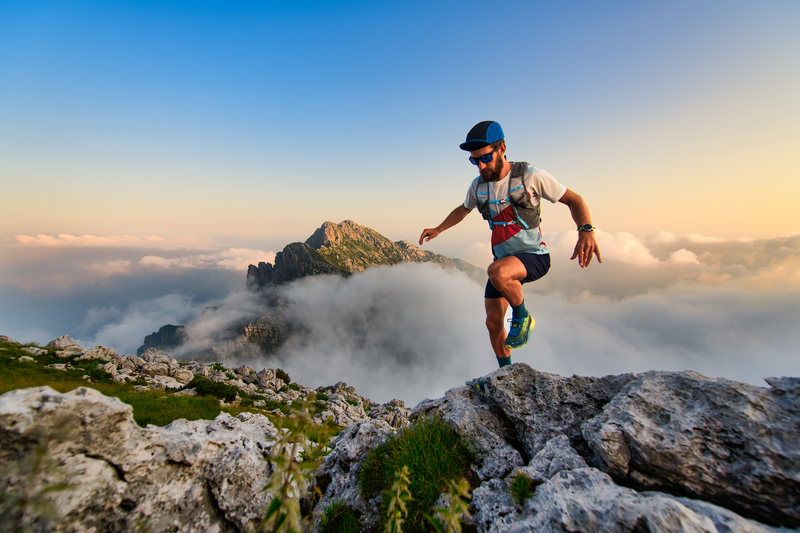
94% of researchers rate our articles as excellent or good
Learn more about the work of our research integrity team to safeguard the quality of each article we publish.
Find out more
ORIGINAL RESEARCH article
Front. Conserv. Sci. , 16 September 2022
Sec. Global Biodiversity Threats
Volume 3 - 2022 | https://doi.org/10.3389/fcosc.2022.768274
This article is part of the Research Topic Global Urban Biodiversity and the Importance of Scale View all 9 articles
Urban biodiversity plays an important role in ecological processes and ecosystem services within cities, making conservation a priority in many municipal sustainability plans. Urban green spaces (UGS) have been a key strategy for conservation by providing habitat for wildlife, including avian communities. While the ecological attributes necessary to enhance the habitability of UGS for avian communities are relatively well known, an understanding of how variation in the surrounding urban matrix influences avian richness outcomes in these spaces, is less understood. As new urban areas continue to develop and UGS become increasingly important habitat areas, urban designers and planners will need a better understanding of the ways in which urban built form patterns at the matrix-level influence avian biodiversity outcomes at the site-level in UGS. To that end, this study investigates the influence of 4 urban built form matrix-level variables , capturing three-dimensional (3D) configuration patterns, and 6 UGS site-level variables on total avian richness and avian richness by foraging guild using generalized linear model methods in 22 UGS. This analysis was conducted using high resolution land cover data, LiDAR data, and twenty years of bird occurrence data from the eBird community science program in well-surveyed UGS in Los Angeles, California. The size of the UGS was the best predictor of richness patterns across all groups, confirming previous findings. However, several urban form metrics, when included with UGS size, improved model prediction for Carnivores, Insectivores, and Omnivore foraging guilds, indicating that urban built form does influence some avian groups within UGS. The results of this study suggest that the most important factor for avian richness in UGS is the size of the green space, but that urban built form plays a role too, especially when considered from a 3D perspective.
By 2100, the impact of land use change globally on biodiversity may be more significant than climate change, as urban land is projected to triple between 2000 and 2030 (Haines-Young, 2009; McDonald et al., 2020). Conversion of previously undeveloped land to urban land uses causes biodiversity loss via fragmentation or loss of habitat at both local and regional scales Aronson et al., 2014. Despite these negative impacts, urban centers in some cases support more species than natural reference systems, including endangered and threatened species (Grimm et al., 2008; Aronson et al., 2014; Elmqvist et al., 2016). Urban green spaces (UGS) have played a critical role in serving as habitat in urban areas and in creating landscape connectivity to natural reference systems (HostetlerKnowles-Yanez, 2003; Amaya-Espinel et al., 2019; La Sorte et al., 2020). This not only makes urban areas, and UGS in particular, important for biodiversity conservation, but demonstrates that urban form is not homogenous and that biodiversity loss from urbanization is not inevitable.
According to current research, the world’s cities are dominated by native avian species despite overall density of species declining in urban regions (Aronson et al., 2014; Lepczyk et al., 2017). As avian species persist and even thrive in cities despite the multitude of disturbances and predators, many questions remain as to how urban form and location influence avian richness (Marzluff, 2017). Several studies have linked various features of urban form, both built and green, to avian biodiversity outcomes (Hostetler and Knowles-Yanez, 2003; Ikin et al., 2013; Strohbach et al., 2013; Sushinsky et al., 2013; Andersson and Colding, 2014; Dale, 2018; Sen, 2019; Lindenmayer et al., 2020; Beaugeard et al., 2020; Kaushik et al., 2020). However, most research is centered around enhancing green infrastructure and urban green spaces to create functional habitat and landscape connectivity via green corridors to natural reference systems (Beninde et al., 2015; Nilon et al., 2017; Garrard et al., 2018).
Research on the benefits of UGS and increased vegetation cover for avian biodiversity have demonstrated consistently positive outcomes (Beninde et al., 2015; Threlfall et al., 2016). A wealth of studies analyzing intra-urban biodiversity variation, including avian biodiversity, have generally found that increases in patch area and corridors that create landscape connectivity to natural reference systems have the most positive relationships with biodiversity in cities, followed by vegetation structure (Beninde et al., 2015; Schütz & Schulze, 2015; Plummer et al., 2020). A recent study of avian richness in New York’s UGS confirmed that UGS size has the most significant impact on avian richness outcomes La Sorte et al., 2020. Another study looking at both avian richness and abundance in UGS in Santiago, Chile, specifically the impacts of urban density, found that increased density negatively influenced bird community outcomes in UGS (Trollope et al., 2009; Amaya-Espinel et al., 2019). Taken together, these findings suggest that UGS cannot be seen as a panacea and consideration of the surrounding urban matrix is critical to ensuring urban avian biodiversity conservation.
The results have been mixed on the influence of various attributes of urban built form on avian biodiversity, especially when avian species are analyzed by ecological guild Amaya-Espinel et al., 2019. Most studies that consider urban built form have focused on 2D measures of density, i.e., degree of urban built-up area. These studies have found mixed results, from negative to neutral and even mixed (Hostetler & Knowles-Yanez, 2003; Aronson et al., 2014; Dale, 2018). Furthermore, another study concluded that using the percentage of built-up surface area to calculate urban density is a poor indicator of urban biodiversity potential (Brunbjerg et al., 2018). This suggests that more detailed measurements of urban built form, beyond two-dimensional (2D) measures and ranges of density, may be needed.
In previous studies, there has been less focus on urban built form configuration, especially from a three-dimensional (3D) perspective, whereby the dimensionality and spatial pattern of urban built form is taken into consideration. Analysis of urban built form configuration has largely been measured in 2D space due to challenges of data acquisition and storage Wentz et al., 2018. When studies do take into consideration 3D metrics, it is usually only building height, which has had mixed results on impacts to avian biodiversity (Loss et al., 2014; MacGregor-Fors et al., 2017; Amaya-Espinel et al., 2019). Urban ecology scholars suggest that configuration, both 2D and 3D space, are necessary to accurately characterize urban form, and can provide a better understanding of ecological connectivity, particularly for avian species (Wentz et al., 2018; Choi et al., 2021). For example, researchers have demonstrated that quantifying urban form both from a 2D and 3D perspective has implications on urban flight corridors for migratory birds as well as acoustic transmission and airflow, which can alter avian flight patterns (Warren et al., 2006; Callaghan et al., 2018; Liu et al., 2021). In another recent study, researchers have determined that quantifying urban landscapes at 1 km scale using multidimensional metrics facilitates the detection of urban biodiversity patterns and underlying processes forming those patterns, providing a more nuanced understanding of the ways in which urban landscapes shape urban biodiversity (Alberti and Wang, 2022). Arguably, capturing more detailed 2D and 3D measures of urban built form surrounding UGS will provide greater insight into the influence of urban built form patterns on avian richness outcomes in UGS.
The pattern and form of new urban areas will have profound impacts on habitats around the world, but increased knowledge on the ways in which urban built form can support avian biodiversity will help shape more positive outcomes. While the ecological attributes that enhance the habitability of UGS for avian communities are relatively well known, an understanding of how variation in the surrounding urban matrix influences richness outcomes in these spaces is less understood, especially when considering 3D measurements of urban built form (Zhou et al., 2011; Connors et al., 2013; Andersson & Colding, 2014; Beninde et al., 2015; McPhearson et al., 2016; Wentz et al., 2018). A better understanding of the role of urban built form in influencing avian richness patterns in UGS will help urban design and planning practitioners develop more effective biodiversity management requirements and plans.
The main aim of this study is to assess if variation in urban built form at the matrix-level influences avian biodiversity outcomes in UGS using 2D and 3D measures of built form. This objective is explored by testing the influence of 4 urban built form matrix-level variables that characterize various aspects of urban built form configuration patterns, and 6 UGS site-level variables on avian richness outcomes grouped by foraging guild. Given the mixed results in several studies investigating different aspects of the urban built environment and avian biodiversity outcomes, I hypothesized that while these variables will demonstrate a relationship to richness patterns, the relationships will not be consistent and will likely change depending on the foraging guild being considered. Most avian biodiversity studies demonstrate the importance of habitat size, composition and structure in outcomes (Beninde et al., 2015). Thus, I hypothesized that urban built form variables will be important to include as predictors in models, but will not be as significant as UGS variables at the site-level, such as patch size, water cover, and vegetation cover, in determining avian richness outcomes. This study fills a gap by contributing a more complete understanding of how urban built form influences avian richness outcomes in UGS.
This study examines avian richness in UGS in the City of Los Angeles, California. Los Angeles has approximately 11 percent open green space, and has 1,255 general green spaces listed by the California Protected Areas Database (CPAD) GreenInfoNetwork, 2018. Los Angeles has a Mediterranean climate and is situated within the California Floristic Province, which covers most of the state, and was designated a biodiversity hotspot in 1996 (California Academy of Sciences, 2005). The City has more than 150 threatened or endangered species Brown, 2018. At the same time, it is home to 3.9 million residents, and is nested within LA County, which has an additional 6.1 million residents. The City has a total area of 130,276 hectares and has varying degrees of development intensity. Los Angeles is an ideal city to study given the wide range of development intensities and range of highly modified green spaces to natural remnant habitats (Figure 1). Moreover, in 2017, the Los Angeles City Council passed Biodiversity Motion 25A which resulted in a custom biodiversity tracking index to conserve biodiversity, and the 2015 Sustainable pLAn has a goal of no-net-biodiversity loss by 2035 Brown, 2019. Responding to these efforts, a follow-up report was released in 2020, with an updated biodiversity index and management framework with local case studies demonstrating successful management of particular areas Zaldivar et al., 2020. Additionally, Los Angeles has superior bird count coverage in the community science platform eBird, leading all U.S. counties in its number of bird count checklists, which provides a useful dataset to quantify avian richness patterns Audubon, 2015.
High resolution aerial imagery, the California Protected Areas Database (CPAD) GIS dataset, and the software ArcGIS were used to calculate site-level attributes for sample UGS. In order to get sample UGS boundaries in Los Angeles, UGS polygons were extracted from the CPAD GIS dataset at the “super units” level, which captured the parcel boundary of the park, and filtered to only include spaces classified as a park (GreenInfoNetwork, 2018). To extract site-level attributes for each sample UGS, a parcel-level land cover layer, which was derived from high resolution aerial imagery, was clipped to the park boundaries layer. This was possible because the parcel-level matched the boundaries of the parks. This layer was generously shared by the Loyola Marymount University Center for Urban Resilience. It was derived from 10cm resolution aerial imagery as part of the Los Angeles Region Imagery Acquisition Consortium (LARIAC 5) land cover and tree canopy assessment captured in 2017 (Center for Urban Resilience, 2017). The following site-level attributes for each sample UGS were calculated in ArcGIS software patch size, tree canopy cover, water cover, soil cover, shrub cover, grass cover, road cover, paved cover, building cover, pervious cover, and impervious cover. The patch size was calculated using the Geometry tool in ArcGIS and was referenced to the CPAD layer to ensure accuracy (Connors et al, 2013).
LiDAR derived building data, Fragstats, and ArcGIS were used to calculate urban matrix attributes. A buffer with a 200-meter radius surrounding each UGS was used to capture the effect of urban built form on avian species within the UGS. Building data falling within the buffers surrounding sample parks were extracted from the LARIAC 5 Buildings 2017 GIS layer provided by the Los Angeles County Open Data Hub (LA County Data Hub, 2017). The layer captures over 3,000,000 building outlines in Los Angeles County, including building height and building area. To characterize urban built form patterns within the 200-meter buffers, 12 landscape metrics developed by Liu et al. (2017) were initially calculated, which describe urban landscape patterns in 2D and 3D. The following initial variables used were Number of Buildings, Number of Tall Buildings, Tall Building Ratio, Mean Architecture Projection Area, Mean Architecture Height, Architecture Height Standard Deviation, Building Coverge Ratio, Floor Area Ratio, Landscape Shape Index, Patch Density, Aggregation Index, and Shannon’s Diversity Index. These variables capture various aspects of the built environment such as the number of buildings, area of the buildings, the varying heights of buildings, density, shape, aggregation, and the diversity of the buildings. All the variables were calculated in ArcGIS using building height and area, except for the landscape metrics (Landscape Shape Index, Patch Density, Aggregation Index, Shannon’s Diversity Index). These variables were calculated in Fragstats 4.1 software (McGarigal and Marks, 1995). Each building was considered as one patch, so the patch density variable represents the mean number of buildings within a 200m buffer. In order to capture the diversity in building height required to calculate the rest of the landscape metrics in Fragstats, buildings were divided into six categories by size: Bungalow (0-4m), Low Building (4 to 10m), Multi-story building (10 to 19m), Middle-height building (19 to 30m), Tall building (30 to 100m), and Super tall building (>100m) Liu et al., 2017. Refer to Supplemental Materials 1 for a full description of each of the 12 variables and the accompanying equations used to calculate each.
To quantify avian richness patterns in UGS in Los Angeles this study utilized avian occurrence data from the eBird community science dataset provided by The Cornell Lab of Ornithology Sullivan et al., 2014. These data are structured around a checklist that represents observations from a single sampling event. Each checklist includes species observed, the number of each species seen, location and time of occurrence, survey protocol used, and measures of sampling effort used to collect data. Both the eBird Basic Dataset (EBD) and Sampling Event data (SED) were downloaded for all years combined, 2002 to 2021, for Los Angeles County (the program was initiated in 2002). Only complete checklists were selected, allowing for identification of species that were not detected, instead of not reported. The SED provides checklist data to capture sampling effort variables. All grouped checklists were combined into single checklists to remove any duplicates using the R package “AUK” Strimas-Mackey et al., 2020. Only occurrences with sampling events falling within boundaries of UGS were selected using a spatial intersect join function from the R package SF Pebesma, 2021.
Due to the unstructured nature of eBird data there are several limitations such as spatial bias, taxonomic bias, temporal bias, and spatial precision that must be addressed to evaluate valid avian richness patterns. To address spatial precision this study used methods outlined by La Sorte et al. (2020), whereby area sampling protocol records were further filtered to only include records where the sampling effort area did not exceed the maximum area of the UGS, and travel sampling protocol records were filtered to only include records where sampling effort distance did not exceed the maximum length of the UGS. All incidental and stationary sampling protocol records were kept. This resulted in 23,754 EBD records and 75 UGS. To address temporal bias all years of eBird data were used. To address taxonomic bias and spatial bias, all years of eBird data were used in a species distribution model designed to use unstructured data and to assess survey completeness of sample areas.
The R package, KnowBR was used to calculate survey completeness of each UGS and predicted avian richness patterns. Survey completeness is the percentage of observed species richness captured by predicted species richness Lobo et al., 2018. This calculation is an estimate of a sampling events’ ability to capture all species expected to be present at a given time and location Colwell et al., 1994. KnowBR estimates the species accumulation curve using the estimator of Ugland et al. (2003) and the slope between number of actual observed species and number of database records (KnowBR, n.d.). Poorly surveyed areas were removed based on the parameters defined by Lobo et al. (2018). Refer to Supplementary Materials 2 for KnowBR results and parameters used to select well surveyed UGS. After taking these factors into account, 24 UGS were well surveyed. Two parks (Cheviot Hills Park and Recreation Center and Woodley Avenue Park) needed to be removed due to lack of sufficient land cover data. Finally, avian species for the 22 UGS were categorized by the following foraging guild categories: carnivores, granivores, insectivores, nectivores, herbivores, and frugivores (González-Salazar et al., 2014).
To assess the influence of urban built form matrix-level and UGS site-level attributes on avian richness in UGS, total avian richness and richness by foraging guild were analyzed using generalized linear models (GLMs). Models were fitted using the lme4 package in R (R Development Core Team, 2015). Total avian richness, Carnivore, and Insectivore models assumed a negative binomial error distribution using Log as the identity-link function. Granivore, Omnivore, and Nectarivore models assumed a Poisson error distribution using Log as the identity-link function. Herbivores and frugivores foraging guilds were not modeled as they were only represented in a small fraction of the UGS. Collinearity among predictor variables was calculated using a correlation matrix. All variables that were highly correlated (r >0.70) were filtered out. The following urban matrix-level predictor variables were selected: Number of Buildings, Mean Architecture Height, Landscape Shape Index, and Shannon’s Diversity Index. The following site-level predictor variables were selected: patch size, tree cover, grass cover, shrub cover, soil cover, and water cover.
A univariate analysis was conducted first to determine the strength of each predictor on its own. To control for Type 1 errors and the false discovery rate in the multiple comparisons the Benjamini-Hochberg adjustment was used (Benjamini & Hochberg, 1995). To determine the most predictive models for each group the MuMIn package in R was used for model analysis and selection (Burnham and Anderson, 2002). The models were constrained to two variables to avoid overfitting. The most parsimonious group of models for each group were selected using the Akaike information criterion (AICc). To determine the best models for each group, the ΔAICc of each model needed to have a value of less than 2, which is a calculation using the difference in AICc value of a particular model and the AICc value of the best model found for the group (Burnham and Anderson, 2002). In addition, the final models’ predictors were analyzed using the Variance Inflation Factor (VIF), a multicollinearity regression diagnostic. Any models with predictors that had a VIF greater than or equal to two were removed to ensure robustness of the models. Finally, a model-averaging process was used to obtain averages of estimated coefficients and standard errors for each of the variables in the best performing models.
The average patch size was 43.23 hectares with a standard deviation of 69.66, showing variation across sample sites (Table 1). Similarly, urban built form variables also had high standard deviation values (Table 1). This allowed for a meaningful analysis of the influence of patch size and urban built form on avian richness. Total avian richness averaged 72.6 species ± 28.2, ranging from 25 to 145 different species with seemingly higher levels of richness associated with larger UGS, which was later confirmed through the univariate analysis (Figure 2). Of the foraging guilds evaluated, most species were Insectivores (~ 48.74%), followed by Granivores (~16.35%), Carnivores (~ 16.05%), Omnivores (~ 11.69%), Nectarivores (~ 4.14%), and Other (~ 3.03%) (Tables 2, 3).
Overall UGS site-level variables had more significant relationships to avian richness outcomes compared to urban built form matrix-level variables, especially after applying the Benjamini-Hochberg adjustment (Table 4). Matrix-level variables on their own were not descriptive of avian richness outcomes in urban green spaces. Landscape Shape Index initially demonstrated a significant positive relationship to the foraging guild, Carnivores, but lost its significant once controlled for Type I errors. Shannon’s Diversity Index similarly had a significant relationship to Granivores, though it was negatively associated, and was later reduced after the Benjamini-Hochberg adjustment. Patch size was strongly associated with richness across all foraging guilds, even after controlling for Type I errors, except for Omnivores and Nectarivores where the significance was reduced after the Benjamini-Hochberg adjustment (Figure 3, Table 4). Shrub cover also demonstrated significant positive relationships even after controlling for Type I errors for Insectivores and Granivores. Water cover initially demonstrated significant positive relationships across all groups except for Granivores, Insectivores, and Nectarivores, but lost significance after adjusting P values. For a full list of results by variable across each group refer to Supplementary Materials 3–8.
Table 4 Results from univariate model analysis quantifying the influence of urban green space (site-level) and urban built form (matrix-level) variables on avian richness in 22 urban green spaces in Los Angeles.
Figure 3 Relationship between an increase in patch size and avian richness outcomes. (A) total avian richness, (B) carnivores, (C) insectivores, (D) granivores, (E) omnivores, (F) nectarivores.
While urban built form variables were not informative on their own, they were important to include in several models for the following forging guilds: Carnivores, Insectivores, and Omnivores (Table 5). Several models that included urban built form variables were deemed as the best performing models by meeting the AICc criteria of a ΔAICc value of less than 2, including models 6, 7, 8, and 9 (Table 6). Again, Landscape Shape Index was positively associated to richness outcomes in Carnivores and demonstrated a positive relationship to Insectivores too once patch size was included in the model. Shannon’s Diversity Index demonstrated an association with Omnivores again but was positively associated to outcomes once patch size was included in the model. Insectivores as a group were best described by urban built form variables compared to other groups. Number of Buildings, Mean Architecture Height, and Landscape Shape Index were present in the best performing models for Insectivores. An increase in the number of buildings was negatively associated to richness outcomes, while an increase in Mean Architecture Height and Landscape Shape Index had positive relationships.
Table 5 Results of model selection process to quantify the influence of urban green space (site-level) and urban built form (matrix-level) attributes on avian richness in urban green spaces.
Overall, the best performing models in each group were either 4 (Patch Size and Water Cover) or 1 (Patch Size), except for Carnivores, where model 8 was the best (patch size and Landscape Shape Index) (Tables 5, 6). Patch size was the best predictor of avian richness across all groups followed by water cover. An increase in most UGS site-level variables was positively associated to richness outcomes, except for soil cover and tree cover. An increase in tree cover was negatively associated with Carnivores and increased soil cover had negative relationships to both Insectivores and Granivores. To see the AICc, ΔAICc, and weight for each of the best performing models by group see Supplementary Materials 9.
The main aim of this study was to assess if variation in urban built form influences avian biodiversity outcomes in UGS using 2D and 3D measures of built form. The results of this study suggest that urban built form variables at the matrix-level do influence avian richness outcomes for some foraging guilds, but are not strong predictors of outcomes unless paired with patch size in a model. While urban built form variables are not strong predictors of avian richness patterns in UGS individually, they do improve model prediction when they are included with patch size for Carnivores, Insectivores, and Omnivore guilds. Mean Architecture Height, Landscape Shape Index and Shannon’s Diversity Index were present in top performing models, suggesting that urban built form patterns have more complex interactions that go beyond the typical 2D density measures used and do have a relationship with avian richness patterns.
An increase in the number of buildings, was negatively associated with Insectivore richness, confirming a previous study’s finding that an increase in building density has negative impacts on richness patterns in UGS (Amaya-Espinel et al., 2019). Interestingly, an increase in Mean Architecture Height was positively associated with Insectivore richness in model 7. The impact of building height on avian richness has had mixed results in research to date. In some cases, an increase in building height has been positively associated with avian richness (MacGregor-Fors et al., 2017), and in some it has presented negative results (Loss et al., 2014). This suggests that the relationship between avian richness and building height remains complex and varies by species. Also, a diversity in building heights, captured by the Shannon’s Diversity Index variable in model 9, was positively associated with Omnivore richness. This finding suggests that variation in building heights positively impacts richness patterns for some species. Overall, these findings reveal a complex relationship between building heights, their variation, and avian richness patterns, which would have been missed if only average building height was analyzed.
Landscape Shape Index demonstrated positive associations with both Carnivores and Insectivore guilds, suggesting that an increase in complexity of the shape of buildings surrounding urban green spaces can influence richness outcomes. This variable on its own does not have a strong association to outcomes, as demonstrated by the univariate analysis, but does contribute to better model prediction as demonstrated by model 8, one of the best performing models for Carnivores and Insectivores.
It is not clear why an increase in Landscape Shape Index supports avian richness or why an increase in building height variation (Shannon’s Diversity Index) or Mean Architecture Height is positively associated with avian richness. One hypothesis is that these attributes, either aid or inhibit ecological connectivity in the built environment. A limitation of this study is that 3D ecological connectivity was not calculated, which may have shed light on the relationship between urban built form configuration variables and avian richness. Avian species are unique in that they can fly, meaning that what might be considered a barrier to movement for one species is not the same for avian species, which is why a 3D perspective on connectivity is important. For example, scholars have shown that urban flight corridors are an important factor in mapping connectivity, which requires assigning resistive surface scores with 3D building landscapes in mind Liu et al., 2021. The factors that make an attractive urban flight corridor are relatively unknown. However, there is evidence that the configuration of the built environment has implications for acoustic transmission, which may alter avian flight paths due to an ability or inability to communicate (Warren et al., 2006). Another factor that may alter avian flight paths is airflow. One study found that avian species respond to fine-scale airflows, which are moderated by the built environment (Shepard et al., 2016).
While urban built form variables were important in improving the performance of several models, patch size was the strongest predictor and was present in each of the best models across all groups. Ultimately this study confirms previous assertions that the size of the UGS is the strongest predictor (Dale, 2018; Strohbach et al., 2013; Beaugeard et al., 2020; Beninde et al., 2015; Amaya-Espinel et al., 2019; Beaugeard et al., 2020; Lynch, 2019; La Sorte et al., 2020). This finding supports the well-established species-area relationship (Lomolino, 2000). It also demonstrates that the species-area relationship remains the dominant predictor even when considering various urban built form patterns. In addition, this study demonstrates the importance of water cover for avian richness across all groups except for Granivores and Omnivores. The best performing model for all groups except for Carnivores was either patch size alone or patch size with water cover, suggesting the importance of area size and the presence of water. This is likely due to waterfowl and carnivore species with aquatic diets. The finding that water cover is important for many avian species is supported by several studies (Beninde et al., 2015; Callaghan et al., 2018). In sum, the results of this study suggest that the most important factor in avian richness in UGS is the size of the area, but that urban built form plays a role too and improves avian richness model prediction.
The implications of this study for urban avian biodiversity conservation suggests that urban design and planning professionals should aim to maximize UGS size, but that urban built form should not be entirely ignored. A limitation of this study is the small sample size, which was a result of addressing potential limitations of using eBird data. In countering limitations of using eBird data by only selecting well-surveyed UGS, the sample size was reduced from 75 to 22. Thus, more research needs to be conducted ways in which urban built form influences avian richness in UGS using a larger sample size, which will better capture intra and inter heterogeneity. In addition, further investigation needs to be undertaken to understand the underlying mechanisms of urban built form configurations that support or don’t support avian richness, especially as it relates to 3D ecological connectivity.
The raw data supporting the conclusions of this article will be made available by the authors, without undue reservation, with the exception of the land cover data from Loyola Marymount University Center for Urban Resilience. Researchers interested in this dataset may contact Loyola Marymount University Center for Urban Resilience atY3VyZXNAbG11LmVkdQ==to request access. Further inquiries should be directed to the corresponding author.
Ethical review and approval were not required for the animal study because I used secondary data from the community science platform, eBird.
The author confirms being the sole contributor of this work and has approved it for publication.
I am grateful for the financial support provided by NSF NRT: Graduate Traineeship in Integrated Urban Solutions for Food, Energy, and Water Management (INFEWS)–DGE-1735325 and the California NanoSystems Institute.
I would like to express my sincere gratitude to my advisors, Dr. V. Kelly Turner and Dr. Kirsten Schwarz, for their continuous support and invaluable mentorship. Additionally, I would like to express gratitude to my committee and reviewers for their insightful comments and suggestions. Also, I would be remiss if I did not thank Zhiyuan Yao for her help troubleshooting R code for hours and Dr. Nandita Garud for advising me on the GLMs. I deeply appreciate Loyola Marymount University Center for Urban Resilience sharing their land cover and tree canopy assessment of Los Angeles.
The author declares that the research was conducted in the absence of any commercial or financial relationships that could be construed as a potential conflict of interest.
All claims expressed in this article are solely those of the authors and do not necessarily represent those of their affiliated organizations, or those of the publisher, the editors and the reviewers. Any product that may be evaluated in this article, or claim that may be made by its manufacturer, is not guaranteed or endorsed by the publisher.
The Supplementary Material for this article can be found online at: https://www.frontiersin.org/articles/10.3389/fcosc.2022.768274/full#supplementary-material
Alberti M., Wang T. (2022). Detecting patterns of vertebrate biodiversity across the multidimensional urban landscape. Ecol. Lett. 25.4, 1027–1045. doi: 10.1111/ele.13969
Amaya-Espinel J. D., Hostetler M., Henríquez C., Bonacic C. (2019). The influence of building density on Neotropical bird communities found in small urban parks. Landscape and Urban Planning 190, 103578. doi: 10.1016/j.landurbplan.2019.05.009
Andersson E., Colding J. (2014). Understanding how built urban form influences biodiversity. Urban Forestry Urban Greening 13 (2), 221–226. doi: 10.1016/j.ufug.2013.11.002
Aronson M. F. J., La Sorte F. A., Nilon C. H., Katti M., Goddard M. A., Lepczyk C. A., et al. (2014). A global analysis of the impacts of urbanization on bird and plant diversity reveals key anthropogenic drivers. Proc. R. Soc. B: Biol. Sci. 281 (1780), 20133330. doi: 10.1098/rspb.2013.3330
Audubon (2015) Los Angeles Leads all U.S. counties in nationwide bird count. Available at: https://ca.audubon.org/press-release/los-angeles-leads-all-us-counties-nationwide-bird-count.
(2021) TNC omniscape connectivity. Available at: https://tnc.maps.arcgis.com/apps/webappviewer/index.html?id=3cbb9454372e43ffac44b9dda07b5551.
ModestR software for species distribution data management. Available at: http://www.ipez.es/modestr/.
Beaugeard E., Brischoux F., Angelier F. (2020). Green infrastructures and ecological corridors shape avian biodiversity in a small French city. Urban Ecosyst. 24(3), 549–560. doi: 10.1007/s11252-020-01062-7
Beninde J., Veith M., Hochkirch A. (2015). Biodiversity in cities needs space: A meta-analysis of factors determining intra-urban biodiversity variation. Ecol. Lett. 18 (6), 581–592. doi: 10.1111/ele.12427
Benjamini Y., Hochberg Y. (1995). Controlling the false discovery rate: A practical and powerful approach to multiple testing. J. R. Stat. Society: Ser. B (Methodological) 57 (1), 289–300. doi: 10.1111/j.2517-6161.1995.tb02031.x
Brown I. (2018). 2018 biodiversity report city of Los Angeles (Los Angeles, CA: LA Sanitation and Environment). Available at: https://www.lacitysan.org/cs/groups/public/documents/document/y250/mdi0/~edisp/cnt024743.pdf.
Brown I. T. (2019) Managing cities as urban ecosystems: Analysis tools for biodiversity stewardship in Los Angeles [UCLA]. Available at: https://escholarship.org/uc/item/4c81w4nr#metrics.
Brunbjerg A. K., Hale J. D., Bates A. J., Fowler R. E., Rosenfeld E. J., Sadler J. P. (2018). Can patterns of urban biodiversity be predicted using simple measures of green infrastructure? Urban Forestry Urban Greening 32, 143–153. doi: 10.1016/j.ufug.2018.03.015
Burnham K. P., Anderson D. R. (2002). Model selection and multimodel inference: a practical information-theoretic approach. 2nd ed (New York: Springer-Verlag).
California Academy of Sciences (2005) Hotspot California on the edge. Available at: https://www.nps.gov/goga/learn/management/upload/-1214-HOTSPOT-California-On-The-Edge-1.pdf (Accessed 2021).
Callaghan C. T., Major R. E., Lyons M. B., Martin J. M., Kingsford R. T. (2018). The effects of local and landscape habitat attributes on bird diversity in urban greenspaces. Ecosphere 9 (7), e02347. doi: 10.1002/ecs2.2347
Center for Urban Resilience (2017). LA landcover and tree canopy assessment [Unpublished raw data]. Loyola Marymount University.
Choi H., Song Y., Kang W., Thorne J. H., Song W., Kun Lee D. (2021). LiDAR-derived three-dimensional ecological connectivity mapping for urban bird species. Landscape Ecol. 36 (2), 581–599. doi: 10.1007/s10980-020-01165-8
Colwell R. K., Coddington J. A., Hawksworth D. L. (1994). Estimating terrestrial biodiversity through extrapolation. Philos. Trans. R. Soc. London Ser. B: Biol. Sci. 345 (1311), 101–118. doi: 10.1098/rstb.1994.0091
Connors J. P., Galletti C. S., Chow W. T. L. (2013). Landscape configuration and urban heat island effects: Assessing the relationship between landscape characteristics and land surface temperature in phoenix, Arizona. Landscape Ecol. 28 (2), 271–283. doi: 10.1007/s10980-012-9833-1
Dale S. (2018). Urban bird community composition influenced by size of urban green spaces, presence of native forest, and urbanization. Urban Ecosyst. 21 (1), 1–14. doi: 10.1007/s11252-017-0706-x
Elmqvist T., Zipperer W., Güneralp B. (2016). “Urbanization, habitat loss, biodiversity decline: Solution pathways to break the cycle,” in Routledge handbook of urbanization and global environmental change, vol. 2016 . Eds. Seta K., Solecki W. D., Griffith C. A. (London and New York: Routledge.), 139–151.
ESRI (2021). ArcGIS desktop: Release 10.8.1. calculate geometry tool (Redlands, CA: Environmental Systems Research Institute).
Garrard G. E., Williams N. S. G., Mata L., Thomas J., Bekessy S. A. (2018). Biodiversity sensitive urban design. Conserv. Lett. 11 (2), e12411. doi: 10.1111/conl.12411
González-Salazar C., Martínez-Meyer E., López-Santiago G. (2014). A hierarchical classification of trophic guilds for north American birds and mammals. Rev. Mexicana Biodiversidad 85, 931–941. doi: 10.7550/rmb.38023
GreenInfoNetwork (2018). California protected areas database. Oakland, California. Available at: https://www.calands.org. Retrieved February 2, 2021.
Grimm N. B., Faeth S. H., Golubiewski N. E., Redman C. L., Wu J., Bai X., et al. (2008). Global change and the ecology of cities. Science 319 (5864), 756–760. doi: 10.1126/science.1150195
Haines-Young R. (2009). Land use and biodiversity relationships. Land Use Policy 26, S178–S186. doi: 10.1016/j.landusepol.2009.08.009
Hostetler M., Holling C. S. (2000). Detecting the scales at which birds respond to structure in urban landscapes. Urban Ecosyst. 4 (1), 25–54. doi: 10.1023/A:1009587719462
Hostetler M., Knowles-Yanez K. (2003). Land use, scale, and bird distributions in the phoenix metropolitan area. Landscape Urban Plann. 62 (2), 55–68. doi: 10.1016/S0169-2046(02)00096-8
Ikin K., Beaty R. M., Lindenmayer D. B., Knight E., Fischer J., Manning A. D. (2013). Pocket parks in a compact city: How do birds respond to increasing residential density? Landscape Ecol. 28 (1), 45–56. doi: 10.1007/s10980-012-9811-7
Ives C. D., Lentini P. E., Threlfall C. G., Ikin K., Shanahan D. F., Garrard G. E., et al. (2016). Cities are hotspots for threatened species. Global Ecol. Biogeogr. 25 (1), 117–126. doi: 10.1111/geb.12404
Kaushik M., Tiwari S., Manisha K. (2020). Habitat patch size and tree species richness shape the bird community in urban green spaces of rapidly urbanizing region of India. Urban Ecosystems, 25 (2), 423–436. doi: 10.1101/2020.10.23.348391
LA County Data Hub (2017). LARIAC 5 buildings (Socrata). Los Angeles, California. Available at: https://data.lacounty.gov/.
La Sorte F. A., Aronson M. F. J., Lepczyk C. A., Horton K. G. (2020). Area is the primary correlate of annual and seasonal patterns of avian species richness in urban green spaces. Landscape Urban Plann. 203, 103892. doi: 10.1016/j.landurbplan.2020.103892
Lepczyk C. A., La Sorte F. A., Aronson M. F. J., Goddard M. A., MacGregor-Fors I., Nilon C. H., et al. (2017). Global patterns and drivers of urban bird diversity. Ecology and conservation of birds in urban environments. Eds. Murgui E., Hedblom M. (eds) Cham, Switzerland: Springer.
Lindenmayer D. B., Blanchard W., Foster C. N., Scheele B. C., Westgate M. J., Stein J., et al. (2020). Habitat amount versus connectivity: An empirical study of bird responses. Biol. Conserv. 241, 108377. doi: 10.1016/j.biocon.2019.108377
Liu Z., Huang Q., Tang G. (2021). Identification of urban flight corridors for migratory birds in the coastal regions of shenzhen city based on three-dimensional landscapes. Landscape Ecol. 36 (7), 2043–2057. doi: 10.1007/s10980-020-01032-6
Liu M., Hu Y.-M., Li C.-L. (2017). Landscape metrics for three-dimensional urban building pattern recognition. Appl. Geogr. 87, 66–72. doi: 10.1016/j.apgeog.2017.07.011
Lobo J. M., Hortal J., Yela J. L., Millán A., Sánchez-Fernández D., García-Roselló E., et al. (2018). KnowBR: An application to map the geographical variation of survey effort and identify well-surveyed areas from biodiversity databases. Ecol. Indic. 91, 241–248. doi: 10.1016/j.ecolind.2018.03.077
Lomolino M. V. (2000). Ecology’s most general, yet protean pattern: The species-area relationship. J. Biogeogr. 27 (1), 17–26.
Loss S. R., Will T., Loss S. S., Marra P. P. (2014). Bird–building collisions in the united states: Estimates of annual mortality and species vulnerability. Condor 116 (1), 8–23. doi: 10.1650/CONDOR-13-090.1
Lynch A. J. (2019). Creating effective urban greenways and stepping-stones: Four critical gaps in habitat connectivity planning research. J. Plann. Lit. 34 (2), 131–155. doi: 10.1177/0885412218798334
MacGregor-Fors I., Quesada J., Lee J., Yeh P. (2017). Space invaders: House sparrow densities along three urban-agricultural landscapes. Avian Conserv. Ecol. 12 (2), 11. doi: 10.5751/ACE-01082-120211
Marzluff J. M. (2017). A decadal review of urban ornithology and a prospectus for the future. Ibis 159 (1), 1–13. doi: 10.1111/ibi.12430
McDonald R. I., Mansur A. V., Ascensão F., Colbert M., Crossman K., Elmqvist T., Ziter C., et al. (2020). research gaps in knowledge of the impact of urban growth on biodiversity. Nat. Sustain. 3 (1), 16–24. doi: 10.1038/s41893-019-0436-6
McGarigal K., Marks B. J. (1995). FRAGSTATS: Spatial pattern analysis program for quantifying landscape structure Vol. 122 (Portland, OR: U.S. Department of Agriculture, Forest Service, Pacific Northwest Research Station), p, 351. Gen. Tech. Rep. PNW-GTR-351. doi: 10.2737/PNW-GTR-351
McPhearson T., Pickett S. T. A., Grimm N. B., Niemelä J., Alberti M., Elmqvist T., et al. (2016). Advancing urban ecology toward a science of cities. BioScience 66 (3), 198–212. doi: 10.1093/biosci/biw002
Nilon C. H., Aronson M. F. J., Cilliers S. S., Dobbs C., Frazee L. J., Goddard M. A., et al. (2017). Planning for the future of urban biodiversity: A global review of city-scale initiatives. BioScience 67 (4), 332–342. doi: 10.1093/biosci/bix012
Pebesma E. (2021). Simple Features for R: Standardized Support for Spatial Vector Data. The R Journal 10 (1), 439–446. Available at: https://CRAN.R-project.org/package=sf.
Plummer K. E., Gillings S., Siriwardena G. M. (2020). Evaluating the potential for bird-habitat models to support biodiversity-friendly urban planning. J. Appl. Ecol. 57 (10), 1902–1914. doi: 10.1111/1365-2664.13703
R Development Core Team (2015). R: A language and environment for statistical computing. R Foundation for Statistical Computing, Vienna, Austria. Available at: http://www.R-project.org/.
Schütz C., Schulze C. H. (2015). Functional diversity of urban bird communities: Effects of landscape composition, green space area and vegetation cover. Ecol. Evol. 5 (22), 5230–5239. doi: 10.1002/ece3.1778
Sen S. (2019). A Comparative Study of Avian Biodiversity in Two Sites Viz. Urban and Suburban and in Two Seasons Viz. Winter and Spring Chowdhury K., Sen S. 2019 Harvest 4(1), 70–110. Kolkata, India. Available at SSRN: https://ssrn.com/abstract=3633781
Shepard E. L.C., Williamson C., Windsor S. P. (2016). Fine-scale flight strategies of gulls in urban airflows indicate risk and reward in city living. Philos. Trans. R. Soc. B: Biol. Sci. 371 (1704), 20150394. doi: 10.1098/rstb.2015.0394
Strimas-Mackey M., Hochachka W. M., Ruiz-Gutierrez V., Robinson O. J., Miller E. T., Auer T., et al. (2020). Best Practices for Using eBird Data Version 1.0. https://cornelllabofornithology.github.io/ebird-best-practices/. Cornell Lab of Ornithology, Ithaca, New York. doi: 10.5281/zenodo.3620739. Zenodo.
Strohbach M. W., Lerman S. B., Warren P. S. (2013). Are small greening areas enhancing bird diversity? insights from community-driven greening projects in Boston. Landscape Urban Plann. 114, 69–79. doi: 10.1016/j.landurbplan.2013.02.007
Sullivan B. L., Aycrigg J. L., Barry J. H., Bonney R. E., Bruns N., Cooper C. B., et al. (2014). The eBird enterprise: An integrated approach to development and application of citizen science. Biol. Conserv. 169, 31–40. doi: 10.1016/j.biocon.2013.11.003
Sushinsky J. R., Rhodes J. R., Possingham H. P., Gill T. K., Fuller R. A. (2013). How should we grow cities to minimize their biodiversity impacts? Global Change Biol. 19 (2), 401–410. doi: 10.1111/gcb.12055
Threlfall C. G., Williams N. S. G., Hahs A. K., Livesley S. J. (2016). Approaches to urban vegetation management and the impacts on urban bird and bat assemblages. Landscape Urban Plann. 153, 28–39. doi: 10.1016/j.landurbplan.2016.04.011
Trollope S. T., White J. G., Cooke R. (2009). The response of ground and bark foraging insectivorous birds across an urban–forest gradient. Landscape Urban Plann. 93 (2), 142–150. doi: 10.1016/j.landurbplan.2009.06.013
Ugland K. I., Gray J. S., Ellingsen K. E. (2003). The species–accumulation curve and estimation of species richness. J. Anim. Ecol. 72 (5), 888–897. doi: 10.1046/j.1365-2656.2003.00748.x
Warren P. S., Katti M., Ermann M., Brazel A. (2006). Urban bioacoustics: It’s not just noise. Anim. Behav. 71 (3), 491–502. doi: 10.1016/j.anbehav.2005.07.014
Wentz E. A., York A. M., Alberti M., Conrow L., Fischer H., Inostroza L., et al. (2018). Six fundamental aspects for conceptualizing multidimensional urban form: A spatial mapping perspective. Landscape Urban Plann. 179, 55–62. doi: 10.1016/j.landurbplan.2018.07.007
Zaldivar E. C., Minamide T. J., Mowery L. B., Djiri M., Garcia J. P., Helou A. E., et al. (2020). “2020 biodiversity report city of Los Angeles,” Los Angeles: LA Sanitation and Environment, Los Angeles, California, 110. Available at: https://www.lacitysan.org/san/sandocview?docname=cnt052553.
Keywords: urban biodiversity, urban form, EBird, urban matrix, urban morphology, avian richness, urban green space
Citation: Rogers ML (2022) Urban built form shapes avian richness in green spaces. Front. Conserv. Sci. 3:768274. doi: 10.3389/fcosc.2022.768274
Received: 31 August 2021; Accepted: 16 August 2022;
Published: 16 September 2022.
Edited by:
Piotr Tryjanowski, Poznan University of Life Sciences, PolandReviewed by:
Andy Chan, University of Nottingham, United KingdomCopyright © 2022 Rogers. This is an open-access article distributed under the terms of the Creative Commons Attribution License (CC BY). The use, distribution or reproduction in other forums is permitted, provided the original author(s) and the copyright owner(s) are credited and that the original publication in this journal is cited, in accordance with accepted academic practice. No use, distribution or reproduction is permitted which does not comply with these terms.
*Correspondence: Morgan L. Rogers, bXJvZ2VyczE5QGcudWNsYS5lZHU=
Disclaimer: All claims expressed in this article are solely those of the authors and do not necessarily represent those of their affiliated organizations, or those of the publisher, the editors and the reviewers. Any product that may be evaluated in this article or claim that may be made by its manufacturer is not guaranteed or endorsed by the publisher.
Research integrity at Frontiers
Learn more about the work of our research integrity team to safeguard the quality of each article we publish.