- 1College of Medicine and Biological Information Engineering, Northeastern University, Shenyang, China
- 2Key Laboratory of Intelligent Computing in Medical Image, Ministry of Education, Northeastern University, Shenyang, China
- 3Department of Electrical and Computer Engineering, University of Texas at El Paso, El Paso, TX, United States
- 4Department of Radiology, The Affiliated Hospital of Guizhou Medical University, Guiyang, China
- 5Department of Otolaryngology, The Affiliated Hospital of Guizhou Medical University, Guiyang, China
- 6Department of Radiology, The Seventh Affiliated Hospital, Southern Medical University, Foshan, China
Identification of congenital sensorineural hearing loss (SNHL) and early intervention, especially by cochlear implantation (CI), are crucial for restoring hearing in patients. However, high accuracy diagnostics of SNHL and prognostic prediction of CI are lacking to date. To diagnose SNHL and predict the outcome of CI, we propose a method combining functional connections (FCs) measured by functional magnetic resonance imaging (fMRI) and machine learning. A total of 68 children with SNHL and 34 healthy controls (HC) of matched age and gender were recruited to construct classification models for SNHL and HC. A total of 52 children with SNHL that underwent CI were selected to establish a predictive model of the outcome measured by the category of auditory performance (CAP), and their resting-state fMRI images were acquired. After the dimensional reduction of FCs by kernel principal component analysis, three machine learning methods including the support vector machine, logistic regression, and k-nearest neighbor and their voting were used as the classifiers. A multiple logistic regression method was performed to predict the CAP of CI. The classification model of voting achieves an area under the curve of 0.84, which is higher than that of three single classifiers. The multiple logistic regression model predicts CAP after CI in SNHL with an average accuracy of 82.7%. These models may improve the identification of SNHL through fMRI images and prognosis prediction of CI in SNHL.
Introduction
Congenital sensorineural hearing loss (SNHL) occurs in 0.2–0.4% of live births. It affects approximately 40,000 children in the United States (US) each year, and nearly two-thirds of cases result in bilateral hearing loss (Prosser et al., 2015; Lieu et al., 2020). In China, approximately 30 million people suffer from congenital SNHL and there are ∼23,000 newborn deaf children and ∼50–60,000 late-onset deafness patients every year (National Bureau of Statistics of the People’s Republic of China [NBSPRC], 2007). Hearing loss results from the blockage or attenuation of auditory input to the brain and changes the connectivity and processing of the auditory stimulus by the brain (Halliday et al., 2017; Wilson et al., 2017). Bilateral SNHL can impair the speech development of children and even result in difficulties in socialization and poor academic performance (Tuller and Delage, 2014). Among numerous treatments, cochlear implantation (CI) is highly effective for SNHL (Kral and O’Donoghue, 2010). Therefore, early identification, determination of the cause and appropriate treatment plans are essential for the patient’s recovery.
Medical imaging assumes a crucial role in the diagnostic evaluation of congenital SNHL. In addition to determining the underlying cause, it can also identify related abnormalities caused by hearing loss and evaluate the applicability of surgical intervention (Woolley et al., 1997; Jackson et al., 2015; Gillard et al., 2020). Preferred imaging modalities for evaluating children’s SNHL are high-resolution computed tomography (HRCT) and magnetic resonance imaging (MRI) of the temporal bone. The diagnosis rate of HRCT is ∼30% (Chen et al., 2014). MRI can detect more brain abnormalities and has a higher diagnosis rate than HRCT (Buchman et al., 2006; Ratnanather, 2020). In particular, MRI is highly effective in identifying cochlear nerve defects, which are common in SNHL (Manno et al., 2021).
Previous studies reported significant differences in the MRI-based cerebral volume and gray matter microstructure in children with SNHL (Moon et al., 2020). Hearing loss may affect white matter tracts linking the eighth cranial nerve to subcortical nuclei (i.e., cochlear) and primary auditory cortices (i.e., Heschl’s gyri) and the gray matter of primary auditory cortices (Feng et al., 2018; Tarabichi et al., 2018). Cross-modal reorganization in the auditory deprived cortex was extensively reported (Lomber et al., 2010; Ding et al., 2015). Specifically, for sensory inputs such as hearing, vision, and touch in individuals who lack one sensory mode, another sensory mode can “take over” the cortical area belonging to the lacking sensory mode (Neville et al., 1998; Sadato et al., 2004). Studies involving animal autopsies support this cross-modal plasticity. Cell structure changes were observed in the auditory cortex of deaf cats, whose magnitudes are related to the age of onset of deafness (Butler and Lomber, 2013).
Functional magnetic resonance imaging (fMRI) is a non-invasive technique that can be used to study brain function changes in a variety of diseases, providing valuable information for explaining pathogenesis and guiding clinical practice (Mulders et al., 2015; Puschmann and Thiel, 2017; Vos et al., 2017; Xia et al., 2017; Tang et al., 2019; Jin et al., 2021). According to previous studies, resting-state fMRI (rs-fMRI) can reflect underlying neuronal activity (Logothetis, 2002; Qi et al., 2015; Zhu et al., 2019; Qian et al., 2021). The analysis of functional connections (FCs) calculates the temporal correlation of blood-oxygen-level-dependent (BOLD) signal fluctuations between brain regions. A positive FC indicates that the activity between two voxels or brain regions is synchronized. Recent studies with FC show that SNHL infants exhibit functional reorganization of the auditory network in the early stage of the sensitive or critical period (Wang et al., 2019, 2021; Chen et al., 2020; Cui et al., 2022). Alterations in the regional homogeneity measured by rs-fMRI have also been reported in the auditory, visual, motor, and other related brain cortices for children with congenital SNHL (Guo et al., 2021).
Accurate prediction of the clinical outcome after CI will help project realistic expectations of the benefit for each patient with SNHL, prepare additional rehabilitation for patients with under-performance, and even improve the implantation criteria and procedures (Velde et al., 2021). The CI outcome is assessed by the categories of auditory performance (CAP) score, which can be predicted by the preoperative auditory brainstem response (ABR) and the area ratio of the vestibulocochlear nerve to the facial nerve (Han et al., 2019). Using demographic, audiological and hearing-related clinical history, as well as etiology features, machine learning models can outperform linear ones in the prediction of CI outcomes in adult patients, although their overall accuracy remains limited (Shafieibavani et al., 2021).
It is noted that by using rs-fMRI images, the accurate identification method of SNHL and good prognostic prediction of CI are not well investigated. In this study, we propose to combine FCs measured by rs-fMRI and machine learning to identify SNHL and predict the outcome of CI measured by CAP. To the best of our knowledge, this is the first such reported study.
Materials and Methods
Participants
Initially 83 children with congenital SNHL aged 0–11 years prior to undergoing CI surgery from May 2014 to October 2020 at the Affiliated Hospital of Guizhou Medical University were selected in this study (Figure 1). At the same time, we selected 42 patients for the normal hearing control group, who attended the hospital for other treatments.
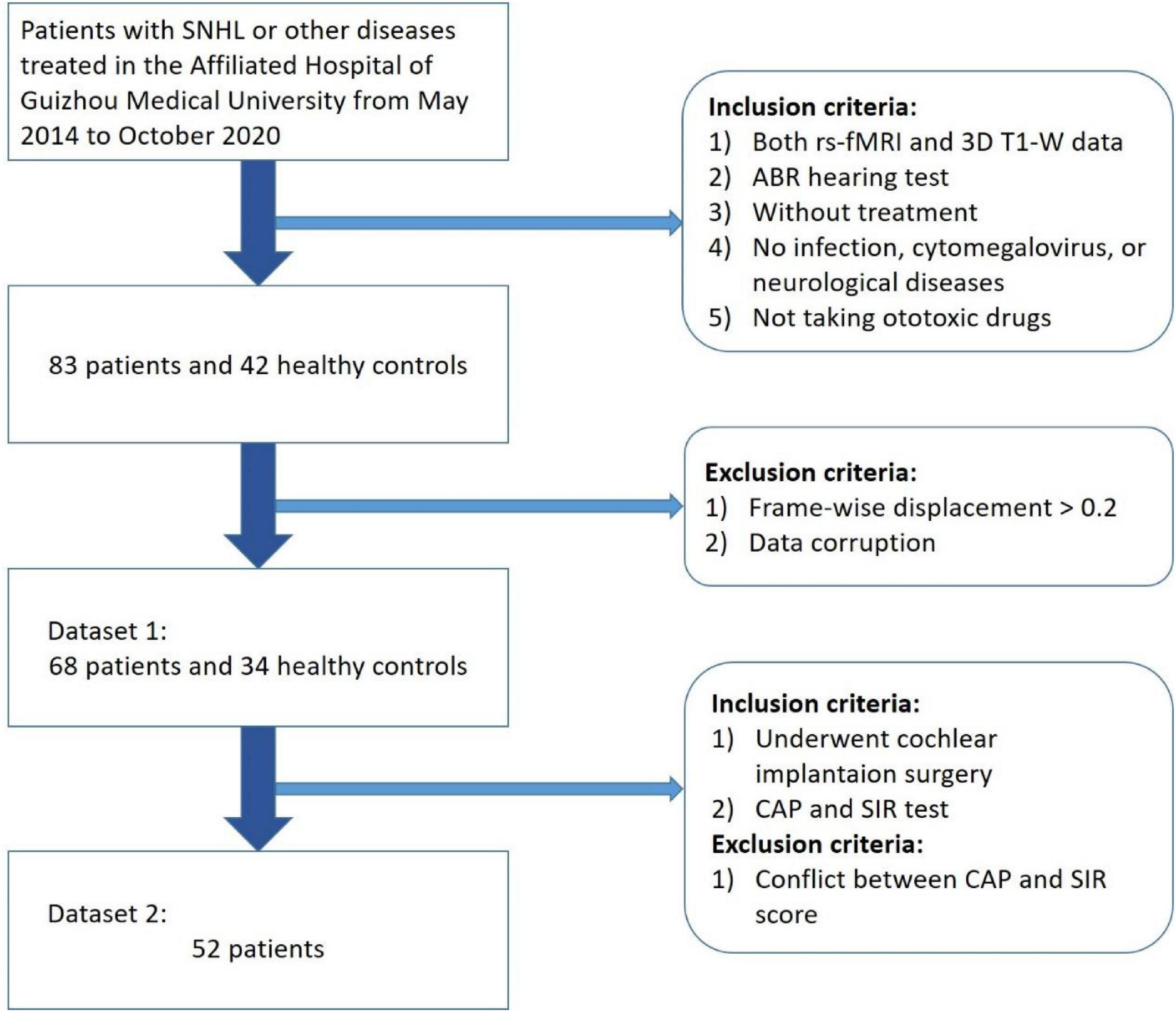
Figure 1. Participant selection procedure (a total of 68 children patients with congenital SNHL and 34 health controls were selected as Dataset 1 and 52 patients who underwent cochlear implantation were selected as Dataset 2).
All children were subjected to hearing screening. Patients with an auditory brainstem response (ABR) higher than 90 dB were considered to have severe bilateral hearing loss. All deaf children participating in the study had not worn hearing aids, had not taken ototoxic drugs, nor had a history of cytomegalovirus, infection, trauma, or any other neurological disease. Due to the relatively young age of the subjects in this study, patients were orally administered 10% chloral hydrate solution with a dosage of 0.5 mL/kg to ensure the quality of the scan. Before the examination, all of the subjects’ parents signed an informed consent form. This study was approved by the ethics committee of the Affiliated Hospital of Guizhou Medical University.
During image preprocessing, 15 SNHL and 8 HC children were excluded due to head movement and data corruption such as missing files, errors in file format conversion, etc. The remaining 68 SNHL patients and 34 HCs constituted Dataset 1. Among the 68 SNHL patients, 53 patients who underwent CI surgery took the CAP test and the speech intelligibility rating (SIR) test. One CI device was implanted into the unilateral ear with the lower hearing loss measured by ABR for each patient. All CI devices were provided by the same brand and manufacturer. No related complications were observed in the children involved in this study. One patient was excluded due to a conflict between CAP and SIR scores (CAP = 1; SIR = 3). Finally, 52 SNHL patients who underwent CI constituted Dataset 2.
Magnetic Resonance Imaging Image Acquisition
The MR images of all participants were acquired before receiving any treatment. In this study, we used a Philips Achieva 3.0TX series MR scanner with eight-channel phased coils. The high-resolution T1-weighted images were obtained with the following parameters: echo time (TE) = 4.6 ms, repetition time (TR) = 9.4 ms, flip angle (FA) = 8°, slice thickness = 1.6 mm, slice interval = 0.8 mm, field of view (FOV) = 220 × 220 mm2, acquisition matrix = 276 × 227 (i.e., the pixel spacing was 0.797 and 0.969 mm, respectively).
Using the echo plane imaging sequence, the BOLD-based functional MR images were obtained with the flowing the parameters: TE = 30 mm, TR = 2000 ms, time point = 200, FA = 90°, FOV = 220 × 220 mm2, slice thickness = 3.40 mm, the number of slices = 35.
Study Procedure
The overall study procedure is illustrated in Figure 2. It comprises two main tasks, namely, (1) classification of SNHL and HC and (2) prediction of CAP after CI in SNHL. For task (1), Dataset 1 was used with five main steps: (I) Image preprocessing; (II) Construction of the functional brain network; (III) De-duplication and flattening; (IV) Dimensionality reduction by kernel principal component analysis (KPCA); (V) Classification by machine learning methods. Task (2) employed Dataset 2. After the dimensionality reduction, the obtained principal components and parameters of age, gender, and ABR were used to build a multiple logistic regression (MLR) model to predict CAP after CI in SNHL. The details of each task and step are given as follows.
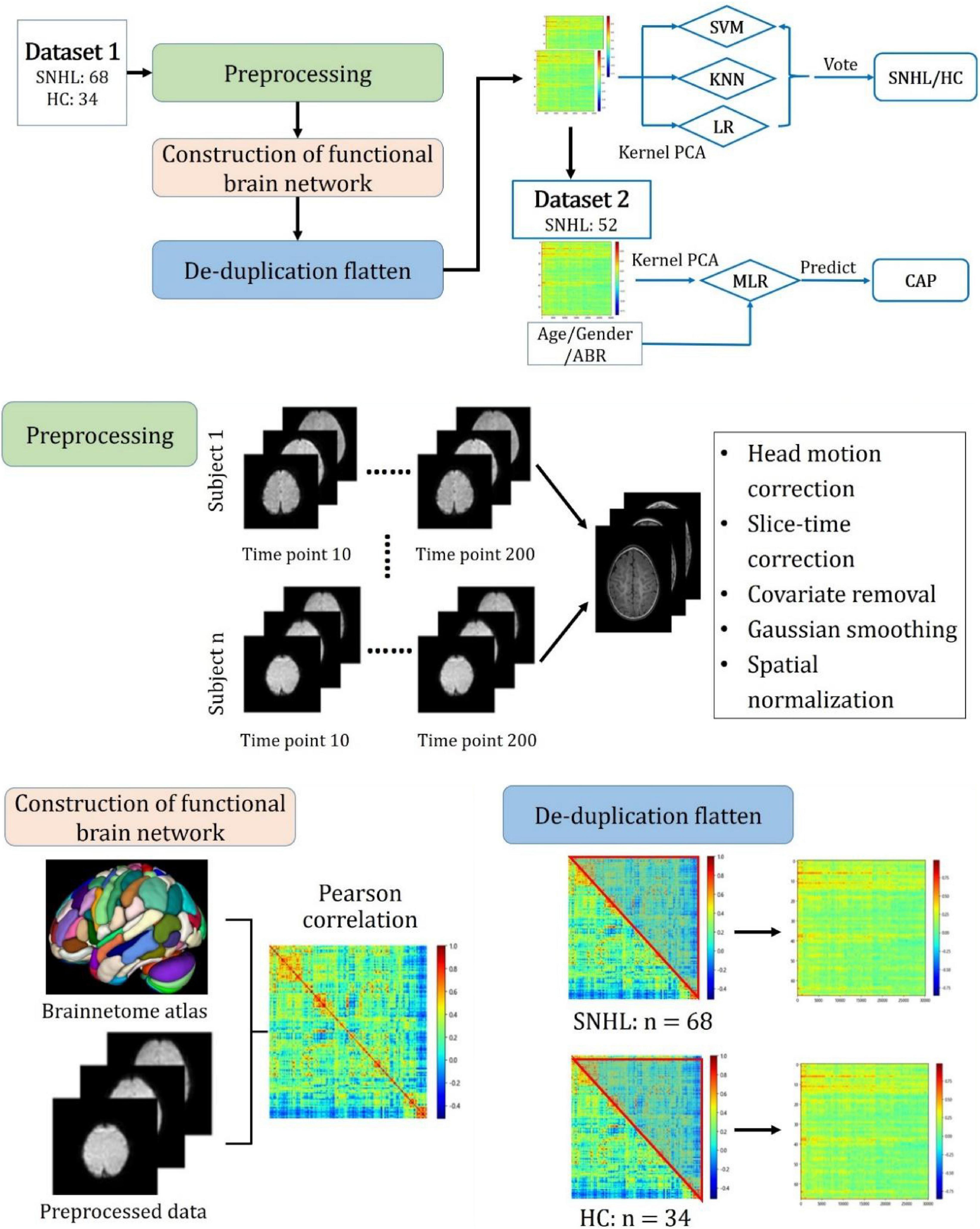
Figure 2. Overall study procedure with main steps including preprocessing, construction of functional brain network, de-duplication flattening, construction of machine learning models for SNHL identification, and building of multiple logistic regression model for predicting the outcome after cochlear implantation measured by the category of auditory performance.
Data Preprocessing and Construction of Brain Networks
We preprocessed rs-fMRI images using the Matlab R2018a platform with data processing and analysis for brain imaging (DPABI) and statistical parameter mapping (SPM) software SPM12. First, we converted the files from the DICOM format into a standard NIFTI format. Second, the first 10 time-points in the serial of rs-fMRI data were discarded to avoid errors caused by unstable magnetic fields. Third, the slice time correction, head motion correction, and covariate removal were performed on rs-fMRI data. Fourth, the initial coordinates of T1-weighted images (T1WI) and fMRI images were manually located to the anterior commissure, and images with excessive displacement and rotation deviations were corrected manually. Fifth, the processed images were normalized to the space of the Montreal Neurological Institute (MNI). Finally, the images were filtered and smoothed using a Gaussian filter with the default setting. Instances where the average frame displacement of the image exceeded 0.2 were considered to have exceeded the allowed head movement were thus removed from the data set.
Preprocessed rs-fMRI data were used to construct the functional brain network. In this study, the Human Brainnetome Atlas released by the Chinese Academy of Sciences was applied to parcellate the whole brain into 246 subregions (Fan et al., 2016). The Pearson coefficient between time series in different subregions can be calculated, which is referred to as the FC. After eliminating the double-counted FCs, we finally obtained a vector of 30,135 FCs for each participant.
Dimension Reduction Method
The principal component analysis (PCA) is a dimension reduction method that transforms multiple related original variable indicators into several independent comprehensive ones. It uses several important principal components to explain most original data and improve analysis efficiency. In the case of a non-linear attribute in the original data, the principal components extracted by principal component analysis cannot reflect this non-linear attribute. Kernel PCA (KPCA) is a non-linear transformation based on the original data, which extracts the non-linear relationship between the data. Therefore, KPCA is adopted in this study.
Moreover, the selection of optimal kernel functions and the number of features for different classifiers was conducted by the grid search method. The Kernel function can choose a linear, sigmoid, poly, radial basis function (RBF) kernel. Studies have shown (Gillies et al., 2016) that the feature number is generally selected as 10% of the sample size to obtain a better classification effect. Hence, this study selected 10 features as the benchmark, and the number of features is searched from 2 to 20.
Machine Learning Model
We selected three Models of support vector machine (SVM), logistic regression classifier (LR) and nearest neighbor algorithm classifier (KNN) as machine learning classifiers. The voting was conducted by prediction of the three classifiers. The voting method involves averaging the predicted probabilities of the samples according to different classifiers. To ensure the best performance of the classifier, the internal hyperParameters of the machine learning classifier and the parameters of the KPCA are simultaneously determined by the grid search. We performed 10-fold cross-validation on Dataset 1 and subsequently combined the results of each fold to obtain the final precision, recall, F1-score, accuracy, and area under curve (AUC).
Prediction of Category of Auditory Performance After Cochlear Implantation
Because the CAP test results are all integer ratings, this study employs a multiple logistic regression method to perform a multi-class analysis on Dataset 2. Studies showed (Wu et al., 2016) that the effect of surgery is highly correlated with the age of CI. Therefore, we have introduced age as an independent variable. In Dataset 2, we preprocessed the fMRI data and calculated the FC, and then used KPCA for dimensionality reduction. Then, we selected CAP as the dependent variable; age, and gender as independent variables; principal components, and ABR data as covariates, and conducted MLR analysis using SPSS25 software.
MLR is represented as follows.
Here, P denotes the posterior probability of the sample point xi belonging to the category r, and xi is a feature vector with dimension D. The weight vector of the i-th category is set to wi, and there is a total of K weight vectors.
Results
Demographic and Clinical Information
Tables 1, 2 summarize the demographic and clinical characteristics of all participants (Dataset 1) and the participants who underwent CI (Dataset 2). The age in the HC and SNHL groups was 45.62 ± 27.63 and 46.24 ± 24.38 months (mean ± SD) and the ranges 12–117 and 12–137 months, respectively. There were no significant differences in gender and age between HC and SNHL groups (p > 0.05).
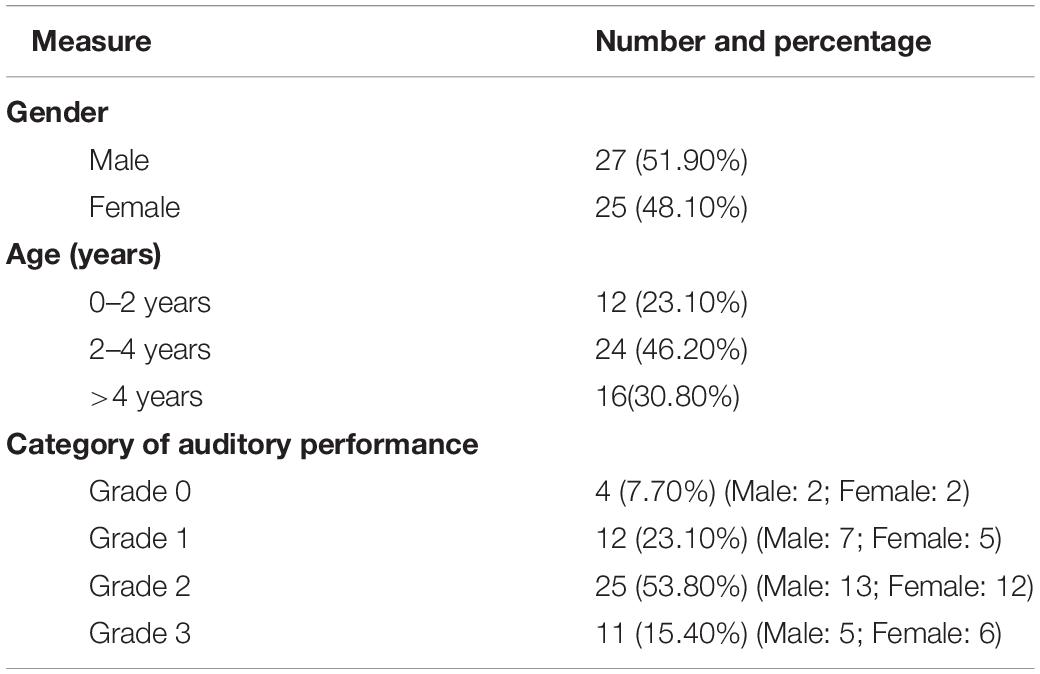
Table 2. Demographic and clinical characteristics of participants who underwent cochlear implantation.
Dataset 2 includes 25 females and 27 males. There were 12 subjects aged range of 0–2 years (23.10%), 24 subjects of 2–4 years (46.20%), and 16 of > 4 years (30.80%). The number of subjects in Grade 0, 1, 2, and 3 of CAP was 4 (7.70%), 12 (23.10%), 25 (53.80%), and 11 (15.40%), respectively.
Optimal Model Parameters
Table 3 shows the optimal KPCA parameters and internal hyperparameters of each classifier. Sigmoid kernels are more suitable for SVM and KNN classifiers, and their optimal number of features is six and five, respectively. Linear kernels are more suitable for LR, and the optimal number of features is ten. For SVM classifier, the optimal hyperparameters are C = 0.62, gamma = 4.268, and Kernel = “RBF”. The optimal number of neighbors is nine in KNN. The optimal type of penalty is “L2”, and the solver is “L-BFGS” in LR.
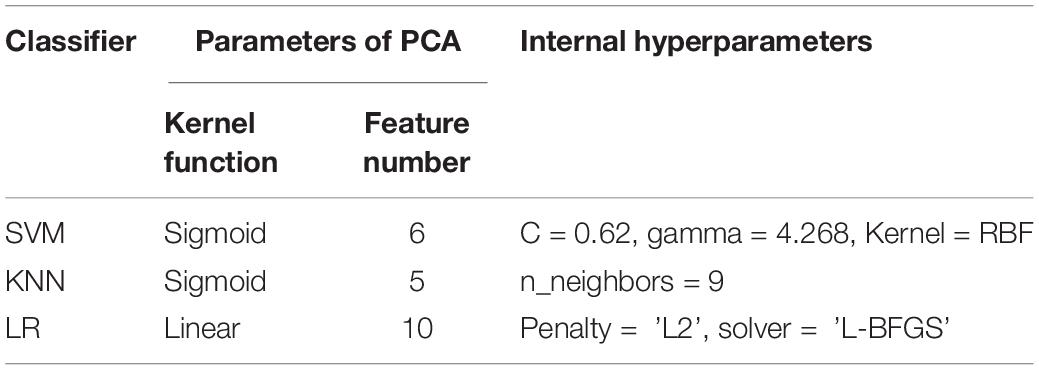
Table 3. Optimal parameters of dimensionality reduction and internal hyperparameters of the classifiers.
Classification Performance of Healthy Controls vs. Sensorineural Hearing Loss
Figure 3 shows the receiver operating characteristic curve and the confusion matrix of the classification of HC vs. SNHL by three machine-learning methods and their voting. The AUCs of SVM, KNN, and LR are 0.79, 0.79, and 0.80, respectively. No significant difference was found among the three classifiers (DeLong test, p > 0.05). After the voting, the AUC increased to 0.84, higher than every single classifier, although significance is not observed (DeLong test, p > 0.05). In the confusion matrix of the voting method, 18 of 68 SNHL are wrongly predicted as HC and 4 of 34 HC are wrongly predicted as SNHL.
Table 4 shows the precision, recall, F1-score, accuracy, and AUC of three machine-learning methods and their voting. As expected, the voting yields higher performance compared to the three single machine-learning methods for all measures: precision of 0.81; recall of 0.77; F1-score of 0.78; and accuracy of 0.77.
Prediction Performance of Category of Auditory Performance After Cochlear Implantation
Table 5 lists the fitting information of CAP after CI by multiple logistic regression (MLR). The final value of MLR using –2 Log likelihood as the fitting condition reaches 54.716, and the interception is 126.504. For the likelihood ratio, the value of Chi-square, degree of freedom, and significance is 71.788, 42, and 0.003, respectively. The significance is 0.003, considerably below 0.05, indicating a high degree of fitting. For the pseudo R-squared test, the final value of Cox-Snell, Nagelkerke, and McFadden reaches 0.749, 0.821, and 0.567, respectively. All values are close to 1.0 and larger than 0.5, indicating a good fitting result.
Defining wj⋅xi as Gj, the fitted parameters are given as follows.
Here, f1,f2,…,f10 are the features After dimensionality reduction. The value age1 represents 1 if it is in the range of 0–2 years; otherwise, it is 0. The value age2 represents 1 if it is in the range of 2–4 years; otherwise it is 0. If the subject is a female, the value female is 1; otherwise, it is 0.
Pi represents the probability that the subject belongs to the i-th category of auditoria performance.
Using Eqs (2–6) and the input data off1,f2,…,f10, ABR, age1, age2, and female, we obtain the statistical results listed in Table 6. The prediction accuracy to CAP of 0, 1, 2, and 3 is 100.0, 83.3, 92.0, and 54.5%. For CAP of 0, 1, and 2, the prediction is very good. However, the prediction performance is poor for CAP of 3. 4 of 11, and 1 of 11 SNHL patients with CAP of 3 were wrongly predicted as CAP 2 and 1, respectively. This indicates that there is a minor difference between functional netWorks of CAP 2 and CAP 3. The average accuracy for the prediction of the four categories is 82.7%.
Discussion
The preoperative evaluation of children with SNHL about to undergo cochlear implantation was performed using rs-fMRI images, including auxiliary diagnosis and prediction of postoperative recovery. By combining functional connections measured by fMRI and machine learning methods, the classification models were constructed, and the highest AUC of differentiating SNHL from HC reached 0.84. Furthermore, the multiple logistic regression model was obtained, which predicts CAP after CI in SNHL with an average accuracy of 82.7%.
Diagnosis of Sensorineural Hearing Loss by the Combination of Functional Brain Network and Machine Learning
Numerous studies showed that brain connections and functions of SNHL patients undergo numerous changes compared to the control group; however, their conclusions are not consistent (Kral and O’Donoghue, 2010; Huang et al., 2015; Liu et al., 2015; Shi et al., 2016; Xu et al., 2016; Tobyne et al., 2017; Tarabichi et al., 2018). Our classification models differ from previous studies, as they differentiate SNHL from HC on account of all differences between the two groups, which are embedded in the principal components extracted from FCs in the brain. Specific changes of FCs and functions of brain regions cannot be traced back due to the nature of kernel PCA.
Due to the complexity of the etiology of congenital SNHL, the diagnosis and cause determination of congenital SNHL often require a comprehensive evaluation combined with multiple diagnostic methods. Imaging methods are mostly employed to detect anatomical structures of the cochlea, labyrinth, and cranial nerves (Mulders et al., 2015; Puschmann and Thiel, 2017; Vos et al., 2017; Xia et al., 2017; Tang et al., 2019). Anatomical abnormalities can help confirm the cause of SNHL. Furthermore, in some cases, these abnormalities may completely exclude surgical intervention.
However, previous studies have shown that in imaging examinations, the diagnostic rate of HRCT is estimated to be 30% (Chen et al., 2014), and the effect of MRI is slightly higher. Our study focuses on the identification of SNHL based on the characteristics of brain function connections. The classification accuracy can reach 0.77, which outperforms traditional imaging methods. This may help doctors diagnose SNHL early and make clinical decisions.
Prediction of Category of Auditory Performance After Cochlear Implantation in Sensorineural Hearing Loss by Multiple Logistic Regression
Previous studies (Woolley et al., 1997; Jackson et al., 2015) showed that the decision whether to carry out surgical interventions generally relies on the discovery of abnormal anatomical structures, and there is no clear decision basis for the types of abnormalities. This study predicts the CAP as a prognostic measure after CI in SNHL, based on the characteristics of brain function connections. The average accuracy achieves 82.7%. This may contribute to decision-making in surgical intervention, and help establish psychological expectations for patients and their families and thus reduce unnecessary medical disputes.
The deprivation of auditory input in the early sensitive period has a significant impact on the internal organization of the brain, with lasting effects. The FCs we choose can represent changes in the patient’s brain function, and the age at the time of surgery is an important parameter for predicting postoperative effects. Simultaneously, to consider the different development speeds due to gender differences, gender is used as a reference factor (Sharma and Campbell, 2011; Kral and Sharma, 2012).
Limitations and Future Work
This study has certain limitations. First, the prediction accuracy of this study is not particularly high, which may be related to the large number of younger subjects whose brain connections and structural changes are not yet evident. All children in this study are patients with severe congenital deafness, and the diagnostic effect for mild cases is temporarily unknown. Second, currently, it is not possible to determine the specific location of abnormal brain regions based on the diagnostic results, it is impossible to prompt the cause, and further research is required for validation. Third, the evaluation index CAP of the surgical effect generally contains eight levels, whereas the evaluation results obtained only range from 0 to 3, such that it is impossible to make more accurate regression predictions. This means that if there is a pathology with a postoperative effect that is better than grade 3, it cannot be predicted. Fourth, the participants ranged in age from 12 to 137 months, which is when the human brain undergoes dramatic changes. Narrowing the age range may make the present results more convincing while decreasing the number of participants.
In the future, research will be carried out in the direction of improving the diagnostic accuracy and disease traceability to provide an important basis for the diagnosis of the cause of SNHL. As the volume of data increases, we expect that the predictive ability of the postoperative effect will be enhanced, which may provide useful support for the diagnosis and treatment of SNHL.
Conclusion
We used functional brain connections to identify sensorineural hearing loss and predict the outcome of cochlear implantation by machine learning methods. The constructed machine learning models, in particular the one after voting, accurately classified participants into SNHL and HC. The constructed multiple logistic regression model enables the prediction of CAP after CI in SNHL with a high average accuracy. These models might help improve the diagnosis of SNHL through fMRI images and prognosis prediction of CI in SNHL.
Data Availability Statement
The datasets presented in this article are not readily available because they must be approved by the Ethics Committee of The Affiliated Hospital of Guizhou Medical University. Requests to access the datasets should be directed to HY, MzMxNjkzODYxQHFxLmNvbQ==.
Ethics Statement
The studies involving human participants were reviewed and approved by The Affiliated Hospital of Guizhou Medical University. Written informed consent to participate in this study was provided by the participants’ legal guardian/next of kin.
Author Contributions
QS performed experiments and analyzed the data along with SQ. SQ, WQ, HZ, and HY conceived the study, presented the results, and wrote the manuscript along with QS. YY, HZ, and HY collected and analyzed the data. LY and CJ supervised the algorithm development and analyzed the data. All authors read and approved the final manuscript.
Funding
This work was partly supported by the National Natural Science Foundation of China under Grant (Nos. 82072008 and 81871846) and the Fundamental Research Funds for the Central Universities (N181904003, N172008008, and 2124006-3).
Conflict of Interest
The authors declare that the research was conducted in the absence of any commercial or financial relationships that could be construed as a potential conflict of interest.
Publisher’s Note
All claims expressed in this article are solely those of the authors and do not necessarily represent those of their affiliated organizations, or those of the publisher, the editors and the reviewers. Any product that may be evaluated in this article, or claim that may be made by its manufacturer, is not guaranteed or endorsed by the publisher.
Abbreviations
ABR, auditory brainstem response; AUC, area under curve; BOLD, blood-oxygen-level-dependent; CAP, category of auditory performance; CI, cochlear implantation; DPABI, data processing & analysis for brain imaging; FA, flip angle; FC, functional connection; fMRI, functional magnetic resonance imaging; FOV, field of view; HC, health control; HRCT, high-resolution computed tomography; KNN, k-nearest neighbor; KPCA, kernel principal component analysis; LR, logistic regression; MLR, multiple logistic regression; MRI, magnetic resonance imaging; PCA, principal component analysis; rs-fMRI, resting-state fMRI; SIR, speech intelligibility rate; SNHL, sensorineural hearing loss; SVM, support vector machine; TE, echo time; TR, repetition time.
References
Buchman, C. A., Roush, P. A., Teagle, H. F., Brown, C. J., Zdanski, C. J., and Grose, J. H. (2006). Auditory neuropathy characteristics in children with cochlear nerve deficiency. Ear Hear. 27, 399–408. doi: 10.1097/01.aud.0000224100.30525.ab
Butler, B. E., and Lomber, S. G. (2013). Functional and structural changes throughout the auditory system following congenital and early-onset deafness: implications for hearing restoration. Front. Syst. Neurosci. 7:92. doi: 10.3389/fnsys.2013.00092
Chen, J., Hu, B., Qin, P., Gao, W., Liu, C., Zi, D., et al. (2020). Altered brain activity and functional connectivity in unilateral sudden sensorineural hearing loss. Neural Plast. 2020:9460364. doi: 10.1155/2020/9460364
Chen, J. X., Kachniarz, B., and Shin, J. J. (2014). Diagnostic yield of computed tomography scan for pediatric hearing loss: a systematic review. Otolaryngol. Head Neck Surg. 151, 718–739. doi: 10.1177/0194599814545727
Cui, W., Wang, S., Chen, B., and Fan, G. (2022). Altered functional network in infants with profound bilateral congenital sensorineural hearing loss: a graph theory analysis. Front. Neurosci. 15:810833. doi: 10.3389/fnins.2021.810833
Ding, H., Qin, W., Liang, M., Ming, D., Wan, B., Li, Q., et al. (2015). Cross-modal activation of auditory regions during visuo-spatial working memory in early deafness. Brain 138, 2750–2765. doi: 10.1093/brain/awv165
Fan, L., Li, H., Zhuo, J., Zhang, Y., Wang, J., Chen, L., et al. (2016). The human brainnetome atlas: a new brain atlas based on connectional architecture. Cereb. Cortex 26, 3508–3526. doi: 10.1093/cercor/bhw157
Feng, G., Ingvalson, E. M., Grieco-Calub, T. M., Roberts, M. Y., Ryan, M. E., Birmingham, P., et al. (2018). Neural preservation underlies speech improvement from auditory deprivation in young cochlear implant recipients. Proc. Natl. Acad. Sci. U S A. 115, E1022–E1031. doi: 10.1073/pnas.1717603115
Gillard, D. M., Chuang, N. A., Go, J. L., and Kari, E. (2020). Abnormal cochleovestibular anatomy and imaging: lack of consistency across quality of images, sequences obtained, and official reports. Int. J. Pediatr. Otorhinolaryngol. 134:110021. doi: 10.1016/j.ijporl.2020.110021
Gillies, R. J., Kinahan, P. E., and Hricak, H. (2016). Radiomics: images are more than pictures, they are data. Radiology 278, 563–577. doi: 10.1148/radiol.2015151169
Guo, P., Lang, S., Jiang, M., Wang, Y., Zeng, Z., Wen, Z., et al. (2021). Alterations of regional homogeneity in children with congenital sensorineural hearing loss: a resting-state fMRI study. Front. Neurosci. 15:678910. doi: 10.3389/fnins.2021.678910
Halliday, L. F., Tuomainen, O., and Rosen, S. (2017). Language development and impairment in children with mild to moderate sensorineural hearing loss. J. Speech Lang. Hear. Res. 60, 1551–1567. doi: 10.1044/2016_JSLHR-L-16-0297
Han, J. J., Suh, M. W., Park, M. K., Koo, J. W., Lee, J. H., and Oh, S. H. (2019). A predictive model for cochlear implant outcome in children with cochlear nerve deficiency. Sci. Rep. 9:1154. doi: 10.1038/s41598-018-37014-7
Huang, L., Zheng, W., Wu, C., Wei, X., Wu, X., Wang, Y., et al. (2015). diffusion tensor imaging of the auditory neural pathway for clinical outcome of cochlear implantation in pediatric congenital sensorineural hearing loss patients. PLoS One 10:e0140643. doi: 10.1371/journal.pone.0140643
Jackson, N. M., Givens, V. B., Carpenter, C. C., Allen, L. M., Morrell, B. B., Hurth, C., et al. (2015). Cochlear trajectory in pediatric patients. Laryngoscope 125, 961–965. doi: 10.1002/lary.24984
Jin, C., Qi, S., Teng, Y., Li, C., Yao, Y., Ruan, X., et al. (2021). altered degree centrality of brain networks in Parkinson’s disease with freezing of gait: a resting-state functional MRI study. Front. Neurol. 12:743135. doi: 10.3389/fneur.2021.743135
Kral, A., and O’Donoghue, G. M. (2010). Profound deafness in childhood. N. Engl. J. Med. 363, 1438–1450. doi: 10.1056/nejmra0911225
Kral, A., and Sharma, A. (2012). Developmental neuroplasticity after cochlear implantation. Trends Neurosci. 35, 111–122. doi: 10.1016/j.tins.2011.09.004
Lieu, J. E. C., Kenna, M., Anne, S., and Davidson, L. (2020). Hearing loss in children: a review. JAMA 324, 2195–2205. doi: 10.1001/jama.2020.17647
Liu, B., Feng, Y., Yang, M., Chen, J. Y., Li, J., Huang, Z. C., et al. (2015). functional connectivity in patients with sensorineural hearing loss using resting-state MRI. Am. J. Audiol. 24, 145–152. doi: 10.1044/2015_AJA-13-0068
Logothetis, N. K. (2002). The neural basis of the blood-oxygen-level-dependent functional magnetic resonance imaging signal. Philos. Trans. R. Soc. Lond. B. Biol. Sci. 357, 1003–1037. doi: 10.1098/rstb.2002.1114
Lomber, S. G., Meredith, M. A., and Kral, A. (2010). Cross-modal plasticity in specific auditory cortices underlies visual compensations in the deaf. Nat. Neurosci. 13, 1421–1427. doi: 10.1038/nn.2653
Manno, F. A. M., Rodriguez-Cruces, R., Kumar, R., Ratnanather, J. T., and Lau, C. (2021). Hearing loss impacts gray and white matter across the lifespan: systematic review, meta-analysis and meta-regression. Neuroimage 231:117826. doi: 10.1016/j.neuroimage.2021.117826
Moon, P. K., Qian, J. Z., McKenna, E., Xi, K., Rowe, N. C., Ng, N. N., et al. (2020). Cerebral volume and diffusion MRI changes in children with sensorineural hearing loss. Neuroimage Clin. 27:102328. doi: 10.1016/j.nicl.2020.102328
Mulders, P. C., van Eijndhoven, P. F., Schene, A. H., Beckmann, C. F., and Tendolkar, I. (2015). Resting-state functional connectivity in major depressive disorder: a review. Neurosci. Biobehav. Rev. 56, 330–344. doi: 10.1016/j.neubiorev.2015.07.014
National Bureau of Statistics of the People’s Republic of China [NBSPRC] (2007). The Main Data Manual Of the Second National Sample Survey of Persons with Disabilities. Beijing: China Statistics Press, 2–38.
Neville, H. J., Bavelier, D., Corina, D., Rauschecker, J., Karni, A., Lalwani, A., et al. (1998). Cerebral organization for language in deaf and hearing subjects: biological constraints and effects of experience. Proc. Natl. Acad. Sci. 95, 922–929. doi: 10.1073/pnas.95.3.922
Prosser, J. D., Cohen, A. P., and Greinwald, J. H. (2015). Diagnostic evaluation of children with sensorineural hearing loss. Otolaryngol. Clin. North Am. 48, 975–982. doi: 10.1016/j.otc.2015.07.004
Puschmann, S., and Thiel, C. M. (2017). Changed crossmodal functional connectivity in older adults with hearing loss. Cortex 86, 109–122. doi: 10.1016/j.cortex.2016.10.014
Qi, S., Meesters, S., Nicolay, K., Romeny, B. M., and Ossenblok, P. (2015). The influence of construction methodology on structural brain network measures: a review. J. Neurosci. Methods 253, 170–182. doi: 10.1016/j.jneumeth.2015.06.016
Qian, H., Qin, D., Qi, S., Teng, Y., Li, C., Yao, Y., et al. (2021). Less is better: single-digit brain functional connections predict T2DM and T2DM-induced cognitive impairment. Front. Neurosci. 14:588684. doi: 10.3389/fnins.2020.588684
Ratnanather, J. T. (2020). Structural neuroimaging of the altered brain stemming from pediatric and adolescent hearing loss-Scientific and clinical challenges. Wiley Interdiscip. Rev. Syst. Biol. Med. 12:e1469. doi: 10.1002/wsbm.1469
Sadato, N., Yamada, H., Okada, T., Yoshida, M., Hasegawa, T., Matsuki, K., et al. (2004). Age-dependent plasticity in the superior temporal sulcus in deaf humans: a functional MRI study. BMC Neurosci. 5:56. doi: 10.1186/1471-2202-5-56
Shafieibavani, E., Goudey, B., Kiral, I., Zhong, P., Jimeno-Yepes, A., Swan, A., et al. (2021). Predictive models for cochlear implant outcomes: Performance, generalizability, and the impact of cohort size. Trends Hear. 25:23312165211066174. doi: 10.1177/23312165211066174
Sharma, A., and Campbell, J. (2011). A sensitive period for cochlear implantation in deaf children. J. Matern. Fetal Neonatal Med. 24(Suppl. 1), 151–153. doi: 10.3109/14767058.2011.607614
Shi, B., Yang, L. Z., Liu, Y., Zhao, S. L., Wang, Y., Gu, F., et al. (2016). Early-onset hearing loss reorganizes the visual and auditory network in children without cochlear implantation. Neuroreport 27, 197–202. doi: 10.1097/WNR.0000000000000524
Tang, Y., Zhou, Q., Chang, M., Chekroud, A., Gueorguieva, R., Jiang, X., et al. (2019). Altered functional connectivity and low-frequency signal fluctuations in early psychosis and genetic high risk. Schizophr. Res. 210, 172–179. doi: 10.1016/j.schres.2018.12.041
Tarabichi, O., Kozin, E. D., Kanumuri, V. V., Barber, S., Ghosh, S., Sitek, K. R., et al. (2018). Diffusion tensor imaging of central auditory pathways in patients with sensorineural hearing loss: a systematic review. Otolaryngol. Head Neck Surg. 158, 432–442. doi: 10.1177/0194599817739838
Tobyne, S. M., Osher, D. E., Michalka, S. W., and Somers, D. C. (2017). Sensory-biased attention networks in human lateral frontal cortex revealed by intrinsic functional connectivity. Neuroimage 162, 362–372. doi: 10.1016/j.neuroimage.2017.08.020
Tuller, L., and Delage, H. (2014). Mild-to-moderate hearing loss and language impairment: how are they linked? Lingua 139, 80–101. doi: 10.1016/j.lingua.2013.10.009
Velde, H. M., Rademaker, M. M., Damen, J., Smit, A. L., and Stegeman, I. (2021). Prediction models for clinical outcome after cochlear implantation: a systematic review. J. Clin. Epidemiol. 137, 182–194. doi: 10.1016/j.jclinepi.2021.04.005
Vos, F., Koini, M., Schouten, T. M., Seiler, S., Grond, J., Lechner, A., et al. (2017). A comprehensive analysis of resting state fMRI measures to classify individual patients with Alzhemimer’s disease. Alzheimers Dement. 13:26. doi: 10.1016/j.jalz.2017.06.2300
Wang, S., Chen, B., Yu, Y., Yang, H., Cui, W., Fan, G., et al. (2021). Altered resting-state functional network connectivity in profound sensorineural hearing loss infants within an early sensitive period: A group ICA study. Hum. Brain Mapp. 42, 4314–4326. doi: 10.1002/hbm.25548
Wang, S., Chen, B., Yu, Y., Yang, H., Cui, W., Li, J., et al. (2019). Alterations of structural and functional connectivity in profound sensorineural hearing loss infants within an early sensitive period: a combined DTI and fMRI study. Dev. Cogn. Neurosci. 38:100654. doi: 10.1016/j.dcn.2019.100654
Wilson, B. S., Tucci, D. L., Merson, M. H., and O’Donoghue, G. M. (2017). Global hearing health care: new findings and perspectives. Lancet 390, 2503–2515. doi: 10.1016/s0140-6736(17)31073-5
Woolley, A. L., Oser, A. B., Lusk, R. P., and Bahadori, R. S. (1997). Preoperative temporal bone computed tomography scan and its use in evaluating the pediatric cochlear implant candidate. Laryngoscope 107, 1100–1106. doi: 10.1097/00005537-199708000-00017
Wu, C., Huang, L., Tan, H., Wang, Y., Zheng, H., Kong, L., et al. (2016). Diffusion tensor imaging and MR spectroscopy of microstructural alterations and metabolite concentration changes in the auditory neural pathway of pediatric congenital sensorineural hearing loss patients. Brain Res. 1639, 228–234. doi: 10.1016/j.brainres.2014.12.025
Xia, S., Song, T., Che, J., Li, Q., Chai, C., Zheng, M., et al. (2017). Altered brain functional activity in infants with congenital bilateral severe sensorineural hearing loss: a resting-state functional MRI study under sedation. Neural Plast. 2017:8986362. doi: 10.1155/2017/8986362
Xu, H., Fan, W., Zhao, X., Li, J., Zhang, W., Lei, P., et al. (2016). Disrupted functional brain connectome in unilateral sudden sensorineural hearing loss. Hear. Res. 335, 138–148. doi: 10.1016/j.heares.2016.02.016
Keywords: sensorineural hearing loss, resting-state fMRI, functional brain network, cochlear implantation, machine learning, multiple logistic regression
Citation: Song Q, Qi S, Jin C, Yang L, Qian W, Yin Y, Zhao H and Yu H (2022) Functional Brain Connections Identify Sensorineural Hearing Loss and Predict the Outcome of Cochlear Implantation. Front. Comput. Neurosci. 16:825160. doi: 10.3389/fncom.2022.825160
Received: 30 November 2021; Accepted: 08 March 2022;
Published: 30 March 2022.
Edited by:
Lingzhong Fan, Institute of Automation (CAS), ChinaReviewed by:
Brian McKinnon, University of Texas Medical Branch at Galveston, United StatesYuchun Tang, Shandong University, China
Copyright © 2022 Song, Qi, Jin, Yang, Qian, Yin, Zhao and Yu. This is an open-access article distributed under the terms of the Creative Commons Attribution License (CC BY). The use, distribution or reproduction in other forums is permitted, provided the original author(s) and the copyright owner(s) are credited and that the original publication in this journal is cited, in accordance with accepted academic practice. No use, distribution or reproduction is permitted which does not comply with these terms.
*Correspondence: Shouliang Qi, cWlzbEBibWllLm5ldS5lZHUuY24=; Houyu Zhao, emhhb2hvdXl1amlhQDE2My5jb20=; Hui Yu, MzMxNjkzODYxQHFxLmNvbQ==