- 1CNRS UMR 5293, Institut des Maladies Neurodégénératives, Université de Bordeaux, Bordeaux, France
- 2Department of Medical Neurobiology, The Hebrew University of Jerusalem, Jerusalem, Israel
- 3College of Information Technology, United Arab Emirates University, Al-Ain, United Arab Emirates
- 4Research Center for Translational Medicine (KUTTAM), Koç University, Istanbul, Turkey
- 5Department of Biological Sciences, University of Manitoba, Winnipeg, MB, Canada
- 6School of Psychology & Marcs Institute for Brain and Behaviour, Western Sydney University, Sydney, NSW, Australia
- 7School of Psychology, Faculty of Society and Design, Bond University, Robina, QLD, Australia
- 8Faculty of Health Sciences, Department of Human Anatomy and Physiology, University of Johannesburg, Johannesburg, South Africa
Many studies on the drift-diffusion model (DDM) explain decision-making based on a unified analysis of both accuracy and response times. This review provides an in-depth account of the recent advances in DDM research which ground different DDM parameters on several brain areas, including the cortex and basal ganglia. Furthermore, we discuss the changes in DDM parameters due to structural and functional impairments in several clinical disorders, including Parkinson's disease, Attention Deficit Hyperactivity Disorder (ADHD), Autism Spectrum Disorders, Obsessive-Compulsive Disorder (OCD), and schizophrenia. This review thus uses DDM to provide a theoretical understanding of different brain disorders.
Introduction
In daily life, we make numerous decisions; some are less complex, such as choosing a lane in the traffic. Others are very complex such as deciding which experiment to perform to investigate a research question best. Various studies attempt to understand decision-making on formal grounds using biophysical and abstract models. Biophysical models explore the interaction between the neural areas and the changes in the neurotransmitters such as GABA and glutamate (Jocham et al., 2012), while abstract models, such as the drift-diffusion model (DDM), attempt to explain the observed behavior (Ratcliff and Childers, 2015). One of these models, the DDM of decision-making, has gained significant attention. DDM estimates a number of model parameters such as decision threshold, drift rate, and bias based on the observed responses and the response time.
Several studies attribute DDM parameters to several cortical structures (Kim and Shadlen, 1999; Shadlen and Newsome, 2001; Kiani and Shadlen, 2009). These cortical areas work in conjunction with the basal ganglia (a subcortical structure) that provides auxiliary information to facilitate decisions and influence the DDM parameters. (O'Connell et al. 2018) reviewed the link between neural activity in cortical and subcortical areas to DDM parameters. Computationally, Purcell and Palmeri (2017) show that based on neural activity, DDM parameters can be estimated, and vice versa. Additionally, Mulder et al. (2014a) reviewed non-invasive fMRI studies in humans to spotlight the role of various cortical and subcortical areas [prefrontal cortex, frontal eye field, striatum (STR), and pre-supplementary motor area (pre-SMA)] in controlling the DDM parameters. Another review showed that the posterior parietal cortex (PPC), frontal eye fields, dorsal STR, and lateral intraparietal area activity correlate to DDM parameters in humans and monkeys (Hanks and Summerfield, 2017). The authors further showed that in rodents, the rat PPC and frontal orienting fields (homologs of monkey PPC and frontal eye fields) could be neural correlates of DDM parameters (Hanks and Summerfield, 2017). These reviews corroborate the fact that these cortical and subcortical areas are involved in decision-making and are the sites for DDM parameters. A loss of structural and functional connectivity severely impairs the decisions in motor and cognitive tasks. One particular review focusing on imaging studies on psychosis provides a detailed account of the basal ganglia and the role of dopamine in explaining DDM parameters (Horga and Abi-Dargham, 2019). While there are many studies on DDM parameter changes in disorders, reviews on the topic are almost non-existent (Horga and Abi-Dargham, 2019). This review summarizes electrophysiological, behavioral, and imagining studies that correlate DDM parameters to the cortico-basal ganglia (BG) structures. Furthermore, we discuss DDM parameter changes in various disorders such as Parkinson's disease (PD), attention deficit hyperactivity disorder (ADHD), autism spectrum disorders (ASD), obsessive-compulsive disorder (OCD), and schizophrenia. Below, we first discuss the standard parameters of classical DDM briefly (Section The Drift-Diffusion Model). Following that, we discuss the neural substrates underlying each DDM parameter (Section Neural Substrates of DDM). Subsequently, we discuss how different brain disorders impact different DDM parameters (Section Brain Disorders).
The Drift-Diffusion Model
The drift-diffusion model (also known as the diffusion decision model) was proposed by Ratcliff (1978) as an extension of early random walk models (e.g., Wald and Wolfowitz, 1948; Stone, 1960). The proposed model suggested that two-alternative forced-choice behavior can be modeled as a DDM, accumulating noisy evidence in favor of one alternative over another (Figure 1). The sequential addition of evidence enables the model to reach either of the choice-associated thresholds, terminate the evidence accumulation process, and select the choice (for which the threshold is crossed) as the preferred outcome (Ratcliff et al., 2016). The initial bias (or starting point) for evidence accumulation can influence the choice selection by moving the starting point closer to a decision threshold/choice, thereby making it farther away from the other threshold/choice. Bias toward a choice requires lesser evidence accumulation for the choice closer to the bias while requiring more evidence for the alternative choice before reaching the threshold. The drift rate or the rate of evidence accumulation determines how quickly the evidence reaches the decision threshold. Thus, the three decision-related parameters obtained from DDM are decision threshold, bias, and drift rate.
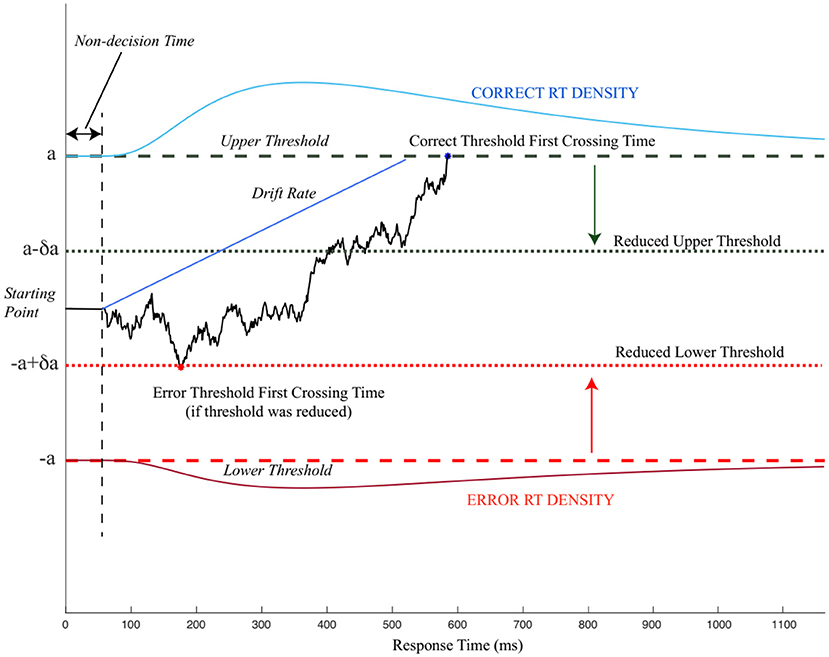
Figure 1. An illustration of the pure drift-diffusion model (DDM). The model accumulates evidence favoring two choices corresponding to correct choice (upper threshold) and incorrect choice (lower threshold). After the non-decision time, which is unrelated to the choices, the DDM starts accumulating evidence in favor of either choice (solid black line). When the evidence reaches either of the threshold (dashed black and red lines), the decision is terminated. The period between starting the evidence accumulation and threshold first crossing time refers to the decision time. If the threshold is reduced (dotted black and red lines), accumulating evidence may terminate the decision-making process earlier with incorrect choice selection modified from Wong et al. (2015).
As with any computational model, the DDM also has several limitations, as discussed in Ratcliff et al. (2016). These limitations led to the development of many variants and alternatives of DDM (Usher and McClelland, 2001; Palmer et al., 2005; Wagenmakers et al., 2007; Ratcliff and McKoon, 2008; Vandekerckhove and Tuerlinckx, 2008; Wiecki et al., 2013). In this review, we focus on only the classical (standard) DDM model. The core parameters of the conventional DDM, including decision threshold, drift rate, bias, are discussed below.
Decision Threshold
The decision threshold limits the amount of evidence gathered before decision finalization. While evaluating a stimulus to two decision threshold hypotheses, the decision-making system gathers evidence in favor of one hypothesis over the other in light of the information received. The decision terminates when one of the two decision thresholds, each representing a different hypothesis, is reached. Further empirical evidence also shows that these thresholds are dependent on multiple factors such as instructions emphasizing speed vs. accuracy (Mulder et al., 2013), task familiarity (Balci et al., 2011), and choice certainty/confidence (Philiastides et al., 2014; Lim et al., 2017).
One of the central concepts of decision-making research is the speed-accuracy tradeoff (SAT) paradigm, which shows that faster decisions are less accurate while slower decisions are more accurate. In a limited time-bound two alternate-forced-choice task, where time is of critical importance, participants must optimize the speed and accuracy to maximize rewards through training (Wald and Wolfowitz, 1948; Bogacz et al., 2006; Balci et al., 2011; Drugowitsch et al., 2012; Spieser et al., 2017; Desender et al., 2019; Riesel et al., 2019). The SAT is modulated within the framework of DDM by decision threshold setting (Mulder et al., 2014a). Specifically, reducing the threshold reduces the evidence required at the expense of task accuracy and vice versa. Earlier work shows that human participants can learn to optimize their decision by adopting the reward-rate maximizing decision threshold (Balci et al., 2011; Desender et al., 2019; Stafford et al., 2020).
In most time-constrained experiments, if a trial is not completed within a predefined time, the trial is considered a failure, and no rewards are obtained. In these time-constrained conditions, movements are often executed before the decision threshold is reached. The decision-making system accumulates evidence to refine or modify the motor output even after motor execution (Resulaj et al., 2009; Wong et al., 2015). The pre-decision movement execution enables a reduction of movement time while allowing for more online movement corrections.
The motor system prepares and executes the movement when certain choice confidence is reached to maximize movement execution and movement correction time. Even after movement execution, the model accumulates evidence to revalidate the selected choice (Krajbich and Rangel, 2011). Though many of the studies suggest that the decision threshold is fixed/constant, a few recent studies show that the threshold may not be fixed (O'Connell et al., 2018). One study indicated that during task execution, the thresholds are dynamically determined (Philiastides et al., 2014; Lim et al., 2017).
Drift Rate
Evidence accumulation is a noisy process. A random Gaussian noise (with known mean and SD) is added at each step within a trial during the evidence sampling process. The drift rate is computed by averaging the rate of evidence accumulation from the start of evidence accumulation to the decision threshold. Averaging also serves the essential purpose of averaging out the instantaneous noise added. Therefore, the DDM parameter, drift rate, account for how quickly evidence is accumulated toward the correct decision threshold. By controlling for other parameters, higher drift rates mean shorter reaction times and higher accuracy. While choosing between rewarded and neutral stimuli (low conflict decisions), the drift rate was observed to be higher, and consequently, RT was found to be shorter (Ratcliff and Frank, 2012; Wiecki et al., 2013; Bond et al., 2018). Additionally, choice-value influences the drift rate, with high payoff decisions having higher drift rates (Starns et al., 2012; Bottemanne and Dreher, 2019). Drift rates also depend on the reliability of sensory evidence, with low-reliability evidence showing lower rates (Balci et al., 2011; Hanks et al., 2011; Drugowitsch et al., 2012; Mulder et al., 2012). In their study, Clay et al. (2017) showed that in high loss aversion (defined as increased sensitivity to losses), participants have a lower drift rate due to over fixation (which is related to higher evidence accumulation) on the non-selected choice even for easy trials (Clay et al., 2017). Additionally, with additional training on a task due to an increase in drift rate, a shorter RT is observed (Dutilh et al., 2009; Balci et al., 2011; Gur et al., 2020). During the aging process in rodents and humans, and/or lower accuracy is observed (Salthouse, 1996; Ratcliff et al., 2007a; McGovern et al., 2018; Gur et al., 2020; Theisen et al., 2020).
Together, these studies show that the drift rates are affected by stimulus quality, stimulus value, training, and the separation between choices.
Bias
The starting point of evidence accumulation, or bias, plays a significant role in determining RT. A change in bias toward a favored choice (mathematically equivalent to reducing the threshold for the preferred choice) results in a lesser evidence requirement for reaching the threshold for favored choice and vice versa, thereby leading to faster RT and quicker decisions. Additionally, the task difficulty of the trial modulates the bias by favoring a more familiar choice (Mulder et al., 2012). The prior probability of associated payoffs is unknown in an unfamiliar task, and no prior memory exists to estimate the trial properties (Bogacz et al., 2006; Mulder et al., 2012). On repeating the trials, the stimulus characteristics are obtained, stored, and recalled for later trials (Simen et al., 2009; Leite and Ratcliff, 2011). Reward associated with the stimulus also influences the bias with higher value stimulus biasing the starting point (Mulder et al., 2012, 2014b; Rao et al., 2012; Fan et al., 2018). While bias is considered a static value predetermined before starting evidence accumulation, Hanks et al. (2011) showed that bias is dynamically altered in an ongoing trial to initiate faster RT.
Neural Substrates Of DDM
For a site to qualify as an evidence accumulation area, the neuronal populations in the candidate brain area should increase their neural activity after stimulus presentation. This activity increase continues till a decision is reached, following which the neural activity returns to baseline (Tremel and Wheeler, 2015; Yartsev et al., 2018).
In this section, we discuss several cortical and subcortical basal ganglia areas that may show characteristics of being correlated with DDM parameters.
Cortical Areas
The prefrontal cortex (PFC), frontal eye fields (FEF), lateral intraparietal area (LIP), superior colliculus (SC), fusiform, occipital, and inferior frontal gyri are observed to be sites for evidence accumulation (Ratcliff et al., 2003, 2007b; Tremel and Wheeler, 2015; Peel et al., 2017; Reppert et al., 2018). This neural activity indicating evidence accumulation in downstream elements is not a surprising finding as it may only mean that the upstream elements are involved in the ongoing decision-making process (Selen et al., 2012). This review explicitly discusses PFC, FEF, LIP, and SC in greater detail (see Table 1 for the summary).
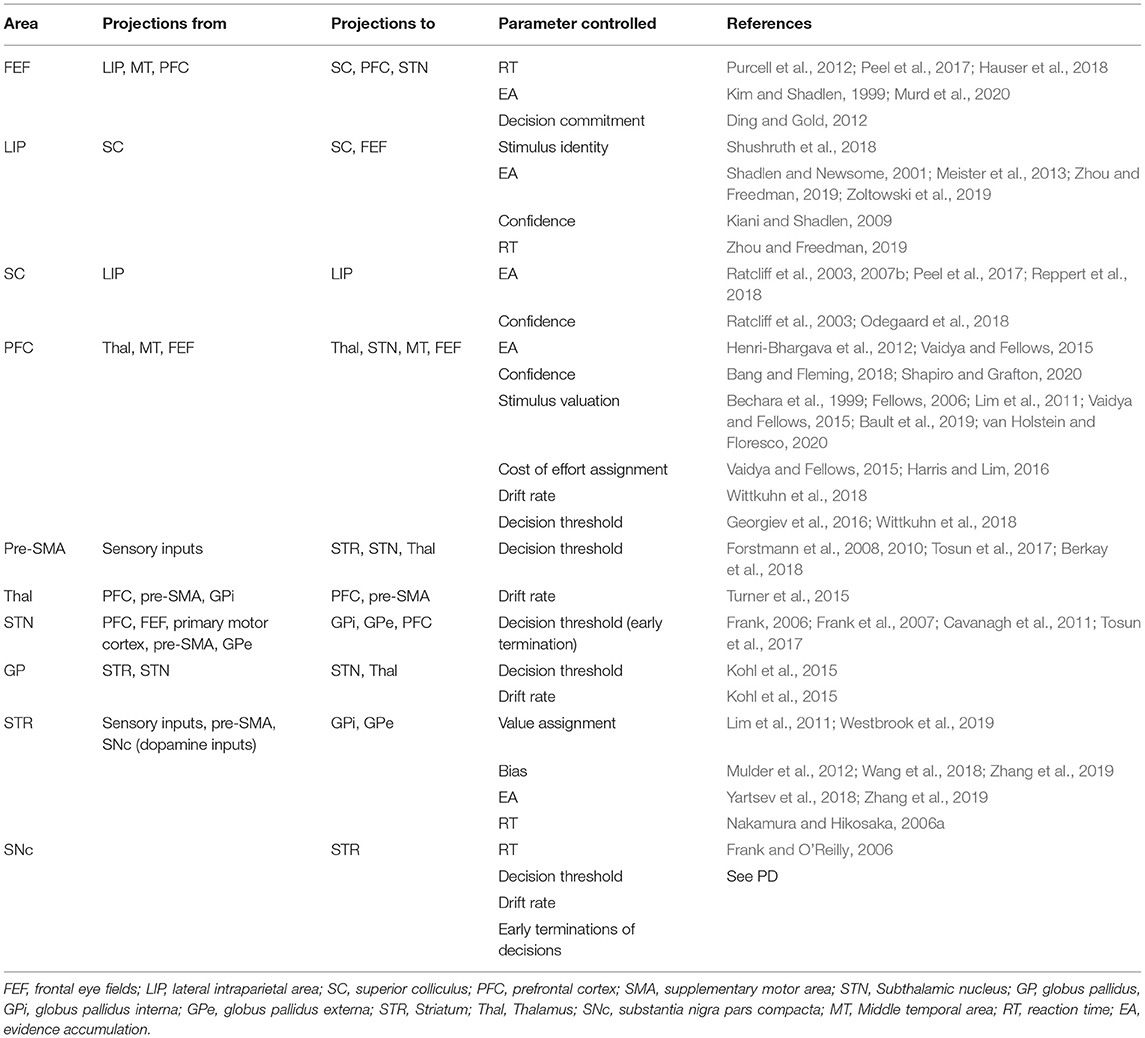
Table 1. Frontal and basal ganglia areas, their connectivity, and effect on drift-diffusion model (DDM) parameters.
Frontal Eye Fields
Many DDM studies are conducted in non-human primates where monkeys indicate the choice using eye movements. The FEF's involvement in eye movement and cognitive tasks has been widely studied. FEF receives inputs from PFC, LIP, middle temporal area (MT) while it outputs to PFC, STN, and SC (Purcell et al., 2010). Saccades showed longer latency with slow velocity and higher errors upon inhibiting FEF using muscimol (a GABA-A receptor agonist; Dias and Segraves, 1999). FEF inhibition reduces SC activity and increases overall RT (Peel et al., 2017). Neural activity in FEF and principal sulcus encodes decisions and performs evidence accumulation, as evident from persistent activity between 200 and 300 ms after cue onset until the saccade onset. Neural activity is modulated during movement toward the response field for low conflict conditions, where the evidence was mostly in favor of one of the two choices (Kim and Shadlen, 1999). To test whether the FEF integrates choice-specific outcomes or categorizes the evidence into discrete actions, Murd et al. (2020) stimulated FEF using TMS. The authors found that the task performance was affected through stimulation only during the choice-specific integration phase and not during the categorization phase. This study provides additional support for the role of FEF in evidence accumulation (Ding and Gold, 2012; Murd et al., 2020).
Lateral Intraparietal Area (LIP)
The LIP neuronal activity corresponds to sensory processing, memory processing, saccade-related responses, direction selectivity, and choices information (Shushruth et al., 2018). During a random dot motion (RDM) decision, where the participant indicates the direction of a cloud of dots motion, LIP neurons' firing rates were higher for correct choices when the motion was toward the receptive field. Interestingly, this activity increase was both before and during the motion display, indicating that LIP neurons access stimulus history for the decision-making process (Rao et al., 2012). The LIP activity was in the intermediate range on selecting an opt-out option (disbursing small yet guaranteed reward), suggesting the presence of confidence encoding neurons (Kiani and Shadlen, 2009). As LIP neurons are involved in evidence accumulation, these neurons combine both sensory and value information for a decision. LIP neurons' activity showed variations in response to the strength of sensory input and the value of the target (both in and outside the receptive field; Shadlen and Newsome, 2001; Zhou and Freedman, 2019; Zoltowski et al., 2019). As contrary to other reports showing that individual neurons are involved in evidence accumulation, Meister et al. (2013) found that the population-level activity in the LIP is more representative of the evidence accumulation.
Superior Colliculus (SC)
As SC has reciprocal connections with LIP, LIP suppression diminishes SC activity (Peel et al., 2017). Similar to LIP, increasing the number of distractors decreased overall activity in SC (Zylberberg et al., 2012). In decision-making, context confidence can be correlated to the probability of correct decisions. The confidence is measured through either opt-out trials (as discussed earlier) or self-reporting. Population-level activity shows that decision confidence and decision accuracy covary and SC only encodes decision accuracy and not subjective accuracy (Odegaard et al., 2018). Several studies implicate SC to evidence accumulation (Ratcliff et al., 2003, 2007b; Peel et al., 2017; Reppert et al., 2018; Schall, 2019).
Prefrontal Cortex
The ventromedial prefrontal cortex (vmPFC) plays a crucial role in value processing and preparatory activity by predicting, assigning, and dynamically updating the value to choices (Kable and Glimcher, 2007; Tusche et al., 2010; Henri-Bhargava et al., 2012; Selen et al., 2012; Bault et al., 2019; Shapiro and Grafton, 2020). The vmPFC also serves as an evidence accumulator and tracks the decision confidence (Henri-Bhargava et al., 2012; Vaidya and Fellows, 2015; Bang and Fleming, 2018; Shapiro and Grafton, 2020). Damage to the vmPFC impairs the value assignment to choices while comparing the choices remains unaffected (Fellows, 2006; Lim et al., 2011; Vaidya and Fellows, 2015; Bault et al., 2019). A separate study also found that vmPFC inactivation selectively increased risky choices for poorly rewarded outcomes (Bechara et al., 1999; van Holstein and Floresco, 2020).
The dorsomedial prefrontal cortex (dmPFC) assigns values and effort cost to unattended options for determining better options during exploration (Vaidya and Fellows, 2015; Harris and Lim, 2016; Bault et al., 2019). Additionally, the dlPFC (dorsolateral prefrontal cortex) is involved in evidence accumulation and associated with drift rate. Deactivation of dlPFC neurons using rTMS resulted in lower drift rates and impaired evidence accumulation (Wittkuhn et al., 2018). Continuous theta-burst stimulation (cTBS) of dlPFC (with inhibitory effect) decreased the drift rate only in high coherence trials (Georgiev et al., 2016). All these studies suggest the diverse roles of PFC neurons in processing different value-related parameters and accumulating evidence.
Pre-Supplementary Motor Area (preSMA)
Premotor areas are associated with the decision threshold modulation. For instance, Georgiev et al. (2016) found that cTBS of preSMA reduced the thresholds on trials, emphasizing accuracy. However, this was a counter-intuitive finding under the cortico-striatal theory of threshold modulation (for review, see Bogacz et al., 2010) since this manipulation would be expected to increase the threshold by reducing the excitability of the dorsal STR. The cortico-striatal theory of threshold modulation relies on the idea of the activation of the dorsal STR by the consistent cortical input coding for a particular action. The activation of the STR results in the disinhibition of globus pallidus interna (GPi), which at its resting state inhibits the thalamus and thereby cortex. The disinhibition of GPi results in the action execution by releasing the cortical areas associated with the desired action from inhibition while the other actions continue being inhibited (Forstmann et al., 2008). This functional architecture implements threshold modulation by modulating the likelihood of the desired action plan to be gated by basal ganglia. Thus, the inhibition of preSMA by cTBS would be expected to increase the threshold by reducing the excitability of the dorsal STR (Georgiev et al., 2016). Consistent with the cortico-striatal theory of threshold modulation, Tosun et al. (2017) and Berkay et al. (2018) found that the inhibition of preSMA using cTBS resulted in heightened decision thresholds in tasks where participants were asked to be as fast and as accurate as possible. Berkay et al. (2018) also found that increasing the excitability of the same brain region (by intermittent theta-burst stimulation) resulted in reduced decision thresholds. These findings were consistent with the correlational evidence that showed a relationship between pre-SMA and striatal activity and decision thresholds (Forstmann et al., 2008) and the relationship between pre-SMA-striatal connectivity and decision threshold modulation (Forstmann et al., 2008, 2010). Importantly, these effects were not observed with tDCS (Transcranial Direct-Current Stimulation) (de Hollander et al., 2016), which suggests a weaker efficacy of tDCS in modulating cortical excitability.
Thalamus
We found only one study that correlated thalamic neural activity to DDM parameters. In a two-alternative forced-choice RDM (Turner et al., 2015), recorded fMRI activations and thalamus neural activity corresponded to the drift rate. BOLD activations were higher for the high drift rate trials compared to low drift rate trials. Additionally, this study found that thalamus activity was not indicative of the bias change (Turner et al., 2015).
The Basal Ganglia
Recent studies highlight the role of the BG on DDM parameters. The BG (Figure 2) has several subregions, including STR, subthalamic nucleus (STN), globus pallidus (interna and externa), and substantia nigra (pars reticulata and pars compacta). These nuclei interact via excitatory and inhibitory connectivity, which result in a complex decision parameter control [for review, see (Moustafa et al., 2016a,b)]. The relation of BG on DDM parameters is presented below (for summary, also see Table 1).
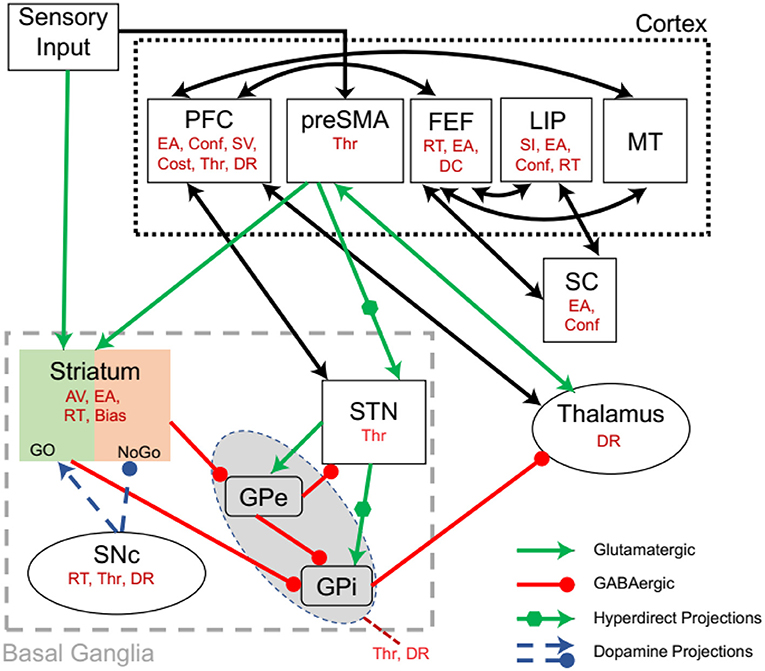
Figure 2. Interactions between the cortico-basal ganglia system showing the effect of an area on DDM parameters (in red). Based on the sensory inputs, the pre-supplementary motor area (pre-SMA) determines competing motor commands. Together sensory and pre-SMA inputs are projected to the striatum (STR). The pre-SMA also projects to subthalamic nucleus (STN) via a hyperdirect pathway. The action of dopamine from SNc modulates the Go and No-Go neurons in the STR. STR inhibits globus pallidus externa (GPe), which in turn inhibits globus pallidus interna (GPi). STR also inhibits GPi and STN has hyperdirect projections to GPi. GPi inhibits the thalamus. The STR-GPi pathways have an overall disinhibiting effect on the thalamus, while the STR-GPe-GPi has an overall inhibitory effect on the thalamus. PFC, Prefrontal Cortex; preSMA, pre-supplementary motor area; FEF, Frontal eye field; LIP, Lateral intraparietal area; MT, Middle temporal area; EA, Evidence accumulation; Conf, Confidence; SV, Stimulus valuation; Thr, Threshold; DR, Drift rate; AV, Action valuation; RT, Reaction time; selection; DC, Decision commitment; SI, Stimulus identity. Modified from Ratcliff and Frank (2012).
Subthalamic Nucleus
The subthalamic nucleus receives inputs from PFC, FEF, primary motor cortex, and pre-SMA while it projects to ventral pallidum and GPi; STN also has bidirectional connections with the GPe (Benarroch, 2008). This connectivity pattern enables the functional coupling of these areas and the execution of sophisticated decision-making features. As the PFC and BG are distinct areas, the interaction between them is primarily controlled through the STN, which delays the BG gating to enable PFC to make the correct decisions and non-impulsive decisions (Frank, 2006; Frank et al., 2007). Additionally, during high conflict decisions, STN and PFC are functionally coupled (Zavala et al., 2013, 2014). The STN and vmPFC bias the decision toward a more valuable choice (Mulder et al., 2014b), and stimulation of STN lowers the decision threshold and terminates the decisions prematurely (Cavanagh et al., 2011).
Globus Pallidus
A study compared the DDM parameters changes in healthy controls, PD with GPi-DBS (Deep Brain Stimulation) ON and PD with GPi-DBS OFF. The authors suggested that the GPi controls decision threshold and drift rate (Kohl et al., 2015). As the decision threshold and drift rate changes were compared between the three groups mentioned above, the exact DDM parameter changes in healthy controls remain unknown. This is further discussed in Section Parkinson's Disease.
The Striatum
The STR is the primary input of the BG. A suppression of the caudate nucleus using muscimol selectively impaired the learning of new sequential motor procedures, while a suppression of the putamen selectively impaired learned motor sequence execution (Miyachi et al., 1997). Selectively inhibiting the D1 receptors in caudate increased saccadic RT for high reward expectation, and D2 receptor inhibition increased RT for small rewards (Nakamura and Hikosaka, 2006a). Interestingly, if stimulation followed the correct response, learning was enhanced (Nakamura and Hikosaka, 2006b). Optogenetic stimulation of the STR in mice performing a visual change detection task resulted in task performance changes by positively biasing the expected or valued visual events (Wang et al., 2018; Zhang et al., 2019). This bias is determined by the interactions between frontoparietal and frontostriatal circuits (Mulder et al., 2012). Another study involving behavioral, pharmacological, optogenetic, electrophysiological, and computational methods explored the role of the anterior dorsal STR in rodent auditory evidence accumulation tasks. The authors found that the anterior dorsal STR directly influences accumulation-based decisions with its neurons encoding for accumulated evidence throughout the decision process (Yartsev et al., 2018; Zhang et al., 2019).
The Substantia Nigra Pars Compacta
The substantia nigra pars compacta (SNc) is a critical area that is involved in dopamine production. SNc projections to STR enable dynamic modulation of DA-based switching between Go and No-Go pathways of the BG during cognitive learning (Frank and O'Reilly, 2006). D2 agonists enhanced the executive performance in low working memory patients while in high working memory participants, this effect was reversed (Gibbs and D'Esposito, 2005). Notably, the D2 antagonist increased Go learning while the opposite effect was seen for the D2 agonist. The effect of D2 agonists on D2 post-synaptic receptors results in the overall inhibition of the No-Go pathway leading to more Go selections and hence, faster RTs (Frank and O'Reilly, 2006). Additional indirect evidence for the SNc involvement comes from the studies on PD with medications. These studies show that the decision threshold, drift rate, early terminations of decisions are impacted by PD medications and are further discussed in Parkinson's Disease.
Brain Disorders
In this section, we discuss how several brain disorders, including Parkinson's disease (PD), ADHD, ASD, OCD, and schizophrenia, and their associated neural damage impact DDM parameters. These diseases were selected as they impact the BG and PFC regions, and prior DDM studies on these patient population groups exist (see Table 2 for the summary).
Parkinson's Disease
Parkinson's disease (PD) is a BG motor disorder characterized by the loss of dopaminergic cells in the SNc. Though the primary impairment in PD is motor dysfunction (Moustafa et al., 2016a), several studies also show cognitive impairments in the disease (Moustafa et al., 2016b). When making decisions, utilizing the prior history of the stimulus to change the bias adequately is essential. Patients with PD are unable to use the previous information to make bias changes (Herz et al., 2016; Perugini et al., 2016). The patients with PD with hallucinations show lower drift rates, higher decision threshold, shorter non-decision time, along with inefficient and inflexible context-dependent evidence accumulation modulation (O'Callaghan et al., 2017). Levodopa medication shortens the premotor time and the motor time, enabling patients with PD to make faster yet inaccurate decisions (Rihet et al., 2002; Hasbroucq et al., 2003).
Huang et al. (2015) studied DDM parameter changes, particularly the drift rate and evidence accumulation in SAT, in medicated and non-medicated patients with PD. The authors presented participants with two versions of the moving-dots task for SAT and task difficulty. By changing the coherence between the moving dots, the task difficulty was altered. RT in all participant groups (healthy control and patients with PD) also increased when the task difficulty was increased. Although patients with PD had more performance errors, patients with PD on medication had more errors and lower drift rates than patients with PD off medication. This suggests that dopamine influences sensory information processing. In the SAT, PD patients off medication showed more errors in speed trials than accuracy trials and had slower RTs when compared to controls. No difference in the RT and errors was observed for patients with PD on medication and controls. A comparison of the DDM parameters revealed that both medicated and unmedicated patients with PD had lower drift rates and higher decision thresholds (Huang et al., 2015).
As discussed above (in section Subthalamic nucleus), STN delays BG gating until PFC makes the correct decision, and STN-DBS forces the decision to terminate early. Similar findings are also reported in patients with PD where STN-DBS and medication led to faster yet inaccurate decisions (Fluchère et al., 2018). RT fractionation showed that DBS reduced both premotor (stimulus onset to EMG onset) and motor time (EMG onset to movement onset; Fluchère et al., 2018). Herz et al. (2018) performed continuous and closed-loop DBS to STN. Patients with PD could adjust the decision threshold for difficult (high conflict) trials only when DBS was performed 400–500 ms after cue onset. Stimulation during this time window also enabled participants to make trial-to-trial adjustments and faster RT. However, for stimulation after 500 ms, the response time remained unaffected (Herz et al., 2018).
Globus pallidus interna -DBS significantly increased RT during response initiation, but the stop signal RT remained unchanged. Compared with controls, the drift rate was lower in both ON and OFF GPi-DBS groups. DBS stimulation also reduced the decision threshold (Kohl et al., 2015). For stop trials utilizing the No-Go pathway, the GPi-DBS-ON condition had a lower response delay. Still, DBS OFF was comparable to that of controls, and GPi-DBS did not affect the speed of conflict resolution (Kohl et al., 2015).
Patients with PD with hallucinations show a lower drift rate, increased decision threshold, and inflexible evidence accumulation modulation (O'Callaghan et al., 2017). Medications increase the speed but at the cost of accuracy. For speed favoring trials, the non-medicated patients with PD show a lower RT. Furthermore, medicated patients show decreased drift rate when compared to the non-medicated patients with PD. In both the medicated and non-medicated patients, the decision threshold is higher compared to controls. Like medication, STN-DBS resulted in faster and inaccurate decisions. PD participants could change the decision threshold when the stimulation was performed within a specific time range. GPi-DBS selectively increased RT for response initiation but not for response termination and reduced the decision threshold. At the same time, the GPi-DBS (both on and off groups) showed a reduced drift rate.
Schizophrenia
Schizophrenia is characterized by altered sensory perceptions, cognitive impairments, and emotional dysregulation (Moustafa et al., 2016c, 2017; Ganguly et al., 2018). These symptoms originated from the dysfunction in the basal ganglia, frontal lobes, and temporal lobes (Buchsbaum, 1990). Post mortem studies in humans unveil increased striatal and globus pallidus volume in basal ganglia in paranoid hallucinatory schizophrenics. At the same time, no such changes were observed in the cortex and white matter (Heckers et al., 1991; Mamah et al., 2007). The higher volumes of these areas result from abnormal maturation that reduces basal ganglia volume during adolescence. Neuroleptic medications differing in their D2 receptor affinity also affect the striatal (caudate and STR) and globus pallidus volume. Therapeutic interventions using typical neuroleptics increased the BG volume. Treatment with atypical neuroleptic (such as clozapine) reduced the BG volumes over 2 years of treatment (Corson et al., 1999).
The drift-diffusion model fits show that patients with schizophrenia favored the accuracy over the speed with impaired learning on negative feedback (Moustafa et al., 2015). Using the reward vs. punishment learning task (in which participants learn to maximize the reward and minimize the punishments in different trials), Moustafa et al. (2015) showed that patients with schizophrenia had a slower RT due to slower encoding and slower motor time. Furthermore, a slower drift rate in punishment trials may be attributed to favoring accuracy, whereby patients were more cautious toward punishments. The authors also demonstrated that the initial bias in patients with schizophrenia and controls was similar, but participants failed to modify the bias based on the prior history of the stimulus (Moustafa et al., 2015). Further elaborating on this study, Fish et al. (2018) showed that the drift rate was lower and non-decision time was higher for patients with schizophrenia and their unaffected siblings. No significant difference in the initial bias and the decision threshold was observed for controls, patients with schizophrenia, and siblings (Fish et al., 2018).
Hierarchical (Bayesian) drift-diffusion model fit for billiard-ball collision timing reporting task suggests that the bias and not the drift rate was the main reason for premature response and impaired response inhibition that led to increased temporal estimation error (Limongi et al., 2018). The tradeoff favoring speed over accuracy conflicts with Moustafa et al. (2015); this may be due to the stricter time constraints imposed by Limongi et al. (2018).
People with schizophrenia favor the accuracy over the speed with slower RT and slower motor time. Additionally, participants showed impaired bias modulation and a lower drift rate for the punishment trials while the decision threshold remained unaffected. Incidentally, stricter timings cause premature response initiation, which may be related to bias but not drift rate.
Autism Spectrum Disorders (ASD)
In ASD, the communication and behavioral impairment symptoms appear early during development. Some of the cognitive deficits in ASD persistently selecting the same choices, sensory hypersensitivity, and impaired interactions with dynamic objects (Sinha et al., 2014). These deficits arise due to abnormal sensory processing, spanning the superior temporal sulcus, fusiform face area, inferior parietal lobe, amygdala (AMY), extra striate body area in the lateral occipitotemporal cortex, PFC, and even BG (Gilbert et al., 2008; McPartland et al., 2011; Prat et al., 2016; Subramanian et al., 2017). It was demonstrated using a small sample study (comprising of only 7 ASD and six controls) that ASD children had 67% more PFC neurons with 79% more neurons in dlPFC and 29% more mPFC neurons when compared to neurotypical controls (Courchesne et al., 2011).
In numerical cognition tasks that involved indicating if an arithmetic equation is valid (2 + 3 = 5) or invalid (2 + 3 = 6), a positive correlation between fMRI activation in DLPFC and dmPFC, and numerical abilities was observed in ASD. At the same time, the healthy controls showed a negative correlation. In this study, accuracy and RT remained unchanged in ASD and controls while the decision thresholds were significantly higher (Karalunas et al., 2018; Iuculano et al., 2020). Pirrone et al. (2017) found that ASD participants showed higher thresholds while accuracy and drift rate were unaffected. Perhaps ASD participants follow a cautious approach by prioritizing accuracy over speed (Pirrone et al., 2017, 2020; Powell et al., 2019). After an error is encountered, RT for subsequent trials was slower. Comparing the post-error RT in 8–12-year-old children with high-functioning autism with controls revealed that the post-error RT is not impaired in high-functioning autism. fMRI analysis revealed that anterior medial PFC showed higher activation for error trials while the controls showed decreased activation (Goldberg et al., 2011). Overall, in ASD, the decision thresholds are higher, and the drift rate and RT remain unchanged.
Obsessive-Compulsive Disorder (OCD)
Obsessive-compulsive disorder is a neuropsychiatric condition characterized by obsessions, intrusive thoughts, and compulsions, which are mental or behavioral acts that are hard to abstain from performing. The symptom profile in patients with OCD has a very diverse range. Therefore, the dimensional approach suggests four different symptom dimensions for OCD, namely symmetry/ordering, hoarding, contamination/cleaning, and obsessions/checking (Mataix-Cols et al., 2005). The literature is limited for studies investigating the decision-making processes of patients with OCD using DDMs. There are two studies conducted with subclinical OCD populations and four studies with patients with OCD. The response times of patients with OCD were found to be longer than healthy controls (Banca et al., 2015; Erhan et al., 2017; Mandali et al., 2019; Marton et al., 2019). The studies were conducted with subclinical OCD populations using the RDM and self-report questionnaires to assess participants' OCD-like features. The positive correlation between the scores gathered by the self-report questionnaire assessing OCD-like features and threshold was reported by both the studies (Erhan and Balci, 2017). The interaction between questionnaire score and drift rate increase with increased stimulus strength was also reported (Hauser et al., 2017).
One study compared patients with OCD and healthy controls using different coherence levels using the random-dot motion tasks (RDM) (Banca et al., 2015). Compared to healthy controls, the patients with OCD had a higher threshold in low signal-to-noise ratio conditions (coherence levels of 0.025 and 0.005) and lower drift rate in high signal-to-noise ratio conditions (coherence levels of 0.45 and 0.7). By using a similar experimental paradigm, Banca et al. (2015) and Marton et al. (2019) reported that patients with OCD show a lesser increase in drift rates as coherence increases (Marton et al., 2019). This finding implies that patients with OCD cannot fully utilize the signal in the stimulus. This study also does not report any significant difference in threshold settings between patients with OCD and healthy controls (Marton et al., 2019). The only research on the pediatric OCD population that used the DDM reported lower drift rates in children with OCD (Erhan et al., 2017). For post-error responses, patients with OCD have higher thresholds than healthy controls. On the other hand, the more pronounced decrease in decision threshold of patients with OCD compared to healthy volunteers was reported in the speed favoring (slow responses were penalized) SAT trials (Banca et al., 2015).
To our knowledge, the only study which does not use the RDM task but uses DDM utilizes the sequential learning task (Mandali et al., 2019). In this task, participants learned to differentiate the stimulus pairs associated with different reward rates. By considering the discrepancies in reward rates between pairs and distance of reward rate from chance levels, the conditions were segregated into conflict and certainty conditions. Patients with OCD performed poorly only in high conflict and high uncertainty conditions. Patients with OCD compared to healthy controls showed a lower drift rate only when the trials are complex, and reward probability is uncertain. In this condition, healthy controls responded quickly and randomly, but patients with OCD required more effort to find the correct answer.
To summarize, patients with OCD show increased RT, lower drift rate in high signal-to-noise ratio conditions, and higher decision threshold for low signal-to-noise ratio conditions. Though patients with OCD can modulate the drift rate for stimulus strength and coherence increase, they are unable to modulate it like controls. In children, lower drift rates and higher decision thresholds for post-error trials are observed.
Attention-Deficit Hyperactivity Disorder (ADHD)
Attention-deficit hyperactivity disorder is a neuropsychiatric condition characterized by the symptom domains of inattention, hyperactivity-impulsivity, or both (American Psychiatric Association, 2013). ADHD is no longer perceived as a childhood disease, as the symptoms persist into adulthood for many patients. Some meta-analyses estimated the prevalence of ADHD among children as 7.2% and among adults as 2.5% (Simon et al., 2009; Thomas et al., 2015). The current theories for a neurobiological understanding of ADHD have a common suggestion for core issues in ADHD as dopamine (DA) alterations (Ziegler et al., 2016). The dopamine deficiency in ADHD is also compatible with the efficacy of stimulant medication (Wilens, 2008). A meta-analysis for functional neuroimaging studies of patients with ADHD reported a hypoactivity in the frontal areas (dorsolateral prefrontal, inferior prefrontal, and orbitofrontal cortex), dorsal anterior midcingulate cortex, superior parietal regions, caudate nucleus, and thalamus (Dickstein et al., 2006). These changes persist into adulthood except for a tendency for the improvement of caudate nucleus pathology (Kasparek et al., 2015).
The majority of studies reviewed by Ziegler et al. (2016) show a reduced drift rate and lower threshold in ADHD (Ziegler et al., 2016). The application of stimulant medication eliminated the differences in drift rates between ADHD and typically developing individuals (Fosco et al., 2017). Patients with ADHD were tested with random-dot motion tasks under the accuracy and speed emphasis (Mulder et al., 2010). Compared to typically developing children, patients with ADHD were faster under the accuracy emphasis, but the response times of these groups were similar in speed trials. DDM analysis revealed that patients with ADHD had lower thresholds in accuracy sessions and higher thresholds in speed sessions. This finding indicates that patients with ADHD do not optimize the speed-accuracy tradeoff as efficiently as control participants. The lower level of flexibility in the threshold setting of patients with ADHD was also reported by another study using a different paradigm (Weigard and Huang-Pollock, 2014). Weigard et al. used an implicit contextual cueing task and found that the thresholds of patients with ADHD were unresponsive to contextual cues. The decision-making and attentional alterations of adults with ADHD diagnosis were summarized in a meta-analysis (Mowinckel et al., 2015). Studies using simple perceptual decisions, reinforcement learning, risky decision-making, temporal discounting, and continuous performance tasks were analyzed by Mowinckel et al. (2015). The most significant effect size was found to reduce the drift rate (−1.62).
Therefore, in ADHD, the drift rate and decision threshold are reduced. The drift rate improved after taking stimulant medication. When performing accuracy trials, the ADHD group, compared to controls, had faster RT, while in speed trials, the two groups showed similar RT. ADHD participants also show impaired threshold adjustments for contextual cue changes.
Discussion
Decision-making involves a selection of an outcome (from available choices) to maximize the rewards. Attempts to study the decision-making led to the development of various theoretical models such as the DDM. The DDM estimates the decision threshold, drift rate, and bias toward a choice based on the observed choices and reaction time. The electrophysiological, imaging, and behavioral experiments trace the DDM parameter computations to several cortical areas such as the FEF, LIP, SC, PFC, and pre-SMA. Neural activity in basal ganglia does not correspond to the determination of these parameters. BG facilitates decisions by choice valuation, assigning a cost to choice, learning an association between the choice and action, assigning the value to actions, signaling reward, task initiation, task termination, goal information presentation, sensory evidence gain modulation, the urgency to commit to a decision, integrating reward history to choices, selecting responses, determining utility, and learning a new task. DDM parameters are thus influenced by the close interactions between the cortical and BG areas. Impairment of any of the structures or a change in functional connectivity can lead to aberrations in decisions and the DDM parameters.
In PD, the loss of dopaminergic neurons in the SNc impact the RT, drift rate, and threshold. However, as SNc is a part of the more extensive BG network, almost all the DDM parameters are impacted. The increased threshold and slower drift rate result in patients being unable to gather enough evidence to cross a decision threshold or reach sufficiently high choice confidence for any of the available choices. This results in the patients continuing to gather evidence and may result in classical motor deficits like freezing of gait (Moustafa et al., 2016a,b). It has been further observed that when the patients are presented with additional visual information like strips on the floor, they can overcome the freezing (Cao et al., 2020). The additional visual cues may alleviate the freezing by reducing the decision threshold. PD medications lower the threshold, which results in decisions terminating without enough evidence gathering. This is consistent with the various cognitive and motor studies suggesting the medication increases the impulsivity in PD. In Schizophrenics, a lower BG volume and reduced BG activation are observed. This results in reduced activation of the STR and reduced functional connectivity between the BG and PFC, which leads to abnormal action valuation (Bernard et al., 2017). The valuation change impairs the patient to integrate the prior trial information and modulate the bias.
As the pathology is arguably limited mainly to the STR, the decision threshold remains largely unaltered. Furthermore, the altered D2 affinity in Schizophrenics disinhibits the NoGo pathways (which is involved in punishment learning), and therefore, the Schizophrenics follow the punishment avoidant approach (Cox et al., 2015). Current neurobiological understanding of OCD implies the serotonergic dysfunction-related hyperactivity of cortico-striato-thalamocortical pathways (Dougherty et al., 2018). A reduction in serotonin level interrupts its inhibitory role in the STR, thalamus, and cortical areas, resulting in hyperactivation of cortico-striato-thalamocortical loops. The cortico-striato-thalamocortical loops involved in the pathophysiology of OCD are particularly indicated as the caudate nucleus, dorsal anterior cingulate cortex, and orbitofrontal cortex (Dougherty et al., 2018). These structures are found to be abnormally active at rest and with symptom provocation (Dougherty et al., 2018). One theory suggests that compulsions are a way to relieve anxiety caused by obsessions. The source of anxiety in patients with OCD is reported to be caused by amygdala hyperactivity (Simon et al., 2010). Another approach to explain OCD symptoms is based on the feeling of incompleteness to counteract “not-just right experiences.” Low-frequency rTMS application to pre-SMA reduced the incompleteness-driven symptoms (Mantovani et al., 2013). In ASD, the increase in PFC neurons (Courchesne et al., 2011) and reduced volumes of BG and thalamus (Estes et al., 2011) result in higher decision thresholds. In ADHD, hypoactive PFC, BG, and thalamus (Zhu et al., 2016) and the impaired functional connectivity between the STR and thalamus (Mills et al., 2012) results in lower decision threshold and drift rates.
Many of the motor and cognitive processes leverage the interaction between cortical and BG areas; structural and/or functional damage can lead to motor and cognitive deficits leading to many disorders. In this review, we highlight how the DDM parameters differ in various disorders. The studies featured in this review present a significant understanding of the neural basis of DDM, including cortical and BG areas. Given the close interactions between frontal cortical regions and BG, it is of utmost importance that both frontal and BG areas are studied together in future DDM studies.
Future studies should be directed toward probing multiple DDM parameters and several BG and PFC areas using microelectrode arrays and finding interactions between all the regions. As discussed above, simulation-based functional inactivation of each of these areas will further refine our understanding of various clinical disorders. DDM parameters provide a robust estimate of decision properties that can be used to estimate cognitive performance quantitatively. These quantitative estimates may enable tracing the latent variables, which allow for making mechanistic inferences about various therapies, disease progression, and efficacies of neurostimulation.
Author Contributions
All authors listed have made a substantial, direct, and intellectual contribution to the work and approved it for publication.
Funding
This work received financial support from the United Arab Emirates University (grant no. CIT 31T129).
Conflict of Interest
The authors declare that the research was conducted in the absence of any commercial or financial relationships that could be construed as a potential conflict of interest.
Publisher's Note
All claims expressed in this article are solely those of the authors and do not necessarily represent those of their affiliated organizations, or those of the publisher, the editors and the reviewers. Any product that may be evaluated in this article, or claim that may be made by its manufacturer, is not guaranteed or endorsed by the publisher.
References
American Psychiatric Association (2013). Diagnostic and Statistical Manual of Mental Disorders (DSM-5®). Arlington, VA: American Psychiatric Pub. doi: 10.1176/appi.books.9780890425596
Balci, F., Simen, P., Niyogi, R., Saxe, A., Hughes, J. A., Holmes, P., et al. (2011). Acquisition of decision making criteria: reward rate ultimately beats accuracy. Atten. Percept. Psychophys. 73, 640–657. doi: 10.3758/s13414-010-0049-7
Banca, P., Vestergaard, M. D., Rankov, V., Baek, K., Mitchell, S., Lapa, T., et al. (2015). Evidence accumulation in obsessive-compulsive disorder: the role of uncertainty and monetary reward on perceptual decision-making thresholds. Neuropsychopharmacology 40, 1192–1202. doi: 10.1038/npp.2014.303
Bang, D., and Fleming, S. M. (2018). Distinct encoding of decision confidence in human medial prefrontal cortex. Proc. Natl. Acad. Sci. U. S. A. 115, 6082–6087. doi: 10.1073/pnas.1800795115
Bault, N., Pellegrino, G. d., Puppi, M., Opolczynski, G., Monti, A., Braghittoni, F., et al. (2019). Dissociation between private and social counterfactual value signals following ventromedial prefrontal cortex damage. J. Cogn. Neurosci. 31, 639–656. doi: 10.1162/jocn_a_01372
Bechara, A., Damasio, H., Damasio, A. R., and Lee, G. P. (1999). Different contributions of the human amygdala and ventromedial prefrontal cortex to decision-making. J. Neurosci. 19, 5473–5481. doi: 10.1523/JNEUROSCI.19-13-05473.1999
Benarroch, E. E (2008). Subthalamic nucleus and its connections: anatomic substrate for the network effects of deep brain stimulation. Neurology 70, 1991–1995. doi: 10.1212/01.wnl.0000313022.39329.65
Berkay, D., Eser, H. Y., Sack, A. T., Cakmak, Y. O., and Balci, F. (2018). The modulatory role of pre-SMA in speed-accuracy tradeoff: a bi-directional TMS study. Neuropsychologia 109, 255–261. doi: 10.1016/j.neuropsychologia.2017.12.031
Bernard, J. A., Russell, C. E., Newberry, R. E., Goen, J. R., and Mittal, V. A. (2017). Patients with schizophrenia show aberrant patterns of basal ganglia activation: evidence from ALE meta-analysis. Neuroimage Clin. 14, 450–463. doi: 10.1016/j.nicl.2017.01.034
Bogacz, R., Brown, E., Moehlis, J., Holmes, P., and Cohen, J. D. (2006). The physics of optimal decision making: a formal analysis of models of performance in two-alternative forced-choice tasks. Psychol. Rev. 113, 700–765. doi: 10.1037/0033-295X.113.4.700
Bogacz, R., Hu, P. T., Holmes, P. J., and Cohen, J. D. (2010). Do humans produce the speed?accuracy trade-off that maximizes reward rate? Q. J. Exp. Psychol. 63, 863–891. doi: 10.1080/17470210903091643
Bond, K., Dunovan, K., and Verstynen, T. (2018). Value-Conflict and Volatility Influence Distinct Decision-Making Processes. Pittsburgh, PA: Department of Psychology and Center for the Neural Basis of Cognition Carnegie Mellon University. doi: 10.32470/CCN.2018.1068-0
Bottemanne, L., and Dreher, J. C. (2019). Vicarious rewards modulate the drift rate of evidence accumulation from the drift diffusion model. Front. Behav. Neurosci. 13:142. doi: 10.3389/fnbeh.2019.00142
Buchsbaum, M. S (1990). Frontal lobes, basal ganglia, temporal lobes–three sites for schizophrenia? Schizophrenia Bullet. 16, 377–378. doi: 10.1093/schbul/16.3.377
Cao, S. S., Yuan, X. Z., Wang, S. H., Taximaimaiti, R., and Wang, X. P. (2020). Transverse strips instead of wearable laser lights alleviate the sequence effect toward a destination in Parkinson's disease patients with freezing of gait. Front. Neurol. 11:838. doi: 10.3389/fneur.2020.00838
Cavanagh, J. F., Wiecki, T. V., Cohen, M. X., Figueroa, C. M., Samanta, J., Sherman, S. J., et al. (2011). Subthalamic nucleus stimulation reverses mediofrontal influence over decision threshold. Nat. Neurosci. 14, 1462–1467. doi: 10.1038/nn.2925
Clay, S. N., Clithero, J. A., Harris, A. M., and Reed, C. L. (2017). Loss aversion reflects information accumulation, not bias: a drift-diffusion model study. Front. Psychol. 8:1708. doi: 10.3389/fpsyg.2017.01708
Corson, P. W., Nopoulos, P., Miller, D. D., Arndt, S., and Andreasen, N. C. (1999). Change in basal ganglia volume over 2 years in patients with schizophrenia: typical versus atypical neuroleptics. Am. J. Psychiatr. 156, 1200–1204.
Courchesne, E., Mouton, P. R., Calhoun, M. E., Semendeferi, K., Ahrens-Barbeau, C., Hallet, M. J., et al. (2011). Neuron number and size in prefrontal cortex of children with autism. J. Am. Med. Assoc. 306, 2001–2010. doi: 10.1001/jama.2011.1638
Cox, S. M. L., Frank, M. J., Larcher, K., Fellows, L. K., Clark, C. A., Leyton, M., et al. (2015). Striatal D1 and D2 signaling differentially predict learning from positive and negative outcomes. NeuroImage 109, 95–101. doi: 10.1016/j.neuroimage.2014.12.070
de Hollander, G., Labruna, L., Sellaro, R., Trutti, A., Colzato, L. S., Ratcliff, R., et al. (2016). Transcranial direct current stimulation does not influence the speed-accuracy tradeoff in perceptual decision-making: evidence from three independent studies. J. Cogn. Neurosci. 28, 1283–1294. doi: 10.1162/jocn_a_00967
Desender, K., Boldt, A., Verguts, T., and Donner, T. H. (2019). Confidence predicts speed-accuracy tradeoff for subsequent decisions. eLife 8:e43499. doi: 10.7554/eLife.43499
Dias, E. C., and Segraves, M. A. (1999). Muscimol-induced inactivation of monkey frontal eye field: effects on visually and memory-guided saccades. J. Neurophysiol. 81, 2191–2214. doi: 10.1152/jn.1999.81.5.2191
Dickstein, S. G., Bannon, K., Xavier Castellanos, F., and Milham, M. P. (2006). The neural correlates of attention deficit hyperactivity disorder: an ALE meta-analysis. J. Child Psychol. Psychiatr. 47, 1051–1062. doi: 10.1111/j.1469-7610.2006.01671.x
Ding, L., and Gold, J. I. (2012). Neural correlates of perceptual decision making before, during, and after decision commitment in monkey frontal eye field. Cereb. Cortex 22, 1052–1067. doi: 10.1093/cercor/bhr178
Dougherty, D. D., Brennan, B. P., Stewart, S. E., Wilhelm, S., Widge, A. S., and Rauch, S. L. (2018). Neuroscientifically informed formulation and treatment planning for patients with obsessive-compulsive disorder. J. Am. Med. Assoc. Psychiatr. 75:1081. doi: 10.1001/jamapsychiatry.2018.0930
Drugowitsch, J., Moreno-Bote, R., Churchland, A. K., Shadlen, M. N., and Pouget, A. (2012). The cost of accumulating evidence in perceptual decision making. J. Neurosci. 32, 3612–3628. doi: 10.1523/JNEUROSCI.4010-11.2012
Dutilh, G., Vandekerckhove, J., Tuerlinckx, F., and Wagenmakers, E. J. (2009). A diffusion model decomposition of the practice effect. Psychon. Bull. Rev. 16, 1026–1036. doi: 10.3758/16.6.1026
Erhan, C., and Balci, F. (2017). Obsessive compulsive features predict cautious decision strategies. Q. J. Exp. Psychol. 70, 179–190. doi: 10.1080/17470218.2015.1130070
Erhan, C., Bulut, G. C., Gokce, S., Ozbas, D., Turkakin, E., Dursun, O. B., et al. (2017). Disrupted latent decision processes in medication-free pediatric OCD patients. J. Affect Disord. 207, 32–37. doi: 10.1016/j.jad.2016.09.011
Estes, A., Shaw, D. W., Sparks, B. F., Friedman, S., Giedd, J. N., Dawson, G., et al. (2011). Basal ganglia morphometry and repetitive behavior in young children with autism spectrum disorder. Autism Res. 4, 212–220. doi: 10.1002/aur.193
Fan, Y., Gold, J. I., and Ding, L. (2018). Ongoing rational calibration of reward-driven perceptual biases. Elife 7:e36018. doi: 10.7554/eLife.36018
Fellows, L. K (2006). Deciding how to decide: ventromedial frontal lobe damage affects information acquisition in multi-attribute decision making. Brain 129, 944–952. doi: 10.1093/brain/awl017
Fish, S., Toumaian, M., Pappa, E., Davies, T. J., Tanti, R., Saville, C. W., et al. (2018). Modelling reaction time distribution of fast decision tasks in schizophrenia: evidence for novel candidate endophenotypes. Psychiatr. Res. 269, 212–220. doi: 10.1016/j.psychres.2018.08.067
Fluchère, F., Burle, B., Vidal, F., Van den Wildenberg, W., Witjas, T., Eusebio, A., et al. (2018). Subthalamic nucleus stimulation, dopaminergic treatment and impulsivity in Parkinson's disease. Neuropsychologia 117, 167–177. doi: 10.1016/j.neuropsychologia.2018.02.016
Forstmann, B. U., Anwander, A., Schafer, A., Neumann, J., Brown, S., Wagenmakers, E. J., et al. (2010). Cortico-striatal connections predict control over speed and accuracy in perceptual decision making. Proc. Natl. Acad. Sci. U. S. A. 107, 15916–15920. doi: 10.1073/pnas.1004932107
Forstmann, B. U., Dutilh, G., Brown, S., Neumann, J., von Cramon, D. Y., Ridderinkhof, K. R., et al. (2008). Striatum and pre-SMA facilitate decision-making under time pressure. Proc. Natl. Acad. Sci. U. S. A. 105, 17538–17542. doi: 10.1073/pnas.0805903105
Fosco, W. D., White, C. N., and Hawk, L. W. Jr. (2017). Acute stimulant treatment and reinforcement increase the speed of information accumulation in children with ADHD. J. Abnorm. Child Psychol. 45, 911–920. doi: 10.1007/s10802-016-0222-0
Frank, M. J (2006). Hold your horses: a dynamic computational role for the subthalamic nucleus in decision making. Neural Netw. 19, 1120–1136. doi: 10.1016/j.neunet.2006.03.006
Frank, M. J., and O'Reilly, R. C. (2006). A mechanistic account of striatal dopamine function in human cognition: psychopharmacological studies with cabergoline and haloperidol. Behav. Neurosci. 120:497. doi: 10.1037/0735-7044.120.3.497
Frank, M. J., Samanta, J., Moustafa, A. A., and Sherman, S. J. (2007). Hold your horses: impulsivity, deep brain stimulation, and medication in parkinsonism. Science 318, 1309–1312. doi: 10.1126/science.1146157
Ganguly, P., Soliman, A., and Moustafa, A. A. (2018). Holistic management of schizophrenia symptoms using pharmacological and non-pharmacological treatment. Front. Public Health 6:166. doi: 10.3389/fpubh.2018.00166
Georgiev, D., Rocchi, L., Tocco, P., Speekenbrink, M., Rothwell, J. C., and Jahanshahi, M. (2016). Continuous theta burst stimulation over the dorsolateral prefrontal cortex and the pre-SMA alter drift rate and response thresholds respectively during perceptual decision-making. Brain Stimul. 9, 601–608. doi: 10.1016/j.brs.2016.04.004
Gibbs, S. E., and D'Esposito, M. (2005). A functional MRI study of the effects of bromocriptine, a dopamine receptor agonist, on component processes of working memory. Psychopharmacology 180, 644–653. doi: 10.1007/s00213-005-0077-5
Gilbert, S. J., Bird, G., Brindley, R., Frith, C. D., and Burgess, P. W. (2008). Atypical recruitment of medial prefrontal cortex in autism spectrum disorders: an fMRI study of two executive function tasks. Neuropsychologia 46, 2281–2291. doi: 10.1016/j.neuropsychologia.2008.03.025
Goldberg, M. C., Spinelli, S., Joel, S., Pekar, J. J., Denckla, M. B., and Mostofsky, S. H. (2011). Children with high functioning autism show increased prefrontal and temporal cortex activity during error monitoring. Dev. Cogn. Neurosci. 1, 47–56. doi: 10.1016/j.dcn.2010.07.002
Gur, E., Duyan, Y. A., Arkan, S., Karson, A., and Balci, F. (2020). Interval timing deficits and their neurobiological correlates in aging mice. Neurobiol. Aging 90, 33–42. doi: 10.1016/j.neurobiolaging.2020.02.021
Hanks, T. D., Mazurek, M. E., Kiani, R., Hopp, E., and Shadlen, M. N. (2011). Elapsed decision time affects the weighting of prior probability in a perceptual decision task. J. Neurosci. 31, 6339–6352. doi: 10.1523/JNEUROSCI.5613-10.2011
Hanks, T. D., and Summerfield, C. (2017). Perceptual decision making in rodents, monkeys, and humans. Neuron 93, 15–31. doi: 10.1016/j.neuron.2016.12.003
Harris, A., and Lim, S. L. (2016). Temporal dynamics of sensorimotor networks in effort-based cost-benefit valuation: early emergence and late net value integration. J. Neurosci. 36, 7167–7183. doi: 10.1523/JNEUROSCI.4016-15.2016
Hasbroucq, T., Tandonnet, C., Micallef-Roll, J., Blin, O., and Possamai, C. A. (2003). An electromyographic analysis of the effect of levodopa on the response time of healthy subjects. Psychopharmacology 165, 313–316. doi: 10.1007/s00213-002-1304-y
Hauser, C. K., Zhu, D., Stanford, T. R., and Salinas, E. (2018). Motor selection dynamics in FEF explain the reaction time variance of saccades to single targets. eLife 7:e33456. doi: 10.7554/eLife.33456
Hauser, T. U., Allen, M., Rees, G., Dolan, R. J., and Consortium, N. (2017). Metacognitive impairments extend perceptual decision making weaknesses in compulsivity. Sci. Rep. 7:6116. doi: 10.1038/s41598-017-06116-z
Heckers, S., Heinsen, H., Heinsen, Y., and Beckmann, H. (1991). Cortex white matter, and basal ganglia in schizophrenia: a volumetric postmortem study. Biol. Psychiatr. 29, 556–566. doi: 10.1016/0006-3223(91)90091-Y
Henri-Bhargava, A., Simioni, A., and Fellows, L. K. (2012). Ventromedial frontal lobe damage disrupts the accuracy, but not the speed, of value-based preference judgments. Neuropsychologia 50, 1536–1542. doi: 10.1016/j.neuropsychologia.2012.03.006
Herz, D. M., Bogacz, R., and Brown, P. (2016). Neuroscience: impaired decision-making in Parkinson's disease. Curr. Biol. 26, R671–R673. doi: 10.1016/j.cub.2016.05.075
Herz, D. M., Little, S., Pedrosa, D. J., Tinkhauser, G., Cheeran, B., Foltynie, T., et al. (2018). Mechanisms underlying decision-making as revealed by deep-brain stimulation in patients with Parkinson's disease. Curr. Biol. 28, 1169–1178.e6. doi: 10.1016/j.cub.2018.02.057
Horga, G., and Abi-Dargham, A. (2019). An integrative framework for perceptual disturbances in psychosis. Nat. Rev. Neurosci. 20, 763–778. doi: 10.1038/s41583-019-0234-1
Huang, Y. T., Georgiev, D., Foltynie, T., Limousin, P., Speekenbrink, M., and Jahanshahi, M. (2015). Different effects of dopaminergic medication on perceptual decision-making in Parkinson's disease as a function of task difficulty and speed–accuracy instructions. Neuropsychologia 75, 577–587. doi: 10.1016/j.neuropsychologia.2015.07.012
Iuculano, T., Padmanabhan, A., Chen, L., Nicholas, J., Mitsven, S., de los Angeles, C., et al. (2020). Neural correlates of cognitive variability in childhood autism and relation to heterogeneity in decision-making dynamics. Dev. Cogn. Neurosci. 2020:100754. doi: 10.1016/j.dcn.2020.100754
Jocham, G., Hunt, L. T., Near, J., and Behrens, T. E. (2012). A mechanism for value-guided choice based on the excitation-inhibition balance in prefrontal cortex. Nat. Neurosci. 15, 960–961. doi: 10.1038/nn.3140
Kable, J. W., and Glimcher, P. W. (2007). The neural correlates of subjective value during intertemporal choice. Nat. Neurosci. 10, 1625–1633. doi: 10.1038/nn2007
Karalunas, S. L., Hawkey, E., Gustafsson, H., Miller, M., Langhorst, M., Cordova, M., et al. (2018). Overlapping and distinct cognitive impairments in attention-deficit/hyperactivity and autism spectrum disorder without intellectual disability. J. Abnormal Child Psychol. 46, 1705–1716. doi: 10.1007/s10802-017-0394-2
Kasparek, T., Theiner, P., and Filova, A. (2015). Neurobiology of ADHD from childhood to adulthood. J. Attention Disord. 19, 931–943. doi: 10.1177/1087054713505322
Kiani, R., and Shadlen, M. N. (2009). Representation of confidence associated with a decision by neurons in the parietal cortex. Science 324, 759–764. doi: 10.1126/science.1169405
Kim, J. N., and Shadlen, M. N. (1999). Neural correlates of a decision in the dorsolateral prefrontal cortex of the macaque. Nat. Neurosci. 2, 176–185. doi: 10.1038/5739
Kohl, S., Aggeli, K., Obeso, I., Speekenbrink, M., Limousin, P., Kuhn, J., et al. (2015). In Parkinson's disease pallidal deep brain stimulation speeds up response initiation but has no effect on reactive inhibition. J. Neurol. 262, 1741–1750. doi: 10.1007/s00415-015-7768-6
Krajbich, I., and Rangel, A. (2011). Multialternative drift-diffusion model predicts the relationship between visual fixations and choice in value-based decisions. Proc. Natl. Acad. Sci. U. S. A. 108, 13852–13857. doi: 10.1073/pnas.1101328108
Leite, F. P., and Ratcliff, R. (2011). What cognitive processes drive response biases? A diffusion model analysis. Judgm. Decision Mak. 6, 651–687.
Lim, K., Wang, W., and Merfeld, D. M. (2017). Unbounded evidence accumulation characterizes subjective visual vertical forced-choice perceptual choice and confidence. J. Neurophysiol. 118, 2636–2653. doi: 10.1152/jn.00318.2017
Lim, S. L., O'Doherty, J. P., and Rangel, A. (2011). The decision value computations in the vmPFC and striatum use a relative value code that is guided by visual attention. J. Neurosci. 31, 13214–13223. doi: 10.1523/JNEUROSCI.1246-11.2011
Limongi, R., Bohaterewicz, B., Nowicka, M., Plewka, A., and Friston, K. J. (2018). Knowing when to stop: aberrant precision and evidence accumulation in schizophrenia. Schizophrenia Res. 197, 386–391. doi: 10.1016/j.schres.2017.12.018
Mamah, D., Wang, L., Barch, D., de Erausquin, G. A., Gado, M., and Csernansky, J. G. (2007). Structural analysis of the basal ganglia in schizophrenia. Schizophrenia Res. 89, 59–71. doi: 10.1016/j.schres.2006.08.031
Mandali, A., Weidacker, K., Kim, S. G., and Voon, V. (2019). The ease and sureness of a decision: evidence accumulation of conflict and uncertainty. Brain 142, 1471–1482. doi: 10.1093/brain/awz013
Mantovani, A., Shubeck, J., Gowatsky, J., Simpson, H., and Greenberg, B. J. C. N. (2013). P 247. incompleteness and harm avoidance in obsessive compulsive disorder: different response to transcranial magnetic stimulation. Clin. Neurophysiol. 124, e182–e183. doi: 10.1016/j.clinph.2013.04.323
Marton, T., Samuels, J., Nestadt, P., Krasnow, J., Wang, Y., Shuler, M., et al. (2019). Validating a dimension of doubt in decision-making: a proposed endophenotype for obsessive-compulsive disorder. PLoS ONE 14:218182. doi: 10.1371/journal.pone.0218182
Mataix-Cols, D., do Rosario-Campos, M. C., and Leckman, J. F. (2005). A multidimensional model of obsessive-compulsive disorder. Am. J. Psychiatr. 162, 228–238. doi: 10.1176/appi.ajp.162.2.228
McGovern, D. P., Hayes, A., Kelly, S. P., and O'Connell, R. G. (2018). Reconciling age-related changes in behavioural and neural indices of human perceptual decision-making. Nat. Hum. Beha v. 2, 955–966. doi: 10.1038/s41562-018-0465-6
McPartland, J. C., Coffman, M., and Pelphrey, K. A. (2011). Recent advances in understanding the neural bases of autism spectrum disorder. Curr. Opin. Pediatr. 23, 628–632. doi: 10.1097/MOP.0b013e32834cb9c9
Meister, M. L., Hennig, J. A., and Huk, A. C. (2013). Signal multiplexing and single-neuron computations in lateral intraparietal area during decision-making. J. Neurosci. 33, 2254–2267. doi: 10.1523/JNEUROSCI.2984-12.2013
Mills, K., Bathula, D., Costa Dias, T., Iyer, S., Fenesy, M., Musser, E., et al. (2012). Altered cortico-striatal–thalamic connectivity in relation to spatial working memory capacity in children with ADHD. Front. Psychiatr. 3:2. doi: 10.3389/fpsyt.2012.00002
Miyachi, S., Hikosaka, O., Miyashita, K., Karadi, Z., and Rand, M. K. (1997). Differential roles of monkey striatum in learning of sequential hand movement. Exp. Brain Res. 115, 1–5. doi: 10.1007/PL00005669
Moustafa, A. A., Chakravarthy, S., Phillips, J. R., Crouse, J. J., Gupta, A., Frank, M. J., et al. (2016b). Interrelations between cognitive dysfunction and motor symptoms of Parkinson's disease: behavioral and neural studies. Rev. Neurosci. 27, 535–548. doi: 10.1515/revneuro-2015-0070
Moustafa, A. A., Chakravarthy, S., Phillips, J. R., Gupta, A., Keri, S., Polner, B., et al. (2016a). Motor symptoms in Parkinson's disease: a unified framework. Neurosci. Biobehav. Rev. 68, 727–740. doi: 10.1016/j.neubiorev.2016.07.010
Moustafa, A. A., Garami, J. K., Mahlberg, J., Golembieski, J., Keri, S., Misiak, B., et al. (2016c). Cognitive function in schizophrenia: conflicting findings and future directions. Rev. Neurosci. 27, 435–448. doi: 10.1515/revneuro-2015-0060
Moustafa, A. A., Kéri, S., Somlai, Z., Balsdon, T., Frydecka, D., Misiak, B., et al. (2015). Drift diffusion model of reward and punishment learning in schizophrenia: modeling and experimental data. Behav. Brain Res. 291, 147–154. doi: 10.1016/j.bbr.2015.05.024
Moustafa, A. A., Misiak, B., and Frydecka, D. (2017). Neurocomputational models of schizophrenia. Computati. Models Brain Behav. 73:ch6. doi: 10.1002/9781119159193.ch6
Mowinckel, A. M., Pedersen, M. L., Eilertsen, E., and Biele, G. (2015). A meta-analysis of decision-making and attention in adults with ADHD. J. Atten. Disord. 19, 355–367. doi: 10.1177/1087054714558872
Mulder, M. J., Boekel, W., Ratcliff, R., and Forstmann, B. U. (2014b). Cortico-subthalamic connection predicts individual differences in value-driven choice bias. Brain Struct. Funct. 219, 1239–1249. doi: 10.1007/s00429-013-0561-3
Mulder, M. J., Bos, D., Weusten, J. M., van Belle, J., van Dijk, S. C., Simen, P., et al. (2010). Basic impairments in regulating the speed-accuracy tradeoff predict symptoms of attention-deficit/hyperactivity disorder. Biol. Psychiatr. 68, 1114–1119. doi: 10.1016/j.biopsych.2010.07.031
Mulder, M. J., Keuken, M. C., van Maanen, L., Boekel, W., Forstmann, B. U., and Wagenmakers, E. J. (2013). The speed and accuracy of perceptual decisions in a random-tone pitch task. Attent. Percept. Psychophys. 75, 1048–1058. doi: 10.3758/s13414-013-0447-8
Mulder, M. J., van Maanen, L., and Forstmann, B. U. (2014a). Perceptual decision neurosciences – a model-based review. Neuroscience 277, 872–884. doi: 10.1016/j.neuroscience.2014.07.031
Mulder, M. J., Wagenmakers, E. J., Ratcliff, R., Boekel, W., and Forstmann, B. U. (2012). Bias in the brain: a diffusion model analysis of prior probability and potential payoff. J. Neurosci. 32, 2335–2343. doi: 10.1523/JNEUROSCI.4156-11.2012
Murd, C., Moisa, M., Grueschow, M., Polania, R., and Ruff, C. C. (2020). Causal contributions of human frontal eye fields to distinct aspects of decision formation. Sci. Rep. 10:7317. doi: 10.1038/s41598-020-64064-7
Nakamura, K., and Hikosaka, O. (2006a). Role of dopamine in the primate caudate nucleus in reward modulation of saccades. J. Neurosci. 26, 5360–5369. doi: 10.1523/JNEUROSCI.4853-05.2006
Nakamura, K., and Hikosaka, O. (2006b). Facilitation of saccadic eye movements by postsaccadic electrical stimulation in the primate caudate. J. Neurosci. 26, 12885–12895. doi: 10.1523/JNEUROSCI.3688-06.2006
O'Callaghan, C., Hall, J. M., Tomassini, A., Muller, A. J., Walpola, I. C., Moustafa, A. A., et al. (2017). Visual hallucinations are characterized by impaired sensory evidence accumulation: insights from hierarchical drift diffusion modeling in Parkinson's disease. Biol. Psychiatr. 2, 680–688. doi: 10.1016/j.bpsc.2017.04.007
O'Connell, R. G., Shadlen, M. N., Wong-Lin, K., and Kelly, S. P. (2018). Bridging neural and computational viewpoints on perceptual decision-making. Trends Neurosci. 41, 838–852. doi: 10.1016/j.tins.2018.06.005
Odegaard, B., Grimaldi, P., Cho, S. H., Peters, M. A. K., Lau, H., and Basso, M. A. (2018). Superior colliculus neuronal ensemble activity signals optimal rather than subjective confidence. Proc. Natl. Acad. Sci. U. S. A. 115, E1588–E1597. doi: 10.1073/pnas.1711628115
Palmer, J., Huk, A. C., and Shadlen, M. N. (2005). The effect of stimulus strength on the speed and accuracy of a perceptual decision. J. Vis. 5, 376–404. doi: 10.1167/5.5.1
Peel, T. R., Dash, S., Lomber, S. G., and Corneil, B. D. (2017). Frontal eye field inactivation diminishes superior colliculus activity, but delayed saccadic accumulation governs reaction time increases. J. Neurosci. 37, 11715–11730. doi: 10.1523/JNEUROSCI.2664-17.2017
Perugini, A., Ditterich, J., and Basso, M. A. (2016). Patients with Parkinson's disease show impaired use of priors in conditions of sensory uncertainty. Curr. Biol. 26, 1902–1910. doi: 10.1016/j.cub.2016.05.039
Philiastides, M. G., Heekeren, H. R., and Sajda, P. (2014). Human scalp potentials reflect a mixture of decision-related signals during perceptual choices. J. Neurosci. 34, 16877–16889. doi: 10.1523/JNEUROSCI.3012-14.2014
Pirrone, A., Dickinson, A., Gomez, R., Stafford, T., and Milne, E. (2017). Understanding perceptual judgment in autism spectrum disorder using the drift diffusion model. Neuropsychology 31:173. doi: 10.1037/neu0000320
Pirrone, A., Johnson, I., Stafford, T., and Milne, E. (2020). A diffusion model decomposition of orientation discrimination in children with Autism Spectrum Disorder (ASD). Eur. J. Dev. Psychol. 17, 213–230. doi: 10.1080/17405629.2018.1561364
Powell, G., Jones, C. R., Hedge, C., Charman, T., Happé, F., Simonoff, E., et al. (2019). Face processing in autism spectrum disorder re-evaluated through diffusion models. Neuropsychology 33:445. doi: 10.1037/neu0000524
Prat, C. S., Stocco, A., Neuhaus, E., and Kleinhans, N. M. (2016). Basal ganglia impairments in autism spectrum disorder are related to abnormal signal gating to prefrontal cortex. Neuropsychologia 91, 268–281. doi: 10.1016/j.neuropsychologia.2016.08.007
Purcell, B. A., Heitz, R. P., Cohen, J. Y., Schall, J. D., Logan, G. D., and Palmeri, T. J. (2010). Neurally constrained modeling of perceptual decision making. Psychol. Rev. 117, 1113–1143. doi: 10.1037/a0020311
Purcell, B. A., and Palmeri, T. J. (2017). Relating accumulator model parameters and neural dynamics. J. Math. Psychol. 76, 156–171. doi: 10.1016/j.jmp.2016.07.001
Purcell, B. A., Schall, J. D., Logan, G. D., and Palmeri, T. J. (2012). From salience to saccades: multiple-alternative gated stochastic accumulator model of visual search. J. Neurosci. 32, 3433–3446. doi: 10.1523/JNEUROSCI.4622-11.2012
Rao, V., DeAngelis, G. C., and Snyder, L. H. (2012). Neural correlates of prior expectations of motion in the lateral intraparietal and middle temporal areas. J. Neurosci. 32, 10063–10074. doi: 10.1523/JNEUROSCI.5948-11.2012
Ratcliff, R (1978). A theory of memory retrieval. Psychol. Rev. 85:59. doi: 10.1037/0033-295X.85.2.59
Ratcliff, R., Cherian, A., and Segraves, M. (2003). A comparison of macaque behavior and superior colliculus neuronal activity to predictions from models of two-choice decisions. J. Neurophysiol. 90, 1392–1407. doi: 10.1152/jn.01049.2002
Ratcliff, R., and Childers, R. (2015). Individual differences and fitting methods for the two-choice diffusion model of decision making. Decision 2:237. doi: 10.1037/dec0000030
Ratcliff, R., and Frank, M. J. (2012). Reinforcement-based decision making in corticostriatal circuits: mutual constraints by neurocomputational and diffusion models. Neural Computat. 24, 1186–1229. doi: 10.1162/NECO_a_00270
Ratcliff, R., Hasegawa, Y. T., Hasegawa, R. P., Smith, P. L., and Segraves, M. A. (2007b). Dual diffusion model for single-cell recording data from the superior colliculus in a brightness-discrimination task. J. Neurophysiol. 97, 1756–1774. doi: 10.1152/jn.00393.2006
Ratcliff, R., and McKoon, G. (2008). The diffusion decision model: theory and data for two-choice decision tasks. Neural Computat. 20, 873–922. doi: 10.1162/neco.2008.12-06-420
Ratcliff, R., Smith, P. L., Brown, S. D., and McKoon, G. (2016). Diffusion decision model: current issues and history. Trends Cogn. Sci. 20, 260–281. doi: 10.1016/j.tics.2016.01.007
Ratcliff, R., Thapar, A., and McKoon, G. (2007a). Application of the diffusion model to two-choice tasks for adults 75–90 years old. Psychol. Aging 22:56. doi: 10.1037/0882-7974.22.1.56
Reppert, T. R., Servant, M., Heitz, R. P., and Schall, J. D. (2018). Neural mechanisms of speed-accuracy tradeoff of visual search: saccade vigor, the origin of targeting errors, and comparison of the superior colliculus and frontal eye field. J. Neurophysiol. 120, 372–384. doi: 10.1152/jn.00887.2017
Resulaj, A., Kiani, R., Wolpert, D. M., and Shadlen, M. N. (2009). Changes of mind in decision-making. Nature 461, 263–266. doi: 10.1038/nature08275
Riesel, A., Kathmann, N., and Klawohn, J. (2019). Flexibility of error-monitoring in obsessive-compulsive disorder under speed and accuracy instructions. J. Abnorm. Psychol. 128, 671–677. doi: 10.1037/abn0000463
Rihet, P., Possamai, C. A., Micallef-Roll, J., Blin, O., and Hasbroucq, T. (2002). Dopamine and human information processing: a reaction-time analysis of the effect of levodopa in healthy subjects. Psychopharmacology 163, 62–67. doi: 10.1007/s00213-002-1127-x
Salthouse, T. A (1996). The processing-speed theory of adult age differences in cognition. Psychol. Rev. 103, 403–428. doi: 10.1037/0033-295X.103.3.403
Schall, J. D (2019). Accumulators, neurons, and response time. Trends Neurosci. 42, 848–860. doi: 10.1016/j.tins.2019.10.001
Selen, L. P., Shadlen, M. N., and Wolpert, D. M. (2012). Deliberation in the motor system: reflex gains track evolving evidence leading to a decision. J. Neurosci. 32, 2276–2286. doi: 10.1523/JNEUROSCI.5273-11.2012
Shadlen, M. N., and Newsome, W. T. (2001). Neural basis of a perceptual decision in the parietal cortex (area LIP) of the rhesus monkey. J. Neurophysiol. 86, 1916–1936. doi: 10.1152/jn.2001.86.4.1916
Shapiro, A. D., and Grafton, S. T. (2020). Subjective value then confidence in human ventromedial prefrontal cortex. PLoS ONE 15:e0225617. doi: 10.1371/journal.pone.0225617
Shushruth, S., Mazurek, M., and Shadlen, M. N. (2018). Comparison of decision-related signals in sensory and motor preparatory responses of neurons in Area LIP. J. Neurosci. 38, 6350–6365. doi: 10.1523/JNEUROSCI.0668-18.2018
Simen, P., Contreras, D., Buck, C., Hu, P., Holmes, P., and Cohen, J. D. (2009). Reward rate optimization in two-alternative decision making: empirical tests of theoretical predictions. J. Exp. Psychol. Hum. Percept. Perform. 35:1865. doi: 10.1037/a0016926
Simon, D., Kaufmann, C., Müsch, K., Kischkel, E., and Kathmann, N. (2010). Fronto-striato-limbic hyperactivation in obsessive-compulsive disorder during individually tailored symptom provocation. Psychophysiology. 47, 728–738. doi: 10.1111/j.1469-8986.2010.00980.x
Simon, V., Czobor, P., Bálint, S., Mészáros, A., and Bitter, I. J. T. B.J. o. P. (2009). Prevalence and correlates of adult attention-deficit hyperactivity disorder: meta-analysis. Br. J. Psychiatr. 194, 204–211. doi: 10.1192/bjp.bp.107.048827
Sinha, P., Kjelgaard, M. M., Gandhi, T. K., Tsourides, K., Cardinaux, A. L., Pantazis, D., et al. (2014). Autism as a disorder of prediction. Proc. Natl. Acad. Sci. U. S. A. 111, 15220–15225. doi: 10.1073/pnas.1416797111
Spieser, L., Servant, M., Hasbroucq, T., and Burle, B. (2017). Beyond decision! Motor contribution to speed–accuracy trade-off in decision-making. Psychon. Bullet. Rev. 24, 950–956. doi: 10.3758/s13423-016-1172-9
Stafford, T., Pirrone, A., Croucher, M., and Krystalli, A. (2020). Quantifying the benefits of using decision models with response time and accuracy data. Behav. Res. Methods. 52, 2142–2155. doi: 10.3758/s13428-020-01372-w
Starns, J. J., Ratcliff, R., and White, C. N. (2012). Diffusion model drift rates can be influenced by decision processes: an analysis of the strength-based mirror effect. J. Exp. Psychol. Learn. Mem. Cogn. 38:1137. doi: 10.1037/a0028151
Stone, M (1960). Models for choice-reaction time. Psychometrika 25, 251–260. doi: 10.1007/BF02289729
Subramanian, K., Brandenburg, C., Orsati, F., Soghomonian, J. J., Hussman, J. P., and Blatt, G. J. (2017). Basal ganglia and autism - a translational perspective. Autism Res. 10, 1751–1775. doi: 10.1002/aur.1837
Theisen, M., Lerche, V., von Krause, M., and Voss, A. (2020). Age differences in diffusion model parameters: a meta-analysis. Psychol. Res. 85, 2012–2021. doi: 10.1007/s00426-020-01371-8
Thomas, R., Sanders, S., Doust, J., Beller, E., and Glasziou, P. J. P. (2015). Prevalence of attention-deficit/hyperactivity disorder: a systematic review and meta-analysis. Pediatrics 135, e994–e1001. doi: 10.1542/peds.2014-3482
Tosun, T., Berkay, D., Sack, A. T., Çakmak, Y. Ö., and Balci, F. (2017). Inhibition of pre–supplementary motor area by continuous theta burst stimulation leads to more cautious decision-making and more efficient sensory evidence integration. J. Cogn. Neurosci. 29, 1433–1444. doi: 10.1162/jocn_a_01134
Tremel, J. J., and Wheeler, M. E. (2015). Content-specific evidence accumulation in inferior temporal cortex during perceptual decision-making. Neuroimage 109, 35–49. doi: 10.1016/j.neuroimage.2014.12.072
Turner, B. M., Van Maanen, L., and Forstmann, B. U. (2015). Informing cognitive abstractions through neuroimaging: the neural drift diffusion model. Psychol. Rev. 122:312. doi: 10.1037/a0038894
Tusche, A., Bode, S., and Haynes, J. D. (2010). Neural responses to unattended products predict later consumer choices. J. Neurosci. 30, 8024–8031. doi: 10.1523/JNEUROSCI.0064-10.2010
Usher, M., and McClelland, J. L. (2001). The time course of perceptual choice: the leaky, competing accumulator model. Psychol. Rev. 108, 550–592. doi: 10.1037/0033-295X.108.3.550
Vaidya, A. R., and Fellows, L. K. (2015). Testing necessary regional frontal contributions to value assessment and fixation-based updating. Nat. Commun. 6:10120. doi: 10.1038/ncomms10120
van Holstein, M., and Floresco, S. B. (2020). Dissociable roles for the ventral and dorsal medial prefrontal cortex in cue-guided risk/reward decision making. Neuropsychopharmacology 45, 683–693. doi: 10.1038/s41386-019-0557-7
Vandekerckhove, J., and Tuerlinckx, F. (2008). Diffusion model analysis with MATLAB: a DMAT primer. Behav. Res. Methods 40, 61–72. doi: 10.3758/BRM.40.1.61
Wagenmakers, E. J., van der Maas, H. L., and Grasman, R. P. (2007). An EZ-diffusion model for response time and accuracy. Psychon. Bull. Rev. 14, 3–22. doi: 10.3758/BF03194023
Wald, A., and Wolfowitz, J. (1948). Optimum character of the sequential probability ratio test. Ann. Math. Statist. 19, 326–339. doi: 10.1214/aoms/1177730197
Wang, L., Rangarajan, K. V., Gerfen, C. R., and Krauzlis, R. J. (2018). Activation of striatal neurons causes a perceptual decision bias during visual change detection in mice. Neuron 97, 1369–1381.e5. doi: 10.1016/j.neuron.2018.01.049
Weigard, A., and Huang-Pollock, C. (2014). A diffusion modeling approach to understanding contextual cueing effects in children with ADHD. J. Child Psychol. Psychiatr. Allied Discipl. 55, 1336–1344. doi: 10.1111/jcpp.12250
Westbrook, A., Lamichhane, B., and Braver, T. (2019). The subjective value of cognitive effort is encoded by a domain-general valuation network. J. Neurosci. 39:3934. doi: 10.1523/JNEUROSCI.3071-18.2019
Wiecki, T. V., Sofer, I., and Frank, M. J. (2013). HDDM: hierarchical bayesian estimation of the drift-diffusion model in python. Front. Neuroinformat. 7:14. doi: 10.3389/fninf.2013.00014
Wilens, T. E (2008). Effects of methylphenidate on the catecholaminergic system in attention-deficit/hyperactivity disorder. J. Clin. Psychopharmacol. 28, S46–S53. doi: 10.1097/JCP.0b013e318173312f
Wittkuhn, L., Eppinger, B., Bartsch, L. M., Thurm, F., Korb, F. M., and Li, S. C. (2018). Repetitive transcranial magnetic stimulation over dorsolateral prefrontal cortex modulates value-based learning during sequential decision-making. NeuroImage 167, 384–395. doi: 10.1016/j.neuroimage.2017.11.057
Wong, A. L., Haith, A. M., and Krakauer, J. W. (2015). Motor planning. Neuroscientist 21, 385–398. doi: 10.1177/1073858414541484
Yartsev, M. M., Hanks, T. D., Yoon, A. M., and Brody, C. D. (2018). Causal contribution and dynamical encoding in the striatum during evidence accumulation. Elife 7:e34929. doi: 10.7554/eLife.34929.027
Zavala, B., Brittain, J. S., Jenkinson, N., Ashkan, K., Foltynie, T., Limousin, P., et al. (2013). Subthalamic nucleus local field potential activity during the Eriksen flanker task reveals a novel role for theta phase during conflict monitoring. J. Neurosci. 33, 14758–14766. doi: 10.1523/JNEUROSCI.1036-13.2013
Zavala, B. A., Tan, H., Little, S., Ashkan, K., Hariz, M., Foltynie, T., et al. (2014). Midline frontal cortex low-frequency activity drives subthalamic nucleus oscillations during conflict. J. Neurosci. 34, 7322–7333. doi: 10.1523/JNEUROSCI.1169-14.2014
Zhang, Z., Cheng, H., Lin, Z., and Yang, T. (2019). A sequence learning model for decision making in the brain. bioRxiv 555862. doi: 10.1101/555862
Zhou, Y., and Freedman, D. J. (2019). Posterior parietal cortex plays a causal role in perceptual and categorical decisions. Science 365:180. doi: 10.1126/science.aaw8347
Zhu, Y., Yang, D., Ji, W., Huang, T., Xue, L., Jiang, X., et al. (2016). The relationship between neurocircuitry dysfunctions and attention deficit hyperactivity disorder: a review. BioMed Res. Int. 2016:3821579. doi: 10.1155/2016/3821579
Ziegler, S., Pedersen, M. L., Mowinckel, A. M., and Biele, G. (2016). Modelling ADHD: a review of ADHD theories through their predictions for computational models of decision-making and reinforcement learning. Neurosci. Biobehav. Rev. 71, 633–656. doi: 10.1016/j.neubiorev.2016.09.002
Zoltowski, D. M., Latimer, K. W., Yates, J. L., Huk, A. C., and Pillow, J. W. (2019). Discrete stepping and nonlinear ramping dynamics underlie spiking responses of LIP neurons during decision-making. Neuron 102, 1249–1258.e10. doi: 10.1016/j.neuron.2019.04.031
Keywords: drift diffusion models, decision making, prefrontal cortex, basal ganglia, neural mechanism
Citation: Gupta A, Bansal R, Alashwal H, Kacar AS, Balci F and Moustafa AA (2022) Neural Substrates of the Drift-Diffusion Model in Brain Disorders. Front. Comput. Neurosci. 15:678232. doi: 10.3389/fncom.2021.678232
Received: 09 March 2021; Accepted: 25 November 2021;
Published: 07 January 2022.
Edited by:
Spase Petkoski, INSERM U1106 Institut de Neurosciences des Systèmes, FranceReviewed by:
Kenji Morita, The University of Tokyo, JapanJyotika Bahuguna, Helmholtz Association of German Research Centres (HZ), Germany
Copyright © 2022 Gupta, Bansal, Alashwal, Kacar, Balci and Moustafa. This is an open-access article distributed under the terms of the Creative Commons Attribution License (CC BY). The use, distribution or reproduction in other forums is permitted, provided the original author(s) and the copyright owner(s) are credited and that the original publication in this journal is cited, in accordance with accepted academic practice. No use, distribution or reproduction is permitted which does not comply with these terms.
*Correspondence: Hany Alashwal, aGFsYXNod2FsQHVhZXUuYWMuYWU=