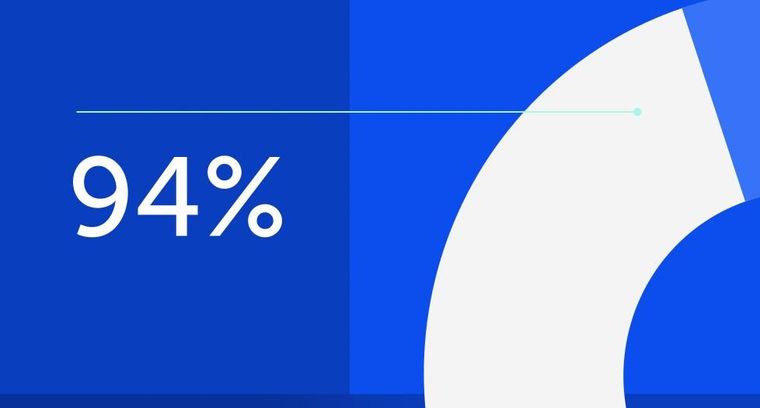
94% of researchers rate our articles as excellent or good
Learn more about the work of our research integrity team to safeguard the quality of each article we publish.
Find out more
ORIGINAL RESEARCH article
Front. Conserv. Sci., 26 January 2022
Sec. Conservation Genetics and Genomics
Volume 2 - 2021 | https://doi.org/10.3389/fcosc.2021.795365
This article is part of the Research TopicIntegrated Phylogeography and Population Genomics of Coastal EcosystemsView all 8 articles
The niche-centroid hypothesis states that populations that are distributed near the centroid of the species' ecological niche will have higher fitness-related attributes, such as population abundance and genetic diversity than populations near the edges of the niche. Empirical evidence based on abundance and, more recently, genetic diversity data support this hypothesis. However, there are few studies that test this hypothesis in coastal species, such as mangroves. Here, we focused on the black mangrove Avicennia germinans. We combined ecological, heterozygosity, and allelic richness information from 1,419 individuals distributed in 40 populations with three main goals: (1) test the relationship between distance to the niche centroid and genetic diversity, (2) determine the set of environmental variables that best explain heterozygosity and allelic richness, and (3) predict the spatial variation in genetic diversity throughout most of the species' natural geographic range. We found a strong correlation between the distance to the niche centroid and both observed heterozygosity (Ho; ρ2 = 0.67 P < 0.05) and expected heterozygosity (He; ρ2 = 0.65, P < 0.05). The niche variables that best explained geographic variation in genetic diversity were soil type and precipitation seasonality. This suggests that these environmental variables influence mangrove growth and establishment, indirectly impacting standing genetic variation. We also predicted the spatial heterozygosity of A. germinans across its natural geographic range in the Americas using regression model coefficients. They showed significant power in predicting the observed data (R2 = 0.65 for Ho; R2 = 0.60 for He), even when we considered independent data sets (R2= 0.28 for Ho; R2 = 0.25 for He). Using this approach, several genetic diversity estimates can be implemented and may take advantage of population genomics to improve genetic diversity predictions. We conclude that the level of genetic diversity in A. germinans is in agreement with expectations of the niche-centroid hypothesis, namely that the highest heterozygosity and allelic richness (the basic genetic units for adaptation) are higher at locations of high environmental suitability. This shows that this approach is a potentially powerful tool in the conservation and management of this species, including for modelling changes in the face of climate change.
Genetic diversity plays a central role in the evolution of species; thus, understanding the factors that affect it and how to estimate it in natural populations are crucial for conservation efforts both now and in the future (Hendry et al., 2011). Genetic diversity is the raw material for natural selection to produce adaptive evolutionary change, and it is a strong predictor of fitness and extinction risk (Frankham, 1996, 2005, 2012; Reed and Frankham, 2003; Spielman et al., 2004). Many factors influence genetic diversity among and within species and genomes (Romiguier et al., 2014; Ellegren and Galtier, 2016). These include, for example, effective population size, which undoubtedly has a key role in determining genetic diversity at the population level (Frankham, 2012; Ellegren and Galtier, 2016).
Genetic diversity and population density are not homogeneous among populations across the landscape of a species' geographic range. Two hypotheses seek to explain this variation empirically—the abundant-centre hypothesis and the niche-centroid hypothesis (Brown, 1984; Martínez-Meyer et al., 2013). The abundant-centre hypothesis predicts a peak of abundance in populations near the geographic centre of the range, while peripheral populations suffer a loss of genetic variation because of reduced effective population size, drift, and inbreeding (Diniz-Filho et al., 2009). Although the abundant-centre hypothesis has received repeated support, there are many exceptions (e.g., Pfeifer et al., 2009; Abeli et al., 2014; Pironon et al., 2015; Dallas et al., 2017; Santini et al., 2018; Kennedy et al., 2020). These exceptions point out that habitat suitability is not necessarily related to the geographic distance from the centre of its distribution range. Rather, fitness, and fitness-related attributes such as demographic parameters may be more strongly influenced by the suitability of local environmental conditions (Hutchinson, 1957; Martínez-Meyer et al., 2013; Lira-Noriega and Manthey, 2014), an idea expressed by the niche-centroid hypothesis. A species' ecological niche is a multidimensional hyper-volume constructed with n-axes, each representing a fundamental variable for population survival (Hutchinson, 1957). The niche hyper-volume has an internal structure such that its centroid represents the optimal conditions (e.g., the highest intrinsic population growth rate) (Maguire, 1973), while populations outside the environmentally defined niche show zero or negative growth (Lira-Noriega and Manthey, 2014). Therefore, distances to environmental niche centroids might better reflect fitness-related attributes, such as population abundance and genetic diversity (Martínez-Meyer et al., 2013).
Recent papers testing the niche-based hypothesis have found that the distance to the niche centroid is negatively related to population abundance and genetic variation, measured mainly as expected heterozygosity, nucleotide diversity, and allelic richness (Yañez-Arenas et al., 2012; Martínez-Meyer et al., 2013; Lira-Noriega and Manthey, 2014; Micheletti and Storfer, 2015; Osorio-Olvera et al., 2016, 2020a; Altamiranda-Saavedra et al., 2020). Under this hypothesis, the highest amount of genetic variation is expected at locations of high environmental suitability, which occurs in regions closer to the niche centroid, while population genetic variability declines as the distance to the niche centroid increases (Lira-Noriega and Manthey, 2014). If population size varies in geographic space, a negative relationship between genetic variation and the distance to the niche centroid is also expected, since larger populations generally harbour more genetic diversity (Diniz-Filho et al., 2009, 2015; Martínez-Meyer et al., 2013).
Integrating ecological niche modelling with population genetics studies can test the link between environmental suitability and population genetic diversity (Micheletti and Storfer, 2015). Meanwhile, predicting the genetic variability of species on the geographical space and defining the environmental variables that best correlate with genetic variability could have important implications for species ecology and conservation biology (Guo et al., 2020). Many studies applying niche modelling techniques have concentrated on temperate terrestrial species, while tropical and marine species have received less attention (Eckert et al., 2008).
Mangrove species play a crucial role in maintaining biodiversity and providing many ecosystem services such as carbon storage, climatic regulation, purifying water, and protection from coastal erosion caused by storms and tsunamis (Costanza et al., 1997; Donato et al., 2011; Tomlinson, 2016; Alongi, 2018). Located at the interface of land and sea, mangrove forests are subject to a variety of environmental stressors such as UV radiation, salinity, tidal inundation, and climatic variability. However, few studies have focused on understanding the environmental requirements associated with the distribution of mangroves (e.g., Crase et al., 2013; Record et al., 2013; Rodríguez-Medina et al., 2020). The information on environmental requirements is relevant to assess the spatial changes of mangrove communities, their composition and relationship to environmental predictors of geographic distribution, and in predicting the effects of future climate change (Rodríguez-Medina et al., 2020). In this study, we focus on the black mangrove Avicennia germinans. First, we evaluate whether environmental suitability, measured as the distance to the niche centroid is a good predictor of the genetic diversity of this species. Then, we determine the set of environmental variables that better explain its genetic variability, and finally, we predict the spatial variation in heterozygosity throughout most of its natural geographic distribution range in the Americas.
Mangroves are flowering trees that live on the coastlines of tropical and subtropical regions of the world (Tomlinson, 2016). Global distributions of mangroves are limited mainly by the physiological tolerance of each species to low temperatures (Duke et al., 1998). The range of conditions over which mangroves occur naturally encompasses other environmental extremes (Clough, 1992). Mangrove species evolved to withstand the tidal regimes of coastal and estuarine shorelines, such as seawater, periodical flooding, and exposure to waves, wind, and offshore currents (Duke, 2017). Mangroves can grow in a wide variety of soil types, including heavy consolidated clays, unconsolidated silts, calcareous and mineral sands, coral rubble, and organic peats, varying in salinity up to 90 ppt (Clough, 1992). Mangroves are also adapted to anaerobic, acidic, or alkaline soils with varying nutrients such as nitrogen, phosphorous, potassium, calcium, magnesium, sodium, and chlorine, common in tidal water (Hossain and Nuruddin, 2016).
Mangrove forests develop best on tropical shorelines with a low wave energy and abundant supply of fine-grained sediments. These ideal conditions occur in areas of high rainfall or ample supply of fresh water through river discharges. Also, these optimal areas are persistently cloudy with a low precipitation-to-evaporation ratio, mild solar radiation fluxes, and negligible climate seasonality. In contrast, high wave energy and sandy substrate are not favourable for mangrove establishment (Clough, 1992; Woodroffe, 1992).
The black mangrove, Avicennia germinans, is a conspicuous mangrove species that grows in the intertidal zone of the tropical and subtropical coastlines of America and West Africa. It is one of the most tolerant species to high salinity, aridity, and low temperatures (Clough, 1992). This species occurs in sympatry with the red mangrove Rhizophora spp. throughout most of its distribution range. However, at local scales, they occupy different intertidal zones, characterised by different types of soils. Avicennia germinans seem to prefer firmer and more sandy substrates associated with fibrous soils (i.e., with a high percentage of the organic matter in the form of undecomposed remains of Rhizophora rootles) (Jordan, 1964; Diop et al., 1997).
The molecular genetic data of black mangrove derives from a total of 1,419 trees from 40 populations covering most of the species' natural geographic range in the Americas, both on the Caribbean and Pacific coasts (Supplementary Table 1). We used two previously published data sets: one from Mexico exclusively (Ochoa-Zavala et al., 2019) and another with Central America and Caribbean localities (Cerón-Souza et al., 2015). The Mexican study had 29 localities with 40 individuals per locality, for a total of 1,144 individuals. After excluding two loci with high null allele frequency, we used eight microsatellite loci ranging between 2.0 and 4.5 alleles per locus (Ochoa-Zavala et al., 2019). The Central American and Caribbean study included 11 localities. The number of individuals ranged from 13 to 40 individuals per population, for a total of 275 and used 11 microsatellites ranging from 3.3 to 9.8 alleles per locus (Cerón-Souza et al., 2015, Supplementary Table 2). Three of the microsatellites were shared between the two studies: AgT4 (tetranucleotide), GT_006 (dinucleotide GT), and CT_004 (dinucleotide CT) (Cerón-Souza et al., 2015; Ochoa-Zavala et al., 2019).
The observed and expected heterozygosity and allelic richness for each locality depends directly on several factors, including the motif, the number of repetitions of each motif, the number of microsatellite molecular markers used, and the number of individuals included in each population (Ellegren, 2000; Väli et al., 2008; Pemberton et al., 2009). To eliminate variation due to a different number of microsatellites between the two studies, we randomly eliminated three of the non-shared markers from the 11-marker set from the study of non-Mexican populations to use a total of eight markers from each dataset. Thus, the microsatellites used from the non-Mexican populations were the three shared loci plus CA_002 (dinucleotide CA), GA_003 (dinucleotide GA), CT_003 (dinucleotide CT), GT_003 (dinucleotide GT), and CTT_001 (trinucleotide CTT). Therefore, the non-Mexican database contained six microsatellites with a dinucleotide motif, one with a trinucleotide motif, and one with a tetranucleotide motif. In comparison, the microsatellites used from the Mexican populations were: Agerm1-25 (TG), Agerm1-18 (AG), AgT4 (CATA), AgD6 ([ATT][GT]), Agerm1-22 (TTTCTT), and AgT9 ([CA][GA][CAGA]). The genetic diversity was calculated separately for each of the 40 populations using observed and expected heterozygosity (Ho, He, respectively) in Arlequin version 3.5 (Excoffier and Lischer, 2010). In addition, we obtained allelic richness (Ar) based on a sample size of 11 alleles using the rarefaction method in hierfstat (Goudet and Jombart, 2015) in R (R Core Team, 2019).
To verify that estimates of heterozygosity (Ho and He) and Ar are not affected by microsatellites' features, we compared the mean values obtained using the set of eight markers with estimates derived from three additional databases: one with three shared loci, a second of one tetranucleotide locus, and a third with four dinucleotide loci. Mean values of each parameter showed the same trend at each locality (Supplementary Figures 1A–E). Then, to corroborate the consistency in our results, we repeated the correlation analyses (see section Assessing the relationship between Niche-centroid distance and genetic diversity) using Ho, He, and Ar computed from these three datasets. For comparison, we also included the correlation coefficient values obtained using all eight microsatellite loci. Values of correlation coefficients showed very similar distribution and median values (Supplementary Figures 1B–F). It must be noticed that the dinucleotide dataset produced a wider distribution of Ho and He, but a similar median value (Supplementary Figures 1D,F). Thus, it is likely that levels of genetic diversity of A. germinans populations correlate with their distance to the niche centroid independently of the microsatellite markers used.
We obtained 3,710 occurrence data for A. germinans from the Global Biodiversity Information Facility (GBIF). We followed standardised steps to curate the data. First, we eliminated wrongly georeferenced records and dubious and duplicated localities. Then, to avoid problems related to spatial autocorrelation, we thinned the data using a spatial filter of 0.08333333 degrees (~9.3 km), which was the spatial resolution of the environmental layers used to model the niche. The process of data curation left us with 227 geo-referenced records of the presence of the species over the extent of its geographic distributional range. We split environmental information at occurrence localities into training and testing data using a random partition of 70% of the records for training and 30% for testing (159 and 68, respectively) (step 1 in Figure 1).
Figure 1. General workflow of ecological niche modelling. Each step of the workflow is detailed in section ecological niche modelling (see Methods). White arrows indicate when information from a previous step was used.
We obtained 341 variables from three different sets of predictors at five arc-minutes of spatial resolution (~ 9.3 km): 19 bioclimatic variables from WorldClim (Hijmans et al., 2005), 316 soil variables retrieved from the SoilGrids database (Hengl et al., 2014), and six variables related to salinity at the sea surface obtained from the Bio-Oracle 2.1 database (Assis et al., 2017). The WorldClim bioclimatic variables came from monthly temperature and precipitation reports from weather stations from 1970 to 2000. The soil variables refer to the following soil properties: depth to the bedrock, organic carbon stock, bulk density, clay, silt, and sand content, soil pH, and soil cation-exchange capacity; soil grids also integrate the most probable class and predicted probabilities for each World Reference Base soil unit and Soil Taxonomy suborder (Hengl et al., 2014). Salinity variables were from satellite-based and in situ oceanographic data (Tyberghein et al., 2012). Salinity rasters for analysis included: maximum, minimum, range, and mean salinity, as well as salinity of the most saline month and salinity of the least saline month (step 2 in Figure 1).
We obtained the Pearson pairwise correlation matrix between all predictors at the occurrence localities to select which environmental data to use to fit ecological niche models (ENM) by retaining variables with a correlation value ≤ 0.8. This resulted in a total of 92 predictors (11, 79, and 2 from WorldClim, Soil Grids, and Bio-Oracle, respectively; see the list of variables in Supplementary Table 3). We masked all of the raster layers using the polygon of M, which is the region hypothesised to be accessible for a species (Barve et al., 2011). Because the black mangrove has high dispersal capacity (Nettel and Dodd, 2007), we defined M as the area occupied by mangroves forests (Giri et al., 2011) along the coastlines of America and West Africa and applied a buffer of 0.5 degrees (step 2 in Figure 1).
To model the black mangrove's niche, we used Minimum Volume Ellipsoid (MVE) models, given that theoretical and empirical work supports the idea that fundamental niches have convex shapes like ellipsoids (Maguire, 1973; Jiménez et al., 2019; Osorio-Olvera et al., 2020a,b). Three parameters define ellipsoids: the niche centroid, the shape matrix, and the cutoff value. The niche centroid is the point in the niche space where fitness reaches its maximum value (Hutchinson, 1957; Maguire, 1973; Martínez-Meyer et al., 2013; Osorio-Olvera et al., 2019). The shape matrix measures how dependent two niche axes are and how they covary. The cutoff value defines the ellipsoid's surface. The function ellipsoid_selection of the ntbox R package (Osorio-Olvera et al., 2020b) allows fitting MVE. This algorithm finds the ellipsoid of the smallest volume that contains a k proportion of training points. Each model is evaluated based on its capability to predict occurrence points via the statistical and performance criteria defined by the user. The ntbox R package uses the binomial test to compute statistical significance and estimates performance via the omission rate, which indicates how well-models created with training data predict occurrence points. In this study, we used as modelling variables the least correlated variables estimated in the environmental data section (steps 2 and 3 in Figure 1; Supplementary Table 3). Thus, we fit MVEs in two, three, and four dimensions within the M polygon using a proportion of k = 0.95 of the training data. To select the best models, we used a p ≤ 0.05 for the binomial test and an omission rate of ≤ 0.10 (step 3 in Figure 1).
We evaluated the relationship between distance to the niche centroid (DNC) and population genetic diversity in two consecutive steps (step 4 in Figure 1). First, based on the models selected in the ENM calibration and selection process, we extracted the environmental information of the geographic positions of the black mangrove populations with genetic diversity data (n = 40). Then, we estimated the Mahalanobis distance to the niche's centroid in R. To do this, for the mahalanobis function, we used as input the parameters that define ellipsoids models: niche-centroid, shape matrix, and the environmental information of each population with genetic diversity data. This produced a vector of distances to the centroid of each ellipsoid model for each genetic diversity record (step 4 in Figure 1). Using this vector of distances, we performed non-linear regression between centroid-distance and the estimated values of Ho, He, and Ar separately. Parameters of the regression models were estimated using the nlsfit function of the R package easynls. Thus, the set of environmental variables that best explained the genetic variability of A. germinans was based on models (for Ho, He, and Ar) that included the combination of variables that (1) met the evaluation criteria (statistical test and good performance, step 3 in Figure 1), and (2) showed the strongest correlation (ρ) between DNC and genetic diversity (hereafter best-fit models). The best-fit model was used to predict the geographic variation in heterozygosity. To do this, we computed a vector (which we called MD) containing the Mahalanobis distance between the niche-centroid and the environmental data of variables from the best-fit-model. We evaluated the regression model at every element of the MD vector and converted this information into a raster (step 4 in Figure 1).
To evaluate the models' accuracy when predicting Ho and He, we first performed linear regressions between the observed (computed from microsatellite data) and predicted (from best-fit-model) genetic estimates, using the observed genetic estimates as the independent variable (x). We tested the assumption of normal distribution of residuals using the Shapiro-Wilk normality test (Royston, 1995) and homoscedasticity by examining the correlation (Pearson method) between the residuals vs. fitted values. Finally, we used independent microsatellite estimates of Ho and He from Mori et al. (2015) and Kennedy et al. (2020) to perform linear regressions using the same strategy as above. We used tangent transformations for observed and predicted Ho and He to meet the assumption of normality of residuals. All statistics were performed using R.
We fit a total of 2,798,250 candidate niche models (4,095; 121,485; and 2,672,670; for ellipsoids built in two, three, and four dimensions, respectively). All of the candidate models generated, only 257,196 models met the statistical test and performance criteria (p ≤ 0.05 and an omission rate ≤ 0.10). Of these models, 50,120 indicated significant relationships between niche-centroid distances and Ho (44,574 and 5,546 were negative and positive, respectively). For He, 41,795 models showed significant associations with DNC (7,319 negative and 34,476 positive) and 58,855 (8,056 positive and 50,799 negative) significant associations were obtained for Ar.
The model fit with Ho, He, and Ar indicated an ecological niche of A. germinans characterized by four dimensions, related to precipitation seasonality (bio15 from WorldClim) and soil classification variables (World Reference Base for Soil Resources – WRB, and the United States Department of Agriculture Soil Taxonomy - USDA). Soil types referred to the estimated probability of occurrence of haplic arenosols, leptic cambisols, luvic phaeozems (IUSS Working Group WRB, 2007), and argids (Soil Survey staff, 2010). Note that Ar retrieve almost the same environmental variables as heterozygosity but included leptic cambisols instead of haplic arenosols. Haplic arenosols comprise sandy soils. Their coarse structure accounts for their general high permeability and low water and nutrient storage capacity, without mentioning other particular soil features (IUSS Working Group WRB, 2007). Leptic cambisols are moderately developed soils with clay or carbonate content; characterized by the absence of organic matter, aluminium and/or iron compounds. Cambisols occur in dry regions and are less common in the humid tropics and subtropics (IUSS Working Group WRB, 2007). Luvic phaeozems are dark soils rich in organic matter with a certain clay content through or up to 50 cm below its upper limit (IUSS Working Group WRB, 2007). Argids are aridisols with an argillic or natric subsuperficial horizon. Therefore, they refer to an accumulation of phyllosilicate clays or silicate clays, respectively (Soil Survey staff, 2010). In summary, the ecological niche of A. germinans was characterized by precipitation seasonality and by soils associated with organic matter, sand, and clay. These soils have some sodium accumulation in subsuperficial horizons.
Regression coefficients indicated strong negative relationships between genetic diversity estimates (ρ2 = 0.67 P < 0.05 for Ho; ρ2 = 0.65, P < 0.05 for He; ρ2 = 0.65, P < 0.05 for Ar) and the distance to the centroid of the ecological niche (Figure 2). Spatial predictions of heterozygosity were estimated with the regression coefficients from the DNC models, based on the equations: y = 0.73 x/ (0.33 + x 1.80) and y = 0.76 x/ (0.3 + x 1.80) for Ho and He, respectively (Figures 3, 4). Our tests to evaluate the accuracy of genetic diversity predictions indicated that both models showed considerable explanatory power for the observed data (R2 = 0.65 for Ho; R2 = 0.60 for He; P < 0.05), even when we consider other independent data sets (R2= 0.28 for Ho; R2 = 0.25 for He; P < 0.05) that surveyed a larger number of microsatellite markers to estimate Ho and He: 22 loci in Mori et al. (2015) and 12 in Kennedy et al. (2020) (Supplementary Figure 2).
Figure 2. Relationship between observed (Ho) and expected heterozygosity (He), and Allelic richness (Ar) with the distance to the niche centroid of Avicennia germinans in the Americas.
Figure 3. Observed heterozygosity (Ho) predicted by niche-centroid-distance over the geographic distribution of Avicennia germinans in the Americas.
Figure 4. Expected heterozygosity (He) predicted by niche-centroid-distance over the geographic distribution of Avicennia germinans in the Americas.
Estimates of DNC suggest that populations closer to the black mangrove's niche centroid are located mainly in the Caribbean coast, specifically on the Yucatan Peninsula and in Honduras, Panama, and the Lesser Antilles (Supplementary Table 4). Black mangrove seemed to be more likely to occur in arenosols, cambisols, and argids when in peripheral populations than near to the centroid-niche (Figure 5) and in phaeozems when nearer to the niche centroid. Unfortunately, there is no additional information from shapefiles of haplic arenosols, leptic cambisols, luvic phaeozems, or argids. Therefore, we cannot make inferences beyond their associations with organic matter, sand, or clay composition.
Figure 5. Range values recorded from populations close to the niche centroid (centre) and populations farther from the central-niche (peripheral) in relation to the environmental variables from the best-fit model for genetic diversity estimates: observed and expected heterozygosity, and allelic richness.
Among the models that showed a significant negative relationship with genetic diversity estimates, we identified models with significantly higher correlation (ρ ranging from −0.8 to −0.7) between niche-centroid distances and Ho, He, and Ar. We observed seven specific environmental variables from these models that appear with high frequency (black stars in Supplementary Figure 3). These variables were related to Luvic Phaeozem soils, Argid soils, precipitation variability (bio15), Aric Regosol soils, Ochrept soils, mean diurnal range (bio02), and mean temperature of the wettest quarter (bio08). Note that Luvic Phaeozems, Argids, and precipitation variability were variables included within the best-fit models for Ho, He, and Ar. Aric regosol refers to weakly developed soils that are particularly common in arid areas, with remnants of diagnostic horizons (IUSS Working Group WRB, 2007). Ochrepts originally formed a subgroup within the inceptisols (Smith, 1986), which are young soils with a weak but notable degree of profile development (Soil Survey staff, 2010). Similar to the other soil types, it seems more likely to find Aric Regosols and Ochrepts in populations farther from the niche centroid (Figure 6). We could not obtain additional information from shapefiles about specific features of these soil types.
Figure 6. Range values recorded from populations close to the niche centroid (centre) and populations farther from the central-niche (peripheral) considering variables of higher frequency of the models with significantly higher correlation (ρ ranging from −0.8 to −0.7) between the distance to the niche's centroid and observed (Ho) and expected heterozygosity (He) and allelic richness (Ar). Light blue boxes refer to variables included within the best-fit models for Ho, He, and Ar.
Precipitation-related variables showed a clear trend between populations near the niche-centroid vs. those at the periphery. These variables were precipitation variability (bio15), mean diurnal range (bio02), and mean temperature of the wettest quarter (bio08). Populations close to the niche centroid exhibited lower precipitation variability than populations farther from the centroid (Figure 6), exhibiting less pronounced differences between dry and rainy seasons. The mean diurnal temperature range showed a trend in which central populations appear to experience, on average, more stable temperatures. In contrast, the mean temperature of the wettest quarter was warmer in the periphery than in central populations (Figure 6).
In this study, we evaluated whether there is a correlation between populations' distance from the niche centroid and their genetic variation. We defined the environmental variables that best predicted the spatial genetic variation of A. germinans along the coastlines of the Americas. Our results underscore the critical role that soil features and precipitation-related variables have on black mangrove population dynamics and distribution and how these variables relate to current genetic variation among populations of A. germinans. These results are relevant in the face of global changes affecting mangroves' distribution, defining conservation units, and understanding adaptation to future environments (Hoffmann and Sgro, 2011; Cavanaugh et al., 2014; Wee et al., 2018).
How far a population is located from the centroid of its niche can determine population abundance, genetic diversity, and genetic structure (Yañez-Arenas et al., 2012; Martínez-Meyer et al., 2013; Lira-Noriega and Manthey, 2014; Micheletti and Storfer, 2015; Osorio-Olvera et al., 2016; Altamiranda-Saavedra et al., 2020). Under this niche-based point of view, distance to the ecological niche centroid can explain (among other factors) spatial patterns of genetic variation because environmental variables impact population dynamics. Thus, there is a close link between the set of habitable conditions and geographic genetic variation (Lira-Noriega and Manthey, 2014). We found a strong correlation between genetic variation and soil types. Therefore, this result suggests that the presence of organic matter, sand, and clay particles influence black mangrove development.
Mangrove forests represent significant global carbon sinks (Donato et al., 2011; Alongi, 2014). Stocks of soil organic carbon (a measurable component of organic matter) vary greatly with climate, vegetation type, parent material, soil texture (silt, clay, and sand composition), and land use, among others (Jobbágy and Jackson, 2000; Gruba et al., 2015; Matsui et al., 2015; Gonçalves et al., 2017). Nonetheless, the annual precipitation is an essential predictor of spatial variation in the carbon concentrations of global mangrove soils (Jardine and Siikamäki, 2014). Studies that have assessed the correlation between the soil organic carbon and soil texture have suggested a close relationship among precipitation regimes, soil organic carbon, and clay content (Wiesmeier et al., 2011; Saiz et al., 2012; Feng et al., 2013). Recently, Zhong et al. (2018) found increased soil organic carbon and clay content levels in sites characterized by higher precipitation levels. The authors argued that higher rainfall and temperature favour the mineralization of soils and the production of clay particles. Thus, this promotes net primary productivity, which incorporates carbon in litter and root exudates. Other related studies highlight that the variation in clay mineral content partly produce soil organic carbon stocks (Blanco-Canqui and Lal, 2004).
At the local level (site-specific), the metabolic activity of microbes governs soil organic carbon storage, which in turn is mediated by the vegetation density of a diverse plant community via higher root inputs, including root exudation (Lange et al., 2015). This process could be occurring near the niche centroid (i.e., Panama). The abundant litter input by more productive trees (e.g., higher diameter at breast height and height) of the dense mangrove forest could promote organic matter accumulation by producing higher litter mass and increasing soil organic carbon (Rahman et al., 2021). Hence, it makes sense that in peripheral populations, Luvic Phaeozem soils are practically absent.
In addition, we found studies that suggested two mechanisms by which the proportion of clay and sand particles may act on mangrove growth and establishment. First, clay could be a favourable substrate for rooting mangrove propagules (seedling density and soil texture r = 0.62; p < 0.01) (Ukpong, 1997). Moreover, soil properties are essential components of the mangrove's environment because soil texture is a crucial determinant of trees' height and density (stems per hectare) in Avicennia and other mangrove species (Ukpong, 1997). On the other hand, seedlings of A. germinans and Laguncularia racemosa growing in hypersaline conditions in 100% sand failed to survive. However, seedlings grown in soil composed of 90% sand and 10% clay had 100% survival in hypersaline conditions, although they showed some leaf discolouration (McMillan, 1975). Seedlings grown in 75% sand and 25% clay soil had a 100 % survival and no observable effect on leaves (McMillan, 1975).
Temperature and precipitation are known to influence global distribution patterns in numerous taxa, including mangrove trees (Woodward and Williams, 1987; Duke et al., 1998; Tomlinson, 2016). Indeed, low air and soil temperatures explain a reduction in growth rate (Clough, 1992). In mangroves, extreme cold events such as frost have harmful effects (e.g., dieback) (Woodroffe and Grindrod, 1991; Krauss et al., 2008). Despite this, recent studies have concluded that temperature alone does not define the latitudinal range limits of the mangrove genera Avicennia, Rhizophora, and Laguncularia (Quisthoudt et al., 2012; Ximenes et al., 2018). Other factors may contribute to determining range limits and habitat suitability for A. germinans' successful establishment. Tree height is a reliable indicator of productivity and the relative suitability of a site (Li et al., 2010). Tree height of A. germinans is correlated negatively with latitude and positively with rainfall (Arreola-Lizárraga et al., 2004; Méndez-Alonzo and López-Portillo, 2008). Indeed, tree height is the most critical factor determining tree diameter (DBH), growth rate, and wood production of mangroves trees at the global scale (Xiong et al., 2019). For instance, there are records of low biomass in the limits of mangrove ecosystems distribution in western Mexico, where aridity and cold winter temperatures are common (Hutchinson et al., 2014). Rainfall affects mangrove tree growth by enhancing and supporting photosynthetic carbon gain (Xiong et al., 2019). The identification of precipitation seasonality (and other three additional precipitation-related variables) in the model that best explained the geographic variation in genetic diversity of A. germinans highlights its importance as a component of niche suitability.
The population genetics theory predicts that genetic diversity in a population results from a complex interplay between different evolutionary forces that depends on its effective population size (Kimura, 1983). We found solid non-linear relationships between genetic diversity and niche-centroid distance. Non-linear relationships between abundance and distance to the niche centroid imply that optimal niche conditions are relatively narrow (cf., Rabinowitz, 1981). Thus, few sites hold suitable conditions for maintaining large populations (Martínez-Meyer et al., 2013). Our data in A. germinans suggest this pattern. Populations mainly located along the coasts of the Caribbean Sea (v.gr., Central America, Puerto Rico, Trinidad, and French Guiana) bore high genetic diversity and larger effective population size. However, island populations may maintain high genetic diversity owing to high gene flow and show genetic differentiation from continental coasts due to ocean currents (see Cerón-Souza et al., 2015). Populations of A. germinans located near the niche centroid are expected to deal with changing environments, owing to higher genetic variability, and thus might be more likely to survive and produce offspring. Nevertheless, this could depend on whether species differ in distributional range, life-history traits, or evolutionary history. Thus, eco-geographic and historical circumstances can have different demographic consequences across the species range (Griffin and Willi, 2014).
Many woody plants have high heterozygosity throughout their populations (Hamrick and Godt, 1996). They have long generation times and, in many cases, have extensive gene flow over large geographic distances through both pollen and seeds (Petit and Hampe, 2006). Studies have shown that life-history traits such as life form and dispersal capacity influence patterns of genetic variation (Hamrick and Godt, 1996). In many cases, high gene flow rates can erase patterns of genetic diversity. Assessment of the correlation between habitat suitability and abundance has shown that dispersal capability also influences the strength of this correlation (Osorio-Olvera et al., 2016). Eventually, increasing gene flow rates can blur the relationship between DNC and abundance. Therefore, we would expect a similar result for genetic diversity patterns. Hence, detecting the relationship between DNC and genetic diversity in woody plants may be difficult; however, our results support the notion that A. germinans (and, in general, mangroves) are an ideal model to test the niche-centroid hypothesis (Kennedy et al., 2020).
The distance to the ecological niche centroid is a powerful tool to infer the role of some critical variables in population dynamics, especially when limited information is available. Since environmental conditions are not stable over time, the geographic ranges of many species have changed in concert with habitat suitability, resulting in geographic variation in effective population size; and the long-term estimates of heterozygosity are useful to track those changes. Furthermore, because losses of rare alleles occur during founder events, population bottlenecks, and sporadic changes in effective population size, we recommend targeting the analysis of allelic diversity in future studies to increase the likelihood to identify this geographical pattern (Eckert et al., 2008), especially for species with long generation times and high gene flow rates.
Levels of genetic diversity vary significantly in natural populations and species. Determinants of this variation are still controversial because many confounding factors affect genetic polymorphism, such as mutation rate, mating system, gene flow rates, population bottlenecks, environmental perturbations, and population size (Petit and Hampe, 2006; Romiguier et al., 2014). Linear regression analyses showed that our predicted models of observed and expected heterozygosity indicated considerably explanatory power of observed values (R2= 0.28 for Ho; R2 = 0.25 for He; P < 0.05), similar to other related studies (Martínez-Meyer et al., 2013; Lira-Noriega and Manthey, 2014). Thus, correlation analysis between DNC and genetic variation is helpful to predict the spatial variation of heterozygosity throughout most of its natural geographic distribution. In the literature, some more complex models allow prediction of current (and future) genetic diversity, population size, and connectivity among populations (e.g., Benoliel-Carvalho et al., 2019; Hearn et al., 2019). Here, we may be able to predict spatial heterozygosity using a simple approach with a reasonable certainty rate.
Nowadays, various genetic diversity estimates can be applied, and the implementation of population genomics may further improve the prediction of population genetic diversity. These results are relevant to plant conservation and evolutionary biology because evolutionary potential is primarily a function of genetic variation (Reed and Frankham, 2003; Wee et al., 2018). Modelling such genetic parameters provides a helpful way to support and envisage management plans. Thus, this is a valuable tool for conservation and management efforts. The current niche models, coupled with general circulation models, could project likely future changes in genetic diversity (while recognizing the effects of uncertainties and assumptions on niche modelling; Wiens et al., 2009).
There are many predictions for mangrove forests in the face of climate change (Record et al., 2013; Alongi, 2015; Cerón-Souza et al., 2015). Given the multiple ecosystem services they provide, it is imperative to develop models that can anticipate the genetic diversity changes for more accurate information about which places are likely to be most at risk, in order to inform decision-making by conservationists and resource managers.
In conclusion, this study supports that black mangrove genetic diversity meets the niche-centroid hypothesis, in which the highest heterozygosity and allelic richness (the basic genetic units for adaptation) was observed at locations of high environmental suitability. Our findings contribute to understanding how habitat quality may affect the demography of populations of one of the most conspicuous mangrove species in the Americas. The strong correlation between heterozygosity and allelic richness estimates and DNC illustrates how the environmental variables that define the fundamental niche impact individuals' establishment, growth, development, and survival. Soil features and precipitation are related to the population dynamics of A. germinans, explaining, in part, extant genetic variation. Habitat suitability of A. germinans is spatially structured with few populations containing high genetic diversity, mainly located along the Caribbean coast. The ability to predict the amount of genetic variation across the natural range of a species and model future changes in the face of climate change are potent tools in species conservation and management efforts, especially in defining conservation units (Wee et al., 2018).
The datasets analysed for this study can be found in the DRYAD REPOSITORY [https://doi.org/10.5061/dryad.4r0q7m5] and Supplementary Material.
JN-F, MO-Z, and LO-O designed and conceptualized the project. JN-F, MO-Z, VJ-L, IC-S, and ER-O collected the samples and performed genetic analyses. MO-Z and LO-O performed niche modelling and interpreted the data and wrote the first draft of the manuscript. All authors contributed critically to the drafts and approved the final version of the manuscript for publication.
This work was supported by the National Commission for the Knowledge and Use of Biodiversity (CONABIO), Grant No: KE008 to JN-F; and NSF-AGEP Pre-doctoral Fellowship (HRD-0302696) to ER-O; and NSF-AGEP Pre-doctoral Fellowship (HRD-0302696) to ER-O.
The authors declare that the research was conducted in the absence of any commercial or financial relationships that could be construed as a potential conflict of interest.
All claims expressed in this article are solely those of the authors and do not necessarily represent those of their affiliated organizations, or those of the publisher, the editors and the reviewers. Any product that may be evaluated in this article, or claim that may be made by its manufacturer, is not guaranteed or endorsed by the publisher.
The authors thank the members of the Laboratorio de Genética Ecológica y Evolución. MO-Z, acknowledges the fellowship granted for graduate studies by the National Council of Science and Technology (CONACyT) and the Graduate Program in Biological Sciences at the National Autonomous University of Mexico (UNAM). MO-Z also acknowledges the postdoctoral fellowship granted from the Dirección General de Asuntos del Personal Académico (DGAPA), UNAM. I.C.S. thanks the University of Puerto Rico (Rio Piedras campus) and the Smithsonian Tropical Research Institute for their support in collecting and analysing the genetic data provided for this study. ER-O thanks the University of Puerto Rico (Rio Piedras Campus), and the U.S. EPA-Science to Achieve Results (STAR) program (Grant # U915985). LO-O acknowledges CONACyT for a postdoctoral fellowship (2019-000012-01 EXTV-00046; CVU 368747) and Programa de Apoyo a Proyectos de Investigación e Innovación Tecnológica of the UNAM (IA203922).
The Supplementary Material for this article can be found online at: https://www.frontiersin.org/articles/10.3389/fcosc.2021.795365/full#supplementary-material
Abeli, T., Gentili, R., Mondoni, A., Orsenigo, S., and Rossi, G. (2014). Effects of marginality on plant population performance. J. Biogeogr. 41, 239–249. doi: 10.1111/jbi.12215
Alongi, D. M. (2014). Carbon cycling and storage in mangrove forests. Annual Rev. Marine Sci. 6, 195–219. doi: 10.1146/annurev-marine-010213-135020
Alongi, D. M. (2015). The impact of climate change on mangrove forest. Curr. Climate Change Rep. 1, 30–39. doi: 10.1007/s40641-015-0002-x
Alongi, D. M. (2018). “Climate regulation by capturing carbon in mangroves,” in The Wetland Book: I: Structure and Function, Management, and Methods, eds C. M. Finlayson, M. Everard, K. Irvine, R. J. McInnes, B. A. Middleton, A. A. van Dam, and N. C. Davidson (Dordrecht: Springer), 1213–1219. doi: 10.1007/978-90-481-9659-3_236
Altamiranda-Saavedra, M., Osorio-Olvera, L., Yáñez-Arenas, C., Marín-Ortiz, J. C., and Parra-Henao, G. (2020). Geographic abundance patterns explained by niche centrality hypothesis in two Chagas disease vectors in Latin America. PLoS ONE. 15:e241710. doi: 10.1371/journal.pone.0241710
Arreola-Lizárraga, J. A., Flores-Verdugo, F. J., and Ortega-Rubio, A. (2004). Structure and litter fall of an arid mangrove stand on the Gulf of California, Mexico. Aquatic Botany 79, 137–143. doi: 10.1016/j.aquabot.2004.01.012
Assis, J., Tyberghein, L., Bosh, S., Verbruggen, H., Serrão, E. A., and De Clerck, O. (2017). Bio-ORACLE v2.0: extending marine data layers for bioclimatic modelling. Global Ecol. Biogeogr. 27, 277–284. doi: 10.1111/geb.12693
Barve, N., Barve, V., Jiménez-Valverde, A., Lira-Noriega, A., Maher, S. P., Peterson, T., et al. (2011). The crucial role of the accessible area in ecological niche modeling and species distribution modeling. Ecol. Model. 222, 1810–1819. doi: 10.1016/j.ecolmodel.2011.02.011
Benoliel-Carvalho, S., Torres, J., Tarroso, P., and Velo-Antón, G. (2019). Genes on the egde: a framework to detect genetic diversity imperiled by climate change. Global Change Biol. 25, 4034–4047. doi: 10.1111/gcb.14740
Blanco-Canqui, H., and Lal, R. (2004). Mechanisms of carbon sequestration in soil aggregates. Critical Rev. Plant Sci. 23, 481–504. doi: 10.1080/07352680490886842
Brown, J. H. (1984). On the relationship between abundance and distribution of species. Am. Natural. 124, 255–279.
Cavanaugh, K. C., Kellner, J. R., Forde, A. J., Gruner, D. S., Parker, J. D., Rodriguez, W., et al. (2014). Poleward expansion of mangroves is a threshold response to decreased frequency of extreme cold events. Proc. Natl. Acad. Sci. U.S.A. 111, 723–727. doi: 10.1073/pnas.1315800111
Cerón-Souza, I., Gonzalez, E. G., Schwarzbach, A. E., Salas-Leiva, D. E., Rivera-Ocasio, E., Toro-Perea, N., et al. (2015). Contrasting demographic history and gene flow patterns of two mangrove species on either side of the Central American Isthmus. Ecol. Evol. 5, 3486–3499. doi: 10.1002/ece3.1569
Clough, B. F. (1992). “Primary productivity and growth of mangroves forest,” in Tropical Mangrove Ecosystem, eds A. I. Robertson and D. M. Alongi. (Washington, DC: American Geophysical Union), 225–249. doi: 10.1029/CE041p0225
Costanza, R., d'Arge, R., de Groot, R., Farber, S., Grasso, M., Hannon, B., et al. (1997). The value of the world's ecosystem services and natural capital. Nature 387, 253–260. doi: 10.1038/387253a0
Crase, B., Liedloft, A., Vest, P. A., Burgman, M. A., and Wintle, B. A. (2013). Hydroperiod is the main driver of the spatial pattern of the dominance in mangrove communities. Global Ecol. Biogeogr. 22, 806–817. doi: 10.1111/geb.12063
Dallas, T., Decker, R. R., and Hastings, A. (2017). Species are not most abundant in the centre of their geographic range or climatic niche. Ecol. Lett. 20, 1526–1533. doi: 10.1111/ele.12860
Diniz-Filho, J. A., Nabout, J. C., Bini, L. M., Soares, T. N., Pires de Campos Telles, M., de Marco, P., et al. (2009). Niche modelling and landscape genetics of Caryocar Brasiliense (“Pequi” tree: Caryocaraceae) in Brazilian Cerrado: an integrative approach for evaluating central-peripheral population patterns. Tree Genet. Genomes 5, 617–627. doi: 10.1007/s11295-009-0214-0
Diniz-Filho, J. A., Rodrigues, H., Pires de Campos Telles, M., de Oliveira, G., Terribile, L. C., Soares, T. N., et al. (2015). Correlation between genetic diversity and environmental suitability: taking uncertainty from ecological niche models into account. Mol. Ecol. Resources 15, 1059–1066. doi: 10.1111/1755-0998.12374
Diop, E. S., Soumare, A., Diallo, N., and Guisse, A. (1997). Recent changes of mangroves of the Saloum River Estuary, Senegal. Mangroves Salt Marshes 1, 163–172. doi: 10.1023/A:1009900724172
Donato, D. C., Kauffman, J. B., Murdiyarso, D., Kurnianto, S., Stidham, M., and Kanninen, M. (2011). Mangroves among the most carbon-rich forests i the Tropics. Nat. Geosci. 4, 293–297. doi: 10.1038/ngeo1123
Duke, N. C. (2017). “Mangrove Floristics and Biogeography Revisited: Further Deductions from Biodiversity Hot Spots, Ancestral Discontinuities, and Common Evolutionary Processes,” in Mangrove Ecosystems: A Global Biogeographic Perspective, eds V. H. Rivera-Monroy, S. Y. Lee, E. Kristensen, and R. R. Twilley (Cham: Springer International Publishing AG), 17–53. doi: 10.1007/978-3-319-62206-4_2
Duke, N. C., Ball, M. C., and Ellison, J. C. (1998). Factors influencing biodiversity and distributional gradients in mangroves. Global Ecol. Biogeogr. Lett. 7, 27–47. doi: 10.2307/2997695
Eckert, C. G., Samis, E., and Lougheed, S. C. (2008). Genetic variation across species' geographical ranges: the central-marginal hypothesis and beyond. Mol. Ecol. 17, 1170–1188. doi: 10.1111/j.1365-294X.2007.03659.x
Ellegren, H. (2000). Heterogeneous mutation processes in human microsatellite DNA sequences. Nat. Genet. 24, 400–402. doi: 10.1038/74249
Ellegren, H., and Galtier, N. (2016). Determinants of genetic diversity. Nat. Rev. Genet. 17, 422–433. doi: 10.1038/nrg.2016.58
Excoffier, L., and Lischer, H. E. L. (2010). Arlequin suite ver 3.5: a new series of programs to perform population genetics analyses under Linux and Windows. Mol. Ecol. Resources 10, 564–567. doi: 10.1111/j.1755-0998.2010.02847.x
Feng, W. T., Plante, A. F., and Six, J. (2013). Improving estimates of maximal organic carbon stabilization by fine soil particles. Biogeochemistry 112, 81–93. doi: 10.1007/s10533-011-9679-7
Frankham, R. (1996). Relationship of genetic variation to population size in wildlife. Conservat. Biol. 10, 1500–1506.
Frankham, R. (2005). Genetics and extinction. Conserv. Biol. 126, 131–140. doi: 10.1016/j.biocon.2005.05.002
Frankham, R. (2012). How closely does genetic diversity in finite populations conform to predictions of neutral theory? Large deficits in regions of low recombination. Heredity 108, 167–178. doi: 10.1038/hdy.2011.66
Giri, C., Ochieng, E., Tieszen, L. L., Zhu, Z., Singh, A., Loveland, T., et al. (2011). Status and distribution of mangrove forests of the world using earth observation satellite data. Global Ecol. Biogeogr. 20, 154–159. doi: 10.1111/j.1466-8238.2010.00584.x
Gonçalves, D. R. P., de Moraes, S.á, J. C., Mishra, U., Cerri, C. E. P., Ferreira, L. A., and Furlan, F. J. F. (2017). Soil type and texture impacts on soil organic carbon storage in a sub-tropical agro-ecosystem. Geoderma 286, 87–97. doi: 10.1016/j.geoderma.2016.10.021
Goudet, J., and Jombart, T. (2015). HIERFSTAT: Estimation and Tests of Hierarchical F-Statistics. R package version 0.04-22. Available online at: https://CRAN.R-project.org/package=hierfstat
Griffin, P. C., and Willi, Y. (2014). Evolutionary shifts to self-fertilization restricted to geographic range margins in North American Arabidopsis lyrata. Ecol. Lett. 17, 484–490. doi: 10.1111/ele.12248
Gruba, P., Socha, J., Błońska, E., and Lasota, J. (2015). Effect of variable soil texture, metal saturation of soil organic matter (SOM) and tree species composition on spatial distribution of SOM in forest soils in Poland. Sci. Total Environ. 521–522, 90–100. doi: 10.1016/j.scitotenv.2015.03.100
Guo, W., Banerjee, A. K., Ng, W. L., Yuan, Y., Li, W., and Huang, Y. (2020). Chloroplast DNA phylogeography of the Holly mangrove Acanthus ilicifolius in the Indo-West Pacific. Hydrobiologia 847, 3591–3608. doi: 10.1007/s10750-020-04372-1
Hamrick, J. L., and Godt, J. W. (1996). Effects of life history traits on genetic diversity in plant species. Philosophical Transactions R. Soc. B. 351, 1291–1298. doi: 10.1098/rstb.1996.0112
Hearn, A. J., Cushman, S. A., Goossens, B., Ross, J., Ewan, A. M., Hunter, L. T. B., et al. (2019). Predicting connectivity, population size and genetic diversity of Sunda clouded leopards across Sabah, Borneo. Landscape Ecol. 34, 275–290. doi: 10.1007/s10980-018-0758-1
Hendry, A. P., Kinnison, M. T., Heino, M., Day, T., Smith, T. B., Fitt, G., et al. (2011). Evolutionary principles and their practical application. Evolut. Applicat. 4, 159–183. doi: 10.1111/j.1752-4571.2010.00165.x
Hengl, T., de Jesus, J. M., MacMillan, R. A., Batjes, N. H., Heuvelink, G. B. M., Ribeiro, E., et al. (2014). SoilGrids1km - Global Soil Information Based on Automated Mapping. PLoS ONE 9:e105992. doi: 10.1371/journal.pone.0105992
Hijmans, R. J., Cameron, S. E., Parra, J. L., Jones, P. G., and Jarvis, A. (2005). Very high resolution interpolated climates surfaces for global land areas. Int. J. Climatol. 25, 1965–1978. doi: 10.1002/joc.1276
Hoffmann, A. A., and Sgro, C. M. (2011). Climate change and evolutionary adaptation. Nature. 470, 479–485, doi: 10.1038/nature09670
Hossain, M. D., and Nuruddin, A. A. (2016). Soil and mangrove: a review. J. Environ. Sci. Tech. 9, 198–207. doi: 10.3923/jest.2016.198.207
Hutchinson, G. E. (1957). Concluding remarks. Cold Spring Harbor Symposia on Quant. Biol. 22, 415–427. doi: 10.1101/SQB.1957.022.01.039
Hutchinson, J., Manica, A., Swetnam, R., Balmford, A., and Spalding, M. (2014). Predicting global patterns in mangrove forest biomass. Conserv. Lett. 7, 233–240. doi: 10.1111/conl.12060
IUSS Working Group WRB (2007). World Reference Base for Soil Resources: A Framework for International Classification, Correlation and Communication. World Soil Resources Reports No. 103. FAO, Rome. 128.
Jardine, S. L., and Siikamäki, J. V. (2014). A global predictive model of carbon in mangrove soils. Environ. Res. Lett. 9:104013. doi: 10.1088/1748-9326/9/10/104013
Jiménez, L., Soberón, J., Christen, J. A., and Soto, D. (2019). On the problem of modeling a fundamental niche from occurrence data. Ecol. Modell. 397, 74–83. doi: 10.1016/j.ecolmodel.2019.01.020
Jobbágy, E. G., and Jackson, R. B. (2000). The vertical distribution of soil organic carbon and its relation to climate and vegetation. Ecol. Appl. 10, 423–236. doi: 10.1890/1051-0761(2000)010[0423:TVDOSO]2.0.CO;2
Jordan, H. D. (1964). The relation of the vegetation and soil to development of mangroves swamps for rice growing in Sierra Leone. J. Appl. Ecol. 1, 209–212. doi: 10.2307/2401600
Kennedy, J. P., Preziosi, R. F., Rowntree, J. K., and Feller, I. C. (2020). Is the central-marginal hypothesis a general rule? Evidence from three distributions of an expanding mangrove species, Avicennia germinans (L.) L. Mol. Ecol. 29, 704–719. doi: 10.1111/mec.15365
Kimura, M. (1983). The Neutral Theory of Molecular Evolution. Cambridge: Cambridge University Press. 367 p. doi: 10.1017/CBO9780511623486
Krauss, K. W., Lovelock, C. E., McKee, K. L., Lopez-Hoffman, L., Ewe, S. M. L., and Sousa, W. P. (2008). Environmental drivers in mangrove establishment and early development: a review. Aquatic Botany 89, 105–127. doi: 10.1016/j.aquabot.2007.12.014
Lange, M., Eisenhauer, N., Sierra, C. A., Besser, H., Engels, C., Griffiths, R. I., et al. (2015). Plant diversity increases soil microbial activity and soil carbon storage. Nat. Commun. 6:6707. doi: 10.1038/ncomms7707
Li, Q., Qi, F., and Luxin, Z. (2010). Study of the height growth dynamic based on tree-ring data in Populus euphratica from the lower reach of the Heihe River, China. Dendrochronologia 28, 49–64. doi: 10.1016/j.dendro.2009.03.004
Lira-Noriega, A., and Manthey, J. D. (2014). Relationship of genetic diversity and niche centrality: a survey and analysis. Evolution 68, 1082–1093. doi: 10.1111/evo.12343
Maguire, B. (1973). Niche response structure and the analytical potentials of its relationship to the habitat. Am. Natural. 107, 213–246.
Martínez-Meyer, E., Díaz-Porras, D. F., Peterson, A. T., and Yañez-Arenas, C. (2013). Ecological niche structure and rangewide abundance patterns of species. Biol. Lett. 9:20120637. doi: 10.1098/rsbl.2012.0637
Matsui, N., Meepol, W., and Chukwamdee, J. (2015). Soil organic carbon in mangrove ecosystems with different vegetation and sedimentological conditions. J. Marine Sci. Eng. 3, 1404–1424. doi: 10.3390/jmse3041404
McMillan, C. (1975). “Interaction of soil texture with salinity tolerences of black mangrove (Avicennia) and white mangrove (Laguncularia) from North America,” in Proceedings of the International Symposium on the Biology and Management of Mangroves, eds G. E.Walsh, S. C. Snedaker, and H. J. Teas (Gainesville: University of Florida), 561–566.
Méndez-Alonzo, R., and López-Portillo, J. (2008). Latitudinal variation in leaf and tree traits of the mangrove Avicennia germinans (Avicenniaceae) in the central region of the Gulf of Mexico. Biotropica 40, 449–456. doi: 10.1111/j.1744-7429.2008.00397.x
Micheletti, S. J., and Storfer, A. (2015). A test of the central-marginal hypothesis using population genetics and ecological niche modelling in an endemic salamander (Ambystoma barbourin). Mol. Ecol. 24, 967–979. doi: 10.1111/mec.13083
Mori, G. M., Zucchi, M. I., and Souza, A. P. (2015). Multiple-geographic-scale genetic structure of two mangrove tree species: the roles of Mating system, hybridization, limited dispersal and extrinsic factors. PLoS ONE 10:e0118710. doi: 10.1371/journal.pone.0118710
Nettel, A., and Dodd, R. S. (2007). Drifting propagules and receding swamps: genetic footprints of mangrove recolonization and dispersal along tropical coasts. Evolution 61, 958–971. doi: 10.1111/j.1558-5646.2007.00070.x
Ochoa-Zavala, M., Jaramillo-Correa, J. P., Piñero, D., Nettel-Hernanz, A., and Núñez-Farfán, J. (2019). Data from: contrasting colonization patterns of black mangrove (Avicennia germinans (L.) L.) gene pools along the Mexican coasts. Dryad Digital Repository doi: 10.5601/dryad.4r0q7m5
Osorio-Olvera, L., Falconi, M., and Soberón, J. (2016). Sobre la relación entre idoneidad del hábitat y la abundancia poblacional bajo diferentes escenarios de dispersión. Revista Mexicana de Biodiversidad 87, 1080–1088. doi: 10.1016/j.rmb.2016.07.001
Osorio-Olvera, L., Lira-Noriega, A., Soberón, J., Peterson, A. T., Falconi, M., Contreras-Díaz, R. G., et al. (2020b). ntbox: an r package with graphical user interface for modelling and evaluating multidimensional ecological niches. Methods Ecol Evol. 11, 1199–1206. doi: 10.1111/2041-210X.13452
Osorio-Olvera, L., Soberón, J., and Falconi, M. (2019). On population abundance and niche structure. Ecography 42, 1415–1425. doi: 10.1111/ecog.04442
Osorio-Olvera, L., Yañez-Arenas, C., Martínez-Meyer, E., and Peterson, A. T. (2020a). Relationships between population densities and niche-centroid distances in North American birds. Ecol. Lett. 23, 555–564. doi: 10.1111/ele.13453
Pemberton, T. J., Sandefur, C. I., Jakobsson, M., and Rosenberg, N. A. (2009). Sequence determinants of human microsatellite variability. BMC Genomics 10, 612, doi: 10.1186/1471-2164-10-612
Petit, R. J., and Hampe, A. (2006). Some evolutionary consequences of being a tree. Annual Rev. Ecol. Evol. Systemat. 37, 187–214. doi: 10.1146/annurev.ecolsys.37.091305.110215
Pfeifer, M., Schatz, B., Picó, F. X., Passalacqua, N. G., Fay, M. F., Carey, P. D., et al. (2009). Phylogeography and genetic structure of the orchid Himantoglossum hircinum (L.) Spreng. across its European central-marginal gradient. J. Biogeogra. 36, 2353–2365. doi: 10.1111/j.1365-2699.2009.02168.x
Pironon, S., Villellas, J., Morris, W. F., Doak, D. F., and García, M. B. (2015). Do geographic, climatic or historical ranges differentiate the performance of central versus peripheral populations? Global Ecol. Biogeogr. 24, 611–620. doi: 10.1111/geb.12263
Quisthoudt, K., Schmitz, N., Randin, C. F., Dahdouh-Guebas, F., Robert, E. M. R., and Koedam, N. (2012). Temperature variation among mangrove latitudinal range limits worldwide. Trees 26, 1919–1931. doi: 10.1007/s00468-012-0760-1
R Core Team (2019). R: A Language and Environment for Statistical Computing. Available Online at: https://www.r-project.org
Rabinowitz, D. (1981). “Seven forms of rarity,” in The Biological Aspects of Rare Plant Conservation, ed H. Synge (New York, NY: John Wiley and Sons Ltd.), 205–217.
Rahman, M. S., Donoghue, D. N. M., and Bracken, L. J. (2021). Is soil organic carbon understated in the largest mangrove forest ecosystems? Evidence from the Bangladesh Sundarbans. Catena 200l:105159. doi: 10.1016/j.catena.2021.105159
Record, S., Charney, N. D., Zakaria, R. M., and Ellison, A. M. (2013). Projecting global mangrove species and community distributions under climate change. Ecosphere 4, 1–23. doi: 10.1890/ES12-00296.1
Reed, D. H., and Frankham, R. (2003). Correlation between fitness and genetic diversity. Conservat. Biol. 17, 230–237. doi: 10.1046/j.1523-1739.2003.01236.x
Rodríguez-Medina, K., Yañez-Arenas, C., Peterson, A. T., Euán Ávila, J., and Herrera-Silveira, J. (2020). Evaluating the capacity of species distribution modeling to predict the geographic distribution of the mangrove community in Mexico. PLoS ONE 15:e0237701. doi: 10.1371/journal.pone.0237701
Romiguier, J., Gayral, P., Ballenghien, M., Bernard, A., Cahais, V., Chenuil, Y., et al. (2014). Comparative population genomics in animals uncovers the determinants of genetic diversity. Nature 515, 261–263. doi: 10.1038/nature13685
Royston, P. (1995). Remark AS R94: A remark on algorithm AS 181: The W-test for Normality. J. R. Stat. Soc. Ser. C. 44, 547–551. Available Online at: https://www.jstor.org/stable/2986146
Saiz, G., Bird, M. I., Domingues, T., Schrodt, F., Schwarz, M., Feldpausch, T. R., et al. (2012). Variation in soil carbon stocks and their determinants across a precipitation gradient in West Africa. Glob. Chang. Biol. 18, 1670–1683. doi: 10.1111/j.1365-2486.2012.02657.x
Santini, L., Pironon, S., Maiorano, L., and Thuiller, W. (2018). Addressing common pitfalls does not provide more support to geographical and ecological abundance-centre hypotheses. Ecography 42, 696–705. doi: 10.1111/ecog.04027
Smith, G. D. (1986). The Guy Smith Interviews: Rationale for Concepts in Soil Taxonomy. Department of Agronomy, Washington: New York State College of Agriculture and Life Science Cornell University. Washington, DC. 259.
Soil Survey staff (2010). Keys to Soil Taxonomy. 11 edition. United States Department of Agriculture, Natural Resources Conservation Service. Washington, DC. 346pp.
Spielman, D., Brook, B. W., and Frankham, R. (2004). Most species are not driven to extinction before genetic factors impact them. PNAS 101, 15261–15264. doi: 10.1073/pnas.0403809101
Tomlinson, P. B. (2016). The Botany of Mangroves. Cambridge: Cambridge University Press. 418. doi: 10.1017/CBO9781139946575
Tyberghein, L., Verbruggen, H., Pauly, K., Troupin, C., Mineur, F., and De Clerck, O. (2012). Bio-ORACLE: a global environmental dataset for marine species distribution modelling. Global Ecol. Biogeogr. 21, 272–281. doi: 10.1111/j.1466-8238.2011.00656.x
Ukpong, I. E. (1997). Vegetation and its relation to soil nutrient and salinity in the Calabar mangrove swamp, Nigeria. Mangroves Salt Marshes 1, 211–218. doi: 10.1023/A:1009952700317
Väli, U., Einarsson, A., Waits, L., and Ellegren, H. (2008). To what extent do microsatellite markers reflect genome-wide genetic diversity in natural populations? Mol. Ecol. 17, 3808–3817, doi: 10.1111/j.1365-294X.2008.03876.x
Wee, A. K. S., Mori, G. M., Lira-Medeiros, C. F., Núñez-Farfán, J., Takayama, K., Faulks, L., et al. (2018). The integration and application of genomic information in mangrove conservation. Conserv. Biol. 33, 206–209. doi: 10.1111/cobi.13140
Wiens, J. A., Stralberg, D., Jongsomijit, D., Howell, C. A., and Snyder, M. A. (2009). Niches, model, and climate change: Assessing the assumptions and uncertainties. PNAS 106, 19729–19736. doi: 10.1073/pnas.0901639106
Wiesmeier, M., Barthold, F., Blank, B., and Kogel-Knabner, I. (2011). Digital mapping of soil organic matter stocks using Random Forest modeling in a semi-arid steppe ecosystem. Plant Soil. 340, 7–24. doi: 10.1007/s11104-010-0425-z
Woodroffe, C. (1992). “Mangroves sediments and geomorphology,” in Tropical Mangrove Ecosystem, eds A. I. Robertson and D. M. Alongi (Washington, DC: American Geophysical Union), 7–41. doi: 10.1029/CE041p0007
Woodroffe, C. D., and Grindrod, J. (1991). Mangrove biogeography-the role of quaternary environmental and sea-level change. J. Biogeogr. 18, 479–492. doi: 10.2307/2845685
Woodward, F. I., and Williams, B. G. (1987). Climate and plant distribution at global and local scales. Vegetatio 69, 189–197. doi: 10.1007/BF00038700
Ximenes, A. C., Ponsoni, L., Lira, C. F., Koedam, N., and Dahdouh-Guebas, F. (2018). Does sea surface temperature contribute to determining range limits and expansion of mangroves in Eastern south America (Brazil)? Remote Sensing 10:1787. doi: 10.3390/rs10111787
Xiong, Y., Cakir, R., Phan, S. M., Ola, A., Krauss, K. W., and Lovelock, C. E. (2019). Global patterns of tree stem growth and stand aboveground wood production in mangrove forests. Forest Ecol. Manage. 444, 382–392. doi: 10.1016/j.foreco.2019.04.045
Yañez-Arenas, C., Martínez-Meyer, E., Mandujano, S., and Rojas-Soto, O. (2012). Modelling geographic patterns of population density of the white-tailed deer in central Mexico by implementing ecological niche theory. Oikos 121, 2081–2089. doi: 10.1111/j.1600-0706.2012.20350.x
Keywords: Avicennia germinans, genetic variation, niche-centroid distance, precipitation, soil texture, soil types
Citation: Ochoa-Zavala M, Osorio-Olvera L, Cerón-Souza I, Rivera-Ocasio E, Jiménez-Lobato V and Núñez-Farfán J (2022) Reduction of Genetic Variation When Far From the Niche Centroid: Prediction for Mangrove Species. Front. Conserv. Sci. 2:795365. doi: 10.3389/fcosc.2021.795365
Received: 15 October 2021; Accepted: 20 December 2021;
Published: 26 January 2022.
Edited by:
Gustavo Maruyama Mori, São Paulo State University, BrazilReviewed by:
Ludwig Triest, Vrije University Brussel, BelgiumCopyright © 2022 Ochoa-Zavala, Osorio-Olvera, Cerón-Souza, Rivera-Ocasio, Jiménez-Lobato and Núñez-Farfán. This is an open-access article distributed under the terms of the Creative Commons Attribution License (CC BY). The use, distribution or reproduction in other forums is permitted, provided the original author(s) and the copyright owner(s) are credited and that the original publication in this journal is cited, in accordance with accepted academic practice. No use, distribution or reproduction is permitted which does not comply with these terms.
*Correspondence: Juan Núñez-Farfán, ZmFyZmFuQHVuYW0ubXg=
†Present addresses: Maried Ochoa-Zavala, Laboratorio de Genética de Organismos Acuáticos, Instituto de Ciencias del Mar y Limnología, Universidad Nacional Autónoma de Mexico, Mexico City, Mexico
Vania Jiménez-Lobato, Laboratorio Nacional de Análisis y Síntesis Ecológica, Escuela de Desarrollo Sustentable, Campus Costa Grande, Universidad Autónoma de Guerrero, Cátedras CONACyT, Chilpancingo, Mexico
‡These authors have contributed equally to this work and share first authorship
Disclaimer: All claims expressed in this article are solely those of the authors and do not necessarily represent those of their affiliated organizations, or those of the publisher, the editors and the reviewers. Any product that may be evaluated in this article or claim that may be made by its manufacturer is not guaranteed or endorsed by the publisher.
Research integrity at Frontiers
Learn more about the work of our research integrity team to safeguard the quality of each article we publish.