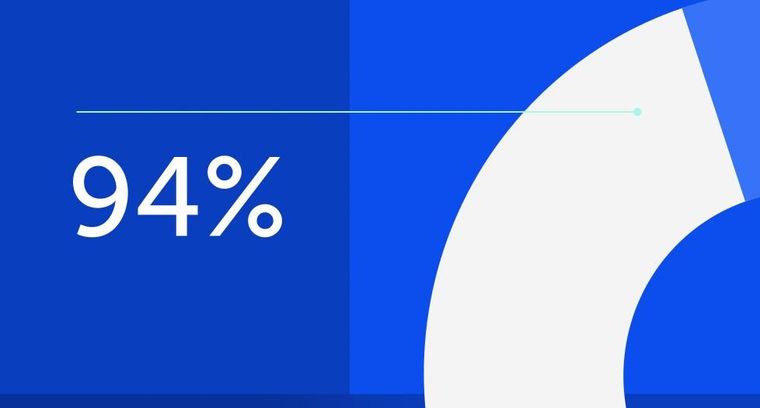
94% of researchers rate our articles as excellent or good
Learn more about the work of our research integrity team to safeguard the quality of each article we publish.
Find out more
SYSTEMATIC REVIEW article
Front. Conserv. Sci., 23 November 2023
Sec. Animal Conservation
Volume 4 - 2023 | https://doi.org/10.3389/fcosc.2023.1218153
Emerging infectious diseases have significantly increased in recent years; approximately 60% of these emerging diseases are of zoonotic origin, and of those, around 70% were identified to start with wild animals. To better understand the impacts of zoonotic diseases on wildlife, there is a need to identify the distribution and impact of zoonotic pathogens in wildlife, particular those species with threatened populations. To investigate this question, we constructed a zoonotic disease database identifying wildlife hosts for each bacterial zoonotic disease and recorded clinical signs of disease for each host-pathogen relationship if found. Species of least concern were found to have a significantly higher prevalence and richness of zoonotic bacterial diseases (n= 0.0608, x2 = 5.898, p=0.01516). The taxonomic level Order was found to be the best predictor for pathogen richness, and the Artiodactyla and Carnivora contain a significantly higher pathogen richness than other vertebrate Orders. Species with the greatest pathogen richness include the wild boar (Sus scrofa, n=66), Northern raccoon (Procyon lotor, n=16) and the red fox (Vulpes vulpes, n=41). Our results indicate bacterial zoonoses are not likely to be a driving factor of species population declines, and future zoonotic disease surveillance efforts should target species in the Artiodactyla and Carnivora families. The wild boar (Sus scrofa) in particular may be a good candidate for zoonotic disease monitoring due to its high pathogen richness, wide range, and large population. Understanding the impacts and distribution of bacterial zoonoses in wildlife populations can help in planning for future wildlife management efforts, particularly in species of conservation concern and wildlife disease monitoring.
Biodiversity loss locally and globally has accelerated in the past few centuries resulting in increasing emerging infectious disease (EIDs) outbreaks (Everard et al., 2020). Emerging infectious diseases can have devastating effects on human populations, particularly those in third-world countries who do not have the resources to treat and recover from an epidemic (Halliday et al., 2015). The 2003 SARS outbreak was estimated to cost between 5 and 50 billion dollars (Cunningham et al., 2017). In addition to EIDs being a financial burden, wildlife populations can be significantly affected by disease. White-nose syndrome results in extreme mortalities with population declines in North American bat populations since its detection in 2006 (Frick et al., 2010). In addition, chytridiomycosis infections have resulted in significant population and species declines and extinction of more than 200 amphibian species (Yap et al., 2015), and a microsporidium protozoon was found to have caused the extinction of Partula turgida, a species of tree snail (Cunningham and Daszak, 1998). To ensure the overall health of the global ecosystem and wildlife conservation, a better understanding of EIDs is needed.
Recent outbreaks of SARS-COV 2, Zika, and Ebola viruses have emphasized the need to investigate zoonotic diseases more, particularly in relation to wildlife populations (Bermejo et al., 2006; Wang et al., 2016; Everard et al., 2020). Zoonoses are diseases capable of transmitting between humans and animals, making them a threat not only to humans but to wildlife populations as well (Messenger et al., 2014). Prior to 2000, wildlife disease was primarily studied in the context of zoo animal health and welfare unless the pathogen was found to negatively impact livestock or popular game species such as white-tailed deer (Cunningham et al., 2017). The tendency to focus on domestic animal surveillance rather than wildlife for zoonoses is primarily due to the difficulties of surveying wildlife populations and the cost of surveying non-domesticated animals (Grogan et al., 2014). As a result, less research is performed on the impact of zoonoses on wildlife, despite records of previous outbreaks indicating zoonoses are just as deadly to wildlife populations. For example, the Zaire strain of the Ebola virus was found responsible for the death of over 5000 gorillas in the early twenty-first century (Bermejo et al., 2006). Similarly, both prairie dog (Cynomys spp.) and ground squirrel (Spermophilus spp.) populations experience mortalities as great as 90% during plague outbreaks caused by the zoonotic bacteria Yersinia pestis (Russell et al., 2019). Neglecting wildlife disease surveillance will have lasting negative effects on wildlife populations and will no doubt negatively impact domestic animals and human populations.
The majority of pathogens involved in EID events are bacterial or viral in nature (Jones et al., 2008). Many studies utilizing large datasets of pathogens tend to focus on viral diseases (Shaw et al., 2020). This preference leaves gaps in our knowledge of bacterial diseases and their impacts on wildlife. Bacterial diseases are becoming more of a global concern due to increasing cases of antibiotic resistance; wildlife near human civilizations is more prone to infections due to increased exposure rates from humans and domestic animals (Laborda et al., 2022). Wildlife can act as reservoirs for antibiotic resistant bacteria and contribute to the spread of antibiotic resistant bacteria to people, domestic animals, and other wildlife populations (Tardón et al., 2021). While antibiotic resistant bacteria are typically found in healthy wildlife hosts, some pathogens have been found to causes signs of disease and even death in wildlife (Heaton et al., 2020). The spread of zoonotic bacteria in the environment increases the chance of EID occurring and puts wildlife populations at risk.
While typically not a main factor of species declines and extinction, disease can still have devasting impacts on wildlife populations, for example, facial tumor disease in Tasmanian devils (Sarcophilus harrisii), fibropapillomatosis in green sea turtles (Chelonia mydas) and white-nose syndrome in six species of North American bats have all caused and continue to threaten major declines in these populations (Altizer et al., 2007; Heard et al., 2013). In the United States, there is increasing evidence that disease plays a role in threatened and endangered species decline and inhibits population recovery (Brand, 2013). Endangered species may be more at risk of zoonotic disease outbreaks due to less genetic diversity and capacity for adaptations to aid recovery (O’Brien and Evermann, 1988). In addition, methods that are used to manage species of conservation concern may also carry a risk of zoonotic disease exposure to wildlife populations. For example, the translocation of endangered species from larger populations to smaller populations to bolster new population growth can cause elevated stress levels, increasing vulnerability to diseases that otherwise would not cause morbidity and mortality (Gartrell et al., 2005). The threat of zoonoses in wildlife populations needs to be evaluated to better understand the present and future threats to species of conservation concern so that we may be better prepared for when outbreaks do occur (Gaydos et al., 2004). It should be noted that efforts are being made to prioritize surveillance and management on zoonotic diseases that can have detrimental impacts on people, domestic animals and the economy (Cox et al., 2013). However, we need to be careful not to neglect other zoonoses in our prioritizations. Identifying effective and appropriate disease management strategies in wildlife will help to prevent species declines and extinctions.
Typically, wildlife disease management is considered only after the disease has been documented in a population, even though preventing disease emergence during preemptive stages is a more effective action (Grant et al., 2017). One way to survey zoonotic pathogens in wildlife is through the sentinel surveillance of reservoir species. Sentinel surveillance focuses on specific populations to enhance our detection of diseases and improve the cost-effectiveness of surveillance (Halliday et al., 2007). Reservoir species are organisms that are capable of contracting, maintaining, and transferring pathogens to other individuals (Ostfeld, 2009). Bats are considered major reservoir species for a large variety of zoonotic viral diseases such as SARS, MERS, Ebola, Marburg, and many others (Luis et al., 2013). Rodents are considered an important carrier of many wildlife diseases, many of which are considered hyper-reservoirs; species capable of carrying greater than two pathogens (Han et al., 2015). Wild canids have been potentially identified as sentinels of wildlife and human health due to their capability of successfully carrying a variety of infectious diseases over a wide geographical range (Aguirre, 2009). Recently, wildlife disease management has started incorporating health and population monitoring, a method known as integrated wildlife monitoring; this method has been used to find increased disease risk in populations with large wild boar populations, particularly when there are increased interactions between wild boar and red deer (Barroso et al., 2023).
Competent reservoir species maintain pathogens in the environment and increase disease spread, and some could possibly be used as sentinel species for disease surveillance. Improving wildlife disease monitoring could have significant socio-economic benefits, such as reducing long-term disease management costs, protecting biodiversity and ecosystem services, and contributing to pre-spillover surveillance for public health and agricultural diseases (Grogan et al., 2014). Despite the benefits associated with sentinel monitoring, this method is generally underutilized in infectious disease surveillance (Rabinowitz et al., 2005). One of the reasons for this could be researchers’ inability to identify appropriate wildlife species or groups to use as sentinel species.
As biodiversity continues to decrease and EIDs continue to increase, there is an even greater need to understand how these pathogens impact wildlife populations. To better understand how bacterial zoonoses impacts wildlife populations, we created a zoonotic disease database that identified all documented mammal, bird, and reptile wildlife hosts for each zoonotic bacterium. We then recorded any signs of disease species experienced associated with the pathogen infection. Large-scale host-pathogen datasets such as this one can answer questions on how zoonoses are dispersed in the environment and what potential factors are correlated with increased disease prevalence. We aimed to answer two main questions using this database: 1) Are species of conservation concern more at risk for bacterial zoonosis infection than those of least concern 2) Are there any relationships between zoonotic bacterial species richness and host taxonomy? For the purpose of this research, species of conservation concern (CC) were identified as those species with a conservation status of Near threatened, Vulnerable, Endangered, Critically Endangered, and Extinct in the Wild. Species without a conservation status were listed as data deficient (DD) and were excluded from the analysis, all other species had healthy population sizes and were listed as least concern (LC). Identifying wildlife more at risk for bacterial zoonoses infections could not only help in targeting conservation methods to more at-risk species but may also identify groups of animals that can be used for zoonotic disease surveillance.
From July of 2020 to December of 2022, we performed an internet-based literature review identifying unique zoonotic bacterial infections in mammal, bird, and reptile wildlife species prior to 2022 (Hirst and Halsey, 2023). Due to time constraints other vertebrate classes were excluded from this review. We identified zoonotic bacteria species using an updated version of a previously published pathogen database (Taylor et al., 2001). The majority of pathogens in this database are known to commonly cause signs of disease in humans and animals, but in order to be as comprehensive as possible, we also included pathogens that have been reported to cause disease in humans once, those found to cause disease only in immunocompromised patients and pathogens that are typically identified as asymptomatic. In addition, we included recently recognized pathogen species from the Center for Diseases Control (CDC), Merck Veterinary Manual, and published literature (Fischer et al., 2000; Raymond et al., 2000; Allsopp et al., 2005; Pitcher and Nicholas, 2005; Lisle et al., 2008; Austin, 2010; Rudenko et al., 2011; Ebani et al., 2012; Parola et al., 2013; Bittar et al., 2014; CDC, 2022; Flahou et al., 2014; Fuke et al., 2016; Fawzy et al., 2020; Gcebe and Hlokwe, 2017; Okaro et al., 2017; Rahim et al., 2017; Sousa et al., 2018; Cheong et al., 2019; Odoi et al., 2020; Lopes et al., 2021; Ochoa and Collado, 2021; Shopland et al., 2020; Zulu et al., 2021; Merck Manual, 2022).
Two literature reviews were performed, the first identified wildlife hosts of each zoonotic bacterial pathogens, and the second identified signs of disease in each host-pathogen interaction. Using Web of Science as our primary search engine, eligibility for host status was distinguished using the following criteria:
1. The article was a peer-reviewed publication containing subject matter related to zoonotic bacterial pathogen infections in mammal, bird, and reptile species.
2. Pathogen infection occurs in wildlife species or captive individuals such as animals in zoos or privately owned. Domestic animals or animals used for agricultural purposes were not included.
3. Pathogen infections were naturally occurring and not a result of deliberate laboratory exposure.
4. Bacterial species identified were of a pathogenic nature or were identified in an abnormal location if pathogenicity was not determined in the article.
In the search fields of Web of Science, the following terms were used to identify host-pathogen interactions: “Pathogen name” and “Wildlife” or “Pathogen species name” and “Animal.” For example, to search for publications regarding host-pathogen interactions for Borrelia burgdorferi and animals, our search terms were [“Borrelia burgdorferi” AND “Wildlife”] or [“Borrelia burgdorferi” AND “Animal”]. If pathogen genus and species names had changed, all names were searched to ensure all available information was found. The full list of search strings is available in the supplemental information (Supplemental 1). For this search, titles and abstracts were reviewed for relevant host information. The first time a potential host is identified, the article is retained for an in-depth review, other articles focusing on this host are not pulled for review, unless the article includes other hosts not yet identified for the pathogen. We aimed to review each title and abstract found with our Web of Science searches using our keyword search terms. However, in some cases, searches would result in thousands of articles, and manual review was not feasible. Due to a large number of publications in some topic areas, we decided to limit the article review process to the first four pages (equivalent to 200 publications) in Web of Science. Due to this limitation, not all zoonotic bacteria in this list may have a complete host range, however, we reviewed every article for 312 of the 366 (85%) of the searches; therefore, we are fairly confident we found a large portion of mammal, bird, and reptile host species. At least one article describing the pathogen and host interaction needed to be found in our search to be eligible for inclusion in our database. If an article was deemed insufficient in identifying the host-pathogen relationship, an alternative article was searched for using the pathogen and host name. Wildlife common names, scientific names, and conservation status were standardized using the red list of International Union for the Conservation of Nature (IUCN). Signs of disease were recorded from the first search if they were described in the articles. Reference lists from selected articles were reviewed for further host-pathogen relationships.
Once host lists for each pathogen were compiled, a secondary search identifying the full range of clinical signs of infection was performed. For the second search, identifying signs of disease in host species, eligibility was distinguished using the following criteria:
1. The article was a peer-reviewed publications containing subject matter related to signs of disease in mammal, bird, and reptile species.
2. Signs of disease in domestic animals or animals used for agricultural purposes were not included, unless signs were unavailable for their wildlife counterparts.
3. Signs of disease were a result of a naturally occurring infection and not due to deliberate laboratory exposure.
4. Signs of disease were linked to a specific pathogen infection, and not a result of multiple, different pathogen infections.
To identify clinical signs for each wildlife species associated pathogen, we used the following terms to identify signs of disease: “Pathogen species name” and “Species scientific name” or “Species common name.” For example, to search for publications regarding host-pathogen interactions for Borrelia burgdorferi and animals, our search terms were [“Borrelia burgdorferi” AND “Peromyscus leucopus”]. The full list of search string combinations is available in the supplemental information (Supplemental 2). In cases where the scientific name has changed recently, or there are multiple common names for the species, each variation was searched to ensure all available information was identified. Titles and abstracts were reviewed for relevant information, unlike the host search, all articles that indicated signs of disease in a host species were retrieved for further review. Signs of disease were then recorded in the database for each host-pathogen relationship. “Signs” were defined as physical or behavioral abnormalities wildlife experience as a result of pathogen infection (i.e., vomiting, pyrexia, lesions, depression, etc.). Signs were included when they were identified as a result of the zoonotic pathogen infection. In many cases signs of disease in living individuals were not observed, so necropsy and histology findings were also included as signs of disease. For both searches, articles were limited to the English language only. Duplicate articles were removed. For both searches, reference lists of the identified studies were also checked for additional studies.
To investigate the presence and prevalence of zoonoses in species of conservation concern, we used a 2-proportion z-test and a one-way analysis of variance (ANOVA). Species that did not have a conservation status in the IUCN database were excluded from this analysis (n=84). We used a 2-proportion z-test where IUCN status was the predictor variable and the presence/absence of pathogens in wildlife species served as the response variable. The data met all the assumptions of the 2- proportion z test. We used a one-way ANOVA with zoonotic bacteria richness as the response variable and IUCN status as the predictor variable. For this analysis, our data met the assumptions of homogeneity of variances via the Levene’s test (F(1,1689) = 3.4559, p=0.063), yet did not meet the assumptions of normality for the residuals via Shapiro-Wilks (W = 0.48613, p < 0.001); however, ANOVA is robust to departures from normality especially when there is a large sample size (Blanca Mena et al., 2017.
To determine which taxonomic level has the greatest zoonotic bacterial richness, we again used ANOVA, where we perform model comparisons including a null and global model via Akaike information criterion (AIC). We transformed the bacterial richness variable using the inverse (1/richness) to meet the assumption of homogeneity (F(55,1690) =1.228, p = 0.124), although the normality was still not met (W = 0908, p < 0.001); ANOVA remained acceptable due to the large sample size and robustness to departures from normality. For the best supported model, we then used Dunn’s Kruskal-Wallis Multiple Comparisons with p-values adjusted with the Bonferroni method post hoc to identify the significantly different comparisons. All analyses were run using R 2022.07.1 R Code Team (2022).
Over 30,000 articles were investigated for potential host-pathogen relationships. Of those, 3,006 were retrieved for a more thorough evaluation, 2,963 from Web of Science, and an additional 43 from article reference lists. After removing duplicate articles, we reviewed 2,981 articles. We then removed an additional 1,012 articles for not meeting the inclusion criteria. Articles were then screened for wildlife host or symptom information. Articles that did not provide any new information were excluded, a final reference list of 1,373 studies were compiled in the bacterial zoonotic database (Figure 1).
Figure 1 Flow chart of bacterial zoonotic disease database inclusion and exclusion based on PRISMA criteria. The diagram displays the total articles screened for both literature reviews, individual statistics for each review was not available.
The database was separated into different excel forms featuring each zoonotic bacterial pathogen genus group. Column A lists the class of the host animal, arranged to show Mammalia first, followed by Aves then Reptilia. Column B contains the hosts common name as it appears in the IUCN red list. Column C identifies whether or not the host-pathogen relationship has only ever occurred in captivity (yes) or has been observed naturally in the wild (no). Columns D and E display the hosts genus and species name respectively, and column F contains the pathogen species name. Column G describes signs of disease hosts experience with pathogen infection. Column F contains citations for each entry. The full list of references was included in a separate file of the database labeled citations_database.
We identified 4,971 records describing pathogenic associations between 370 bacterial pathogens (74 families) and 1,742 unique wildlife species (999 Mammals, 579 Aves, and 158 Reptiles). Data review was limited in 54 of the 366 pathogen-host searches (14.8%). The pathogens with limited searches are denoted in supplemental information 1. We included clinical signs when they were definitively found to be a result of the infecting pathogen. We identified signs of disease in 2,588 records (1606 mammalian hosts, 825 avian hosts, and 127 reptilian hosts). All search results for signs of disease were reviewed in Web of Science. In some cases, pathogen infections were only observed in captive individuals or populations (n=1099). Pathogen host ranges vary, but overall, zoonotic bacterial pathogens have a mean host range of 13.44 hosts. Most pathogens stick to one class of wild animals (n=235), but 43 bacterial pathogens have been found to infect wild animals of Mammal, Aves, and Reptilia classes (Table 1). The Rodentia order had the most host-pathogen relationships (n=924), followed by Carnivora (n=776), Artiodactyla (n=724), Passeriformes (n=381), and Primates (n=304). Signs of disease were not described in 2,413 host-pathogen relationships; and many records that do describe signs of disease are likely incomplete in their assessments (Figure 2). Host species with the greatest pathogen prevalence are described in Table 2. Of these species, no more than 60% of cases have signs of disease described. When signs of disease were described, asymptomatic cases made up over 50% of described host-pathogen relationships in six of the ten hosts.
Figure 2 Host pathogen richness across Reptilia (A), Mammalia (B), and Aves (C) Orders. Descriptions of signs of disease are not always available, the figures compare the number of host pathogen relationships in each Order that have some signs that are described versus those with no data available.
When comparing conservation status and occurrence of zoonotic bacterial pathogen, proportionally, species of least concern (n= 0.0695) were 13.4% more likely to be found with a zoonotic bacterial infection than those of conservation concern, (n= 0.0608, x2 = 5.898, p=0.01516). As a majority of wildlife species served as hosts to more than one pathogen, comparisons of zoonotic bacterial species richness between species of conservation concern and least concern are also crucial. We found significant difference in the mean species richness with conservation status, with species of least concern having significantly higher pathogen richness than species of conservation concern (F = 4.7094, p=0.03022, Figure 3).
Figure 3 A comparison of bacterial zoonosis richness in wildlife species with an IUCN status of conservation concern (CC) and least concern (LC).
In order to investigate how pathogen richness differs between different taxonomic levels, we used an AIC comparison of pathogen richness at the genus, family, and order levels (Table 3). Order was found to be the best model for bacterial pathogen richness with a weight of 1.0 Next, we explored whether there were specific animal orders that were significant different from each other using Dunn’s Kruskal-Wallis Multiple comparisons test to compare the 57 taxonomic orders for mammals, birds, and reptiles include in the database in total, 8 orders were found to show a significant difference between each other; these include: Anseriformes (mean (μ) = 3.50), Artiodactyla (μ = 3.80), Carnivora (μ = 3.82), Chiroptera (μ = 1.2), Passeriformes (μ = 3.0), Psittaciformes (μ = 2.62), Rodentia (μ = 1.84), and Squamata (μ = 1.36),. The Anseriformes order had twice as many zoonoses than Squamata (p = 0.016) significant The Artiodactyla order had a significantly larger bacterial zoonoses richness than Rodentia (p < 0.001), and Squamata (p < 0.001), orders (Figure 4). The Carnivora order had significantly larger bacterial zoonoses richness than the Charadriiformes, Chiroptera (p = 0.016), Passeriformes (p = 0.001), Psittaciformes, (p = 0.023), Rodentia (p < 0.001), and Squamata (p < 0.001) orders.
Figure 4 Comparison of the significantly different taxonomic orders to bacterial zoonosis richness. Different letters indicate pair-wise significance at p < 0.05.
In order to investigate the range and impact of bacterial zoonoses on wildlife populations, we compiled a database of zoonotic bacterial pathogens in mammalian, avian, and reptilian wildlife hosts and identified signs associated with individual pathogen infection. We found zoonotic bacterial pathogens are more prevalent and have greater richness in species of least concern than those of conservation concern, therefore, pathogen prevalence and richness is likely not the driving cause for species decline, at least when it comes to zoonotic bacteria. In addition, bacterial zoonotic pathogens appear to have a broad host range (μ =13.44, SD =27.97), some even jumping to different classes. Previous research found species of least concern were more likely to have greater zoonotic viral richness than those of conservation concern (Johnson et al., 2020; Keesing and Ostfeld, 2021). Altizer et al., 2007 found that non-threatened primates were more likely to harbor pathogens than threatened primates. The differing results could be due to a variety of factors. We included bacterial pathogens of captive animals, while Altizer et al., 2007 did not. Individuals in captive facilities such as zoos tend to be more researched and monitored for diseases and can also be exposed to more diseases than wild animals due to their proximity to humans and different geographical ranges. Shaw et al., 2020 found pathogen richness in viruses to be more strongly correlated with relatedness to humans than zoonotic bacteria. This could imply zoonotic bacteria have broader host ranges than viruses and are more likely to cross species barriers than zoonotic viruses. Our research covers a broader range of host species, including mammals, birds, and reptiles. Most studies focus primarily on mammals which could have been a factor in comparing bacterial richness in species of conservation concern versus those of least concern. It is apparent that more data is needed to better understand the impact zoonotic pathogen richness has on population status; in particular, hosts of viral, helminth, protozoan, prion, and fungal zoonoses would give us a better picture of the distribution of zoonoses in wildlife populations.
We found signs of disease to be overall lacking in the literature. Only 2,588, approximately 52% of the database, host-pathogen relationships had any sort of description of how the pathogen impacted the host species (Figure 2). 1160 host-pathogens were found to result in the death of the host. 1117 host-pathogen relationships have reported asymptomatic host carriers. Unfortunately, many of these cases are likely incomplete in their assessments. Signs of disease, particularly in chronic cases, can be difficult to observe in wildlife. The pathogen that is responsible Lyme disease, borrelia burgdorferi s.l., was thought to reside asymptomatically in its reservoir hosts, but a field study revealed pathogen infection reduced reproductive success in bank voles (Myodes glareolus)(Cayol et al., 2018). Investigations describing signs of disease in wildlife population will help to better understand the epidemiology and severity of zoonotic bacterial pathogens on wildlife populations.
Widescale disease surveillance in wildlife is difficult, time-consuming, and financially impractical. Identifying specific groups of animals to survey could mitigate some of these problems. Previous studies have investigated the proportion of diseases in wildlife species and found mixed results. Johnson et al., 2020 reviewed viral diseases in mammalian wildlife and found viral richness was highest in the Rodentia, Chiroptera, Primates, Artiodactyla, and Carnivora orders. Mollentze and Streicker, 2020, also reviewed viral diseases in wildlife. Their work surveyed both mammalian and avian classes. Unlike the previous review, they found there was no relationship between Order and viral richness. Olival et al., 2017 found zoonotic viruses in wildlife were proportionally higher in species more closely related to humans. A review of bacterial and viral diseases in wildlife by Shaw et al., 2020 found that pathogen richness does not vary at the order level, and bacterial diseases tended to be more host specialized.
Our results found that of the Genus, Family, and Order taxonomic levels, Order was the best predictor for zoonotic bacterial richness. From a surveillance standpoint, the relationship between pathogen richness is of interest as previous studies have found mixed results. While some studies have found significant diseases in certain orders of animals (Johnson et al., 2020), others have found no relationship (Mollentze and Streicker, 2020; Shaw et al., 2020). Order is an ideal taxonomic group to examine as it has a broader range of species, allowing for a more targeted surveillance effort of wildlife species to identify potential emerging zoonotic pathogens. A breakdown of species richness in each Order found some groups to have significantly higher species richness than others (Figure 4). In particular, the Carnivora and Artiodactyla, orders stand out. The Carnivora order compromised 166 unique species, 100 LC, 57 CC, and 9 DD species. The species with the greatest pathogen richness were the northern raccoon (Procyon lotor) with 43 pathogens, red fox (Vulpes vulpes) with 41 pathogens, and the lion (Panthera leo) with 26 pathogens. The Artiodactyla order is comprised of 166 unique species, 84 LC, 72 CC, and 10 DD. The wild boar (Sus scrofa) has the greatest pathogen richness of this order and the overall greatest pathogen richness in the database with 66 host-pathogen relationships found. Also high on the list red deer (Cervus elaphus) with 35 pathogens, the European roe deer (Capreolus capreolus) with 30 pathogens and the white-tailed deer (Odocoileus virginianus) with 25 pathogens. As mentioned above, Carnivora and Artiodactyla groups have also been found to have a higher viral richness than other groups. Members of these two groups would be ideal for preemptive wildlife disease surveillance and may mitigate future disease surveillance. The wild boar in particular would be an ideal candidate for disease surveillance due to its increasing densities, wide geographic range, and its relatedness to domestic pigs put it more at risk of disease transmission from domestic populations (Pittiglio et al., 2018). Many of these cases are lacking in details of how the pathogen impacts the host, but when information is available many of these hosts-pathogen relationships contain asymptomatic carriers (Table 2). Asymptomatic carriers are more likely to spread these zoonotic pathogens because they have no impact on the hosts health and can therefore move and interact with the environment as normal while spreading the disease. Monitoring asymptomatic carries will help to prevent zoonotic disease spread by identifying zoonotic pathogen presence before it can jump to a more susceptible species.
The other significant relationship we found was between the orders Anseriformes and Squamata. Anseriformes is represented by 62 unique species, 54 LC and 8 CC. The mallard has the greatest pathogen richness (21) of this group. Birds and reptiles are not as widely researched as mammals (Shaw et al., 2020). Many of the species in the Anseriformes order are game species; since these species provide an economic benefit, they are more likely to be researched in terms of diseases they carry that can impact humans. Birds have been researched in terms of avian disease spread, many birds have migratory routes that take them thousands of miles, sometimes across countries. The wide range of these animals, plus the congregations with other species they make during migrations make them ideal for transporting and dispersing pathogens (Peterson et al., 2009). Yet there is a lack of avian information in zoonotic pathogen databases such as this one (Shaw et al., 2020). To get a better idea of how birds spread zoonoses, more research focusing on this group will need to be performed.
Another result of interest was that the Rodentia order had significantly lower bacterial pathogen richness than both Carnivora and Artiodactyla. Species of the Order Rodentia are often implicated as having high pathogen richness, particularly for zoonotic diseases (Han et al., 2015; Keesing and Ostfeld, 2021; Lacher et al., 2021). This database did find high pathogen richness in some species including the house mouse (Mus musculus) to carry 32 different zoonotic bacterial pathogens, the brown rat (Rattus norvegicus) to carry 30, and the house rat (Rattus rattus) to carry 24. In this database, 371 species are represented and 231 of these species have been found with only one zoonotic pathogen. Whether or not other species of Rodentia are capable of contracting many diseases remains to be seen, more disease investigations on the lesser researched species will give a better idea of whether or not Rodentia are capable of contracting and maintaining pathogens or if it is just a few specific species. Focusing disease surveillance efforts on a few specific Rodentia species may be biasing where we observe zoonoses. Further efforts identifying zoonoses in Artiodactyla and Carnivora groups may better our understanding of bacterial pathogen prevalence and dispersal in wildlife populations.
Our research was compiled through a comprehensive literature review of available research on host-pathogen interactions. Therefore, our results are likely biased by research intensity differences among hosts and pathogen groups. For example, a search for “Mycobacterium bovis” and “animal” yielded 4,083 articles on Web of Science, but a search for “Trueperella pyogenes” and “animal” only yielded 240 articles. Pathogen surveillance performed by labs, state agencies, or veterinary facilities that have not reported their findings were not included and likely limited the host or symptom data in our database. When wildlife or bacteria were not identified at the species taxonomic level, they could not be included in the database, meaning some of these host lists are likely larger than portrayed here. Many cases of infection involve multiple pathogens. Clinical signs resulting from co-infections could not be included as they could not be determined to belong to a specific pathogen or were likely exacerbated by the other pathogens. As a result, signs of disease data are missing or lacking for some host-pathogen interactions.
This database can be used to further our understanding of how bacterial zoonoses impacts wildlife populations. While this database only covers zoonotic bacterial pathogens and species of mammalian, avian, and reptilian classes, the protocols used here can be applied to other pathogens and animal groups, filling in the gaps in our zoonotic disease knowledge. Species can be looked at individually for all the zoonotic pathogens they can carry, and signs of disease can be used to assess the risk of pathogen outbreaks in individual populations. Pathogens only found in captive individuals may give us ideas of what to look out for in wild populations. Pathogens with large host ranges that can cross class barriers are identified in this database and may need to be investigated more thoroughly. A number of species have no data available on how pathogen infection impacts the host, future research can be performed to fill in these gaps. The analysis in this paper barely scratches the surface of our understanding of bacterial zoonotic diseases in wildlife, this database can be used as a resource for many future works.
As zoonoses become more prevalent and the biodiversity of wildlife species continues to decline, understanding the presence and richness of zoonoses in wildlife populations is imperative. We found conservation status was a factor in pathogen prevalence, and bacterial zoonoses are significantly more likely to be found in species of least concern versus conservation concern. Species of conservation concern are more at risk of individual species lost; therefore, although species of least concern have greater pathogen prevalence and richness, it is still important to monitor zoonotic pathogen prevalence in populations of conservation concern for endangered species management and recovery. We found Order to be the best indicator of pathogen richness, particularly in the Carnivora and Artiodactyla groups. Future disease surveillance efforts may have better results identifying emerging bacterial zoonoses if they investigate species of these two orders. Our study is limited in scope to zoonotic bacteria and three vertebrate classes; expanding the database to all zoonotic pathogens and vertebrates would give a better understanding of the relationships between wildlife and zoonotic pathogens. Nearly half of the host-pathogen relationships found here were lacking information on how the pathogen impacted the host species, further research needs to be done to fill in the gaps so we may better understand how severe pathogen infections are on wildlife species. Zoonoses pose a threat not only to humans and domestic animals but to wildlife as well. Monitoring the presence of zoonoses and understanding how pathogens impact host species is imperative to maintaining healthy populations.
The datasets presented in this study can be found in online repositories. The names of the repository/repositories and accession number(s) can be found below: https://datadryad.org/stash, doi:10.5061/dryad.n5tb2rc12.
KH and SH contributed to the conception and design of this study. SH provided funding and supervised the project. KH created the database and performed statistical analysis. KH wrote the first draft of the manuscript. Both authors contributed to the article and approved the submitted version.
The author(s) declare financial support was received for the research, authorship, and/or publication of this article. This work, KH and SH was supported by grant funding from the Open Philanthropy Project.
We thank the reviewers for providing valuable feedback for this manuscript.
The authors declare that the research was conducted in the absence of any commercial or financial relationships that could be construed as a potential conflict of interest.
All claims expressed in this article are solely those of the authors and do not necessarily represent those of their affiliated organizations, or those of the publisher, the editors and the reviewers. Any product that may be evaluated in this article, or claim that may be made by its manufacturer, is not guaranteed or endorsed by the publisher.
The Supplementary Material for this article can be found online at: https://www.frontiersin.org/articles/10.3389/fcosc.2023.1218153/full#supplementary-material
Aguirre A. A. (2009). Wild canids as sentinels of ecological health: a conservation medicine perspective. Parasite Vector 2, S7. doi: 10.1186/1756-3305-2-s1-s7
Allsopp M. T., Louw M., Meyer E. C. (2005). Ehrlichia ruminantium: an emerging human pathogen? Ann. N Y Acad. Sci. 1063, 358–360. doi: 10.1196/annals.1355.060
Altizer S., Nunn C. L., Lindenfors P. (2007). Do threatened hosts have fewer parasites? A comparative study in primates. J. Anim. Ecol. 76, 304–314. doi: 10.1111/j.1365-2656.2007.01214.x
Austin B. (2010). Vibrios as causal agents of zoonoses. Vet. Microbiol. 140, 310–317. doi: 10.1016/j.vetmic.2009.03.015
Barroso P., Relimpio D., Zearra J. A., Cerón J. J., Palencia P., Cardoso B., et al. (2023). Using integrated wildlife monitoring to prevent future pandemics through one health approach. One Health 16, 100479. doi: 10.1016/j.onehlt.2022.100479
Bermejo M., Rodríguez-Teijeiro J. D., Illera G., Barroso A., Vilà C., Walsh P. D. (2006). Ebola outbreak killed 5000 gorillas. Science 314, 1564–1564. doi: 10.1126/science.1133105
Bittar F., Keita M. B., Lagier J.-C., Peeters M., Delaporte E., Raoult D. (2014). Gorilla gorilla gorilla gut: a potential reservoir of pathogenic bacteria as revealed using culturomics and molecular tools. Sci. Rep-uk 4, 7174. doi: 10.1038/srep07174
Blanca Mena M. J., Alarcón Postigo R., Arnau Gras J., Bono Cabré R., Bendayan R. (2017). Non-normal data: Is ANOVA still a valid option? Psicothema 29, 552–557. doi: 10.7334/psicothema2016.383
Brand C. J. (2013). Wildlife mortality investigation and disease research: contributions of the USGS national wildlife health center to endangered species management and recovery. Ecohealth 10, 446–454. doi: 10.1007/s10393-013-0897-4
Cayol C., Giermek A., Gomez0Chamorro A., Hytönen J., Kallio E. R., Mappes T., et al. (2018). Borrelia afzelii alters reproductive success in a rodent host. Proc. R. Soc B. 285, 20181056. doi: 10.1098/rspb.2018.1056
CDC: Center for Disease Contorl and Prevention (2022). Available at: https://www.cdc.gov/.
Cheong H. C., Lee C. Y. Q., Cheok Y. Y., Tan G. M. Y., Looi C. Y., Wong W. F. (2019). Chlamydiaceae: diseases in primary hosts and zoonosis. Microorg 7, 146. doi: 10.3390/microorganisms7050146
Cox R., Sanchez J., Revie C. W. (2013). Multi-criteria decision analysis tools for prioritising emerging or re-emerging infectious diseases associated with climate change in Canada. PloS One 8 (8), e68338. doi: 10.1371/journal.pone.0068338
Cunningham A. A., Daszak P. (1998). Extinction of a species of land snail due to infection with a microsporidian parasite. Conserv. Biol. 12, 1139–1141. doi: 10.1046/j.1523-1739.1998.97485.x
Cunningham A. A., Daszak P., Wood J. L. N. (2017). One Health, emerging infectious diseases and wildlife: two decades of progress? Philos. Trans. R. Soc. B Biol. Sci. 372, 20160167. doi: 10.1098/rstb.2016.0167
Ebani V. V., Fratini F., Bertelloni F., Cerri D., Tortoli E. (2012). Isolation and identification of mycobacteria from captive reptiles. Res. Vet. Sci. 93, 1136–1138. doi: 10.1016/j.rvsc.2012.05.006
Everard M., Johnston P., Santillo D., Staddon C. (2020). The role of ecosystems in mitigation and management of Covid-19 and other zoonoses. Environ. Sci. Policy 111, 7–17. doi: 10.1016/j.envsci.2020.05.017
Fawzy A., Rau J., Riße K., Schauerte N., Geiger C., Blom J., et al. (2020). Streptobacillus felis, a member of the oropharynx microbiota of the Felidae, isolated from a tropical rusty-spotted cat. Antonie Van Leeuwenhoek 113, 1455–1465. doi: 10.1007/s10482-020-01454-x
Fischer O., Mátlová L., Bartl J., Dvorská L., Melichárek I., Pavlík I. (2000). Findings of mycobacteria in insectivores and small rodents. Folia Microbiol. 45, 147–152. doi: 10.1007/bf02817414
Flahou B., Modrý D., Pomajbíková K., Petrželková K. J., Smet A., Ducatelle R., et al. (2014). Diversity of zoonotic enterohepatic Helicobacter species and detection of a putative novel gastric Helicobacter species in wild and wild-born captive chimpanzees and western lowland gorillas. Vet. Microbiol. 174, 186–194. doi: 10.1016/j.vetmic.2014.08.032
Frick W. F., Pollock J. F., Hicks A. C., Langwig. K. E., Reynolds D. S., Turner G. G., et al. (2010). An emerging disease causes regional population collapse of a common north American bat species. Science 329, 679–682. doi: 10.1126/science.1188594
Fuke N., Hirai T., Makimura N., Goto Y., Habibi W. A., Ito S., et al. (2016). Non-tuberculous mycobacteriosis with T-cell lymphoma in a red panda (Ailurus fulgens). J. Comp. Pathol. 155, 263–266. doi: 10.1016/j.jcpa.2016.06.001
Gartrell B. D., Alley M. R., Mack H., Donald J., McInnes K., Jansen P. (2005). Erysipelas in the critically endangered kakapo (Strigops habroptilus). Avian Pathol. 34, 383–387. doi: 10.1080/03079450500268583
Gaydos J. K., Balcomb K. C., Osborne R. W., Dierauf L. (2004). Evaluating potential infectious disease threats for southern resident killer whales, Orcinus orca: a model for endangered species. Biol. Conserv. 117, 253–262. doi: 10.1016/j.biocon.2003.07.004
Gcebe N., Hlokwe T. M. (2017). Non-tuberculous mycobacteria in South African wildlife: neglected pathogens and potential impediments for bovine tuberculosis diagnosis. Front. Cell Infect. Mi 7. doi: 10.3389/fcimb.2017.00015
Grant E. H. C., Muths E., Katz R. A., Canessa S., Adams M. J., Ballard J. R., et al. (2017). Using decision analysis to support proactive management of emerging infectious wildlife diseases. Front. Ecol. Environ. 15, 214–221. doi: 10.1002/fee.1481
Grogan L. F., Berger L., Rose K., Grillo V., Cashins S. D., Skerratt L. F. (2014). Surveillance for emerging biodiversity diseases of wildlife. PloS Pathog. 10, e1004015. doi: 10.1371/journal.ppat.1004015
Halliday J. E. B., Allan K. J., Ekwem D., Cleaveland S., Kazwala R. R., Crump J. A. (2015). Endemic zoonoses in the tropics: a public health problem hiding in plain sight. Vet. Rec 176, 220–225. doi: 10.1136/vr.h798
Halliday J. E. B., Meredith A. L., Knobel D. L., Shaw D. J., Bronsvoort BM de C., Cleaveland S. (2007). A framework for evaluating animals as sentinels for infectious disease surveillance. J. Roy Soc. Interface 4, 973–984. doi: 10.1098/rsif.2007.0237
Han B. A., Schmidt J. P., Bowden S. E., Drake J. M. (2015). Rodent reservoirs of future zoonotic diseases. Proc. Natl. Acad. Sci. 112, 7039–7044. doi: 10.1073/pnas.1501598112
Heard M. J., Smith K. R., Ripp K., Berger M., Chen J., Dittmeier J., et al. (2013). Increased threat of disease as species move towards extinction. Conserv. Biol. 27, 1378–1388. doi: 10.1111/cobi.12143
Heaton C. J., Gerbig G. R., Sensius L. D., Patel V., Smith T. C. (2020). Staphylococcus aureus epidemiology in wildlife: A systematic review. Antibiotics 9, 89. doi: 10.3390/antibiotics9020089
Hirst K., Halsey S. (2023). Bacterial zoonosis and clinical symptoms in wildlife. doi: 10.5061/dryad.n5tb2rc12. [DATASET]. Dryad Digital Repository. Version 1.
Johnson C. K., Hitchens P. L., Pandit P. S., Rushmore J., Evans T. S., Young C. C. W., et al. (2020). Global shifts in mammalian population trends reveal key predictors of virus spillover risk. Proc. Biol. Sci. 287, 20192736. doi: 10.1098/rspb.2019.2736
Jones K. E., Patel N. G., Levy M. A., Storeygard A., Balk D., Gittleman J. L., et al. (2008). Global trends in emerging infectious diseases. Nature 451, 990–993. doi: 10.1038/nature06536
Keesing F., Ostfeld R. S. (2021). Impacts of biodiversity and biodiversity loss on zoonotic diseases. Proc. Natl. Acad. Sci. USA 118, e2023540118. doi: 10.1073/pnas.2023540118
Laborda P., Sanz-García F., Ochoa-Sánchez L. E., Gil-Gil T., Hernando-Amado S., Martínez J. ,. L. (2022). Wildlife and antibiotic resistance. Front. Cell Infect. Mi 12. doi: 10.3389/fcimb.2022.873989
Lacher T. E., Kennerley R., Long B., McCay S., Roach N. S., Turvey4 S. T., et al. (2021). Support for rodent ecology and conservation to advance zoonotic disease research. Conserv. Biol. 35, 1061–1062. doi: 10.1111/cobi.13763
Lisle G. W., Kawakami R. P., Yates G. F., Collins D. M. (2008). Isolation of Mycobacterium bovis and other mycobacterial species from ferrets and stoats. Vet. Microbiol. 132, 402–407. doi: 10.1016/j.vetmic.2008.05.022
Lopes B. C., Maciel A. L. G., Loiko M. R., Bueno T. S., Coppola M. M., Bertagnolli A. C., et al. (2021). Molecular identification of Mycobacterium spp. isolated from Brazilian wild boars. Mol. Biol. Rep. 48, 1025–1031. doi: 10.1007/s11033-020-06118-7
Luis A. D., Hayman D. T. S., O’Shea T. J., Cryan P. M., Gilbert A. T., Pulliam J. R. C., et al. (2013). A comparison of bats and rodents as reservoirs of zoonotic viruses: are bats special? Proc. R. Soc. B Biol. Sci. 280, 20122753. doi: 10.1098/rspb.2012.2753
Merck Manual (2022) Merck Manual Veterinary Manual. Available at: https://www.merckvetmanual.com/.
Messenger A. M., Barnes A. N., Gray G. C. (2014). Reverse zoonotic disease transmission (Zooanthroponosis): A systematic review of seldom-documented human biological threats to animals. PloS One 9, e89055. doi: 10.1371/journal.pone.0089055
Mollentze N., Streicker D. G. (2020). Viral zoonotic risk is homogenous among taxonomic orders of mammalian and avian reservoir hosts. Proc. Natl. Acad. Sci. 117, 9423–9430. doi: 10.1073/pnas.1919176117
O’Brien S. J., Evermann J. F. (1988). Interactive influence of infectious disease and genetic diversity in natural populations. Trends Ecol. Evol. 3, 254–259. doi: 10.1016/0169-5347(88)90058-4
Ochoa S., Collado L. (2021). Enterohepatic Helicobacter species – clinical importance, host range, and zoonotic potential. Crit. Rev. Microbiol. 47, 1–34. doi: 10.1080/1040841x.2021.1924117
Odoi J. O., Ohya K., Moribe J., Takashima Y., Sawai K., Taguchi K., et al. (2020). Isolation and antimicrobial susceptibilities of nontuberculosis mycobacterium from wildlife in Japan. J. Wildlife Dis. 56, 851–862. doi: 10.7589/2019-10-261
Okaro U., Addisu A., Casanas B., Anderson B. (2017). Bartonella species, an emerging cause of blood-culture-negative endocarditis. Clin. Microbiol. Rev. 30, 709–746. doi: 10.1128/cmr.00013-17
Olival K. J., Hosseini P. R., Zambrana-Torrelio C., Ross N., Bogich T. L., Daszak P. (2017). Host and viral traits predict zoonotic spillover from mammals. Nature 546, 646–650. doi: 10.1038/nature22975
Ostfeld R. S. (2009). Biodiversity loss and the rise of zoonotic pathogens. Clin. Microbiol. Infect. 15, 40–43. doi: 10.1111/j.1469-0691.2008.02691.x
Parola P., Paddock C. D., Socolovschi C., Labruna M. B., Mediannikov O., Kernif T., et al. (2013). Update on tick-borne rickettsioses around the world: a geographic approach. Clin. Microbiol. Rev. 26, 657–702. doi: 10.1128/cmr.00032-13
Peterson A. T., Andersen M. J., Bodbyl-Roels S., Hosner P., Nyári A., Oliveros C., et al. (2009). Prototype forecasting system for bird-borne disease spread in North America based on migratory bird movements. Epidemics. 1, 240–249. doi: 10.1016/j.epidem.2009.11.003
Pitcher D. G., Nicholas R. A. J. (2005). Mycoplasma host specificity: Fact or fiction? Vet. J. 170, 300–306. doi: 10.1016/j.tvjl.2004.08.011
Pittiglio C., Khomenko S., Beltran-Alcrudo D. (2018). Wild boar mapping using population-density statistics: From polygons to high resolution raster maps. PloS One 13 (5), e0193295. doi: 10.1371/journal.pone.0193295
R Core Team. (2022). R: A language and environment for statistical computing. Vienna, Austria: R Foundation for Statistical Computing. Available at: https://www.R-project.org/.
Rabinowitz P. M., Gordon Z., Holmes R., Taylor B., Wilcox M., Chudnov D., et al. (2005). Animals as sentinels of human environmental health hazards: an evidence-based analysis. Ecohealth 2, 26–37. doi: 10.1007/s10393-004-0151-1
Rahim Z., Thapa J., Fukushima Y., Zanden A. G. M., Gordon S. V., Suzuki Y., et al. (2017). Tuberculosis caused by mycobacterium orygis in dairy cattle and captured monkeys in Bangladesh: a new scenario of tuberculosis in south asia. Transbound Emerg. Dis. 64, 1965–1969. doi: 10.1111/tbed.12596
Raymond J. T., Tell L., Bush M., Nichols D. K., Schulman F. Y., Montali R. J. (2000). Subcutaneous atypical mycobacteriosis in captive tiger quolls (Dasyurus maculatus). Vet. Pathol. 37, 137–142. doi: 10.1354/vp.37-2-137
Rudenko N., Golovchenko M., Grubhoffer L., Oliver J. H. (2011). Updates on Borrelia burgdorferi sensu lato complex with respect to public health. Ticks Tick-borne Dis. 2, 123–128. doi: 10.1016/j.ttbdis.2011.04.002
Russell R. E., Tripp D. W., Rocke T. E. (2019). Differential plague susceptibility in species and populations of prairie dogs. Ecol. Evol. 9, 962–11971. doi: 10.1002/ece3.5684
Shaw L. P., Wang A. D., Dylus D., Meier M., Pogacnik G., Dessimoz C., et al. (2020). The phylogenetic range of bacterial and viral pathogens of vertebrates. Mol. Ecol. 29, 3361–3379. doi: 10.1111/mec.15463
Shopland S., Barbon A. R., Rodriguez A., Richardson D. S., Barrows M. (2021). Health screening of the european endangered species program captive population of the pink pigeon (Nesoenas mayeri). J. Zoo Wildlife Med. 51, 970–980. doi: 10.1638/2020-0018
Sousa K. C. M., Herrera H. M., Rocha F. L., Costa F. B., Martins T. F., Labruna M. B., et al. (2018). Rickettsia spp. among wild mammals and their respective ectoparasites in Pantanal wetland, Brazil. Ticks Tick-borne Dis. 9, 10–17. doi: 10.1016/j.ttbdis.2017.10.015
Tardón A., Bataller E., Llobat L., Jiménez-Trigos E. (2021). Bacteria and antibiotic resistance detection in fractures of wild birds from wildlife rehabilitation centres in Spain. Comp. Immunol. Microbiol. Infect. Dis. 74, 101575. doi: 10.1098/rstb.2001.0888
Taylor L. H., Latham S. M., Woolhouse E. J. (2001). Risk factors for human disease emergence. Phil. Trans. R. Soc. Lond. 356 (1411), 983–989. doi: 10.1098/rstb.2001.0888
Wang Z., Wang P., An J. (2016). Zika virus and Zika fever. Virol. Sin. 31, 103–109. doi: 10.1007/s12250-016-3780-y
Yap T. A., Koo M. S., Ambrose R. F., Wake D. B., Vredenburg V. T. (2015). Averting a North American biodiversity crisis. Science 349, 481–482. doi: 10.1126/science.aab1052
Keywords: zoonosis, wildlife, conservation, bacteria, disease
Citation: Hirst KM and Halsey SJ (2023) Bacterial zoonoses impacts to conservation of wildlife populations: a global synthesis. Front. Conserv. Sci. 4:1218153. doi: 10.3389/fcosc.2023.1218153
Received: 06 May 2023; Accepted: 06 November 2023;
Published: 23 November 2023.
Edited by:
Govindhaswamy Umapathy, Centre for Cellular & Molecular Biology (CCMB), IndiaReviewed by:
Benjamin Mubemba, Copperbelt University, ZambiaCopyright © 2023 Hirst and Halsey. This is an open-access article distributed under the terms of the Creative Commons Attribution License (CC BY). The use, distribution or reproduction in other forums is permitted, provided the original author(s) and the copyright owner(s) are credited and that the original publication in this journal is cited, in accordance with accepted academic practice. No use, distribution or reproduction is permitted which does not comply with these terms.
*Correspondence: Kristen M. Hirst, a21oazRuQHVtc3lzdGVtLmVkdQ==
Disclaimer: All claims expressed in this article are solely those of the authors and do not necessarily represent those of their affiliated organizations, or those of the publisher, the editors and the reviewers. Any product that may be evaluated in this article or claim that may be made by its manufacturer is not guaranteed or endorsed by the publisher.
Research integrity at Frontiers
Learn more about the work of our research integrity team to safeguard the quality of each article we publish.