_10
Scholten J., Moe S. R., Hegland S. J. (2018). Red deer (Cervus elaphus) avoid mountain biking trails. Euro. J. Wildl. Res. 64, 1–9. doi: 10.1007/s10344-018-1169-y
Smith J. A., Suraci J. P., Clinchy M., Crawford A., Roberts D., Zanette L. Y., et al. (2017). Fear of the human ‘super predator’ reduces feeding time in large carnivores. Proc. R. Soc B. 284, 20170433. doi: 10.1098/rspb.2017.0433
Suraci J. P., Clinchy M., Zanette L. Y., Wilmers C. C. (2019a). Fear of humans as apex predators has landscape-scale impacts from mountain lions to mice. Ecol. Lett. 22, 1578–1586. doi: 10.1111/ele.13344
Suraci J. P., Smith J. A., Clinchy M., Zanette L. Y., Wilmers C. C. (2019b). Humans, but not their dogs, displace pumas from their kills: An experimental approach. Sci. Rep. 9, 12214. doi: 10.1038/s41598-019-48742-9
Sutherland W. J., Adams W. M., Aronson R. B., Aveling R., Blackburn T. M., Broad S., et al. (2009). One hundred questions of importance to the conservation of global biological diversity. Cons. Biol. 23, 557–567. doi: 10.1111/j.1523-1739.2009.01212.x
Townsend S. E., Hammerich S., Halbur M. (2020). Wildlife occupancy and trail use before and after a park opens to the public. California Fish Wildl. Recreation Special Issue 2020, 74–94. Available at: https://nrm.dfg.ca.gov/FileHandler.ashx?DocumentID=178955.
US Department of Transportation (2019) Recreational Trails Program (RTP) - Project Database. Available at: https://recreationaltrailsinfo.org/.
Wisdom M. J., Preisler H. K., Naylor L. M., Anthony R. G., Johnson B. K., Rowland M. M. (2018). Elk responses to trail-based recreation on public forests. For. Ecol. Manage. 411, 223–233. doi: 10.1016/j.foreco.2018.01.032
Wuerthner G. (2019). Impacts of mountain biking. Wildl. News. Available at: https://www.thewildlifenews.com/2019/06/18/impacts-of-mountain-biking.
Keywords: coyote, ecotourism, human impacts on wildlife, mule deer, occupancy modeling, red fox
Citation: Uetrecht MR, Bermudez A, Novoa D, Reithel J, Rodriguez V, Smith R, Sprott S, Tingley MW and Blumstein DT (2023) Differential response of three large mammal species to human recreation in the Rocky Mountains of Colorado, USA. Front. Conserv. Sci. 4:1234157. doi: 10.3389/fcosc.2023.1234157
Received: 03 June 2023; Accepted: 18 October 2023;
Published: 10 November 2023.
Edited by:
Silvio Marchini, University of Oxford, United KingdomReviewed by:
Peter Thompson, University of Alberta, CanadaWilliam J. McShea, Smithsonian Conservation Biology Institute (SI), United States
Copyright © 2023 Uetrecht, Bermudez, Novoa, Reithel, Rodriguez, Smith, Sprott, Tingley and Blumstein. This is an open-access article distributed under the terms of the Creative Commons Attribution License (CC BY). The use, distribution or reproduction in other forums is permitted, provided the original author(s) and the copyright owner(s) are credited and that the original publication in this journal is cited, in accordance with accepted academic practice. No use, distribution or reproduction is permitted which does not comply with these terms.
*Correspondence: Morgan W. Tingley, bXRpbmdsZXlAZy51Y2xhLmVkdQ==; Daniel T. Blumstein, bWFybW90c0B1Y2xhLmVkdQ==
†These authors share senior authorship