- 1Department of Health and Environmental Sciences, Xi’an Jiaotong-Liverpool University, Suzhou, China
- 2Chair of Wildlife Ecology and Management, Albert-Ludwigs University Freiburg, Freiburg, Germany
- 3Center for Nature and Society, School of Life Sciences, Peking University, Beijing, China
- 4NABU (Nature and Biodiversity Conservation Union), Berlin, Germany
- 5Research and Development Department, Disy Informationssysteme GmbH, Karlsruhe, Germany
- 6Forest Health and Biotic Interaction, Swiss Federal Research Institute WSL, Birmensdorf, Switzerland
- 7Swiss Ornithological Institute, Sempach, Switzerland
The post-2020 global biodiversity framework calls for a transformative change in food systems. Promoting agricultural multifunctionality is a viable approach to this sustainability transformation. The eastern Qinghai–Tibetan Plateau (QTP) is both one of the world’s largest livestock grazing systems and a hotspot of endemic birds in Asia. In this research, we aim to investigate the impact of livestock grazing on alpine bird assemblages at the local scale (alpha diversity) and their variation across the pastoral landscape (beta diversity). In the study area Nyanpo Yutse, we conducted surveys of 126 bird sample plots during two breeding seasons to acquire bird assemblage data. Meanwhile, we employed unmanned aerial vehicles to measure 2D and 3D habitat features within the 150-m radius. We investigated the key habitat variables driving the spatial distributions of both alpha and beta diversities of birds. Particularly, we partitioned beta diversity into its turnover and nestedness components and tested their patterns across sites of four levels of livestock grazing intensities (LGIs). Our results found no significant correlation between LGIs with species richness of birds, while 2D and 3D habitat complexity and built structure were positively correlated with alpha diversity (p < 0.05). At the landscape scale, pairwise LGI differences had no significant correlation (p > 0.05) with any pairwise beta diversity. The ordination plotting detected distinguished habitat preferences among 12 common birds and eight endemic birds. The multiple-site beta diversity of the 126 plots showed high species turnover (>0.871) where LGI was lower than 1.065 sheep units/ha, indicating the importance of moderate grazing for the conservation of diverse avian assemblages at the landscape scale. Our study demonstrated that extensive pastoralism is important for both maintaining the mosaic landscape and conserving avian biodiversity on the eastern QTP. We unveiled one of the ecological mechanisms through which synergies can be realized to support both agricultural production and biodiversity conservation in the Tibetan grazing system.
Introduction
Agriculture is the largest human land use type in the world (Dudley and Alexander, 2017). Agricultural intensification has become one of the major causes of the Anthropocene biodiversity loss (Díaz et al., 2019). Promoting sustainable agricultural landscapes is becoming important for nature conservation under the post-2020 biodiversity framework, which aims to mitigate direct threats to biodiversity through the transformative change approach and increase global protected terrestrial areas from 17% to 30% (Alves-Pinto et al., 2021; CBD, 2021a). However, the key question remains unsolved—in which conditions the food production and biodiversity sustaining functions of agricultural landscapes can be realized in synergy (Fischer et al., 2008; Grau et al., 2013; Fischer et al., 2014).
The impacts of agricultural land use on biodiversity take place across spatial scales, and land-use intensity is the common metric to link human-induced disturbances to biodiversity patterns observed from small to large scales (Bürgi et al., 2015). At the local scale, species richness (i.e., alpha diversity) is postulated to peak at the intermediate level of land-use intensity, i.e., ecological disturbance (Pickett and White, 1985; White and Pickett, 1985), despite the challenges to defining ‘intermediate’ in various ecosystems (Mackey and Currie, 2001; Fox, 2012; Fox, 2013). In environmental-friendly farming, when agricultural activities increase habitat complexity at the local scale, alpha diversity increases in response, since complex habitat can receive more species from the regional species pool (gamma diversity) than simple-structured habitats (Bazzaz, 1975; Tscharntke et al., 2012). At the landscape scale, agricultural land use impacts biodiversity indirectly through its influence on habitat heterogeneity (Tscharntke et al., 2012; Bürgi et al., 2015). A heterogeneous landscape with more habitat types can support more species of differentiated ecological niches (Duelli, 1997). Thus, a viable conservation approach is to maintain a landscape mosaic of diverse habitats, for example, maintaining semi-natural habitats in agricultural landscapes (Bartual et al., 2019), so that assemblages with different species compositions can coexist (Grau et al., 2013; Fischer et al., 2014).
Spatial variation in species composition is measured by beta diversity, which provides information on how biodiversity is organized in space (Whittaker, 1960; Whittaker, 1972). In multifunctional agricultural landscapes, including farmlands and rangelands, beta diversity can also be associated with the provisioning of different ecosystem functions (Mori et al., 2018). Incidence-based beta diversity can be partitioned into species turnover and species nestedness based on presence–absence data (Baselga, 2010). The turnover component measures among-site species replacement or the substitution of new species, while the nestedness component measures the degree to which species in smaller assemblages are subsets of the larger assemblages (Baselga, 2010; 2012). Turnover-related patterns can be formed by the community’s habitat specialization along environmental gradients, reflecting different species’ adaptation strategies or capabilities to the variation of key environmental factors (da Silva et al., 2018; Li et al., 2019). Nestedness-related patterns can be formed by changes in habitat quality along environmental gradients of habitat size, environmental stress, productivity, etc. (Berglund and Jonsson, 2003; Jacquemyn et al., 2007; Stuart et al., 2017). High turnover of species across sites implies that conservation should include multiple habitats, while high nestedness implies that conservation may aim at a few habitat types that have the highest number of species (Angeler, 2013).
A growing body of research has reported the impacts of agricultural land use on beta diversity, revealing a once-overlooked opportunity of using beta diversity to inform conservation planning in agricultural landscapes (Socolar et al., 2016; Buhk et al., 2017; Santana et al., 2017; Fontana et al., 2020). Alpine meadows of the Qinghai–Tibetan Plateau (QTP) are the world’s largest grazing system formed by pastoral land use of over 8,000 years (Miehe et al., 2019). The dominant species of the grassland is a dwarf sedge that is endemic to the QTP, namely, Kobresia pygmaea, the functional traits of which adapts well to the grazing of livestock (Miehe et al., 2009). The eastern QTP is one of the most important centers of endemic birds in Asia (Lei et al., 2007), which are often characterized by unique phylogenetic histories, diversified alongside the uplift of the young Plateau (Lei et al., 2014; Päckert et al., 2015). Przevalski’s Finch (Urocynchramus pylzowi), for example, is the oldest endemic Tibetan passerine, which has been found unexpectedly to be linked to the Ploceidae (weavers and widowbirds) after controversial systematic placements between buntings and finches (Päckert et al., 2016). One of the most abundant endemic birds, the White-rumped Snowfinch (Onychostruthus taczanowskii), nests in burrows of Plateau pika (Ochotona curzoniae), the increasing density of which is recognized as an indicator of grassland degradation (Lai and Smith, 2003). O. taczanowskii is one of the oldest species of snowfinches, the entire clade of which originated from the QTP (Päckert et al., 2020; Qu et al., 2021).
In the regional development planning of Qinghai Provincial Government (2021), the main functions of the eastern QTP region are water retention and biodiversity conservation. However, at the same time, the region also provides the significant function of food provisioning, suggesting a trade-off between agricultural production and nature conservation: over 80% of people in the eastern QTP were subsistence pastoralists (National Development and Reform Commission, 2013), and the stocking rate of the region was 22.16 million sheep units in 2017 (Qian et al., 2021). Pastoral land use is the sole major type of ecological disturbance in the alpine meadow system (Li et al., 2018).
Promoting multifunctionality of agricultural landscapes to achieve the 2030 global biodiversity targets (Li and Lü, 2019), it is crucial to understand the impacts of agricultural land use on local biodiversity and the organization of biodiversity in space. Our previous study has found that the mosaic landscape in the eastern QTP is created by traditional pastoral land use, and different levels of grazing intensity are one of the main drivers leading to the dominance of different vegetation types; e.g., low grazing intensities will result in a shrub-dominated habitat (Li et al., 2018). With the use of Nyanpo Yutse as an in-depth case study, our research aims to unveil the human–landscape–biodiversity interactions in the grazing system of the eastern QTP. To obtain high-quality species distribution and habitat data at a matching scale, we integrated breeding season bird surveys with novel remote sensing technologies—unmanned aerial vehicles (UAVs) were used to measure 2D and 3D habitat features of fine resolution at 10-cm level (Fritz et al., 2018). In this study, we aimed to address the following questions: 1) What are the key human and environmental factors that drive the variation of alpha and beta diversities in space? Particularly, 2) how do pastoral land use influence alpha diversity and both the turnover and nestedness components of beta diversity? 3) How does landscape heterogeneity influence the distribution of common and endemic species in the alpine bird assemblages?
Material and methods
Study area
We carried out the field study in the Nyanpo Yutse Mountain Region (33–34°N, 99–102°E) in Jiuzhi County of the eastern QTP. With the altitude ranging from 3,600 to 5,700 m above sea level, the grassland landscape is characterized by a vast alpine meadow inlaid with shrub patches of varied sizes. Heat and moisture are the two main limiting environmental factors of the natural system (Long, 2003): the average temperature of Nyanpo Yutse is 0.1°C, and the annual precipitation is 760 mm (Li and Li, 2002). Over 75% of the land in Jiuzhi is used by local pastoralists as rangeland (Jiuzhi County Annals Editor's Group, 2005). The average carrying capacity in Jiuzhi county is 1.37 sheep units/ha (People’s Government of Jikdril County, 2011). The high spatial variation in livestock grazing intensity and micro-topography of the eastern QTP results in a mosaic structure of the landscape (Li et al., 2018), which is composed of a variety of land-cover types, e.g., high-biomass Potentilla fruticosa shrubland, alpine meadows dominated by Kobresia species, and degraded grassland dominated by disturbance-tolerant plants, e.g., Potentilla anserina, or annual forbs such as Chenopodium album. In the study area, such degradation of alpine meadows is often related to the spreading of plateau pika O. curzoniae as the result of land-use intensification due to population growth and pasture privatization (Li et al., 2021).
Bird sampling
The bird community data were assembled from bird surveys in two consecutive breeding seasons in the Nyanpo Yutse region. Adopting a stratified sampling method (Gregory et al., 2004), from May to August 2014 and 2015, we placed 140 plots in eight blocks located in four major valleys of the study area within the altitude between 3,700 to 4,200 m above sea level (Supplementary Figure 1). Plots were placed at a minimum distance of 200 m away from each other. Specifically, the 140 plots include 35 plots in shrubland habitat, 35 in shrub–grass mixed or edge zones, 48 in grasslands, 17 in degraded grasslands, and 5 having permanent human settlements within a radius of 50 m. The eight blocks were designed to avoid any spatial clustering of any vegetation type. To minimize observer bias, the same person (first author) conducted the six replicates for the two surveys. During the first 25 working days in 2014, an experienced bird guide also took part in the surveys to validate all species identifications. In each survey, the 140 plots were visited in the early, middle, and late phases of the breeding season, which summed up to six replicates for each plot in the 2 years. The six replicates of the same plot were scheduled randomly from 6:00 a.m. until noon, skipping days with extreme weather conditions including heavy rain, hailing, and snowing. With the use of the standard point count approach (Bibby et al., 1992), all visual or acoustic individual bird occurrences during the 10-min observation interval were recorded with estimated distances from the standing spot at the center of the plot. All occasional birds flying higher than 10 m above the ground were excluded from the dataset to ensure the record can be indicative of local habitat use.
Measuring habitat parameters using unmanned aerial vehicles
UAVs have shown excellent performance in acquiring both 2D and 3D avian habitat data at fine resolution (Fritz et al., 2018). From June to July 2014, we conducted 39 operations using three fixed-wing UAVs (eBees produced by senseFly) equipped with Canon IXUS 127HS cameras. We captured 5,559 individual images of all 140 sample plots of a 200-m radius, covering a total area of 45.8 km2. The average ground sampling distance reached 13 cm (range of 11–16 cm). We processed the images with Photoscan Professional 1.1.6 (Agisoft), which resulted in orthophotos, digital surface models, and point clouds for each sample block.
Acquiring the UAV-derived orthophotos (Figure 1A), we measured key habitat features in each plot, including land-cover types, their composition, and habitat heterogeneity. First, for each of the 140 plots with a 200-m radius, we manually delineated 15 different vegetation types on high-resolution ortho-images with ArcMap 10.3 (ESRI). The 15 vegetation types were grouped into six major land-cover types, namely, shrubland, grass–shrub mixed or edge zone, grassland, degradation (grassland showing bare-ground patches), waterbody and wetland, and built structures, e.g., stockyard, road, and settlement (Figure 1B). The land-cover classification provided us with the detailed size and proportion of each land-cover type of every sample plot. Second, knowing the numbers and extents of all the 15 vegetation types in each plot, we calculated the habitat Shannon–Wiener index as the land cover horizontal diversity indicator or a proxy measure for 2D habitat complexity. Third, we used the fine-scale 3D model (Figure 1C) to measure surface ruggedness by creating a linear model to fit a plane to the sample plot 3D point cloud (Figure 1D). Among the normal vector of the plane used for slope and orientation, we utilized the square root of the estimated variance of the random error (σ) of the fitted model as a predictor for the vertical habitat complexity of a plot (Fritz et al., 2018). We named this predictor ‘3D complexity’. In our sample plots, any geographic structure or 3D object above the flat terrain can increase the value of 3D complexity, e.g., cliffs, houses, or Buddhist prayer flags. All UAV-derived habitat variables for the sampled plots can be found in Supplementary Table 1 and were described in detail in Fritz et al. (2018).
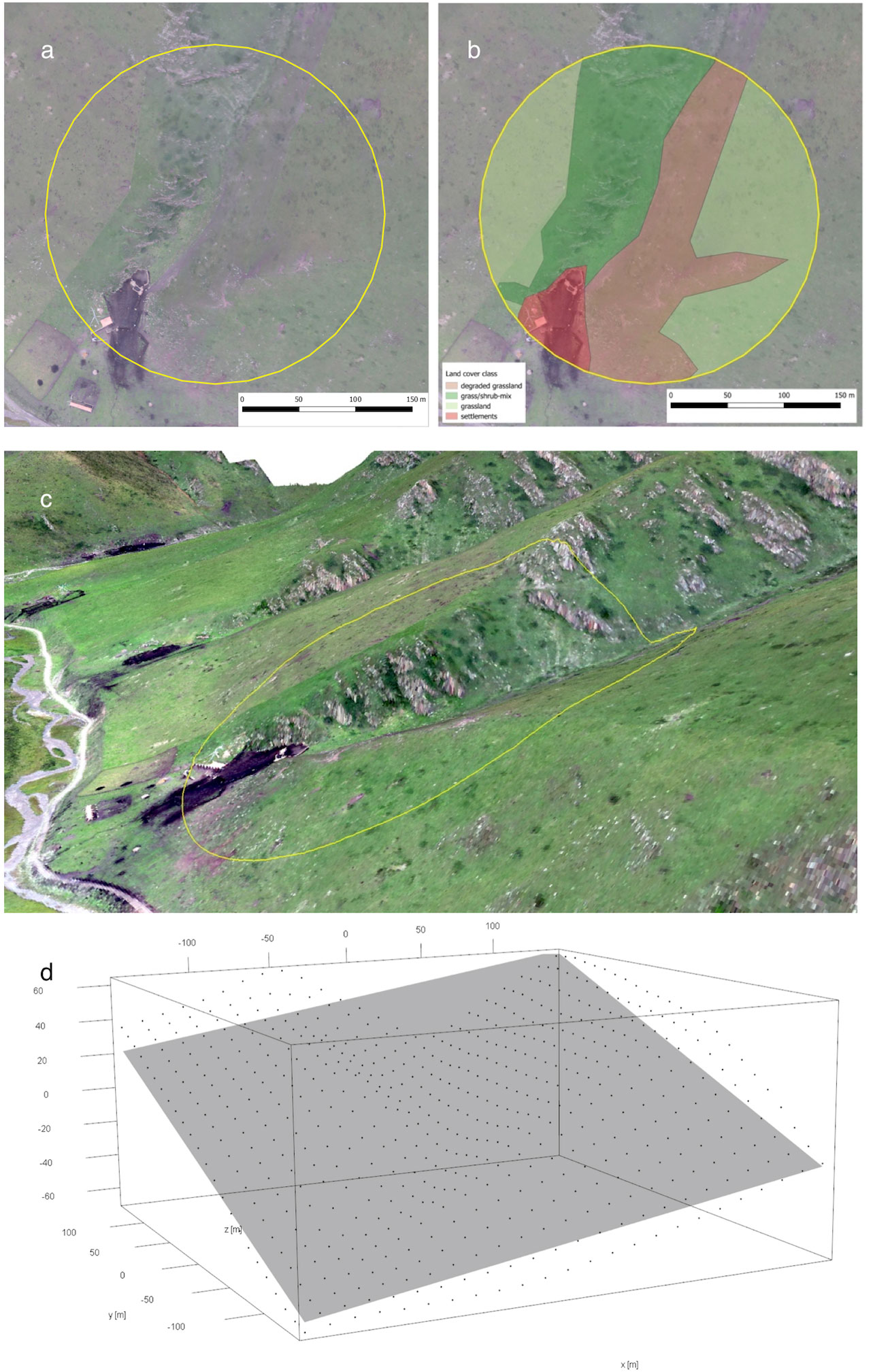
Figure 1 Ortho image (A) of sample plot 510 and the manually classified land cover classes (B). (C) A 3D visualization of the plot facing northeast. This plot has been compared to plots with simpler topography and a high 3D complexity value. The deviation from a best-fit plane is high (D).
To measure livestock grazing intensity for each plot, in 2014 and 2015, we made 66 interviews inthe study area using the approach of participatory mapping (Fagerholm et al., 2012; Brown and Kytta, 2014). Within the interviews, we recorded the time periods of pastoral use, livestock (mostly yak, very few horses, and no sheep) numbers, and the size of the pastures (Li et al., 2017). Combining the information, we calculated the annualized livestock grazing intensity (LGI) for each pasture where the 140 sample plots were located (Bürgi et al., 2015).
N is the standardized number of livestock measured by sheep unit, T is the number of days grazed per year, and S is the pasture size in ha. There are mainly two types of seasonally winter pastures in Nyanpo Yutse. Cold-season pastures are used by single households from October to June, and warm-season pastures are collectively used for household groups for the rest of the 4 months of the year. Therefore, the average size of the cold-season pastures is smaller than that of the warm season. Generally, compared to cold-season pastures, warm-season pastures have lower LGIs.
Determining the scale of effect
UAVs created a continuous dataset up to the 200-m radius. By comparing the explanatory power of habitat parameters at 50-, 100-, 150-, and 200-m radii, we found that the highest variation in bird species composition was explained at the 150-m radius (Fritz et al., 2018). Therefore, we removed 14 plots from further analyses, which have spatial overlap with their neighboring plots at the 150-m radius. This resulted in a reduced dataset containing 126 plots that were used for data analyses. In habitat feature measurements, we used this 150-m radius as the scale for all explanatory variable calculations, i.e., the scale of effect. For the calculation of land-cover extent, we used a 150-m crop from the original 200-m land-cover classification map. For the bird data, we included all entries documented within the 150-m radius.
Calculation of alpha and beta diversity indices
To calculate both alpha and beta diversities for the 126 avian assemblages at the 150-m radius scale, we excluded all raptors and waders, whose home ranges could not be adequately captured at the study scale, resulting in a reduced bird dataset with 7,357 records of 63 bird species. For alpha diversity, we used additive species richness recorded throughout the six replicates to calculate each plot (Li et al., 2018). We used two approaches to decompose beta diversity based on the incidence-based species composition of the 126 avian assemblages (Supplementary Table 2). Both pairwise and multiple-site beta diversities were calculated using the Sørensen dissimilarity index (βSOR). Then each was partitioned into its turnover component measured by Simpson-based dissimilarity (βSIM) and nestedness component measured by nestedness-resultant dissimilarity (βNES) (Baselga, 2010). We used pairwise beta diversity indices and pairwise habitat variables to develop matrix regression models for the 126 sample plots. Multiple-site dissimilarity measures outperform pairwise dissimilarity measures to compare overall species dissimilarity among regions (Baselga, 2013; 2017). Hence, we used multiple-site beta diversity indices to test the effect of livestock grazing intensity on beta diversity distributions across the pastoral landscape.
Data analysis
Species richness of the 126 plots had a normal distribution (Shapiro–Wilk normality test, p > 0.05). We developed linear models to test the relationship between species richness, i.e., alpha diversity, and select habitat variables. The global model was as follows: lm(SpeciesRichness ~ shrub + edge + grass + degradation + water + BuiltStructure + LGI + 2DHabitatComplexity + 3DHabitatComplexity).
To obtain the best-fit model, we performed the automated model selection, removing variables according to the corrected Akaike information criterion (AICc) ranking criterion (Cavanaugh, 1997). Furthermore, we tested the variance inflation factors (VIFs) of the selected parsimonious model to control for collinearity among variables. To investigate beta diversity variations in the pastoral landscape, we used multiple regression on distance matrices (MRM) to model the relationships between the pairwise distance of the nine habitat variables with pairwise beta diversity (Santana et al., 2017), including both the turnover and nestedness components. Furthermore, we also added the geographic distance matrix of each pair of the 126 sample plots into the MRM model. The distance is calculated using the GPS coordinates at the center of each plot. The significance of MRM model coefficients was estimated based on 10,000 permutations (Legendre et al., 1994).
To understand the impact of livestock grazing intensity on alpha and beta diversities of birds, we first separated the 126 sample plots into four groups, using the thresholds of the first, second, and third quartiles of the plots’ measured LGIs. Second, we performed the test of analysis of variance (ANOVA) to investigate the difference in species richness among plots from the four LGI levels. Third, we calculated multiple-site Sørensen dissimilarity, multiple-site dissimilarity of turnover (βSIM), and nestedness (βNES) for plots belonging to each LGI level and compared the results among the four LGI levels.
To explain the variations of beta diversity related to species composition in bird assemblages, we performed a partial constrained redundancy analysis (pRDA) (Ter Braak, 1986). The plotting of pRDA results visualizes how different habitat variables drive the distribution of bird occurrence at the landscape scale (Legendre and Legendre, 2012). To inform conservation management, we performed the pRDA separately using common birds (more than 100 observations in all the six replicates, Table 1) and bird species endemic to China (Lei et al., 2007) or the Tibetan Plateau (BirdLife International, NatureServe, 2011).
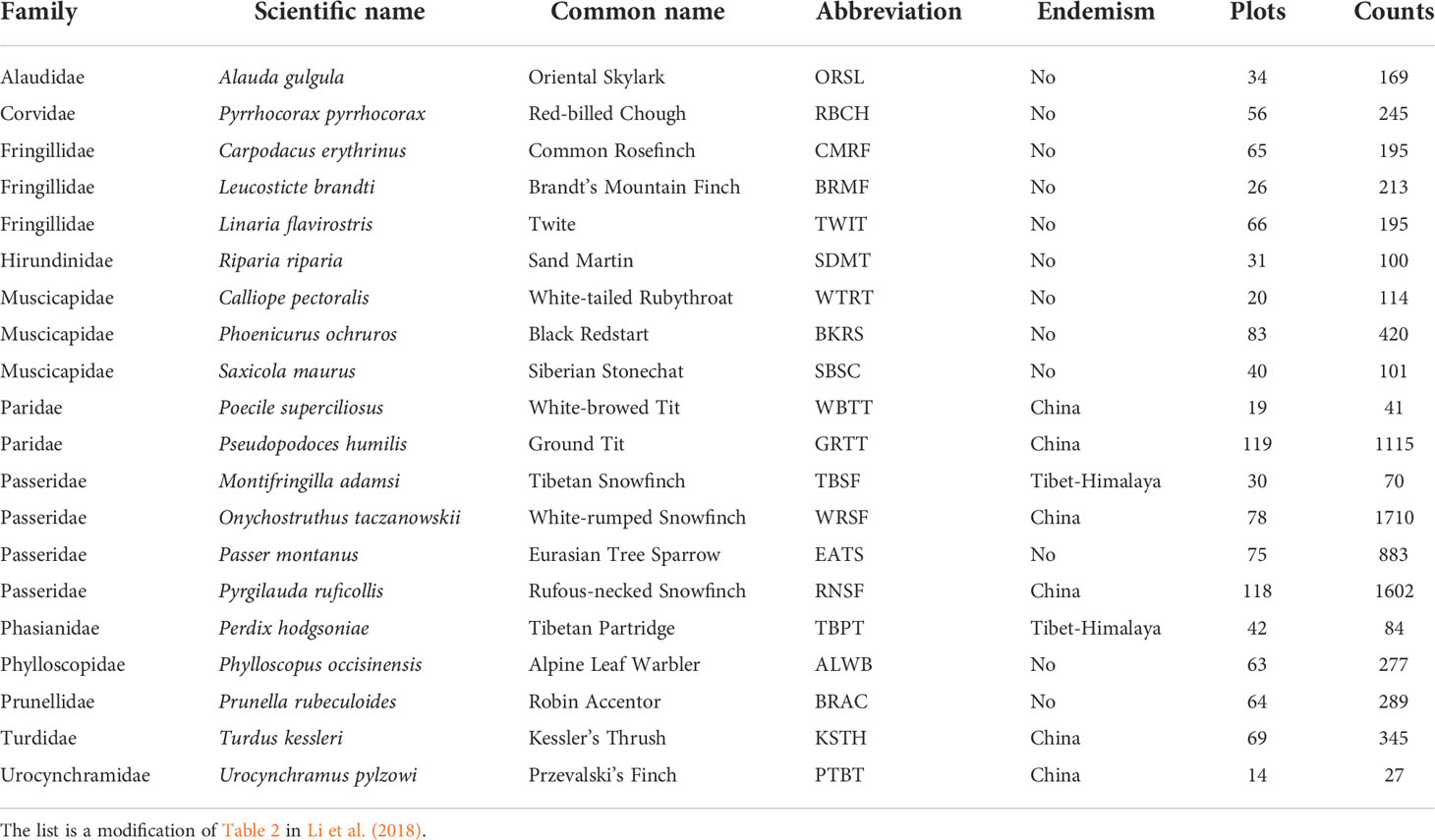
Table 1 List of the 20 common and endemic bird species used for data analyses, with their distribution status shown in column Endemism, the total number of plots where the species was recorded shown in column Plots, and the total recorded abundance throughout the six replicates shown in column Counts.
Data analyses were performed in R version 4.0.5 (R Core Team, 2021) using packages ‘vegan’ (Oksanen et al., 2017), ‘betapart’ (Baselga et al., 2018), ‘MuMin’ (Barton and Barton, 2018), and ‘ecodist’ (Goslee and Urban, 2007). Results were visualized with ‘ggplot2’ (Wickham, 2016).
Results
Bird species
We recorded a total of 77 species from 28 families, among which 63 were used in the data analysis (Supplementary Table 3). Table 1 shows the names of 20 species including twelve common species and eight species that are endemic to the QTP or China (Table 1).
Factors driving alpha and beta diversity spatial distributions
The linear regression model of species richness with habitat variables scoring the lowest AICc had an R2 of 0.429 (p < 0.001, VIFs < 1.1 for all select model variables). Alpha diversity of bird assemblages was positively correlated with the 3D habitat complexity, 2D habitat complexity, and the area of built structures, while the area of degraded grassland and grassland both reduced species richness (Table 2).
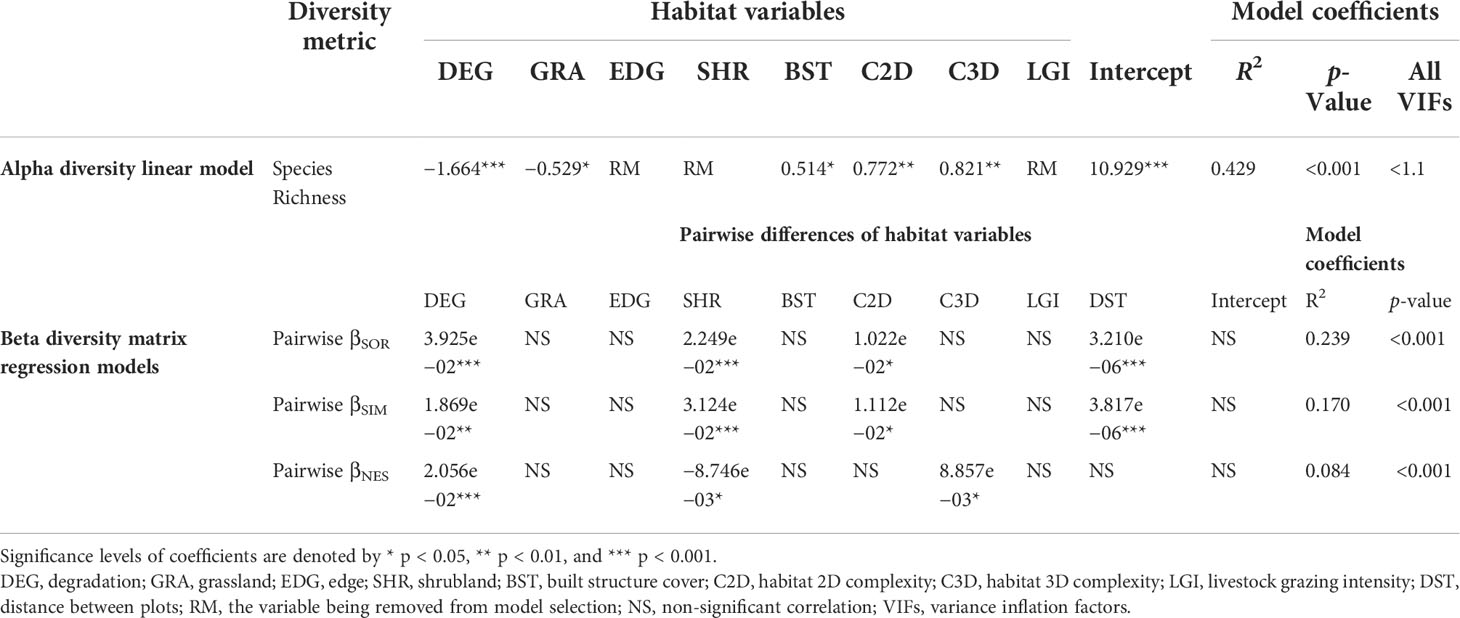
Table 2 Standardized estimates of linear models and matrix regression models between habitat variables with alpha and beta diversity metrics based on 126 bird assemblages in Nyanpo Yutse.
Multiple-site Sørensen dissimilarity index (βSOR) of all the 126 plots is 0.973, which was composed of a high turnover component βSIM 0.962 and a low nestedness component βSNE 0.011. The matrix regression modeling of pairwise beta diversity showed that the turnover and nestedness were driven by distinct landscape heterogeneity components (Table 2). The difference in shrubland cover in the landscape significantly increased the turnover of species (p < 0.001) and decreased the nestedness of species composition (p < 0.05). However, the difference in degraded grassland area significantly increased both the pairwise turnover (p < 0.01) and nestedness components (p < 0.001). The variations of 2D habitat complexity significantly increased the pairwise turnover of bird assemblages (p < 0.05), while the variations of 3D habitat complexity significantly increased pairwise nestedness across the pastoral landscape (p < 0.05).
Impacts of livestock grazing intensity on alpha and beta diversities of alpine birds
We separated the 126 plots into four levels according to their annualized LGIs. The histogram of LGIs is provided in Supplementary Figure 2. LGIs from the first-quantile level (33 plots) ranged at 0–0.190 sheep units/ha, second level (30 plots) 0.190–1.065 sheep units/ha, third level (32 plots) 1.065–2.862 sheep units/ha, and fourth level (31 plots) 2.862–6.790 sheep units/ha. The ANOVA found no significant differences in alpha diversity among plots belonging to the four-quantile LGI levels (p > 0.2 in all pairwise comparisons, Figure 2A). As shown in Figure 2B, increasing LGI levels increased multiple-site dissimilarity of nestedness (βSNE) and decreased turnover (βSIM). Where LGIs were lower than 1.065 sheep units/ha, the multiple sites βSNE dropped substantially, and βSIM increased to larger than 0.871 (i.e., ).
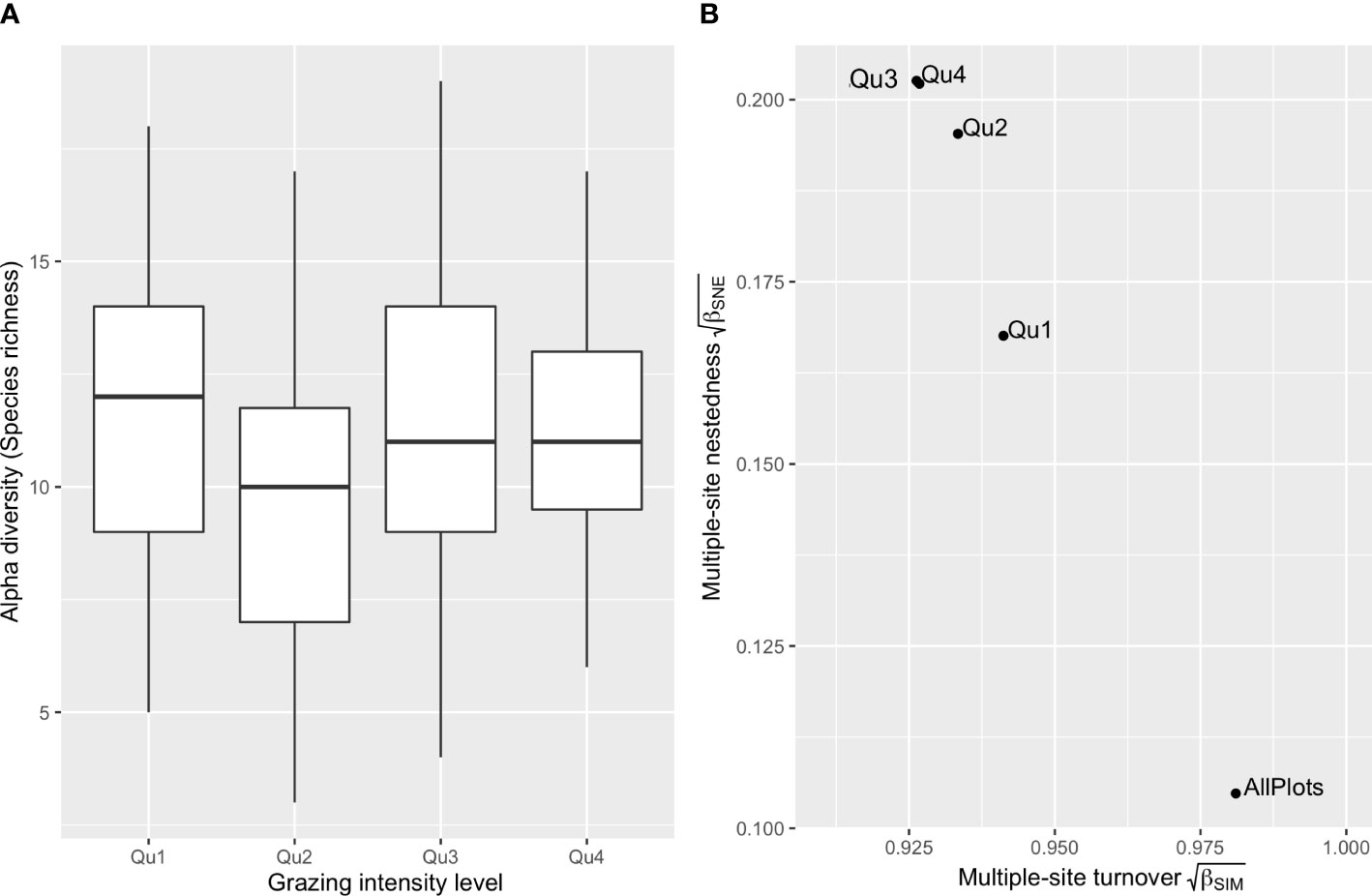
Figure 2 Comparing alpha and beta diversities of four livestock grazing intensity levels. Boxplots of plot-scale species richness of the 126 bird assemblages grouped by the four livestock grazing intensity (LGI) quantiles (A), and incidence-based multiple-site beta diversities of plot groups belonging to the four LGI levels (B). Beta diversity is partitioned into multiple-site species turnover (βSIM) and multiple-site nestedness (βSNE). Qu1 indicates the first quantile of the 126 sample plots of low LGIs, and Qu4 is the fourth quantile of high LGIs.
Species variation in relation to landscape heterogeneity
The first pRDA axis (11.3% of variance) of common birds in Nyanpo Yutse (Figure 3A) reflected the replacement of shrubland specialists (e.g., Alpine Leaf Warbler (ALWB)) with species well adapted to the man-made environment (e.g., Eurasian Tree Sparrow (EATS)) and was associated with the coverage of shrubland (coefficient −0.559) and built structures (0.458). The second pRDA axis had a smaller proportion of variance explained (8.2%). Nevertheless, it clearly distinguished birds preferring a high vertical habitat complexity (e.g., Common Rosefinch (CMRF)).
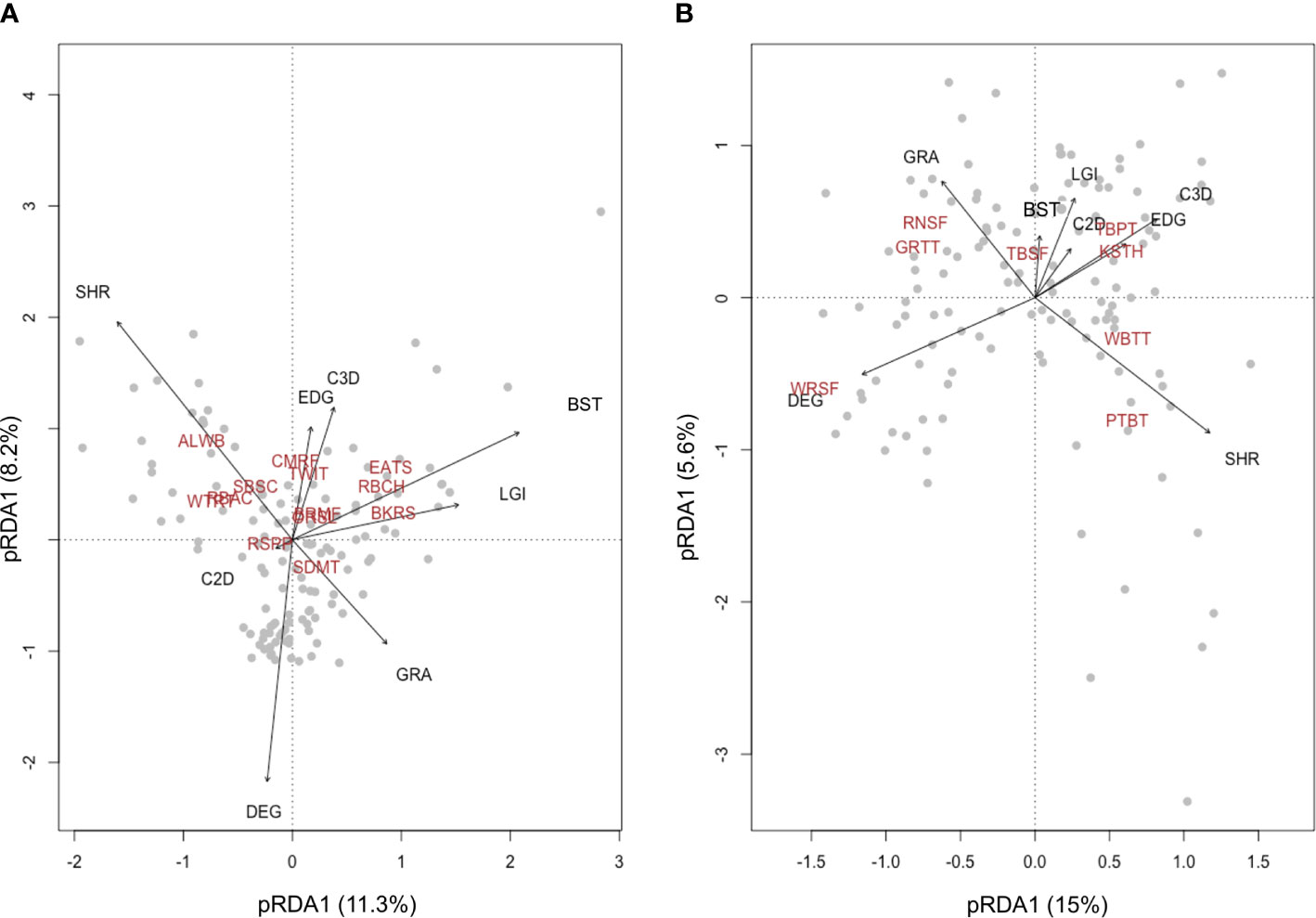
Figure 3 Biplots of the first two redundancy analysis (RDA) axes for bird assemblages containing 12 common species (A) and eight endemic species (B). The proportion of total variation explained by the first two axes is provided. The biplots show species replacement in the pastoral landscape is driven by the spatial change of different habitat variables. Abbreviations for bird species and habitat variables are provided in Tables 1 and 2.
For endemic birds, the first pRDA axis (15% of variance) reflected the replacement of grassland degradation-adapted birds (e.g., White-rumped Snowfinch (WRSF)) with shrubland specialists (e.g., Przevalski’s Finch (PTBT)). The pRDA plotting also well demonstrated the association between the coverage of grassland areas and grassland specialists, including Rufous-necked Snowfinch (RNSF) and Ground Tit (GRTT), as well as the association between the coverage of shrub–grass edge and species preferring this ecotone, including Kessler’s Thrush (KSTH) and Tibetan Partridge (TBPT).
Discussion
Direct effects of livestock grazing on alpha and beta diversities
As the main form of ecological disturbance in grassland systems, livestock grazing can directly impact bird populations through nest trampling, food supply alteration, or change in predation pressure (Barzan et al., 2021). The intermediate disturbance hypothesis (IDH) suggests that biodiversity is maximized in sites bearing intermediate levels of disturbance, where disturbance-tolerant species and competitively dominant species coexist (Grime, 1973; Connell, 1978). However, our results found no significant correlation between LGI and species richness (alpha diversity, Table 2). The ANOVA detected no significant difference (p > 0.2) in species richness among sites belonging to the four LGI levels (Figure 2A).
For beta diversity, the finding that pairwise LGI differences did not show a significant correlation (p > 0.05) with any pairwise beta diversity, including both the turnover and nestedness components, indicated that different grazing management was not the main driver of dissimilarities in species composition across the Nyanpo Yutse landscape. However, multiple-site beta diversity of the four LGI levels (Figure 2B) reveals that annualized LGI above the level of 1.065 sheep units/ha might result in a relatively high nestedness among sites, implying a stronger environmental filtering effect resulting in species loss at these high LGI levels, particularly on winter rangelands (Socolar et al., 2016). Below the LGI level of 1.065 sheep units/ha, among-site replacement of species drives the beta diversity changes, and species compositions at the 150-m radius scale are very different from one site to another. In other words, conservation policies should consider that beta diversity is favored by extensive pastoral land use at the landscape scale. In the Nyanpo Yutse region where the average carrying capacity was approximately 1.37 sheep units/ha, annualized LGI of 1.065 sheep units/ha could be identified as the benchmark level of extensive grazing. It is worth mentioning that for cold-season pastures with smaller sizes and longer using periods, maintaining extensive grazing implies a change of present pasture use, including reducing stocking rate, shifting private pasture usage to collective usage, or setting up a rotational grazing plan.
Effects of habitat complexity and landscape heterogeneity on avian diversities
In the eastern QTP, pastoralism maintained the landscape mosaic composed of different vegetation types (Li et al., 2018). Like other alpine systems, the abandonment of pastures may result in shrub encroachment and lead to a homogenized landscape structure (Kaphengst and Ward, 2008; Sirami and Monadjem, 2012; Koch et al., 2015). In Nyanpo Yutse, human activities created and maintained the heterogeneous habitat structures at both the local (150-m radius in this study) and the landscape scales. At the local scale, we found both 2D and 3D habitat complexity, and the area of built structures was positively correlated with species richness. Our previous findings detected positive correlations between grazing intensity with 2D and 3D habitat complexity (Li et al., 2018). It implied that pastoralism and the presence of human land use in the region had indirectly facilitated the community assembly process to form high-richness bird assemblages compared to abandoned pastures.
Interestingly, the two habitat heterogeneity variables contributed to the pairwise dissimilarity of species compositions, but the underlying mechanisms were different. At the landscape scale, pairwise differences in 2D habitat complexity increased pairwise turnover among the 126 bird assemblages. This pattern indicated that species compositions varied across sites due to a high environmental heterogeneity attracting birds with a diversity of habitat preferences from the regional species pool. For the 12 most commonly distributed birds in Nyanpo Yutse, degraded grassland, grassland, and shrubland can best separate the difference in their habitat preferences (Figure 3A). In comparison, 3D habitat complexity was positively related to pairwise nestedness (Table 2), indicating that vertical differences were favored by most birds except a few flat terrain specialists. In this regard, vertical complexity can be considered as one key feature to measure the ‘quality of bird habitats’ in the grassland landscape where the topographic fluctuations are generally mild. As such, a habitat structure of high vertical complexity qualifies as a ‘keystone structure’ for bird conservation in the eastern QTP (Tews et al., 2004; Manning et al., 2006). Keystone structures have been related to bird abundance and diversity in several habitat types, such as forests (Basile et al., 2021) or wetlands (Calhoun et al., 2017). Agricultural landscapes are known to host artificial keystone structures that positively contribute to biodiversity (Poschlod and Braun-Reichert, 2017), but their relevance in pastoral landscapes has been so far overlooked. In this grassland study particularly, we found that built structures contribute to the increase of habitat vertical complexity from which keystone structures emerge, indicating positive impacts of human activities on avian diversity. It is advisable that future research could examine the impacts of such keystone structures on community compositions and other facets of biodiversity, e.g., species evenness.
In Nyanpo Yutse, the multiple-site turnover component accounts for 98.9% of the overall dissimilarity of species compositions across the 126 sample sites. The high among-site species replacement suggested that landscape heterogeneity in the region is generally very high. Particularly, for the eight endemic birds of higher conservation value, their habitat-use preferences varied substantially and could be grouped into degradation birds, grassland birds, shrub–grass ecotone birds, and shrubland birds (Figure 3B). The dominance of endemic White-rumped Snowfinch in degraded grasslands may be also due to the fact that it is a strong competitor for other open grassland birds to occupy the ecological niche (Zeng and Lu, 2009). This implies that biodiversity conservation on the eastern QTP should not merely focus on protecting any single vegetation type, e.g., shrubland. However, conservation efforts should be made to maintain the landscape mosaic, and extensive pastoralism can be considered an effective and economic means to achieve this goal.
Conservation implications
Over the past decades, biodiversity conservation, shrubland preservation, and pasture restoration have become the major conservation policies of the eastern QTP, in which monetary incentives had been created to minimize pastoral land-use intensity (Meng, 2012; National Development and Reform Commission, 2013). Notably, these large-scale ecological projects aimed to facilitate the natural succession toward shrubland in order to enhance the alpine meadows’ water retention capacity. Our study demonstrates that extensive livestock grazing is important for biodiversity conservation in the eastern QTP: 1) livestock grazing does not show significant adverse impacts on the alpha diversity of birds; 2) extensive pastoralism (defined as annualized LGI < 1.065 sheep units/ha in Nyanpo Yutse) maintains the mosaic landscape where the turnover component contributes to the main share of beta diversity, implying the importance of protecting habitat diversity across the grazing landscape; 3) endemic grassland birds have varied habitat requirements, including degraded grasslands. Protecting those high-conservation-value species will also require the landscape approach to cover as many vegetation types as possible (Brown et al., 2005).
The post-2020 global biodiversity framework of the CBD will aim high to mitigate the drastic loss of species and ecosystems, e.g., increasing protected terrestrial land area from 17% to 30%. The transitional change suggests that it is of equal importance to mitigate the negative impacts of agricultural production on biodiversity, as well as to maximize the conservation function of agricultural landscapes (CBD, 2021b). Indeed, there is a great potential to incorporate species-rich agricultural lands into biodiversity conservation strategies (Dudley and Alexander, 2017). Our Nyanpo Yutse case study unveils the ecological mechanisms through which the multifunctionality of the Tibetan grazing system can be realized. It also shed light on the management of other traditional agricultural landscapes: taking landscape heterogeneity and species turnover in space into account, it will be possible to create synergies between agricultural production and nature conservation through the sensible regulation of land-use intensities.
Nomenclature
The nomenclature of birds follows Gill et al. (2021).
Data availability statement
The original contributions presented in the study are included in the article/Supplementary Material. Further inquiries can be directed to the corresponding author.
Author contributions
We declare the following contributions of the authors: LL, IS, and ZL conceptualized and framed the main perspective of the paper. LL and DT designed the field sampling and data analysis scheme. LL conducted bird surveys, and AF collected the UAV data in the fieldwork. AF developed and computed the UAV-derived habitat predictors. LL and MB performed the data analysis. LL drafted the manuscript, and all authors contributed to the revisions of the manuscript. All authors contributed to the article and approved the submitted version.
Funding
The Chinese Scholarship Council, the Rufford Foundation (14933-1), the Graduate Funding Landesgraduiertenförderung Baden-Württemberg, and the Postdoctoral Research Foundation of China (2019M650322) provided financial support to the study.
Acknowledgments
We thank Ke Du, Xu Shi, and our friends from the Nyanpo Yutse Environmental Association for their assistance in the fieldwork. Yi Zou, Zhiyao Tang, and Klaus Birkhofer provided valuable comments to improve the manuscript. The corresponding author is grateful to Udo Bröring, who sparked the idea of the disturbance intensity study of the Tibetan grazing system.
Conflict of interest
Author DTT was employed by company Disy Informationssysteme GmbH.
The remaining authors declare that the research was conducted in the absence of any commercial or financial relationships that could be construed as a potential conflict of interest.
Publisher’s note
All claims expressed in this article are solely those of the authors and do not necessarily represent those of their affiliated organizations, or those of the publisher, the editors and the reviewers. Any product that may be evaluated in this article, or claim that may be made by its manufacturer, is not guaranteed or endorsed by the publisher.
Supplementary material
The Supplementary Material for this article can be found online at: https://www.frontiersin.org/articles/10.3389/fcosc.2022.902887/full#supplementary-material
References
Alves-Pinto H., Geldmann J., Jonas H., Maioli V., Balmford A., Latawiec A. E., et al. (2021). Opportunities and challenges of other effective area-based conservation measures (OECMs) for biodiversity conservation. Perspect. Ecol. Conserv. 19 (2), 115–120. doi: 10.1016/j.pecon.2021.01.004
Angeler D. G. (2013). Revealing a conservation challenge through partitioned long-term beta diversity: increasing turnover and decreasing nestedness of boreal lake metacommunities. Diversity Distribut. 19 (7), 772–781. doi: 10.1111/ddi.12029
Barton K., Barton M. K. (2018). Package ‘MuMIn’ Model selection and model averaging based on information criteria. R package version 1.15.11.
Bartual A. M., Sutter L., Bocci G., Moonen A. C., Cresswell J., Entling M., et al. (2019). The potential of different semi-natural habitats to sustain pollinators and natural enemies in European agricultural landscapes. Agriculture, Ecosystems & Environment 279, 43–52. doi: 10.1016/j.agee.2019.04.009
Barzan F. R., Bellis L. M., Dardanelli S. (2021). Livestock grazing constrains bird abundance and species richness: A global meta-analysis. Basic. Appl. Ecol. 56, 289–298. doi: 10.1016/j.baae.2021.08.007
Baselga A. (2010). Partitioning the turnover and nestedness components of beta diversity. Global Ecol. Biogeogr. 19 (1), 134–143. doi: 10.1111/j.1466-8238.2009.00490.x
Baselga A. (2012). The relationship between species replacement, dissimilarity derived from nestedness, and nestedness. Global Ecol. Biogeogr. 21 (12), 1223–1232. doi: 10.1111/j.1466-8238.2011.00756.x
Baselga A. (2013). Multiple site dissimilarity quantifies compositional heterogeneity among several sites, while average pairwise dissimilarity may be misleading. Ecography 36 (2), 124–128. doi: 10.1111/j.1600-0587.2012.00124.x
Baselga A. (2017). Partitioning abundance-based multiple-site dissimilarity into components: Balanced variation in abundance and abundance gradients. Methods Ecol. Evol. 8 (7), 799–808. doi: 10.1111/2041-210X.12693
Baselga A., Orme D., Villeger S., De Bortoli J., Leprieur F., Baselga M. A. (2018). Package ‘betapart’ Partitioning beta diversity into turnover and nestedness components, version, 1(0).
Basile M., Storch I., Mikusiński G. (2021). Abundance, species richness and diversity of forest bird assemblages – the relative importance of habitat structures and landscape context. Ecol. Indic. 133, 108402. doi: 10.1016/j.ecolind.2021.108402
Bazzaz F. A. (1975). Plant species diversity in old-field successional ecosystems in southern Illinois. Ecology 56 (2), 485–488. doi: 10.2307/1934981
Berglund H., Jonsson B. G. (2003). Nested plant and fungal communities; the importance of area and habitat quality in maximizing species capture in boreal old-growth forests. Biol. Conserv. 112 (3), 319–328. doi: 10.1016/S0006-3207(02)00329-4
Brown G., Kytta M. (2014). Key issues and research priorities for public participation GIS (PPGIS): A synthesis based on empirical research. Appl. Geogr. 46, 122–136. doi: 10.1016/j.apgeog.2013.11.004
Brown J., Mitchell N., Beresford M., International Union for Conservation of Nature and Natural Resources, World Commission on Protected Areas (2005). “The protected landscape approach : linking nature, culture and community,” in Gland (Cambridge: IUCN - The World Conservation Union).
Buhk C., Alt M., Steinbauer M. J., Beierkuhnlein C., Warren S. D., Jentsch A. (2017). Homogenizing and diversifying effects of intensive agricultural land-use on plant species beta diversity in central Europe–a call to adapt our conservation measures. Sci. Total. Environ. 576, 225–233. doi: 10.1016/j.scitotenv.2016.10.106
Bürgi M., Li L., Kizos T. (2015). Exploring links between culture and biodiversity: studying land use intensity from the plot to the landscape level. Biodivers. Conserv. 24 (13), 3285–3303. doi: 10.1007/s10531-015-0970-0
Calhoun A. J. K., Mushet D. M., Bell K. P., Boix D., Fitzsimons J. A., Isselin-Nondedeu F. (2017). Temporary wetlands: challenges and solutions to conserving a ‘disappearing’ ecosystem. Biol. Conserv. 211, 3–11. doi: 10.1016/j.biocon.2016.11.024
Cavanaugh J. E. (1997). Unifying the derivations for the akaike and corrected akaike information criteria. Stat Probabil. Lett. 33 (2), 201–208. doi: 10.1016/S0167-7152(96)00128-9
CBD. (2021a). "First draft of the post-2020 global biodiversity framework CBD/WG2020/3/3" (United Nations). Available at: https://www.cbd.int/article/draft-1-global-biodiversity-framework.
CBD. (2021a). “"Kunming declaration: Declaration from the high-level segment of the UN biodiversity conference 2020 (Part 1) under the theme: “Ecological civilization: Building a shared future for all life on earth”",” in The high-level segment of the UN biodiversity conference 2020 (Part 1) (United Nations).
Connell J. H. (1978). Diversity in tropical rain forests and coral reefs. Science 199 (4335), 1302–1310. doi: 10.1126/science.199.4335.1302
da Silva P. G., Lobo J. M., Hensen M. C., Vaz-de-Mello F. Z., Hernández M. I. (2018). Turnover and nestedness in subtropical dung beetle assemblages along an elevational gradient. Diversity Distribut. 24 (9), 1277–1290. doi: 10.1111/ddi.12763
BirdLife International, NatureServe. (2011). Bird species distribution maps of the world (Cambridge and Arlington: BirdLife International and NatureServe).
Díaz S. M., Settele J., Brondízio E., Ngo H., Guèze M., Agard J., et al. (2019). The global assessment report on biodiversity and ecosystem services: Intergovernmental Science-Policy Platform on Biodiversity and Ecosystem Services. Nairobi, Kenya: United Nations Environment Programme.
Dudley N., Alexander S. (2017). Agriculture and biodiversity: A review. Biodiversity 18 (2-3), 45–49. doi: 10.1080/14888386.2017.1351892
Duelli P. (1997). Biodiversity evaluation in agricultural landscapes: An approach at two different scales. Agric. Ecosyst. Environ. 62 (2-3), 81–91. doi: 10.1016/S0167-8809(96)01143-7
Fagerholm N., Kayhko N., Ndumbaro F., Khamis M. (2012). Community stakeholders' knowledge in landscape assessments - mapping indicators for landscape services. Ecol. Indic. 18, 421–433. doi: 10.1016/j.ecolind.2011.12.004
Fischer J., Abson D. J., Butsic V., Chappell M. J., Ekroos J., Hanspach J., et al. (2014). Land sparing versus land sharing: moving forward. Conserv. Lett. 7 (3), 149–157. doi: 10.1111/conl.12084
Fischer J., Brosi B., Daily G. C., Ehrlich P. R., Goldman R., Goldstein J., et al. (2008). Should agricultural policies encourage land sparing or wildlife-friendly farming? Front. Ecol. Environ. 6 (7), 380–385. doi: 10.1890/070019
Fontana V., Guariento E., Hilpold A., Niedrist G., Steinwandter M., Spitale D., et al. (2020). Species richness and beta diversity patterns of multiple taxa along an elevational gradient in pastured grasslands in the European Alps. Sci. Rep. 10 (1), 1–11. doi: 10.1038/s41598-020-69569-9
Fox J. W. (2012). The intermediate disturbance hypothesis should be abandoned. Trends Ecol. Evol. 28 (2), 86–92. doi: 10.1016/j.tree.2012.08.014
Fox J. W. (2013). The intermediate disturbance hypothesis is broadly defined, substantive issues are key: a reply to sheil and burslem. Trends Ecol. Evol. 28 (10), 572–573. doi: 10.1016/j.tree.2013.07.008
Fritz A., Li L., Storch I., Koch B. (2018). UAV-derived habitat predictors contribute strongly to understanding avian species–habitat relationships on the Eastern qinghai-Tibetan plateau. Remote Sens. Ecol. Conserv. 4 (1), 53–65. doi: 10.1002/rse2.73
Goslee S. C., Urban D. L. (2007). The ecodist package for dissimilarity-based analysis of ecological data. J. Stat. Softw. 22, 1–19. doi: 10.18637/jss.v022.i07
Grau R., Kuemmerle T., Macchi L. (2013). Beyond ‘land sparing versus land sharing’: environmental heterogeneity, globalization and the balance between agricultural production and nature conservation. Curr. Opin. Environ. Sustain. 5 (5), 477–483. doi: 10.1016/j.cosust.2013.06.001
Gregory R. D., Gibbons D. W., Donald P. F. (2004). “"Bird census and survey techniques,",” in Bird ecology and conservation: a handbook of techniques. Eds. Sutherland W. J., Newton I., Green R. E. (Oxford: Oxford University Press), 17–56.
Grime J. P. (1973). Competitive exclusion in herbaceous vegetation. Nature 242 (5396), 344–347. doi: 10.1038/242344a0
Jacquemyn H., Honnay O., Pailler T. (2007). Range size variation, nestedness and species turnover of orchid species along an altitudinal gradient on réunion island: implications for conservation. Biol. Conserv. 136 (3), 388–397. doi: 10.1016/j.biocon.2006.12.008
Kaphengst T., Ward D. (2008). Effects of habitat structure and shrub encroachment on bird species diversity in arid savanna in northern cape province, south Africa. Ostrich 79 (2), 133–140. doi: 10.2989/OSTRICH.2008.79.2.2.576
Koch B., Edwards P. J., Blanckenhorn W. U., Walter T., Hofer G. (2015). Shrub encroachment affects the diversity of plants, butterflies, and grasshoppers on two Swiss subalpine pastures. Arctic. Antarctic. Alp. Res. 47 (2), 345–357. doi: 10.1657/AAAR0013-093
Lai C. H., Smith A. T. (2003). Keystone status of plateau pikas (Ochotona curzoniae): effect of control on biodiversity of native birds. Biodivers. Conserv. 12 (9), 1901–1912. doi: 10.1023/A:1024161409110
Legendre P., Lapointe F. J., Casgrain P. (1994). Modeling brain evolution from behavior: a permutational regression approach. Evolution 48 (5), 1487–1499. doi: 10.1111/j.1558-5646.1994.tb02191.x
Lei F., Qu Y., Song G. (2014). Species diversification and phylogeographical patterns of birds in response to the uplift of the qinghai-Tibet plateau and quaternary glaciations. Curr. Zool. 60 (2), 149–161. doi: 10.1093/czoolo/60.2.149
Lei F.-M., Wei G.-A., Zhao H.-F., Yin Z.-H., Lu J.-L. (2007). China Subregional avian endemism and biodiversity conservation. Biodivers. Conserv. 16 (4), 1119–1130. doi: 10.1007/s10531-006-9080-3
Li N., Chu H., Qi Y., Li C., Ping X., Sun Y., et al. (2019). Alpha and beta diversity of birds along elevational vegetation zones on the southern slope of Altai mountains: Implication for conservation. Global Ecol. Conserv. 19, e00643. doi: 10.1016/j.gecco.2019.e00643
Li L., Fassnacht F. E., Bürgi M. (2021). Using a landscape ecological perspective to analyze regime shifts in social–ecological systems: a case study on grassland degradation of the Tibetan plateau. Landscape Ecol. 36 (8), 2277-2293. doi: 10.1007/s10980-021-01191-0
Li L., Fassnacht F. E., Storch I., Bürgi M. (2017). Land-use regime shift triggered the recent degradation of alpine pastures in nyanpo yutse of the eastern qinghai-Tibetan plateau. Landscape Ecol. 32 (11), 2187–2203. doi: 10.1007/s10980-017-0510-2
Li D., Li J. (2002). Biodiversity of three river source region (Beijing: China Science and Technology Publishing House).
Li L., Lü Z. (2019). Multifunctionality of land and ecological compensation: Thoughts on biodiversity mainstreaming. Res. Ind. Econ. 7 (4), 12–17. doi: 10.19676/j.cnki.1672-6995.0000306
Li L., Tietze D. T., Fritz A., Lü Z., Bürgi M., Storch I. (2018). Rewilding cultural landscape potentially puts both avian diversity and endemism at risk: A Tibetan plateau case study. Biol. Conserv. 224, 75–86. doi: 10.1016/j.biocon.2018.05.008
Long R. (2003). “"Alpine rangeland ecosystems and their management in the qinghai-Tibetan plateau,",” in The yak. Eds. Wiener G., Han J., Long R., Cai L.(Bangkok: FAO Regional Office for Asia and the Pacific), 359–388.
Mackey R. L., Currie D. J. (2001). The diversity-disturbance relationship: Is it generally strong and peaked? Ecology 82 (12), 3479–3492. doi: 10.1890/0012-9658(2001)082[3479:Tddrii]2.0.Co;2
Manning A. D., Fischer J., Lindenmayer D. B. (2006). Scattered trees are keystone structures–implications for conservation. Biol. Conserv. 132 (3), 311–321. doi: 10.1016/j.biocon.2006.04.023
Meng Y. (2012) The development of sanjiangyuan national ecosystem protection experimental region (Xining: Xining Evening Paper). Available at: http://www.qh.gov.cn/system/2012/01/14/010619802.shtml (Accessed 5.22 2012).
Miehe G., Miehe S., Kaiser K., Reudenbach C., Behrendes L., Duo L., et al. (2009). How old is pastoralism in Tibet? an ecological approach to the making of a Tibetan landscape. Palaeogeogr. Palaeoclimatol. Palaeoecol. 276 (1-4), 130–147. doi: 10.1016/j.palaeo.2009.03.005
Miehe G., Schleuss P.-M., Seeber E., Babel W., Biermann T., Braendle M., et al. (2019). The kobresia pygmaea ecosystem of the Tibetan highlands–origin, functioning and degradation of the world's largest pastoral alpine ecosystem: Kobresia pastures of Tibet. Sci. Tot. Environ. 648, 754–771. doi: 10.1016/j.scitotenv.2018.08.164
Mori A. S., Isbell F., Seidl R. (2018). β-diversity, community assembly, and ecosystem functioning. Trends Ecol. Evol. 33 (7), 549–564. doi: 10.1016/j.tree.2018.04.012
National Development and Reform Commission (2013). "The second phase planning of the qinghai sanjiangyuan ecological protection and construction project". Retrieved from: http://www.sdpc.gov.cn/fzgggz/ncjj/zhdt/201404/W020140411575855950848.pdf
Oksanen J., Blanchet F. G., Friendly M., Kindt R., Legendre P., McGlinn D., et al. (2017). "vegan: Community ecology package". r package version 2.4-3. Retrieved from: https://CRAN.R-project.org/package=vegan.
Päckert M., Favre A., Schnitzler J., Martens J., Sun Y.-H., Tietze D. T., et al. (2020). “Into and out of” the qinghai-Tibet plateau and the Himalayas: Centers of origin and diversification across five clades of Eurasian montane and alpine passerine birds. Ecol. Evol. 10 (17), 9283–9300. doi: 10.1002/ece3.6615
Päckert M., Martens J., Sun Y.-H., Strutzenberger P. (2016). The phylogenetic relationships of przevalski's finch urocynchramus pylzowi, the most ancient Tibetan endemic passerine known to date. Ibis 158 (3), 530–540. doi: 10.1111/ibi.12382
Päckert M., Martens J., Sun Y. H., Tietze D. T. (2015). Evolutionary history of passerine birds (Aves: Passeriformes) from the qinghai-Tibetan plateau: from a pre-quarternary perspective to an integrative biodiversity assessment. J. Ornithol. 156, S355–S365. doi: 10.1007/s10336-015-1185-6
People’s Government of Jikdril County (2011) Notification on the implementation protocol of the grassland eco-compensation poliy in jikdril 2011 (Accessed Dec 4 2020).
Pickett S. T., White P. S. (1985). The ecology of natural disturbance and patch dynamics (Orlando, Fla: Academic Press).
Poschlod P., Braun-Reichert R. (2017). Small natural features with large ecological roles in ancient agricultural landscapes of central Europe - history, value, status, and conservation. Biol. Conserv. 211, 60–68. doi: 10.1016/j.biocon.2016.12.016
Qian Q., Zhang X., Wang J., Ye H., Li Y., Zhang Z. (2021). The spatio-temporal pattern of grazing pressure in the three-river headwaters in qinghai province from 2005 to 2017. Acta Agrestia. Sin. 6), 1311–1317. doi: 10.11733/j.issn.1007-0435.2021.06.022
Qinghai Provincial Government (2021). "14th five-year plan for the national economic and social development of qinghai province". Retrieved from: http://swt.qinghai.gov.cn/sy/swxw/202201/t20220105_188849_wap.html.
Qu Y., Chen C., Chen X., Hao Y., She H., Wang M., et al. (2021). The evolution of ancestral and species-specific adaptations in snowfinches at the qinghai–Tibet plateau. Proc. Natl. Acad. Sci. 118 (13), e2012398118. doi: 10.1073/pnas.2012398118
R Core Team (2021). R: A language and environment for statistical computing (Vienna, Austria: R Foundation for Statistical Computing).
Santana J., Porto M., Reino L., Moreira F., Ribeiro P. F., Santos J. L., et al. (2017). Using beta diversity to inform agricultural policies and conservation actions on Mediterranean farmland. J. Appl. Ecol. 54 (6), 1825–1835. doi: 10.1111/1365-2664.12898
Sirami C., Monadjem A. (2012). Changes in bird communities in Swaziland savannas between 1998 and 2008 owing to shrub encroachment. Diversity Distribut. 18 (4), 390–400. doi: 10.1111/j.1472-4642.2011.00810.x
Socolar J. B., Gilroy J. J., Kunin W. E., Edwards D. P. (2016). How should beta-diversity inform biodiversity conservation? Trends Ecol. Evol. 31 (1), 67–80. doi: 10.1016/j.tree.2015.11.005
Stuart C. T., Brault S., Rowe G. T., Wei C. L., Wagstaff M., McClain C. R., et al. (2017). Nestedness and species replacement along bathymetric gradients in the deep sea reflect productivity: a test with polychaete assemblages in the oligotrophic north-west gulf of Mexico. J. Biogeogr. 44 (3), 548–555. doi: 10.1111/jbi.12810
Ter Braak C. J. F. (1986). Canonical correspondence-analysis - a new eigenvector technique for multivariate direct gradient analysis. Ecology 67 (5), 1167–1179. doi: 10.2307/1938672
Tews J., Brose U., Grimm V., Tielbörger K., Wichmann M., Schwager M., et al. (2004). Animal species diversity driven by habitat heterogeneity/diversity: the importance of keystone structures. J. Biogeogr. 31 (1), 79–92. doi: 10.1046/j.0305-0270.2003.00994.x
Tscharntke T., Tylianakis J. M., Rand T. A., Didham R. K., Fahrig L., Batary P., et al. (2012). Landscape moderation of biodiversity patterns and processes - eight hypotheses. Biol. Rev. 87 (3), 661–685. doi: 10.1111/j.1469-185X.2011.00216.x
White P. S., Pickett S. T. (1985). “Natural disturbance and patch dynamics: an introduction,” in The ecology of natural disturbance and patch dynamics. Eds. Pickett S. T., White P. S. (Orlando, Fla: Academic Press), 3–13.
Whittaker R. H. (1960). Vegetation of the siskiyou mountains, Oregon and California. Ecol. Monogr. 30 (3), 279–338. doi: 10.2307/1943563
Whittaker R. H. (1972). Evolution and measurement of species diversity. Taxon 21 (2-3), 213–251. doi: 10.2307/1218190
Keywords: livestock grazing intensity, biodiversity, turnover, nestedness, unmanned aerial vehicle, agricultural landscape, multifunctionality
Citation: Li L, Tietze DT, Fritz A, Basile M, Lü Z and Storch I (2022) Beta diversities of grassland birds indicate the importance of pastoralism for nature conservation of the Qinghai–Tibetan plateau. Front. Conserv. Sci. 3:902887. doi: 10.3389/fcosc.2022.902887
Received: 23 March 2022; Accepted: 11 July 2022;
Published: 09 August 2022.
Edited by:
Xiaoli Shen, Institute of Botany, Chinese Academy of Sciences (CAS), ChinaReviewed by:
Jianjun Cao, Northwest Normal University, ChinaHong Mingsheng, China West Normal University, China
Cameron Duquette, New Mexico State University, United States
Edward James Raynor, United States Department of Agriculture, United States
Copyright © 2022 Li, Tietze, Fritz, Basile, Lü and Storch. This is an open-access article distributed under the terms of the Creative Commons Attribution License (CC BY). The use, distribution or reproduction in other forums is permitted, provided the original author(s) and the copyright owner(s) are credited and that the original publication in this journal is cited, in accordance with accepted academic practice. No use, distribution or reproduction is permitted which does not comply with these terms.
*Correspondence: Li Li, bGkubGkwMUB4anRsdS5lZHUuY24=