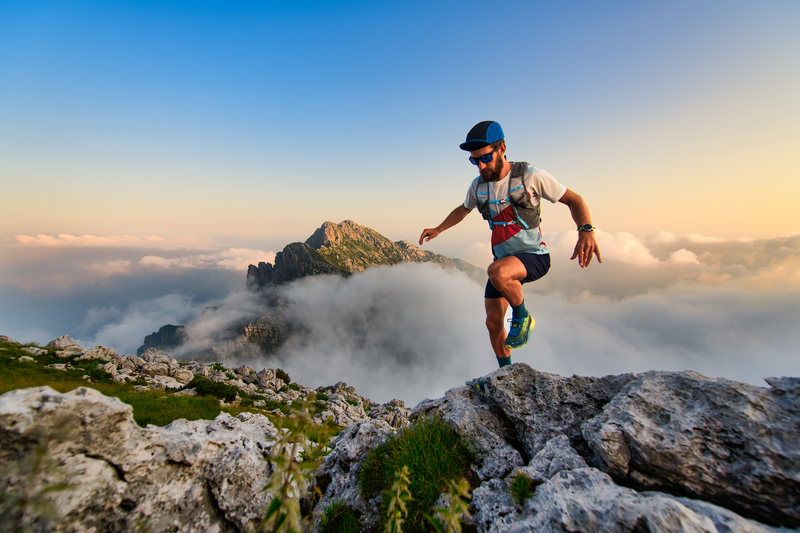
94% of researchers rate our articles as excellent or good
Learn more about the work of our research integrity team to safeguard the quality of each article we publish.
Find out more
ORIGINAL RESEARCH article
Front. Comput. Neurosci. , 16 October 2018
Volume 12 - 2018 | https://doi.org/10.3389/fncom.2018.00061
This article is part of the Research Topic Brain Function: From Experimental and Computational Neuroscience to Brain Engineering View all 13 articles
A correction has been applied to this article in:
Corrigendum: Recording Neural Activity Based on Surface Plasmon Resonance by Optical Fibers-A Computational Analysis
An all optical, non-destructive method for monitoring neural activity has been proposed and its performance in detection has been analyzed computationally. The proposed method is based on excitation of Surface Plasmon Resonance (SPR) through the structure of optical fibers. The sensor structure consists of a multimode optical fiber where, the cladding of fiber has been removed and thin film of gold structure has been deposited on the surface. Impinging the laser light with appropriate wavelength inside the fiber and based on the total internal reflection, the evanescent wave will excite surface plasmons in the gold thin film. The absorption of light by surface plasmons in the gold structure is severely dependent on the dielectric properties at its vicinity. The electrical activity of neural cells (action potential) can modulate the dielectric properties at its vicinity and hence can modify the absorption of light inside the optical fiber. We have computationally analyzed the performance of the proposed sensor with different available geometries using Finite Element Method (FEM). In this regard, we have shown that the optical response of proposed sensor will track the action potential of the neuron at its vicinity. Based on different geometrical structure, the sensor has absorption in different regions of visible spectrum.
Neurons as the main building blocks of central (brain and spinal cord) and peripheral nervous system are well known. Processing and communication of data in various parts of nervous system are performed basically through the propagating variation of transmembrane electric potential of neurons, called action potentials. The electrophysiological function of neurons is the core of memory, cognition, movement, and autonomic functions. Detection, monitoring and recording the electrophysiological activity of neuron(s) are the most important subjects of neurophysiology. The basic therapeutic studies on the various types of neurological disorders including Alzheimer, Parkinson, Multiple Sclerosis (MS), and traumatic brain injuries requires the real time monitoring of the neural activity (Kempuraj et al., 2017). The fundamental studies on the different treatments for the neuron based disorders such as pharmacological treatments, excitation with electric/magnetic fields and stem cell therapy are dependent to the devices in which the electrical activity of the neurons are monitored in real time (Eissa et al., 2018; Tekieh et al., 2018; Zhong et al., 2018). In addition, the advanced studies on the performance of the brain in cognitive neuroscience applications are from demanding areas of monitoring the electrical signaling and activity in different regions of brain (Chen et al., 2016; Hindriks et al., 2016; Sokolov et al., 2016; Deadwyler et al., 2017). The spatial resolution, sensitivity, signal to noise ratio and biocompatibility are the key constraints of different methods in this regard.
Due to the importance of neuron's electrophysiological activity, there have been different proposed techniques of recording the electrical activities and stimulating the neurons. Electrical recording of neural activity using multi electrode structures with different geometries of sharp needles, planar electrodes, and flexible substrates are performed with microelectronics fabricated structures(Gunasekera et al., 2015; Maccione et al., 2015; Chen et al., 2017). Based on the intra/extra cellular recording, geometry of the electrode is an important parameter to consider. Wires and micropipette structures are intruding the cell structure for intracellular recordings, while the planar structures are designed for extracellular recordings (Chen et al., 2017). From material point of view, there have been different types of material (e.g., silicon, platinum, tungsten and gold) applied for fabrication of electrodes. To select the electrode's material and geometry the electrical impedance, signal to noise ratio and biocompatibility should be considered. With the advent of nanostructures and based on their motivating electrical and mechanical properties, there have been different approaches based on the application of nanostructures in extra/intra cellular recordings. Different 1D/2D nanostructures including carbon nanotubes (CNT) and graphene have been applied in fabrication of electrodes (Hanein and Bareket-Keren, 2013; Fabbro et al., 2016; Park et al., 2016). Application of nanostructured electrodes will have the fundamental benefits including higher surface area, electrical conductivity and enhanced adhesion of cell to the substrate (Marchesan et al., 2017; Scaini and Ballerini, 2018). It has been shown that, carbon nanotubes have the potential to mimic the intracellular electrodes. Considering neural prosthesis, CNT structures have also shown the great potential for neural cell cultivation on their surface (Cellot et al., 2011; Giugliano et al., 2012). Graphene based structures have been applied as substrates for stimulation, considering their good adhesion, biocompatibility and great charge injection capacity (Park et al., 2018). Although very applicable, but there are some limitations in the electrical stimulation and recording of action potential. Artifacts in electrical stimulation, electromagnetic interference, and existing tissue damages, have directed the trend to the non-electrical stimulation and recording. There have been various techniques proposed based on exploiting magnetic field (Barry et al., 2016), acoustic waves (Neely et al., 2018) and optics (Kralj et al., 2011; Lu et al., 2018). Different frequency regions and intensity of above mentioned fields will control the penetration depth consequently.
Optical methods of recording neural activity and stimulation of neurons are performed using different techniques. Infrared excitation (Wells et al., 2005; Shapiro et al., 2012), voltage sensitive fluorescent tags (Kulkarni and Miller, 2017; Liu et al., 2017; Nixima et al., 2017), genetically encoding opsins (Chow et al., 2012), and surface plasmon resonance techniques (Kim et al., 2008) are from well-known methods in this regard. Fluorescence-based techniques using voltage sensitive dyes suffer from different problems and difficulties including bleaching, toxicity, labeling procedures and the instrumentation requirements.
Surface plasmon resonance, the collective resonant oscillation of electrons at the interface of metal and dielectric in response to excitation light, has been exploited in different sensing applications (Csáki et al., 2018; Hinman et al., 2018; Lertvachirapaiboon et al., 2018). The maximum absorption of energy from incident light will be occurred in a specific wavelength which is strictly dependent to the dielectric properties of interface. From application point of view, the absorption properties in SPR sensors could provide the base for monitoring the variation in the refractive index of the interface. The detection could be done based on different methods such as wavelength, intensity and angular techniques. As the electric potential can modify the dielectric properties of the ionic liquid, the SPR has been applied along with electrochemical impedance spectroscopy (EIS) techniques (Polonschii et al., 2014). Depending on its fast, label free and high sensitivity response, SPR has been used to monitor neural activity (Kim et al., 2008). The prism based technique has been applied to monitor the activity of nerves in vitro. Using gold nanoparticles, the neural activity has been monitored in vitro (Zhang et al., 2009). Optical fibers are great candidates for transferring light inside the body. The main mechanism of light trapping inside the fiber optics is total internal reflection, the same phenomena applied in prism based technique for excitation of surface plasmon modes in the metallic thin film. Based on their size and geometry, the optical fibers can be easily applied for in vivo applications. The fiber based SPR sensors have been applied for monitoring different biological samples including bacteria, DNA and specific gravity of urine (O'Keeffe et al., 2015; Liang et al., 2016; Zuppolini et al., 2017). The technique has also been applied for monitoring the neural activity in vivo (Kim et al., 2012).
In this paper, a method has been proposed for detection of the neural activity using optical fibers. We have also studied the effect of different available geometrical structures for fabrication of fiber based SPR sensor on the performance of operation. In this regard, based on computational modeling, the performance of three different structures has been evaluated.
The electrical activity of neuron (action potential) will modify the free electron density inside the gold structure and finally will modify the dielectric constant of the gold structure. Figure 1 shows the schematic of method and its operation. As depicted simply in Figure 1, the variation of dielectric properties of gold will be resulted in the absorption of surface plasmons which are excited in gold through fiber structure (total internal reflection). The variation in absorption is monitored from the output of fiber accordingly. In this study, we have considered three different fiber structures to analyze. In the first case the cladding of fiber is totally removed and replaced with deposited thin film on the surface of core, shown in Figure 2A. As for the second structure, half of the cladding is removed and thin film of gold is deposited instead (Figure 2B). In the third case, half of both cladding and core are removed and the gold layer is deposited (Figure 2C).
Figure 2. Three different geometrical structure of fiber for SPR. (A) Fully-removed cladding. (B) Half-removed cladding. (C) Half-removed core.
The absorption spectrums of the optical fibers have been evaluated based on solving the wave equation inside the fiber structure computationally. The cross section of fiber structure has been considered for 2D analysis. The Finite Element Method (FEM) is used through COMSOL Multiphysics environment to solve Equation (1).
Where E demonstrates the electric field component of light. k0 shows the wave number in vacuum, and ε0 and εr represent vacuum permittivity and material's relative permittivity respectively.
For each wavelength the wave equation is solved for the structure to derive the available modes and their propagation constants.
For the metallic structures, generally the dispersion behavior of permittivity is demonstrated by Drude-Lorentz model, Equation (2).
Where ε∞ shows the permittivity at high frequencies, γD is damping factor and ωD is plasma frequency. ωD and γD demonstrate the strength and spectral width of Lorentz oscillator and Δε shows the weighting factor.
Considering the neural activity, the resulted electric field will modify the plasma frequency of gold based on Equation (3) (Huang et al., 2013).
Where N represents the free electron density and ΔN shows the excess charge residing on gold. In our analysis, the electric field distribution around the metallic thin film has been considered uniform.
We have performed the analysis for the structure of proposed sensor with a multi-mode fiber with radius of 5 μm. Fifty nanometer of gold layer has been considered as active element on the surface of core (replaced with cladding). In order to mimic the real biological environment, 1X PBS buffer solution has been considered as the surrounding medium of the fiber structure. Based on different available propagation modes, there will be different electric field distribution inside the optical fiber structure. Figure 3 shows the electric field distribution inside three different structures of Figure 2. The distribution of electric field inside the structure is dictated based on boundary condition, excitation wavelength and the material property. It can be easily seen that the modes inside the fiber with half core and half cladding is arranged to confine the field inside half of the fiber.
Figure 3. Electric field distribution inside the fiber considering three different structures (A–C).
Considering the range of voltages in action potential, we have calculated the absorption spectrum of structure for −100 and +100 mV. Figure 4 shows the absorption spectrum for three different fiber structures in −100, 0, and +100 mV. As it can be seen, different geometries of fibers have their absorption peak in different wavelengths. For each case the magnified insets show the maximum absorption peak. The shift in peak wavelengths in response to applied voltage (from −100 to +100 mV) was1 nm for fully-removed cladding and half-removed core designs, while a shift of 2 nm was calculated for the half-removed cladding design. In order to check the capability of the proposed structure to track the neural response (action potential pattern), based on the spectral response of the sensor, we have calculated the optical intensity for different voltage ranges in action potential. In this regard, Figure 5 shows the optical response for half-removed cladding structures. As shown, the variation of intensity, tracks the pattern of action potential with appropriate level. Considering optical window for biomedical applications, it seems that the structure with absorption peak around 670 nm could be appropriate in this regard. For intensity-based measurement, the proper wavelength for excitation can be selected based on the absorption spectrum of the structures accordingly. In terms of fabrication, the deposition of thin film of gold on the surface usually is performed using sputtering or thermal evaporation technique. In this regard having a uniformly deposition of the entire perimeter of the circular structure of optical fiber is necessary and needs extra modification in the deposition technique. Meanwhile the half removed cladding structure can be deposited like a planar surface. The half-removed cladding structure shows slightly higher wavelength sensitivity to the voltage, also from the fabrication point of view, the fabrication of this structure is not only more desirable but more reliable.
Figure 4. Absorption spectrum of three different geometrical structures in response to electric potential. The inset shows the magnified peaks.
Figure 5. Light intensity of fiber sensor in response to action potential for half-removed cladding. The excitation wavelength is 680 nm.
The performance of an all optical proposed method for recording neural activity has been evaluated computationally. The analysis was based on solving the wave equation in the fiber and metallic thin film structure by finite element method. The results indicated that the proposed method with different available geometries of fibers are sensitive to the neural activity in their vicinity. Considering wavelength and method of fabrication, the optimum geometry of the structure is for the optical fibers where half cladding has been removed and thin film of gold layer has been deposited therefore. The proposed structure is sensitive, biocompatible, non-destructive, and secure from electromagnetic interference and its performance for monitoring action potential is considerable.
PS proposed the idea and with the contribution of TT performed the initial simulations. PS and MA performed the finite element simulations. PS analyzed the results and wrote the manuscript. TT and MA assisted in analysis of data, discussions and writing the manuscript. All authors read and edited the manuscript.
The authors declare that the research was conducted in the absence of any commercial or financial relationships that could be construed as a potential conflict of interest.
The handling Editor declared a shared affiliation, though no other collaboration, with one of the authors PS.
The present article is financially supported by Research Department of School of Medicine (Grant No.: 11859).
Barry, J. F., Turner, M. J., Schloss, J. M., Glenn, D. R., Song, Y., Lukin, M. D., et al. (2016). Optical magnetic detection of single-neuron action potentials using quantum defects in diamond. Proc. Nat. Acad. Sci. U.S.A. 113, 14133–14138. doi: 10.1073/pnas.1601513113
Cellot, G., Toma, F. M., Kasap Varley, Z., Laishram, J., Villari, A., Quintana, M., et al. (2011). Carbon nanotube scaffolds tune synaptic strength in cultured neural circuits: novel frontiers in nanomaterial–tissue interactions. J. Neurosci. 31, 12945–12953. doi: 10.1523/JNEUROSCI.1332-11.2011
Chen, H. I., Jgamadze, D., Serruya, M. D., Cullen, D. K., Wolf, J. A., and Smith, D. H. (2016). Neural substrate expansion for the restoration of brain function. Front. Syst. Neurosci. 10:1. doi: 10.3389/fnsys.2016.00001
Chen, R., Canales, A., and Anikeeva, P. (2017). Neural recording and modulation technologies. Nat. Rev. Mater. 2:16093. doi: 10.1038/natrevmats.2016.93
Chow, B. Y., Han, X., and Boyden, E. S. (2012). Genetically encoded molecular tools for light-driven silencing of targeted neurons. Prog. Brain Res. 196, 49–61. doi: 10.1016/B978-0-444-59426-6.00003-3
Csáki, A., Stranik, O., and Fritzsche, W. (2018). Localized surface plasmon resonance based biosensing. Expert Rev. Mol. Diagn. 18, 279–296. doi: 10.1080/14737159.2018.1440208
Deadwyler, S. A., Hampson, R. E., Song, D., Opris, I., Gerhardt, G. A., Marmarelis, V. Z., et al. (2017). A cognitive prosthesis for memory facilitation by closed-loop functional ensemble stimulation of hippocampal neurons in primate brain. Exp. Neurol. 287, 452–460. doi: 10.1016/j.expneurol.2016.05.031
Eissa, S., Alshehri, N., Rahman, A. M. A., Dasouki, M., Salah, K. M. A., and Zourob, M. (2018). Electrochemical immunosensors for the detection of survival motor neuron (SMN) protein using different carbon nanomaterials-modified electrodes. Biosens. Bioelectron. 101, 282–289. doi: 10.1016/j.bios.2017.10.015
Fabbro, A., Scaini, D., León, V., Vázquez, E., Cellot, G., Privitera, G., et al. (2016). Graphene-based interfaces do not alter target nerve cells. ACS Nano 10, 615–623. doi: 10.1021/acsnano.5b05647
Giugliano, M., Gambazzi, L., Ballerini, L., Prato, M., and Campidelli, S. (2012). “Carbon nanotubes as electrical interfaces to neurons,” in Nanotechnology for Biology and Medicine: At the Building Block Level, eds G. A. Silva and V. Parpura (New York, NY: Springer), 187–207.
Gunasekera, B., Saxena, T., Bellamkonda, R., and Karumbaiah, L. (2015). Intracortical recording interfaces: current challenges to chronic recording function. ACS Chem. Neurosci. 6, 68–83. doi: 10.1021/cn5002864
Hanein, Y., and Bareket-Keren, L. (2013). Carbon nanotube-based multi electrode arrays for neuronal interfacing: progress and prospects. Front. Neural Circuits 6:122. doi: 10.3389/fncir.2012.00122
Hindriks, R., Arsiwalla, X. D., Panagiotaropoulos, T., Besserve, M., Verschure, P. F. M. J., Logothetis, N. K., et al. (2016). Discrepancies between multi-electrode LFP and CSD phase-patterns: a forward modeling study. Front. Neural Circuits 10:51. doi: 10.3389/fncir.2016.00051
Hinman, S. S., McKeating, K. S., and Cheng, Q. (2018). Surface plasmon resonance: material and interface design for universal accessibility. Anal. Chem. 90, 19–39. doi: 10.1021/acs.analchem.7b04251
Huang, Y., Xie, W., Tang, D., and Du, C. (2013). Theoretical analysis of voltage-dependent fiber optic surface plasmon resonance sensor. Opt. Commun. 308, 109–114. doi: 10.1016/j.optcom.2013.06.058
Kempuraj, D., Thangavel, R., Selvakumar, G. P., Zaheer, S., Ahmed, M. E., Raikwar, S. P., et al. (2017). Brain and peripheral atypical inflammatory mediators potentiate neuroinflammation and neurodegeneration. Front. Cell. Neurosci. 11:216. doi: 10.3389/fncel.2017.00216
Kim, S. A., Byun, K. M., Lee, J. K., Kim, J. H., Kim, D.-G. A., Baac, H., et al. (2008). Optical measurement of neural activity using surface plasmon resonance. Opt. Lett. 33, 914–916. doi: 10.1364/OL.33.000914
Kim, S. A., Kim, S. J., Moon, H., and Jun, S. B. (2012). In vivo optical neural recording using fiber-based surface plasmon resonance. Opt. Lett. 37, 614–616. doi: 10.1364/OL.37.000614
Kralj, J.M., Douglass, A.D., Hochbaum, D.R., Maclaurin, D., and Cohen, A.E. (2011). Optical recording of action potentials in mammalian neurons using a microbial rhodopsin. Nat. Methods 9, 90–95 doi: 10.1038/nmeth.1782
Kulkarni, R. U., and Miller, E. W. (2017). Voltage Imaging: pitfalls and Potential. Biochemistry 56, 5171–5177. doi: 10.1021/acs.biochem.7b00490
Lertvachirapaiboon, C., Baba, A., Ekgasit, S., Shinbo, K., Kato, K., and Kaneko, F. (2018). Transmission surface plasmon resonance techniques and their potential biosensor applications. Biosens. Bioelectron. 99, 399–415. doi: 10.1016/j.bios.2017.07.069
Liang, G., Luo, Z., Liu, K., Wang, Y., Dai, J., and Duan, Y. (2016). Fiber optic surface plasmon resonance–based biosensor technique: fabrication, advancement, and application. Crit. Rev. Anal. Chem. 46, 213–223. doi: 10.1080/10408347.2015.1045119
Liu, P., Grenier, V., Hong, W., Muller, V. R., and Miller, E. W. (2017). Fluorogenic targeting of voltage-sensitive dyes to neurons. J. Am. Chem. Soc. 139, 17334–17340. doi: 10.1021/jacs.7b07047
Lu, L., Gutruf, P., Xia, L., Bhatti, D. L., Wang, X., Vazquez-Guardado, A., et al. (2018). Wireless optoelectronic photometers for monitoring neuronal dynamics in the deep brain. Proc. Natl. Acad Sci. U.S.A. 115, E1374–E1383. doi: 10.1073/pnas.1718721115
Maccione, A., Gandolfo, M., Zordan, S., Amin, H., Di Marco, S., Nieus, T., et al. (2015). Microelectronics, bioinformatics and neurocomputation for massive neuronal recordings in brain circuits with large scale multielectrode array probes. Brain Res. Bull. 119, 118–126. doi: 10.1016/j.brainresbull.2015.07.008
Marchesan, S., Ballerini, L., and Prato, M. (2017). Nanomaterials for stimulating nerve growth. Science 356, 1010–1011. doi: 10.1126/science.aan1227.
Neely, R. M., Piech, D. K., Santacruz, S. R., Maharbiz, M. M., and Carmena, J. M. (2018). Recent advances in neural dust: towards a neural interface platform. Curr. Opin. Neurobiol. 50, 64–71. doi: 10.1016/j.conb.2017.12.010
Nixima, K., Okanoya, K., Ichinohe, N., and Kurotani, T. (2017). Fast voltage-sensitive dye imaging of excitatory and inhibitory synaptic transmission in the rat granular retrosplenial cortex. J. Neurophysiol. 118, 1784–1799. doi: 10.1152/jn.00734.2016
O'Keeffe, S., McCarthy, D., Woulfe, P., Grattan, M. W. D., Hounsell, A. R., Sporea, D., et al. (2015). A review of recent advances in optical fibre sensors for in vivo dosimetry during radiotherapy. Br. J. Radiol. 88:20140702. doi: 10.1259/bjr.20140702
Park, D.-W., Brodnick, S. K., Ness, J. P., Atry, F., Krugner-Higby, L., Sandberg, A., et al. (2016). Fabrication and utility of a transparent graphene neural electrode array for electrophysiology, in vivo imaging, and optogenetics. Nat. Protoc. 11, 2201–2222. doi: 10.1038/nprot.2016.127
Park, D.-W., Ness, J. P., Brodnick, S. K., Esquibel, C., Novello, J., Atry, F., et al. (2018). Electrical neural stimulation and simultaneous in vivo monitoring with transparent graphene electrode arrays implanted in GCaMP6f mice. ACS Nano 12, 148–157. doi: 10.1021/acsnano.7b04321
Polonschii, C., David, S., Gáspár, S., Gheorghiu, M., Rosu-Hamzescu, M., and Gheorghiu, E. (2014). Complementarity of EIS and SPR to reveal specific and nonspecific binding when interrogating a model bioaffinity sensor; perspective offered by plasmonic based EIS. Anal. Chem. 86, 8553–8562. doi: 10.1021/ac501348n
Scaini, D., and Ballerini, L. (2018). Nanomaterials at the neural interface. Curr. Opin. Neurobiol. 50, 50–55. doi: 10.1016/j.conb.2017.12.009
Shapiro, M. G., Homma, K., Villarreal, S., Richter, C.-P., and Bezanilla, F. (2012). Infrared light excites cells by changing their electrical capacitance. Nat. Commun. 3:736. doi: 10.1038/ncomms1742
Sokolov, I., Azieva, A., and Burtsev, M. (2016). “Patterns of spiking activity of neuronal networks in vitro as memory traces,” in Biologically Inspired Cognitive Architectures (BICA) for Young Scientists Vol. 449, eds V. V. Klimov, G. V. Rybina and A. V. Samsonovich (Moscow: Springer Verlag), 241–247.
Tekieh, T., Sasanpour, P., and Rafii-Tabar, H. (2018). Electrophysiological effects of low frequency electrical radiation on the neural compartment: a theoretical investigation. Biomed. Phys. Eng. Express 4:2. doi: 10.1088/2057-1976/aaa378
Wells, J., Kao, C., Mariappan, K., Albea, J., Jansen, E. D., Konrad, P., et al. (2005). Optical stimulation of neural tissue in vivo. Opt. Lett. 30, 504–506. doi: 10.1364/OL.30.000504
Zhang, J., Atay, T., and Nurmikko, A. V. (2009). Optical detection of brain cell activity using plasmonic gold nanoparticles. Nano Lett. 9, 519–524. doi: 10.1021/nl801891q
Zhong, X., Luo, J., Rastogi, P., Kanthasamy, A. G., and Jiles, D. C. (2018). Transcranial magnetic stimulation promotes the proliferation of dopaminergic neuronal cells in vitro. AIP Adv. 8:056709. doi: 10.1063/1.5007677
Keywords: neural activity recording, action potential, surface plasmon resonance, fiber optics, COMSOL, optical recording, optogenetics
Citation: Abedini M, Tekieh T and Sasanpour P (2018) Recording Neural Activity Based on Surface Plasmon Resonance by Optical Fibers-A Computational Analysis. Front. Comput. Neurosci. 12:61. doi: 10.3389/fncom.2018.00061
Received: 24 March 2018; Accepted: 11 July 2018;
Published: 03 August 2018.
Edited by:
Reza Lashgari, Brain Engineering Research Center, Institute for Research in Fundamental Sciences, IranReviewed by:
Andreas Offenhäusser, Forschungszentrum Jülich, Helmholtz-Gemeinschaft Deutscher Forschungszentren (HZ), GermanyCopyright © 2018 Abedini, Tekieh and Sasanpour. This is an open-access article distributed under the terms of the Creative Commons Attribution License (CC BY). The use, distribution or reproduction in other forums is permitted, provided the original author(s) and the copyright owner(s) are credited and that the original publication in this journal is cited, in accordance with accepted academic practice. No use, distribution or reproduction is permitted which does not comply with these terms.
*Correspondence: Pezhman Sasanpour, cGVzYXNhbnBvdXJAc2JtdS5hYy5pcg==
Disclaimer: All claims expressed in this article are solely those of the authors and do not necessarily represent those of their affiliated organizations, or those of the publisher, the editors and the reviewers. Any product that may be evaluated in this article or claim that may be made by its manufacturer is not guaranteed or endorsed by the publisher.
Research integrity at Frontiers
Learn more about the work of our research integrity team to safeguard the quality of each article we publish.