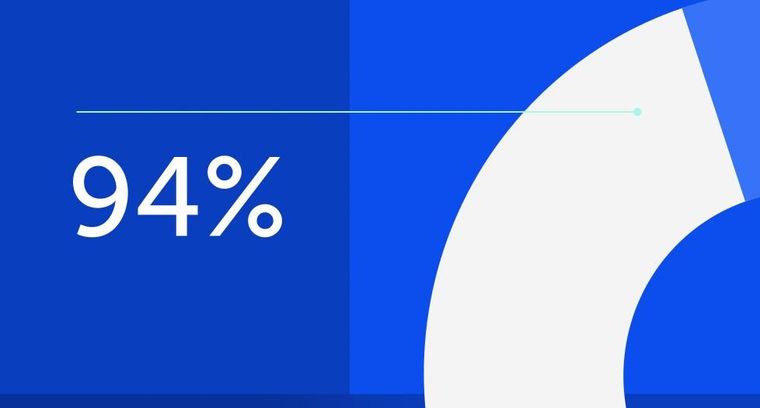
94% of researchers rate our articles as excellent or good
Learn more about the work of our research integrity team to safeguard the quality of each article we publish.
Find out more
ORIGINAL RESEARCH article
Front. Conserv. Sci., 11 October 2022
Sec. Human-Wildlife Interactions
Volume 3 - 2022 | https://doi.org/10.3389/fcosc.2022.999195
Negative interactions between humans and large carnivores like tigers (Panthera tigris) and leopards (Panthera pardus) are of concern for the conservation of these carnivores, as well as for the health and wellbeing of people who experience such interactions routinely. Such interactions not only lead to human deaths, injuries and loss of domestic animals, but also can result in retaliatory persecution of carnivores and cause their population declines. The Dudhwa-Pilibhit landscape in the northern Indian state of Uttar Pradesh is popularly regarded to be the second most affected hotspot of human-tiger conflicts in the country. Yet, very little information is published on human-tiger conflicts in this landscape. In this study, we recorded 7.4 ± 5.8 (range: 1-20) negative interactions per year between people and tigers that led directly to human deaths and injuries. Although there appeared to be peaks in negative interactions in more recent years, these were found to be statistically non-significant. The peaks in the numbers of cases in 2009-10 and 2016-17 can be attributed to higher numbers of human casualties cause by individual ‘problem’ tigers, and not due to an increase in the number of tigers engaging in conflicts. We used binomial generalized linear modelling to model the risk to humans from being attacked by tigers depending on landscape characteristics. This approach demonstrated that the mosaics of forests and human settlements, especially the presence of agriculture, forest patches and waterbodies, were the predominant factors at play. Notably, higher risks were not mediated by the presence of larger cattle population. Proximity of villages to forests or natural vegetation patches was the most significantly contributing factor identified by the model output. This suggested that despite the prevalent perception of attacks on humans occurring in human-dominated areas of this landscape, areas with forests or other natural vegetation entailed higher risks. This was corroborated by field observations, with most attacks occurring within, or close to, forests or dense vegetation. Based on these findings, we recommend that restricting human movements and taking precautionary measures in high-risk areas can significantly reduce negative interactions with tigers in our study area.
Large carnivores maintain large home-ranges (Gittleman and Harvey, 1982; Nowell and Jackson, 1996), and in densely populated countries like India they are expected to come into contact with humans (Karanth and Gopal, 2005; Inskip and Zimmermann, 2009; Carter and Linnell, 2016). In the absence of suitable prey rich habitats or availability of easy prey, such interactions often turn negative (Treves and Karanth, 2003; Goodrich, 2010). Large carnivores residing or moving through human-dominated landscapes are widely known to predate on livestock (Madhusudan, 2003; Wilson et al., 2005; Valeix et al., 2012; Miller et al., 2016; Carter and Linnell, 2016), and, to a lesser degree, cause human fatalities and injuries (Gurung et al., 2008; Goodrich, 2010; Dhanwatey et al., 2013; Packer et al., 2019). When left unmanaged, such scenarios may lead to enhanced antagonism between local people and carnivores (Treves and Bruskotter, 2014; Zimmermann et al., 2020), which can lead to retaliatory killings (Woodroffe and Ginsberg, 1998; Inskip and Zimmermann, 2009) and poaching for illegal wildlife trade (Tilson et al., 2010; Moreto, 2019).
Management strategies should focus on mitigation of crucial factors elevating human-carnivore conflicts. At an ultimate level, the destruction and degradation of natural habitats are widely accepted as the drivers of conflicts between humans and large carnivores around the world (Sillero-Zubiri and Laurenson, 2001; Naughton-Treves et al., 2003; Graham et al., 2005). Nevertheless, within landscapes where humans and large carnivores have co-occurred for extended periods, more proximate factors may also drive such conflicts (Treves et. al., 2004; Miller et al., 2016). Various environmental factors, such as human density, habitat fragmentation, and availability of prey, water and other resources can all be associated with conflicts (Wilson et al., 2005; Atwood and Breck, 2012; Valeix et al., 2012; Dhanwatey et al., 2013; Packer et al., 2019). Such factors can play an even larger role in determining and predicting conflicts in human-modified areas with mosaics of multiple land-use types.
In India, tigers (Panthera tigris) widely co-occur with humans, especially around well-protected tiger reserves (Post and Pandav, 2013). Successful conservation and management efforts within these tiger reserves have resulted in increased population sizes and densities of tigers (Jhala et al., 2020), leading to a higher number of dispersing individuals from these protected habitats (Smith, 1993; Karanth and Gopal, 2005; Lamichhane et al., 2017). Consequently, the fringe areas of many tiger reserves are fraught with incidents of human-tiger conflicts, often leading to the retaliatory killing of tigers. Retaliatory killing and poaching for profit are the biggest threats to tigers in the country (Miquelle et al., 2005; Habib et al., 2017; Dhungana et al., 2018). Persistent conflicts between humans and tigers may also increase the risk of poaching, as people become antagonistic towards tigers, their habitats, and the management that works towards conserving them. While human-tiger conflicts primarily manifest in the form of livestock depredation, attacks on humans are also widespread and increasing in recent times throughout many range states of India (Karanth and Gopal, 2005; Goodrich, 2010; Dhanwatey et al., 2013; Chatterjee et al., 2017). While securing human lives in such interactions is of paramount importance, both morally and politically, it is also important for conservation of tigers, as human fatalities and injuries are the strongest drivers capable to further aggravate human retribution and resentment (Saberwal, 1997; Tilson and Nyhus, 1998; Gulati et al., 2021). Perceived risks from predators increase dramatically with the loss of human lives (Bhattarai and Fischer, 2014), inciting public agitations, damages to government property, retaliatory killings of animals involved, and violence against park managers and wildlife wardens (Pers Obs1*; and e.g. https://www.dailymail.co.uk/news/article-6353363/Indian-villagers-crush-tigress-death-killed-man.html).
Tiger attacks on humans have been broadly understood to be naturally accidental (Seidensticker and McDougal, 1993), although some independent cases indicate a more consistent trend. Like livestock depredation is suggested to result from an interplay of predator presence, land-use, vegetation structure and livestock availability (Miller, 2015), attacks on humans may also be mediated by a variety of factors. It is crucial to clearly understand what factors could potentially lead to higher frequencies of negative human-tiger interactions irrespective of victim activities prior to incidents and the mere presence of tigers and humans in the landscape. Since tigers and other carnivores can track their prey, including livestock, well beyond natural habitats (Sunquist, 2010), spatial modeling has been widely employed to assess risks of livestock depredation and human-carnivore conflicts across large landscapes (Treves et al., 2004; Amirkhiz et al., 2018), also incorporating predator-prey dynamics into the model framework (Miller, 2015). In case of tiger attacks on humans, certain landscape features may increase an overlap of areas used by humans and tigers, increasing the chances of such encounters (e.g. Nyhus and Tilson, 2004; Warrier et al., 2020). Furthermore, tigers and humans respond differently to the presence or absence of different landscape elements. For instance, tigers in the Russian Far East reportedly avoid areas with deep snow as it makes plodding noisy and exhausting (Heptner & Sludskii, 1992, cf. Sunquist, 2010). Such behavioural flexibility may not only influence the probability of a particular interaction between humans and tigers, but also its chance to turn into an attack. Thus, understanding landscape factors predisposing people to tiger attacks is vital to identify high-risk areas, allowing for site-specific management interventions to minimize such incidents.
Here we analyse data on tiger attacks on humans collected over a decade in the Dudhwa and Pilibhit Tiger Reserve landscape in the northern Indian state of Uttar Pradesh. It comprises the last stronghold of the Bengal tiger in the state and is one of the major tiger populations in the Terai ecosystem of the country, harbouring 172 ± 19 adult tigers (Jhala et al., 2020). We attempted to discern what elements of the landscape best predict the probability of tiger attacks on humans. Specifically, we investigated how various land-use and land-cover elements influence the occurrence of attacks on humans by tigers in the study area. We hypothesised that tiger attacks on humans would be best explained by an interplay of distances to protected forests and human settlements, presence of large cattle population in villages, and presence of forest patches acting as refuges for tigers moving out of protected forest habitats. We hope that results of our study will help managers better protect tigers and foster their coexistence with local people by mitigating negative human-tiger interactions.
Dudhwa (28° 30’ 30” N, 80° 40’ 48” E) and Pilibhit (28°38’17.00”N 79°57’18.12”E) Tiger Reserves are located in the Terai-Bhabar belt of northern Uttar Pradesh (Figure 1), across the districts of Pilibhit, Lakhimpur Kheri, Bahraich and Shahjahanpur, and form one of the last strongholds of wild tigers in the state. These protected areas are also part of Level 1 and Level 2 Tiger Conservation Units (TCU) in northern India (Wikramanayake et al., 2011). Dudhwa Tiger Reserve is connected with Shukla-Phanta National Park in Nepal through Dudhwa National Park and with Bardia National Park in Nepal through Katerniaghat Wildlife Sanctuary. Similarly, Pilibhit Tiger Reserve is linked with Kishanpur Sanctuary of Dudhwa Tiger Reserve as well as Shukla Phanta National Park in Nepal.
Figure 1 Study area with the presence and pseudo-absence locations of tiger attacks on humans. Inset: location of study area in India.
The terrain and vegetation of these areas are typical of the Terai Bhabar belt, including wet alluvial grasslands, swamp low forests, alluvial savannah woodlands, moist deciduous forests and plain Sal forests (Champion and Seth, 1968; State of Forest Report, 2011). The primary land-use surrounding the forest patches of protected areas and fragmented natural landscapes constitutes agricultural expanses, predominated by sugarcane, with villages, hamlets and small towns harbouring a high human density of around 500 people per km2 (Chandramouli & General, 2011).
Incidents of tiger attacks on humans in this landscape were recorded from 2003 to 2018. For incidents preceding the year 2009, records were collected from various range offices of the Forest Department (Supplementary Table T1) and were verified post-hoc through interviews with surviving victims, next of kin, or eyewitnesses present. From 2009 onwards, data was collected through the information relayed to the existing Rapid Response Teams (RRT) operated by Wildlife Trust of India in the landscape. The RRTs operate in the landscape under the aegis of the Uttar Pradesh State Forest Department to assist in addressing human-big cat conflict situations. The team comprises a trained wildlife biologist, a sociologist, and a veterinarian, who are tasked with investigating every reported case and taking action to resolve it (Chatterjee et al., 2017). All the accumulated information was verified and further enhanced by interviewing victims (survivors of attacks), eyewitnesses or close family members of the victim by the RRT sociologist. In cases occurring post-2009, each case was also corroborated using evidence of animal presence such as pugmarks and scats, as well as camera trap captures, in and around the incident location by the RRT biologist. The exact locations of attacks were recorded using a handheld GPS (Garmin Vista H/Garmin Etrex 10). For cases before 2009, locations were either recorded after being taken to the spot by victims/eyewitnesses/victim’s relatives or inferred through broad locational information provided, such as distance from the village, compass bearing, forest beat (smallest functional management unit) etc. Various data pertaining to the activity and site of the attacked victim (Table 1) were collected through structured interviews and recorded on preformatted data sheets.
Owing to the difficulty of acquiring true conflict absence points (where tigers are present, but no attack occurred), 1000 random points were generated from the landscape as proxy for the absence of conflicts (pseudo-absence). These points were randomly generated across an estimated buffer region in the study area using ArcGIS 10.6.1 software (ESRI, Redlands USA). This buffer region was estimated through an averaging process of the farthest distances of conflict incidents across the study area.
For each conflict presence and pseudo-absence location, the following continuous predictor variables were considered: distance to the edge of the nearest forest patch (m), distance to the nearest settlement cluster (m), distance to the nearest waterbody (m), total number of settlements, proportion of forest cover, and population of cattle (cows and buffaloes only). Besides these, the following binary predictors were also included: presence of waterbody, presence of forest patch, and presence of agriculture. For all the predictor variables, except the distance to forest edge, environmental parameters were extracted from within a 4 km2 grid cell around each conflict presence and pseudo-absence location. All distances were manually calculated using Google Earth Pro ruler and time slider tool (for recorded incidents, images of corresponding years were used).
Satellite images of Sentinel 2 MSI scene taken over the area on 20 March 2018, with a ground resolution of 10 m was used and classified using Cognition 64 9.0 software with a composite of 10 image sensing bands (Blue, Green, Red, Vegetation Red Edge I, II & III, Near Infrared (NIR), Narrow NIR, Short-Wave Infrared (SWIR) I & II). Object oriented classification was performed to classify the image. This included grouping of pixels that are similar to one another based on the spectral properties, i.e. shape, size, texture and context, from the neighbouring pixels.
The same classes were assigned to the set of similar spectral signature objects verified via Google Earth Pro, such that all these objects accurately represented a particular land-cover class of interest. For simplification, broad eight land-use classes – forest, grassland, waterbody, sedimentation, wetland, barren land, agriculture and settlements – were retained for the final classification. The nearest neighbourhood plugin for QGIS 3.4.1 was used to compute the shortest distance from each conflict presence point and pseudo-absence location to the environmental parameters.
The number of cattle (cows and buffaloes together) was measured in 79 villages out of 112 sampled villages, 84 of which had recorded in total ≥ 2 incidents, including cattle depredation, with tigers in the past. Data was collected through interviews with the village sarpanch (head woman/man) if they had a recorded tally of current cattle population in their villages. This information was then utilised to estimate the cattle population for additional villages in the landscape. To do this, the approximate area bound within the discernible village periphery (for 79 villages) was calculated using the ruler function in Google Earth Pro. A significant Spearman correlation (ρ = 0.776; p < 0.001) was observed between the village size and the cattle number in 79 villages, and their data were subjected to a linear regression analysis to produce an equation between these variables. From our field experience, cattle densities and space use were similar in all villages and we assumed that the number of cattle would linearly increase with a larger space. The derived equation (cattle number = 2140.50 + 128.61*village area (ha); R2 = 0.61) was then used to predict cattle numbers for additional villages.
We conducted a standard normal homogeneity test to test for significance of the fluctuations across years, using the snh.test function in “trend” package (Pohlert, 2020) in R 3.5 (R Core Team, 2018). The conflict presence and pseudo-absence served a binomial response variable. We employed a binomial Generalized Linear Model (GLM) in our analysis (Nelder and Wedderburn, 1972), as GLM allows to model different data types together as covariates and/or factors affecting the response variable and allows for a non-normal distribution of the response variable (Zuur et al., 2009).
We built single and additive logistic regressions with nine habitat predictor variables. Among these, the presence of waterbodies, agriculture, and forest were categorical, while the remaining six variables were continuous. The continuous variables were scaled and normalised to a scale of 0-1 using the min-max normalization (Gökhan et al., 2019). We also calculated the variance inflation factor (VIF) to test for collinearity in the predictor variables. We fixed a cut-off VIF value of 3 corresponding to a R2 value of 0.67. A higher VIF value (~10) was not used as it can reflect only very high correlation (R2 ~ 0.9), while a lower cutoff can be used to evaluate moderate multicollinearity among the variables. The best-fit models were selected based on Akaike Information Criterion corrected for small sample size (AICc) using a ΔAICc < 2 criterion (Burnham and Anderson, 1998). The area under the Receiver Operating Curve (AUC area) was estimated to evaluate the predictive accuracy of the best-fit models. The analysis was done in the “pROC” package (Robin et al., 2011) in R 3.5 (R Core Team, 2018). We also estimated the accuracy of the models using the k-fold cross-validation in “caret” package (Kuhn, 2008) in R 3.5 (R Core Team, 2018). We used a 5-fold cross-validation and repeated each fold for 5 times giving us 25 different combinations of training and test data. Model averaging was carried out when more than one model was found to be satisfying the ΔAICc < 2 criterion (Burnham and Anderson, 1998). Analysis was performed in “MuMIn” package (Barton, 2009) in R 3.5 (R Core Team, 2018).
For developing a risk model map for all the variables selected by the GLM model, raster layers were created using the spline tool. The principal use of the spline tool is to interpolate raster layers by using mathematical functions that estimate values and minimize overall surface curvature, resulting in a smooth surface that moves exactly through the input points. Further, the coefficients of each variable (as computed in the final averaged GLM model) were multiplied by the respective raster layer using the raster calculator tool in ArcGIS (ArcGIS 10.8, ESRI). The final risk model output was derived by overlaying these raster layers using the map algebra tool. The outputs (estimated probabilities) generated by the risk model were classified into 4 categories: very high risk (> 0.75), high risk (0.75 – 0.51), moderate risk (0.50 – 0.25) and low risk (< 0.25).
A total of 118 incidents of negative human-tiger interactions were recorded in the study area from 2003 to 2018, with an annual average of 7.4 ± SD 5.8 (Range: 1-20) incidents involving human victims.
The data on these interactions exhibits two major peaks – the first during 2009-2010 and the second during 2016-2017 (See Figure 2). These peaks were found to be statistically non-significant, and the standard normal homogeneity test suggested the distribution to be homogenous (TSNH = 4.1, p = 0.343), indicating that the peaks may simply represent random fluctuations.
Figure 2 Number of recorded cases (N = 118) of tiger attacks on humans across years from 2003 to 2018.
Most (73.7%, n = 87) of the cases resulted in the death of the victim, with 80.5% (n = 95) of the victims being males and the rest females. The victims had a mean age of 38.7 ± 16.2 years. Almost half (43.2%, n = 51) of attacks were recorded to have occurred in agricultural fields, primarily sugarcane, while 44.9% (n = 53) occurred inside forests. The remaining 11.9% (n = 14) occurred either inside village boundaries (excluding farmlands) or inside homesteads.
In 66.1% (n = 78) of the cases recorded victims were standing or walking when attacked, while in 28.0% (n = 33), victims were either crouching or lying down when attacked. In 67.8% (n = 80) of the cases, the victims were alone when attacked, 13.6% (n = 16) occurred when the victim was accompanied by another person, and in 16.9% (n = 20) the victims were accompanied by two or more persons. For two cases, the exact details about people’s presence could not be determined.
The majority of tiger attacks on humans occurred in the morning (04:00–11:59, 29.7%, n = 35) and afternoon (12:00–15:59, 34.8%, n = 41) hours. Almost quarter (24.6%, n = 29) of the incidents occurred in the evening (16:00–18:59) and only few cases (9.3%, n = 11) were recorded at night (19:00–03:59). Most of the cases (40.7%, n = 48) occurred in the winter season (November–February), followed by monsoons (July–October, 34.8%, n = 41), and the summer season (March–June, 24.6%, n = 29).
We excluded the number of settlements from the analysis because of its multicollinearity (VIF = 6.23). The best models were four (Table 2) and the output of their averaged model is presented in Table 3. In the averaged model, all the variables except the presence of agriculture (p = 0.200), cattle number (p = 0.180) and proportion of forest (p = 0.823) were found to significantly affect the presence of conflict locations. The AUC area was 0.854, indicating a moderately high capacity to accurately predict the locations of tiger attacks (Figure S2). The cross-validation results also reflected the robustness of our model as the average accuracy of the k-fold cross-validation was 0.892 ± SD 0.011. The distance to forest (z = 7.42, p < 0.001) (Table 3) and distance to settlements (z = 4.86, p < 0.001) were most significantly associated with conflict locations (Figure 3). As the distance to forest (Odds ratio = 10-8) and the distance to settlements (Odds ratio = 0.151) decreased, the probability of attacks increased significantly. This aligned with our hypothesis and distance to forest also had the highest importance followed by the distance to settlements and the presence of waterbodies (Odds ratio = 0.145) among all the predictor variables. Moreover, the conflict location was also negatively related with the distance to waterbody (z=2.52, p=0.01).
Figure 3 Relationships between the probability of tiger attacks on humans and predictors estimated from the averaged model. The dotted line represents a 95% confidence interval and the solid line is a relationship curve estimated from the model. Distances are given in metres.
The model output suggests moderate to low probabilities of people getting attacked by tigers over much of the landscape, both inside and outside protected areas (Figure 4). Several high-risk zones were delineated in four protected areas, including Pilibhit (risk probability = 0.56), Dudhwa (0.70), Katerniaghat (0.57) as well as Kishanpur (0.57) and South Kheri (0.55). The very high-risk zones were predicted near the southern end and northern edges of Katerniaghat (risk probability = 0.83), South Kheri (0.94) and Pilibhit (0.89).
Conservation of large carnivores like tigers imposes costs on local communities, especially where they extensively share space. This cost, mainly in the form of loss of life and property (cattle) and changes in lifestyle thereof, is levied on local communities disproportionately as carnivore populations thriving due to intensive conservation efforts further aggravate conflicts with local communities (Saberwal, 1997; Tilson and Nyhus, 1998). Loss of human lives and injuries to humans in particular have been suggested to levy significantly higher costs (Gulati et al., 2021) and thus drive much stronger conflicts with people (Bhattarai and Fischer, 2014) compared to cattle and other property loss.
In our study area, both tigers and leopards regularly come into conflict with humans living around protected forests and forest fragments. Conflicts with tigers alone were responsible for the death of 87 people between 2009-2018 (Chatterjee et al., 2017). The landscape is also rife with cases of cattle loss due to tigers, although this is poorly documented. The best estimates, based on verified records, report 474 cattle losses between 2003 and 2012 (Chatterjee et al., 2017). This is comparable with earlier reports – 161 human deaths and 511 cattle losses for a twenty-year period spanning from 1990 to 2009 (Chauhan, 2011). Although an average of 7.4 ± 5.8 attacks on humans per year in our study appears to be significantly lower than annual average conflict cases recorded from other high conflict areas like the Sundarban Tiger Reserve in West Bengal, India (27.4 ± 12.0 attacks on humans per year) (Chatterjee et al., 2022), consistent cases of tiger attacks on humans seriously jeopardise long-term tiger conservation efforts in this landscape (Inskip et al., 2013; Bhattarai and Fischer, 2014). While the temporal distribution of attacks on humans across years appeared to have two major peaks, these changes were found to be statistically nonsignificant. This is understandable, as in these years individual man-eaters led to multiple attacks on humans within short spans of time. In 2009, two man-eaters who had killed nearly 12 people were caught and eliminated, while in 2016 and 2017 two more adult males identified to have attacked and killed/maimed 18 people were also captured and removed by the RRT and the Forest Department.
The distances to forest, waterbody and human settlement, and the presence of waterbody, best explained the occurrence of tiger attacks on humans in our study. With the distance to forest gaining more importance in the model output, it is suggestive that attacks on humans by tigers are more likely to occur when people venture inside or are close to the forests (highest at around 2.68 km inside the forests). The model suggests that the probability of getting attacked increased sharply at about 900 m and closer distances to the forest (Figure 3). This is similar to findings of other studies on tigers (Gurung et al., 2008; Dhanwatey et al., 2013; Miller, 2015; Dhungana et al., 2018). A confounding inverse relationship with the distance to villages – increasing conflicts with decreasing distances to villages – is that attacks are most likely near villages closer to forests rather than those farther away. This is plausible, as vulnerable villages are the ones situated closer to forests and most likely existing amidst the mosaic of crop fields and forest fragments, exposing their populations to more frequent interactions with tigers (Nyhus and Tilson 2004; Singh et al., 2015; Dhungana et al., 2018).
The presence of, and distances to, waterbodies were also significantly related to tiger attacks on humans, with the probability of conflicts increasing with decreasing distance to water sources in drier areas with fewer waterbodies. This appears intuitive as tigers are amongst a few felid species with a strong affinity to water, widely known to utilize waterholes for cooling off in hot weather (Sunquist, 2010).
There are over 10,000 human settlements in our study area, mostly villages and their hamlets, widely dispersed across the landscape at varying distances from protected forests and invariably surrounded by swathes of agricultural fields. This mosaic is also interspersed with forest and riverine vegetation patches of varying sizes. Tigers that move out of protected forests could possibly seek out these small, forested patches mixed with tall sugarcane crops as temporary refuges (Warrier et al., 2020). Interactions occurring in or close to such areas could potentially lead to attacks (Packer et al., 2019), rather than farther away from forested or heavily vegetated areas closer to villages and towns, where tigers would intuitively be more wary of human presence and thus actively avoid interactions. In four observed cases in this landscape during the study period, where individual tigers had ventured > 200 km away from forested areas, not a single human had been attacked and killed (Pers. Obs.1*).
Also, most of the tiger attacks on humans in our study (n = 104; 88.1%) were accidental in nature or self-defensive as being provoked by humans. Only 14 human deaths (11.9%) between 2003 to 2018 were associated with actual man-eating, with circumstantial evidence pointing at four individual tigers actively hunting humans as prey. These were further corroborated with the cessation of attacks after removal of the involved tigers either by capture or elimination. Attacks could happen when humans stumbled upon resting tigers or tigers would have mistaken humans for wild prey accidentally, and the chances of such encounters were most common when victims were alone (67.8% of recorded cases).
Our study shows that tiger attacks on humans did not increase with higher numbers of cattle. Also, as encounters were minimum at night (9.3% of recorded cases), it is suggestive that tigers did not seem to track cattle deliberately, nor sought humans at night when tigers are most active (Sunquist, 2010; Mondal et al., 2012) but humans generally stay within settlements.
Our risk model outputs delineated specific areas within the landscape where the risks of tiger attacks on humans are predictably higher compared to other areas. A majority of high and very high-risk zones were identified around Pilibhit Tiger Reserve and Katerniaghat Wildlife Sanctuary, with some also located in and around South Kheri Forest Division and Dudhwa National Park. Delineation of such areas may help park managers and conservation organisations focus different mitigation activities within these areas in order to minimize losses of human lives and livelihoods.
Our findings have major implications for tiger conservation in this landscape. Firstly, they demonstrate that annual numbers of tiger attacks on humans cannot indicate temporal patterns as many attacks can be made by few individual tigers. Further, we identified that tiger attacks on humans increase in areas closer to forest and waterbodies with nearby settlements. While this may appear obvious, it should be noted that tigers in this landscape have been widely recorded to travel long distances away from protected areas into densely populated human-dominated areas (Chatterjee et al., 2017) yet most of attacks are recorded either within the forest or close to it. Although it requires further individual-based studies, it is plausible that within, or closer to, forest tigers are less cautious of human beings as it is their natural habitat (with many being born and raised there), but when venturing out into more distant and unknown lands, tigers become more cautious and avoid interactions with humans as far as possible.
Second, we suggest that besides these habitat characteristics, specific human behaviors such as venturing alone into or near the forest, or carrying out activities in a crouching or standing stance, may further increase chances of tiger attacks. Placement of warning or precautionary signs and regular patrolling of staff to gather signs of tiger presence may also inform local people on how to minimize or avoid chance encounters with tigers. The lack of prominent temporal patterns and the significance of certain habitat features in conflict mediation are indicative of habitat fragmentation as a major driver of human-tiger conflict in our study area. Ensuring proper connectivity between habitats through focused land reclamation and habitat recovery is expected to mitigate human-tiger conflicts and secure the long-term survival of tigers.
The raw data supporting the conclusions of this article will be made available by the authors, without undue reservation.
Ethical review and approval was not required for the study on human participants in accordance with the local legislation and institutional requirements. Written informed consent for participation was not required for this study in accordance with the national legislation and the institutional requirements.
MC has led the overall research work from its conceptualization, data collection, analysis as well as drafting of the manuscript. NC and TB have analyzed the data, generated the GLM model results and also have co-written the manuscript. PC carried out GIS ground truthing, image classification, secondary raster data generation, generated the risk model outputs, and also co-wrote sections of the manuscript. RK provided supervisory inputs for design, data collection, analyses and production of the manuscript, and also co-edited the manuscript. All authors contributed to the article and approved the submitted version.
This work was carried out through funding provided by Aircel Pvt. Ltd., a grant from the U.S. Fish and Wildlife Service (F17AP00991), under its Rhinoceros and Tiger Conservation Grant Programme, and a grant from the Chester Zoo, United Kingdom, to the larger landscape conservation projects developed for conflict management in the project landscape, run by the Wildlife Trust of India.
The authors are grateful to the Uttar Pradesh Forest Department for their support towards Wildlife Trust of India’s long-term project, through which this data was collected. The authors also acknowledge the contributions of several project personnel at different points of time in collection and verification of the data on human-tiger conflicts. Particularly, Mr. Ashisdan Francis Ishmael, Dr. Saurabh Singhai, Dr. Reetika Maheshwari, Dr. Devendra Chauhan and Mr. Sumit Saha. The data was collected as part of a long-term project financially supported by Aircel Pvt. Ltd., and the USFWS’s Rhinoceros and Tiger Conservation Grant, and the authors are thankful for this support.
The authors declare that the larger conservation action project under which this study was conducted received funding support from Aircel Pvt. Ltd. The funder had no involvement in the conceptualizing or designing of this study or the larger conservation action project, and also did not have any involvement in the data collection, analyses and writing of this manuscript, or the decision to submit this article for publication.
All claims expressed in this article are solely those of the authors and do not necessarily represent those of their affiliated organizations, or those of the publisher, the editors and the reviewers. Any product that may be evaluated in this article, or claim that may be made by its manufacturer, is not guaranteed or endorsed by the publisher.
The Supplementary Material for this article can be found online at: https://www.frontiersin.org/articles/10.3389/fcosc.2022.999195/full#supplementary-material
Supplementary Figure 1 | Correlation plot of the explanatory variables used in the generalized linear model.
Supplementary Figure 2 | Area under the receiver operating curve (ROC) represents the amount of false negative and false positive of the generalized linear model.
Amirkhiz R. G., Frey J. K., Cain J. W. III, Breck S. W., Bergman D. L. (2018). Predicting spatial factors associated with cattle depredations by the Mexican wolf (Canis lupus baileyi) with recommendations for depredation risk modeling. Biol. Conserv. 224, 327–335. doi: 10.1016/j.biocon.2018.06.013
Atwood T. C., Breck S. W. (2012). Carnivores, conflict, and conservation: defining the landscape of conflict. Available at: https://www.researchgate.net/publication/286152132_Carnivores_conflict_and_conservation_Defining_the_landscape_of_conflict
Barton K. (2009). “Mu-MIn: multi-model inference,” in R package version 0.12. 2/r18. Available at: http://R-Forge.R-project.org/projects/mumin.
Bhattarai B. R., Fischer K. (2014). Human–tiger panthera tigris conflict and its perception in bardia national park, Nepal. Oryx 48 (4), 522–528. doi: 10.1017/S0030605313000483
Burnham K. P., Anderson D. R. (1998). “Practical use of the information-theoretic approach,” in Model selection and inference (New York, NY: Springer), 75–117.
Carter N. H., Linnell J. D. (2016). Co-Adaptation is key to coexisting with large carnivores. Trends Ecol. Evol. 31 (8), 575–578. doi: 10.1016/j.tree.2016.05.006
Champion H. G., Seth S. K. (1968). A revised survey of the forest types of India (Delhi, India: Manager of publications).
Chandramouli C., General R. (2011). “Census of India,” in Rural urban distribution of population, provisional population total (New Delhi: Office of the Registrar General and Census Commissioner, India).
Chatterjee M., Basak K., Paul S., Pahari S., Kaul R. (2022). Characteristics of human-tiger conflicts in Indian sundarban. Harvard. Dataverse. doi: 10.7910/DVN/YS0WTE
Chatterjee M., Krishnendu B., Pandey P. C., Singhai S., Ashraf N. V., Kaul R. (2017). Living with the wild; mitigating conflict between humans and big cat species in uttar pradesh. Conserv. Action Ser. 20, 1–126.
Chauhan N. P. S. (2011). Man-eating and cattle-lifting by tigers and conservation implications in India. Julius-Kühn-Archiv 432), 178. doi: 10.5073/jka.2011.432.000
Dhanwatey H. S., Crawford J. C., Abade L. A., Dhanwatey P. H., Nielsen C. K., Sillero-Zubiri C. (2013). Large Carnivore attacks on humans in central India: A case study from the tadoba-andhari tiger reserve. Oryx 47 (2), 221–227. doi: 10.1017/S0030605311001803
Dhungana R., Savini T., Karki J. B., Dhakal M., Lamichhane B. R., Bumrungsri S. (2018). Living with tigers panthera tigris: Patterns, correlates, and contexts of human–tiger conflict in chitwan national park, Nepal. Oryx 52 (1), 55–65. doi: 10.1017/S0030605316001587
Gittleman J. L., Harvey P. H. (1982). Carnivore home-range size, metabolic needs and ecology. Behav. Ecol. Sociobiology. 10 (1), 57–63. doi: 10.1007/BF00296396
Gökhan A., Güzeller C. O., Eser M. T. (2019). The effect of the normalization method used in different sample sizes on the success of artificial neural network model. Int. J. Assess. Tools Educ. 6 (2), 170–192. doi: 10.21449/ijate.479404
Goodrich J. M. (2010). Human–tiger conflict: a review and call for comprehensive plans. Integr. Zoology. 5 (4), 300–312. doi: 10.1111/j.1749-4877.2010.00218.x
Graham K., Beckerman A. P., Thirgood S. (2005). Human–predator–prey conflicts: Ecological correlates, prey losses and patterns of management. Biol. Conserv. 122 (2), 159–171. doi: 10.1016/j.biocon.2004.06.006
Gulati S., Karanth K. K., Le N. A., Noack F. (2021). Human casualties are the dominant cost of human–wildlife conflict in India. Proc. Natl. Acad. Sci. 118 (8), e1921338118. doi: 10.1073/pnas.1921338118
Gurung B., Smith J. L. D., McDougal C., Karki J. B., Barlow A. (2008). Factors associated with human-killing tigers in chitwan national park, Nepal. Biol. Conserv. 141 (12), 3069–3078. doi: 10.1016/j.biocon.2008.09.013
Habib B., Nigam P., Mondal I., Ghaskadbi P., Hussain Z. (2017). Ensuring safety in the killer fields: Identifying potential villages for measures to reduce electrocution of tigers and associated species in Eastern vidarbha landscape, maharashtra, India. Wildlife. Institute. India. Dehradun. Natl. Tiger. Conserv. Authority. Maharashtra. For. Department. Rep., 115.
Heptner V. H., Sludskii A. A. (1992). Mammals of the soviet union. volume II part 2. carnivore (Hyaenas and cats). vysshaya shkola, Moscow. English. translation. 2(2):95–202
Inskip C., Ridout M., Fahad Z., Tully R., Barlow A., Barlow C. G., et al. (2013). Human–tiger conflict in context: risks to lives and livelihoods in the Bangladesh sundarbans. Hum. Ecol. 41 (2), 169–186. doi: 10.1007/s10745-012-9556-6
Inskip C., Zimmermann A. (2009). Human-felid conflict: A review of patterns and priorities worldwide. Oryx 43 (1), 18–34. doi: 10.1017/S003060530899030X
Jhala Y. V., Qureshi Q., Nayak A. K. (2020). Status of tigers, copredators and prey in India, 2018. National Tiger Conservation Authority, Government of India, New Delhi and Wildlife Institute of India. Dehradun.
Karanth K. U., Gopal R. (2005). An ecology-based policy framework for human-tiger coexistence in India. Conserv. Biol. Ser. - Cambridge. 9, 373. doi: 10.1017/CBO9780511614774.024
Nowell K., Jackson P. (Eds.) (1996). Wild cats: status survey and conservation action plan Vol. Vol. 382 (Gland, Switzerland: IUCN).
Kuhn M. (2008). Building predictive models in r using the caret package. J. Stat. software. 28, pp.1–pp26.
Lamichhane B. R., Persoon G. A., Leirs H., Musters C. J. M., Subedi N., Gairhe K. P., et al. (2017). Are conflict-causing tigers different? another perspective for understanding human-tiger conflict in chitwan national park, Nepal. Global Ecol. Conserv. 11, 177–187. doi: 10.1016/j.gecco.2017.06.003
Madhusudan M. D. (2003). Living amidst large wildlife: livestock and crop depredation by large mammals in the interior villages of bhadra tiger reserve, south India. Environ. Manage. 31 (4), 0466–0475. doi: 10.1007/s00267-002-2790-8
Miller J. R. (2015). Mapping attack hotspots to mitigate human–carnivore conflict: approaches and applications of spatial predation risk modeling. Biodiversity. Conserv. 24 (12), 2887–2911. doi: 10.1007/s10531-015-0993-6
Miller J. R., Jhala Y. V., Jena J. (2016). Livestock losses and hotspots of attack from tigers and leopards in kanha tiger reserve, central India. Regional. Environ. Change 16 (1), 17–29. doi: 10.1007/s10113-015-0871-5
Miquelle D., Nikolaev I., Goodrich J. (2005). Searching for the coexistence recipe: A case study of conflicts between people and tigers in the Russian Far East. People Wildlife. Conflict. Co-existence? 9, 305. doi: 10.1017/CBO9780511614774.020
Mondal K., Gupta S., Bhattacharjee S., Qureshi Q., Sankar K. (2012). Response of leopards to re-introduced tigers in sariska tiger reserve, Western India. Int. J. Biodiversity. Conserv. 4 (5), 228–236. doi: 10.5897/IJBC12.014
Moreto W. D. (2019). Provoked poachers? applying a situational precipitator framework to examine the nexus between human-wildlife conflict, retaliatory killings, and poaching. Criminal. Justice. Stud. 32 (2), 63–80. doi: 10.1080/1478601X.2019.1600816
Naughton-Treves L., Grossberg R., Treves A. (2003). Paying for tolerance: rural citizens' attitudes toward wolf depredation and compensation. Conserv. Biol. 17 (6), 1500–1511. doi: 10.1111/j.1523-1739.2003.00060.x
Nelder J. A., Wedderburn R. W. (1972). Generalized linear models. J. R. Stat. Society.: Ser. A. (General). 135 (3), 370–384. doi: 10.2307/2344614
Nyhus P. J., Tilson R. (2004). Characterizing human-tiger conflict in Sumatra, Indonesia: implications for conservation. Oryx, 38 (1), pp.68–74. doi: 10.1017/S0030605304000110
Packer C., Shivakumar S., Athreya V., Craft M. E., Dhanwatey H., Dhanwatey P., et al. (2019). Species-specific spatiotemporal patterns of leopard, lion and tiger attacks on humans. J. Appl. Ecol. 56 (3), 585–593. doi: 10.1111/1365-2664.13311
Pohlert T. (2020). “Trend: non-parametric trend tests and changepoint detection,” in R package ver. 1.1. 2.
Post G., Pandav B. (2013). Comparative evaluation of tiger reserves in India. Biodiversity. Conserv. 22 (12), 2785–2794. doi: 10.1007/s10531-013-0554-9
R Core Team (2018). R: A language and environment for statistical computing (Vienna, Austria: R Foundation for Statistical Computing). Available at: https://www.R-project.org/.
Robin X., Turck N., Hainard A., Tiberti N., Lisacek F., Sanchez J. C., et al. (2011). pROC: an open-source package for r and s+ to analyze and compare ROC curves. BMC Bioinf. 12 (1), 1–8. doi: 10.1186/1471-2105-12-77
Saberwal V. K. (1997). Saving the tiger: more money or less power?. Conservation Biology, pp.815–817.
Seidensticker J., McDougal C. (1993). Tiger predatory behaviour, ecology and conservation. Symposium. zoological. Soc. London. 65:105–125. doi: 10.18637/jss.v028.i05
Sillero-Zubiri C., Laurenson M. K. (2001). Interactions between carnivores and local communities: conflict or co-existence? Conserv. Biol. Ser. - Cambridge. 5:282–312.
Singh R., Nigam P., Qureshi Q., Sankar K., Krausman P. R., Goyal S. P., et al. (2015). Characterizing human–tiger conflict in and around ranthambhore tiger reserve, western India. Eur. J. Wildlife. Res. 61 (2), 255–261. doi: 10.1007/s10344-014-0895-z
Smith J. L. D. (1993). The role of dispersal in structuring the chitwan tiger population. Behaviour 124 (3-4), 165–195. doi: 10.1163/156853993X00560
State of Forest Report (2011) Forest survey of India, government of India. Available at: http://wipl.net.in/fsi_report_20011/final_2011.pdf.
Sunquist M. (2010). “What is a tiger? ecology and behavior,” in Tigers of the world (Elsevier Inc: William Andrew Publishing), 19–33. doi: 10.1016/B978-0-8155-1570-8.00002-5
Tilson R., Nyhus P. (1998). Keeping problem tigers from becoming a problem species. Conserv. Biol. 12 (2), 261–262. doi: 10.1046/j.1523-1739.1998.097l3.x
Tilson R., Nyhus P. J., Rubianto A. (2010). Poaching and poisoning of tigers in Sumatra for the domestic market. Tigers. World, 12 (2):101–112. doi: 10.1016/B978-0-8155-1570-8.00006-2
Treves A., Bruskotter J. (2014). Tolerance for predatory wildlife. Science 344 (6183), 476–477. doi: 10.1126/science.1252690
Treves A., Karanth K. U. (2003). Human-carnivore conflict and perspectives on carnivore management worldwide. Conserv. Biol. 17 (6), 1491–1499. doi: 10.1111/j.1523-1739.2003.00059.x
Treves A., Naughton-Treves L., Harper E. K., Mladenoff D. J., Rose R. A., Sickley T. A., et al. (2004). Predicting human-carnivore conflict: A spatial model derived from 25 years of data on wolf predation on livestock. Conserv. Biol. 18 (1), 114–125. doi: 10.1111/j.1523-1739.2004.00189.x
Valeix M., Hemson G., Loveridge A. J., Mills G., Macdonald D. W. (2012). Behavioural adjustments of a large carnivore to access secondary prey in a human-dominated landscape. J. Appl. Ecol. 49 (1), 73–81. doi: 10.1111/j.1365-2664.2011.02099.x
Warrier R., Noon B. R., Bailey L. (2020). Agricultural lands offer seasonal habitats to tigers in a human-dominated and fragmented landscape in India. Ecosphere 11 (7), e03080. doi: 10.1002/ecs2.3080
Wikramanayake E., Dinerstein E., Seidensticker J., Lumpkin S., Pandav B., Shrestha M., et al. (2011). A landscape-based conservation strategy to double the wild tiger population. Conserv. Lett. 4 (3), 219–227. doi: 10.1111/j.1755-263X.2010.00162.x
Wilson S. M., Madel M. J., Mattson D. J., Graham J. M., Burchfield J. A., Belsky J. M. (2005). Natural landscape features, human-related attractants, and conflict hotspots: A spatial analysis of human–grizzly bear conflicts. Ursus 16 (1), 117–129. doi: 10.2192/1537-6176(2005)016[0117:NLFHAA]2.0.CO;2
Woodroffe R., Ginsberg J. R. (1998). Edge effects and the extinction of populations inside protected areas. Science 280 (5372), 2126–2128. doi: 10.1126/science.280.5372.2126
Zimmermann A., McQuinn B., Macdonald D. W. (2020). Levels of conflict over wildlife: Understanding and addressing the right problem. Conserv. Sci. Pract. 2 (10), e259. doi: 10.1111/csp2.259
Keywords: negative interactions, coexistence, wildlife, human-tiger conflict, tiger (Panthera tigris), human-wildlife conflict (HWC)
Citation: Chatterjee M, Chatterjee N, Chandel P, Bhattacharya T and Kaul R (2022) Predicting negative human-tiger (Panthera tigris) interactions in mosaic landscapes around Dudhwa and Pilibhit tiger reserves in India. Front. Conserv. Sci. 3:999195. doi: 10.3389/fcosc.2022.999195
Received: 20 July 2022; Accepted: 22 September 2022;
Published: 11 October 2022.
Edited by:
Igor Khorozyan, Consultant in Mammal Research and Biodiversity Conservation, GermanyReviewed by:
Manjari Malviya, Wildlife Institute of India, IndiaCopyright © 2022 Chatterjee, Chatterjee, Chandel, Bhattacharya and Kaul. This is an open-access article distributed under the terms of the Creative Commons Attribution License (CC BY). The use, distribution or reproduction in other forums is permitted, provided the original author(s) and the copyright owner(s) are credited and that the original publication in this journal is cited, in accordance with accepted academic practice. No use, distribution or reproduction is permitted which does not comply with these terms.
*Correspondence: Mayukh Chatterjee, TS5DaGF0dGVyamVlQGNoZXN0ZXJ6b28ub3Jn
Disclaimer: All claims expressed in this article are solely those of the authors and do not necessarily represent those of their affiliated organizations, or those of the publisher, the editors and the reviewers. Any product that may be evaluated in this article or claim that may be made by its manufacturer is not guaranteed or endorsed by the publisher.
Research integrity at Frontiers
Learn more about the work of our research integrity team to safeguard the quality of each article we publish.