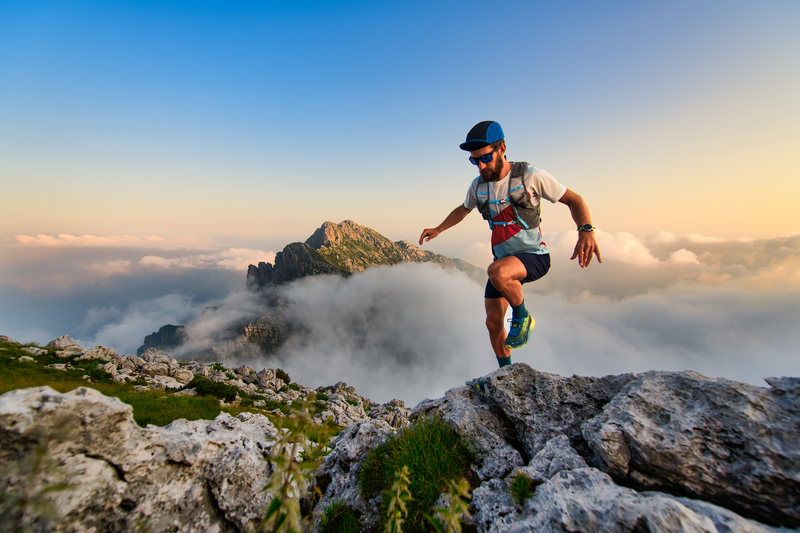
95% of researchers rate our articles as excellent or good
Learn more about the work of our research integrity team to safeguard the quality of each article we publish.
Find out more
EDITORIAL article
Front. Comput. Neurosci. , 31 July 2023
Volume 17 - 2023 | https://doi.org/10.3389/fncom.2023.1249197
This article is part of the Research Topic Psychological and Cognitive Evaluation and Intervention Based on Physiological and Behavioral Computing View all 9 articles
Editorial on the Research Topic
Psychological and cognitive evaluation and intervention based on physiological and behavioral computing
Physiological and behavioral computing is a tool for evaluating mental states and cognitive processes. However, traditional approaches to psychological and cognitive research using physiological and behavioral data have faced several challenges. With the advent of advanced technology, it is now possible to design more effective paradigms using virtual reality, gaming, and new scales, among others. Wearable and non-contact technologies make it possible to monitor multiple physiological and behavioral signals, facilitating the establishment of psychological and cognitive evaluation models with better generalization performance.
This Research Topic aims to summarize the foundations and technologies of physiological and behavioral computing for psychological and cognitive evaluation and intervention. It includes task design, cognitive assessment using virtual reality, gaming, language, and new scales, among others. Additionally, the topic covers the acquisition and processing of physiological and behavioral data, psychological calculation based on physiological signals, as well as cognitive evaluation methods with multimodal signal fusion.
The Research Topic presents eight articles. These papers showcase the potential of physiological and behavioral computing for psychological evaluation and intervention, highlighting the importance of combining information from multiple modalities to improve accuracy and effectiveness.
Chen et al. proposes a novel approach to emotion recognition using multi-modal physiological signals, and the results demonstrate the effectiveness of the proposed 1D convolutional neural network-based method compared to traditional machine learning approaches.
Chung examines the relationship between users' claustrophobic tendencies and their need for space in digital interfaces. The association of stronger claustrophobic tendencies toward physical space and stronger need for digital space was reported in two studies. Such tendencies are likely to influence cognitive tasks on digital devices more than device-related spatial needs. This study provides valuable insights on users' spatial needs in fast-changing digital environments.
Dai et al. investigates the effects on executive vigilance when transcranial direct current stimulation (tDCS) is applied over the left dorsolateral prefrontal cortex (DLPFC). The findings suggest that anodal tDCS over left DLPFC enhances the early stage of relevant information processing and the inhibitory control of distracting stimuli during continuous and monotonous vigilance task.
Fordson et al. proposes a deep learning model for classifying emotional responses based on electrodermal activity signals. The model incorporates knowledge graphs as a way to learn representations from the data, as well as gender and age information and time and frequency EDA features. The authors introduce a weighted feature fusion method to combine knowledge embedding feature vectors and statistical feature vectors for emotional state classification. The results show a significant improvement in the performance of the emotion recognition system, demonstrating the potential of physiological and behavioral computing for psychological evaluation and intervention.
The study in the paper (Vargas et al.) presents a novel use of virtual reality, machine learning and implicit measures for assessment of empathy. Participants were immersed in virtual environments representing social workplace scenarios while their eye-gaze patterns and behaviors were recorded. The findings suggest that the combination of eye-gaze patterns and behaviors during virtual reality experiences may differentiate between empathy dimensions.
The relationship between trait anxiety and attentional bias is unclear. Xing et al. explored the impact of trait anxiety on attentional bias toward emotional stimuli using materials from the International Affective Picture System. Participants with high trait anxiety showed greater avoidance of threatening stimuli during the early period of stimulus presentation. They also displayed a stronger attentional bias toward positive and dysphoric stimuli during the middle and late periods. Hence, trait anxiety may influence the temporal aspects of attention to emotional stimuli, providing insights into the relationship between trait anxiety and selective attention.
Zhang, Yang et al., presents an automatic schizophrenia detection algorithm based on the reading deficit of schizophrenic patients using multimodality media. The proposed algorithm utilizes abnormal speech, head movement, and reading fluency during the reading task to detect schizophrenia. The results obtained indicate that the proposed approach is efficient enough to be used for the assisted auxiliary diagnosis of schizophrenia.
Zhang, Yin et al. proposes a real-time deep learning framework to detect acute stress by fusing multimodal information, including ECG, voice, and facial expressions. The framework utilizes ResNet50 and I3D with the temporal attention module to extract stress-related information, and the matrix eigenvector-based approach to fuse multimodality information. The effectiveness of the framework was validated using the Montreal imaging stress task (MIST) experiment. This work demonstrates the potential of using deep learning technology to detect stress and the importance of combining information from multiple modalities.
In conclusion, this Research Topic presents a collection of papers that demonstrate the potential of physiological and behavioral computing for psychological evaluation and intervention. The results highlight that a thoughtful integration of information from multiple modalities can enhance the accuracy and effectiveness of psychological and cognitive assessment and intervention. It is important to note that the development of new methods and technologies for psychological and cognitive research, as well as for the diagnosis and treatment of mental health disorders, does not follow a one-size-fits-all approach.
All authors listed have made a substantial, direct, and intellectual contribution to the work and approved it for publication.
The authors declare that the research was conducted in the absence of any commercial or financial relationships that could be construed as a potential conflict of interest.
All claims expressed in this article are solely those of the authors and do not necessarily represent those of their affiliated organizations, or those of the publisher, the editors and the reviewers. Any product that may be evaluated in this article, or claim that may be made by its manufacturer, is not guaranteed or endorsed by the publisher.
Keywords: psychological computing, virtual reality, artificial intelligence, cognitive evaluation, physiological signals, behavioral data
Citation: Shu L, Perreira Da Silva M, Zhang L and Chui C-K (2023) Editorial: Psychological and cognitive evaluation and intervention based on physiological and behavioral computing. Front. Comput. Neurosci. 17:1249197. doi: 10.3389/fncom.2023.1249197
Received: 28 June 2023; Accepted: 14 July 2023;
Published: 31 July 2023.
Edited and reviewed by: Si Wu, Peking University, China
Copyright © 2023 Shu, Perreira Da Silva, Zhang and Chui. This is an open-access article distributed under the terms of the Creative Commons Attribution License (CC BY). The use, distribution or reproduction in other forums is permitted, provided the original author(s) and the copyright owner(s) are credited and that the original publication in this journal is cited, in accordance with accepted academic practice. No use, distribution or reproduction is permitted which does not comply with these terms.
*Correspondence: Chee-Kong Chui, bXBlY2NrQG51cy5lZHUuc2c=
Disclaimer: All claims expressed in this article are solely those of the authors and do not necessarily represent those of their affiliated organizations, or those of the publisher, the editors and the reviewers. Any product that may be evaluated in this article or claim that may be made by its manufacturer is not guaranteed or endorsed by the publisher.
Research integrity at Frontiers
Learn more about the work of our research integrity team to safeguard the quality of each article we publish.