- 1Dynamic Online Networks Laboratory, George Washington University, Washington, DC, United States
- 2Physics Department, George Washington University, Washington, DC, United States
- 3École des Hautes Etudes Commerciales de Paris, Jouy-en-Josas, France
Introduction: Collective human distrust—and its associated mis/disinformation—is one of the most complex phenomena of our time, given that approximately 70% of the global population is now online. Current examples include distrust of medical expertise, climate change science, democratic election outcomes—and even distrust of fact-checked events in the current Israel-Hamas and Ukraine-Russia conflicts.
Methods: Here we adopt the perspective of the system being a complex dynamical network, in order to address these questions. We analyze a Facebook network of interconnected in-built communities (Facebook Page communities) totaling roughly 100 million users who, prior to the pandemic, were just focused on distrust of vaccines.
Results: Mapping out this dynamical network from 2019 to 2023, we show that it has quickly self-healed in the wake of Facebook’s mitigation campaigns which include shutdowns. This confirms and extends our earlier finding that Facebook’s ramp-ups during COVID-19 were ineffective (e.g., November 2020). We also show that the post-pandemic network has expanded its topics and has developed a dynamic interplay between global and local discourses across local and global geographic scales.
Discussion: Hence current interventions that target specific topics and geographical scales will be ineffective. Instead, our findings show that future interventions need to resonate across multiple topics and across multiple geographical scales. Unlike many recent studies, our findings do not rely on third-party black-box tools whose accuracy for rigorous scientific research is unproven, hence raising doubts about such studies’ conclusions–nor is our network built using fleeting hyperlink mentions which have questionable relevance.
1 Introduction
There is arguably nothing more complex than human behavior. The COVID-19 pandemic showed how, in moments of uncertainty and concern, the global population (of which approximately 70% are now online) tend to go online to seek advice from their trusted online communities (e.g., Facebook Pages, henceforth known as “Facebook community” or “FC”)—even though such FCs do not typically have any documented medical or scientific expertise (Johnson et al., 2020; Restrepo et al., 2022a; Restrepo et al., 2022b; Illari et al., 2022). Somehow, the collective hearts and heads within and across these in-built FCs get mixed in such a way that established medical and scientific expertise get set aside (Kleineberg and Boguñá, 2016; DiResta, 2018; Lappas et al., 2018; Semenov et al., 2019; Ardia et al., 2020; Chen et al., 2020; Larson, 2020; Starbird et al., 2020; Hootsuite, 2021; Douek, 2022; Rao et al., 2022; Wardle, 2023). This can also leave people open to online manipulation through intentional disinformation which quickly expands to fill any void (NewsDirect, 2021; Jilani, 2022; Meyers, 2022; EU, 2023; Nobel Prize Summit, 2023).
This raises the crucial societal question going forward, of how a complex system of adaptive, decision-making humans with limited information, imperfect memories and knowledge bases, and often strong collective interactions, will behave in future global crises (San Miguel and Toral, 2020; Czaplicka et al., 2022; Diaz-Diaz et al., 2022). In particular, it opens up a new challenge opportunity for complexity science to understand why the online distrust ecosystem is so robust, how it evolves over time, and how well do current mitigations and policies work at scale. We attempt to address these questions here.
We pursue a complex dynamical-network systems approach to understanding this problem (Yalcin et al., 2016; Tria et al., 2018; Mazzeo et al., 2021; Mazzeo and Rapisarda, 2022) in contrast with the rather static existing health approach where the focus is either on the entire population (public health) or a single individual (personal health). Instead, we focus in on the intermediate meso-scale dynamics between the scale of a single individual and the total population, because it is known from complex systems studies that this is the dynamical regime where correlations appear that can then lead to macroscopic changes and transitions. This mesoscopic focus has a direct real-world justification because the online human social media system contains approximately 5 billion people who self-organize into in-built FCs—each of which contains many thousands of individuals and has nothing to do with network community detection (Johnson et al., 2020; Restrepo et al., 2022a; Restrepo et al., 2022b; Illari et al., 2022). Each in-built FC is a separate Facebook page in our study. These Facebook communities (Facebook Pages) then interconnect with each other via page-level links in order to share content feeds and hence share conversations, opinions and information at the page level. Reciting the description of a complex system from Ref. (Johnson et al., 2003) and elsewhere, we expect the online human system to feature the key complex systems characteristics: i) Feedback which can change with time—for example, becoming positive one moment and negative the next—and may also change in magnitude and importance. ii) Non-stationarity. We cannot assume that the dynamical or statistical properties observed in the system’s past will remain unchanged in the system’s future. iii) Many interacting agents. The system contains many individuals or “agents”, which interact in possibly time-dependent ways. Their community-level behavior will respond to the feedback of information from the system as a whole and/or from other communities. iv) Adaptation. A community can adapt its behavior to reflect world events and attract new recruits. v) Evolution. The entire online population evolves, driven by an ecology of interacting communities which interact and adapt under the influence of feedback. The system typically remains far from equilibrium, and hence can exhibit extreme behaviors. vi) Single realization. The system under study is a single realization, implying that standard techniques whereby averages over time are equated to averages over ensembles, may not work. vii) Open system. The system is coupled to the outside world including local and world news, and hence responds to both exogenous (i.e., outside) and endogenous (i.e., internal, self-generated) effects.
As we show in this paper, adopting this complex dynamical-network systems perspective yields various new insights–in particular, why the distrust ecosystem has become so robust, how it evolves over time, and how well current mitigations and policies work. The important task of providing an accompanying mathematical description is a work in progress which could benefit from fascinating recent analyses [see for example, (Yalcin et al., 2016; Tria et al., 2018; San Miguel and Toral, 2020; Mazzeo et al., 2021; Czaplicka et al., 2022; Diaz-Diaz et al., 2022; Mazzeo and Rapisarda, 2022)]. While we ourselves cannot yet offer such a mathematical theory, we hope that the empirical patterns presented here will stimulate such work in the complex systems community. With this in mind, our analysis here focuses around establishing some “stylized facts” using several complex systems tools in order to help future model-building—specifically, we use dynamical networks and n-Venn diagrams for our analysis.
There is of course already a vast literature outside the complex systems field, attempting to understand the problem—mostly from sociological, psychological, political science, and pure computer science perspectives. In addition, the social media giants such as Facebook (Meta, but we refer to them as Facebook for convenience) have made efforts to curtail the associated avalanche of mis/disinformation through strategies like content moderation and removal of anti-vaccine content. We refer for example to (van der Linden et al., 2017; Lazer et al., 2018; RAND Corporation, 2019; Lewandowsky et al., 2020; Smith et al., 2020; WHO, 2020; Wone, 2020; Calleja et al., 2021; The Rockefeller Foundation, 2021; Roozenbeek et al., 2022; Get the facts on coronavirus, 2023; Green et al., 2023; Klein and Hostetter, 2023; Mirza et al., 2023; Society of Editors, 2023; Taylor et al., 2023; The National Academies, 2023; Wardle, 2023) However distrust continues to persist (Ravenelle et al., 2021; Boyle, 2022) which suggests that these interventions have only scratched the surface. Here we pursue the understanding of why this is, in order to gain insight into what is missing from current efforts and hence what can be done.
Our specific contribution here—which is made possible only because we take a complex systems perspective—is the first ever analysis of the post-pandemic evolution of Facebook’s vaccine discourse network through September 2023. This enables us to chart the trajectory that the network has taken from its initial November 2019 state when it was focused around vaccine distrust (Johnson et al., 2020). Our findings reveal a vast web-of-distrust that has developed post-pandemic, that seamlessly integrates diverse topics, locations, and geographical scales and hence challenges traditional approaches to health messaging. Our analysis focuses on Facebook because of its unparalleled reach as the predominant social media platform (Meta, 2020). Central to our study are its inherent social media community features—Facebook Pages (FCs)—that facilitate virtual congregations centered on shared interests, such as parenting. These digital FCs have emerged as pivotal hubs for social interactions on platforms like Facebook. Contemporary research highlights the growing dependence on these FCs for guidance, particularly in areas like family health (Ammari and Schoenebeck, 2016; Laws et al., 2019; Moon et al., 2019). Members develop a profound trust within their respective FCs, often valuing insights from peers with parallel concerns. For instance, parents navigating health decisions for their children tend to resonate more with anecdotal experiences and perspectives shared by fellow parents (Ammari and Schoenebeck, 2016; Laws et al., 2019; Moon et al., 2019).
Our analysis in this paper yields three major insights about the nature of this post-pandemic Facebook vaccine discourse network: 1) an inherent robustness within the network, allowing it to adapt and counteract Facebook’s interventions; 2) the recent convergence of diverse narratives, from COVID-19 to climate change and elections, into a unified web of discourse, underscoring the expansive reach and influence of the network; and 3) the switching of discourse dynamics between global and local scales, demonstrating the network’s versatility and adaptability. This means that traditional mitigation strategies are insufficient for improving distrust at scale because they tend to operate in silos for a specific topic (e.g., monkeypox) and at a specific geographical scale (e.g., state-level health departments for the individual states in the U.S.). It also enables us to propose a potentially far better system-level approach in the form of interventions that resonate across multiple topics and across multiple geographical scales. Our findings also serve to confirm and extend our earlier finding reported in late 2021 and early 2022, that Facebook’s ramp-ups were ineffective during COVID-19 (e.g., ramp-up around November 2020) as shown in Refs. (Restrepo et al., 2022a; Johnson et al., 2022). This lack of impact of Facebook’s mitigation policies during the pandemic (e.g., ramp-up around November 2020) was subsequently independently confirmed in a later separate study (Broniatowski et al., 2023).
In the following sections, we delve into the methodologies, results, and discussion of our study. The Section 2 details the data collection process and analytical tools employed, while the Section 3 presents our key findings on the dynamics of Facebook’s vaccine discourse network. In the Section 4, we interpret these findings in the context of existing literature and propose implications for future interventions and research.
2 Materials and methods
In this Methods section, we detail our approach for analyzing the evolution of vaccine discourse within Facebook communities (FCs) post-pandemic, utilizing a network-based analysis to track changes in trust and narrative focus.
We examine how the distrust discourse changed post-pandemic by analyzing the evolution through time of the pre-pandemic 2019 Facebook network that had developed around vaccine distrust (Johnson et al., 2020). It comprised 1356 interlinked FCs that were pro-vaccine, anti-vaccine, or neutral towards vaccines. Our methodology follows exactly Ref. (Johnson et al., 2020) and the details are reviewed again in the Supplementary Material. Each Facebook page is a Facebook community (i.e., node in Figure 1) with a unique ID. These Facebook communities have nothing to do with community detection in networks. These FCs provide spaces for users to gather around shared interests, promoting trust within the Facebook community and potential distrust of external issues (Kim and Ahmad, 2013; Forsyth, 2014; Chen et al., 2015b; Chen et al., 2015a; Gelfand et al., 2017; Ardia et al., 2020; Arriagada and Ibáñez, 2020; WHO, 2020; Madhusoodanan, 2022; Pertwee et al., 2022; Tram et al., 2022; Freiling et al., 2023; Song et al., 2023). Our trained researchers manually and independently classified each FC as pro-vaccine, anti-vaccine, or neutral. In cases of disagreement, the researchers discussed until reaching consensus. This process yielded a network of 1356 interlinked FCs across countries and languages, with 86.7 million individuals in the largest network component classified as follows: 211 pro-vaccine FCs with 13.0 million individuals (blue nodes, Figure 1); 501 anti-vaccine FCs with 7.5 million individuals (red nodes, Figure 1); and 644 neutral FCs with 66.2 million individuals (non-blue or red nodes, Figure 1). The neutral FCs were further sub-categorized by type, such as parenting. Our methodology is reviewed in detail in Supplementary Section S1 and a complete breakdown of the neutral subcategories is in Supplementary Section S3.
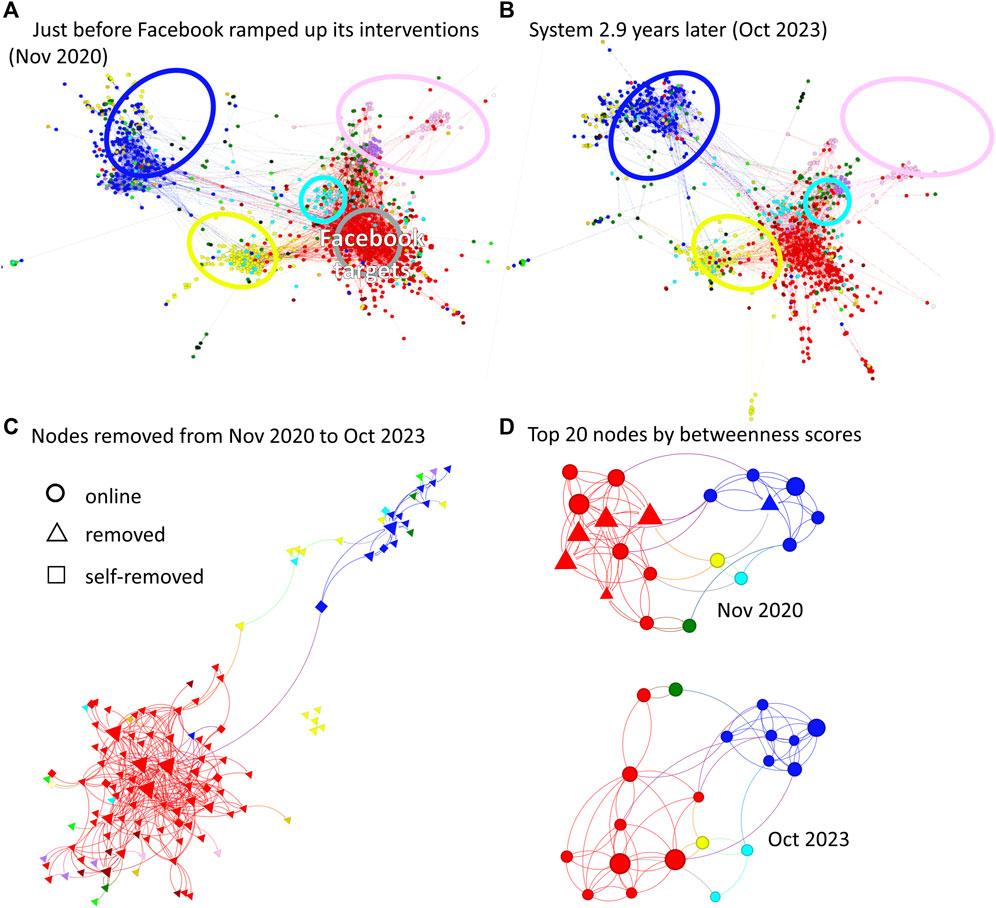
FIGURE 1. Evolution over time of the 2019 Facebook vaccine distrust network (Johnson et al., 2020) which encompasses nearly 100 million users. Starting in November 2020, Facebook amplified its interventions, notably removals [see (C)]. Until that point [i.e., (A)], the focus was on posting factual messages. The ForceAtlas2 layout mechanism means that nodes (i.e., Pages) appearing closer together are more likely to share content (see Supplementary Section S5). (A) and (B) use the same scale: rings indicate approximate locations of node categories (blue, red, neutrals) in the November 2019 network layout, underscoring the intensification of connections within the red (i.e., anti-vaccine) nodes and between red and neutral nodes. Blue nodes are pro-vaccine FCs. Different colors are used to distinguish the specific categories of the neutral FCs (i.e., the “greens”). (D) reveals a self-repairing core “mesh” of high betweenness anti-vaccination (red) FCs from within (A,B) that can—and does persist to—share and distribute extreme content amongst each other and with other nodes in the full ecology, including millions of mainstream users in neutral nodes. The specific nodes within this core mesh [i.e., (D) lower panel] change over time, but its structure remains robust which serves as confirmation of the inefficiency in Facebook’s November 2020 (and other) interventions as reported in Ref. (Restrepo et al., 2022a). This lack of impact was then subsequently confirmed in a later study (Broniatowski et al., 2023). Node size in (C,D) signifies betweenness values, i.e., a node’s capacity to serve as a conduit for content.
To categorize the discourse topics within each of the 1356 FCs, we developed keyword filters for five non-vaccine-related topics that subsequently emerged: COVID-19, monkeypox (mpox), abortion, elections, and climate change. The filters combined regular expressions and keyword searches in multiple languages (see Supplementary Section S7) and were applied to post content, descriptions, image tags, and link text. By “non-vaccine” we mean topics not centered on vaccines in a broad sense. Although the COVID-19 and monkeypox filters included some vaccine terms (for e.g., “monkeepox vax’n”), the intent was to capture disease-specific discourse rather than general vaccine discussion. Distinguishing between COVID-19 and monkeypox posts in an automated manner would have been difficult without additional human classification had we been filtering for general vaccine discussion rather than disease-specific discourse.
A link between FCs A and B (i.e., Facebook pages A and B) indicates FC (page) A recommends FC (page) B to its members at the page level, creating a hyperlink from page A to page B. This shows page A’s interest in page B’s content and goes beyond just mentioning B casually in a URL. Links do not necessarily signify agreement, rather it directs the attention of A’s members to B, and vice versa it exposes A to feedback and content from B. While not all members will necessarily pay attention, a committed minority of 25% can be enough to influence the stance of an online FC (Centola et al., 2018).
Of the 1356 FCs, 342 are local with 3.1 million individuals; the remaining 1014 are global with 83.7 million individuals (see Supplementary Section S2 for details). In terms of geography, a global FC is a page with broad, worldwide focus that is not tied to a specific location, while a local FC is focused on a specific geographic area, such as a neighborhood, city, county, state, or country (e.g., “Vaccine information for parents” or “Global Trends” vs. “Vaccine information for Los Angeles County parents”). In terms of topic, a global FC discusses diverse issues broadly, whereas a local FC has a narrow topical focus (e.g., pages discussing only elections). The size of each FC can be estimated by the number of likes, given that the average user only likes one Facebook page on average (Hootsuite, 2021). However, our analysis and findings are not dependent on this.
In this paper, the terms “global” and “local” are applied to two categorical dimensions—geographic scale and topic—in order to examine the interconnection between geographic and topic glocality. This dual usage of “global” and “local” elucidates how geographic and topic glocality can interrelate and allow FCs to take up different glocal positions. For instance, a FC focused on a narrow topic within a small geographical locality embodies hyperlocality on both dimensions, while one that discusses many topics worldwide embodies hyperglobality. The purpose of this is to analyse the extent to which the post-pandemic, skepticism discourse—whether it be about global health challenges, societal decisions, or environmental concerns—expanded beyond just hyperlocal geography and topics to encompass more hyperglobal geography and topics. The terms “global” and “local” effectively convey this evolution across both dimensions.
We use betweenness centrality to quantify nodes' potential to serve as an information conduit. A node with high betweenness centrality is critical to information flow and is considered a “broker” of information, which is instrumental in understanding the spread of vaccine-related narratives. This quantity is discussed in greater detail in all network science textbooks.
Distinct methodological advantages of our approach as compared to other recent studies [e.g., (Broniatowski et al., 2023)] include the fact that our findings do not rely on third-party black-box tools such as CrowdTangle whose accuracy for rigorous scientific research is unproven and increasingly questionable given Facebook’s internal policies, hence casting doubt on these other studies’ conclusions. Also, these previous studies are restricted since they only consider a mention/hyperlink network where links represent the appearance of a URL in a page’s content. There is no a priori reason or guarantee that these fleeting URLs represent meaningful long-term connections between one FC and another, nor that they influence their subsequent behavior in any significant way. This needs to be proven before any subsequent network analysis can be regarded as meaningful at scale. By contrast, in our study the links between nodes (FCs) are better defined, i.e., if page A “likes” page B then this FC A links to FC B which creates an information conduit from B into A and hence exposes A’s users to B’s content (e.g., new posts from Facebook page B can appear on Facebook page A). Thus, the difference between our network versus those of these other studies, lies in the fact that our study is a follower/like/social network based on the formal page follow/like connections on Facebook itself whereas those other studies are simply a mention network. Technically, we also note that it is not obvious how to create our kind of network using third-party tools such as CrowdTangle as used by these other studies.
3 Results
In this Results section, we present our findings on the post-pandemic evolution of Facebook’s vaccine discourse network. We focus on the dynamics of interconnected Facebook communities, their thematic diversity, and the implications of these changes.
Using the pre-COVID-19 vaccine network from Ref. (Johnson et al., 2020) as a starting point, we mapped the evolving dynamics of these interconnected Facebook communities (FCs, where each Facebook community is a Facebook Page) and their views (Figures 1A–D). Around November 2020, Facebook shifted its approach from primarily posting informational messages to aggressively removing or suppressing content, especially from anti-communities that shared vaccine-skeptical content. However, while these interventions were aimed at curbing the spread of misinformation, our results suggest that the more subtle mainstream science-skeptical content, which may not be outright false but still casts doubt, continued to permeate the network, reaching a broader audience. Figures 1A, B chronicle the evolution of the 2019 Facebook vaccine-view ecology. Figure 1A captures the ecosystem just before Facebook intensified its interventions in November 2020, while Figure 1B offers a snapshot nearly 3 years later (the network at more timestamps is available in Supplementary Section S2). The force-directed layout, ForceAtlas2, implies that nodes that appear nearby to each other in the network layout (i.e., FCs/Pages) are more likely to exchange content. The concentric rings in both figures demarcate the original positions of the FCs from the pre-COVID-19 era of the network (end of 2019), emphasizing the strengthening connections within and between different nodes, particularly within the red nodes and between the red and neutral nodes.
In Supplementary Section S2, we show the network in Figure 1 at four different time points from November 2019 to September 2023. The circular boundaries in Figures 1A, B indicate the approximate positions of node categories back in November 2019. ForceAtlas2 is a force-directed network layout algorithm designed specifically to provide visually interpretable spatializations that expose clusters and connections; its purpose is to transform network data into a map-like representation to aid analysis (Jacomy and Bastian, 2011; Jacomy et al., 2012; Jacomy et al., 2014; Plique, 2021).
Our objective in employing ForceAtlas2 in Figure 1, is not to overinterpret specific node locations or distances, but rather to highlight the broader structural changes over time. The November 2019 circular boundaries in Figures 1A, B serve as a point of comparison to elucidate how clusters of certain node types (particularly anti-vaccine in red) have densified and expanded connections to other nodes by November 2020 and September 2023. Our analysis aims to demonstrate larger topological patterns in terms of interconnectivity among categories, rather than drawing quantitative conclusions from precise spatial positions. For example, the increasing density of red nodes across time periods shows their growing internal robustness as a cluster. By overlaying the November 2019 node category locations, the intent is to guide the eye towards the higher-level structural shifts indicating greater assimilation of red anti-vaccine nodes with neutral nodes. However, due caution is taken not to quantitatively compare specific inter-node distances or locations between time points.
It is of course possible to go further than we do here by analyzing the network using network similarity measures such as the Rand index (which measures the similarity between two data clusterings), the Jaccard index (which compares how many elements two sets share), the Omega index (which compares non-disjoint clustering solutions), and the Normalized Mutual Information (which evaluates the performance of clustering and classification algorithms). While we will explore these in the future, we don’t do that here since our network analysis methodology in this paper focuses on 1) subject matter expert-defined (i.e., clusters are not a result from algorithmic clustering) and 2) non-overlapping categories (no overlap to categorize).
Figure 1C illustrates nodes that were either deleted or made private between November 2020 and September 2023. Here, node size is related to betweenness scores and fan count/follower count for these pages range from millions to tens. Notably, despite the removal of some large, active extreme FCs, the network’s high interconnectivity and operational robustness remains largely intact. This robustness is further highlighted in Figure 1D, which depicts the top 20 nodes based on betweenness centrality—an indicator of the influence a node has over the flow of information in a network. Most notably, red nodes, signifying anti-vaccination communities, rank prominently within this subsystem of top nodes. Even though numerous red nodes underwent removal by Facebook (as seen in Figure 1D upper panel, as indicated by the triangular nodes), a network rewiring phenomenon ensued, leading to the emergence of other red nodes in their stead (shown in Figure 1D lower panel). A similar robustness effect can be observed between November 2019 and November 2020 (see Supplementary Section S2). This confirms our earlier report of the inefficiency in Facebook’s November 2020 (and other) interventions (Restrepo et al., 2022a; Johnson et al., 2022). This initial finding (Restrepo et al., 2022a; Johnson et al., 2022) was subsequently confirmed by a later independent study (Broniatowski et al., 2023).
From the outset, the network experienced an overall upward trajectory in betweenness scores. The magnitude of this elevation varies among top nodes, ranging from a percent difference of +8% to +140%. This variation, as captured by a mean absolute deviation of 21%, underscores the diverse trajectories of nodes within the network. Despite periodic changes and distinct node shifts between November 2020 and September 2023, the vaccine stance composition of this group exhibits remarkable consistency. This stability extends beyond mere node categorization, as can be seen in Figures 1A, B—i.e., the holistic structure and dynamics of the network have remained largely consistent.
The robustness observed in the network’s core structure, even in the face of external interventions, has profound implications. It indicates that there exists a robust and interconnected subset of nodes that consistently rise to prominence, effectively preserving the network’s thematic and structural integrity. This robust core seems to be somewhat impervious to node deletion mitigation efforts, suggesting that such interventions alone might not suffice to significantly alter the discourse or dynamics within the network. Instead, the network appears to have an inherent capacity to “self-heal” and maintain its equilibrium. In essence, while individual nodes may come and go, the network’s backbone remains steadfast. This robustness underscores the challenge of influencing or shifting discourse dynamics solely through node-centric interventions. A more holistic, system-level understanding, and approach is therefore necessary to effectively navigate and influence such robust networks.
When examining the geographic specificity and thematic diversity within Facebook communities (Figure 2A), we observe that among the top 20 nodes sorted by betweenness centrality, 4 are geographically localized (see Supplementary Section S7 for complete breakdown). This descriptive statistic is noteworthy for its informative value—it provides insight into the structure of influence within the network. It suggests that while most top FCs cater to a broader audience, a tangible portion of influential nodes are rooted in local contexts. While global narratives set the overarching tone, it is often the local narratives that drive grassroots actions, sentiments, and perceptions. Thus, their presence underlines the importance of region-specific dialogues even within a global platform like Facebook, and suggests that localized information, when tailored effectively, can have a disproportionately large impact.
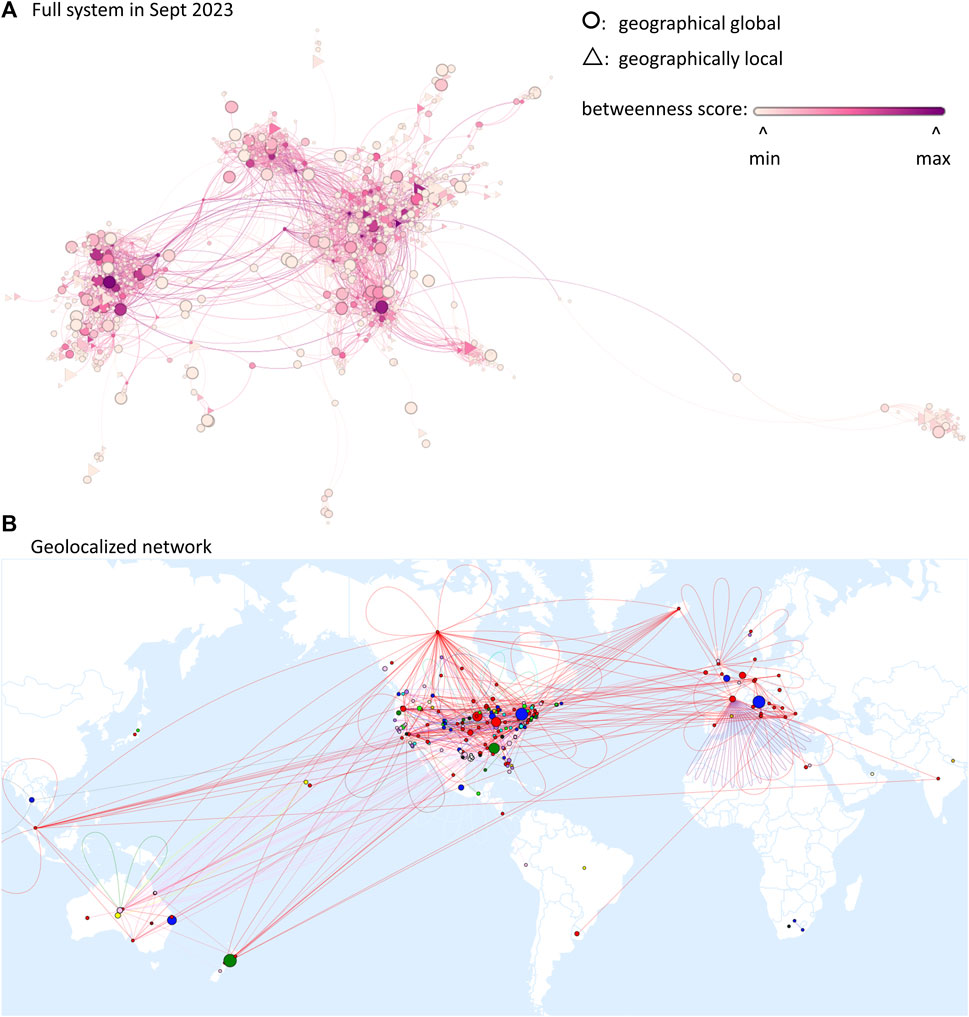
FIGURE 2. Visualization of the post-pandemic 2019 Facebook vaccine network. (A) Nodes (Facebook communities) are sized by number of discourse topics in which each engaged (topic count), shaped by local or global status (whether or not a location is mentioned in a Page’s username), and colored by betweenness centrality score. Visual examination suggests a positive correlation between topic count and betweenness score. (B) Geolocalized network of FCs mentioning locations in usernames. Self-loops are not possible; the apparent “self-loops” are due to the extremely close proximity of locations mentioned in FC usernames. A clear majority of local nodes appear to be in English-speaking countries.
Furthermore, a notable 70% of these top nodes engage in dialogues spanning five key discourses: COVID-19, mpox, abortions, elections, and climate change. The depth of their engagement, however, is not uniform. A minority (3 of 14 nodes) primarily focus on one topic, while the majority (11 of 14 nodes) partake in discussions on at least three topics. The most recurrent thematic combinations are COVID-19, mpox, and elections, followed by COVID-19, mpox, and climate change. This suggests that these FCs are not siloed into singular narratives but rather weave together multiple themes, potentially drawing parallels, intersections, or contrasts between them.
Statistical analysis was utilized to elucidate the relationships between a page’s (FC’s) fan count, betweenness centrality, and the diversity of topics it addresses. We applied Pearson’s product-moment correlation tests to measure linear associations, where “r” represents the correlation coefficient, indicating the strength and direction of the relationship. Additionally, we conducted one-way Analysis of Variance (ANOVA) tests, using the F-statistic (F) to compare the variances between multiple groups, reflecting the ratio of the variance between the groups to the variance within the groups. The “p-value” accompanying both tests signifies the probability of obtaining the observed results, or more extreme, under the null hypothesis that there is no actual association (for correlation) or no difference among group means (for ANOVA). For an in-depth description of the statistical methodologies and the full test results, we refer to Supplementary Section S7.
Our analysis yielded the following insights: 1) Pages discussing a wider range of topics exhibited a weak, yet statistically significant, positive correlation with betweenness centrality (r = 0.121, p < 0.001). This suggests such pages may play a more central role in information dissemination within the network. 2) Larger audience pages (higher fan count) show a propensity to discuss a diverse set of topics, as evidenced by the weak positive correlation between fan count and topic count (r = 0.121, p < 0.001). Furthermore, one-way analysis of variance highlighted significant differences in both betweenness centrality scores [F (5, 1093) = 4.14, p < 0.001)] and fan counts [F (5, 1093) = 4.53, p < 0.001] across different topic count categories. For an in-depth description of the statistical methodologies and the full test results, we again refer to Supplementary Section S7.
This means that a large follower count does not necessarily equate to a page being a central hub for information flow within the network. Betweenness centrality, which gauges a node’s role as a connector in the network, reveals that a page’s influence is not solely dictated by its fan base. For example, a celebrity page with many followers may not be a primary information source, whereas a niche expert page might have fewer followers but serves as a pivotal connection point in certain discussions. Interestingly, while a page’s audience size does not correlate with its network centrality, the breadth of topics that it covers does. A diverse audience will have varied interests, pushing the page admins to cover multiple subjects to keep the audience engaged. Essentially, pages that delve into various topics not only position themselves more centrally in the network but also appeal to a broader audience, highlighting the importance of content diversity in influencing both network prominence and audience engagement.
Figure 2B delves into the geographic indications within FC names. Predominantly, these point to the United States. However, the top 10 also features other nations, primarily English-speaking, such as Australia, Canada, and the United Kingdom. Additionally, while page administrative data included many of these same countries for their administrators, it showcases diversity with countries such as Israel, the Philippines, and Slovenia, which are not represented in page usernames (see Supplementary Section S2). This suggests strategic branding: pages may adopt names that resonate with specific audiences, even as they maintain a globally diverse operational team. It is also observed that 20% of the top 20 FCs by betweenness centrality are geographically localized. This observation serves as an illustrative fact about the network’s makeup: the prevalence or absence of explicit geographic references in FC usernames may reflect a conscious, strategic choice for broader appeal or thematic transcendence. Further, moderator involvement from various countries suggests a universal resonance of the discussions, despite initial impressions given by geolocalized indicators. In essence, while some Facebook communities may appear geographically or thematically localized, the overarching network intertwines global and local, highlighting the nuanced nature of discourse on the platform.
Figure 3 shows nVenn diagrams (see background description in Supplementary Section S8), which are a variant of traditional Venn diagrams that depict quasi-proportional areas representing the relative sizes of overlapping data sets (in this case, FCs categorized by discussion topics). This enables more accurate visual comparisons, especially for highly disparate set sizes. Additionally, nVenn diagrams effectively handle the visualization challenges inherent to comparing multiple (>3) sets, circumventing cluttered overlaps. While network or matrix diagrams could also elucidate topic interconnections (see Supplementary Section S9), mapping all possible topic combinations would require multidimensional matrices. Similarly, network diagrams encoding topics through node colorings or pie charts (see Supplementary Section S6) pose readability constraints when visualizing multiple topical overlaps.
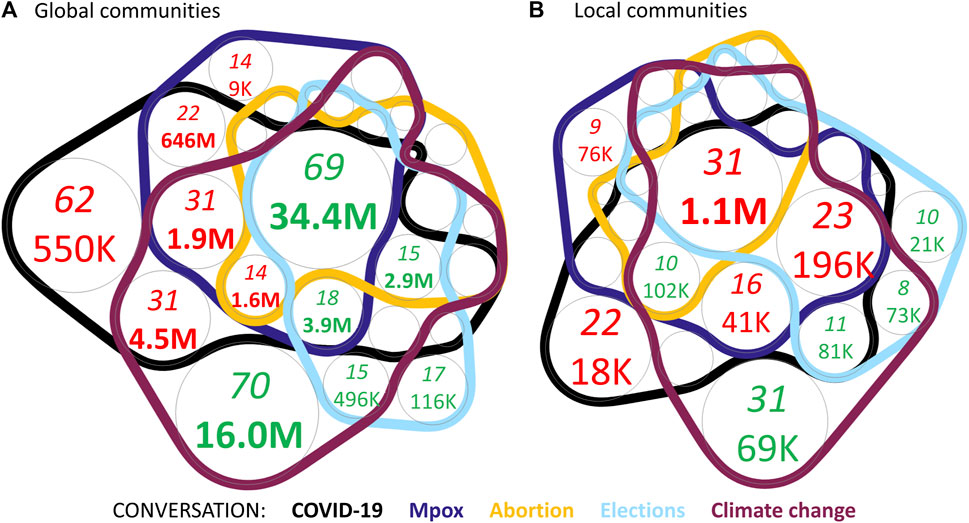
FIGURE 3. Topic mix within (A) global FCs, and (B) local FCs, shown using n-Venn diagrams [see Supplementary Section S8 for simple example explaining an n-Venn diagram (Pérez-Silva et al., 2018)]. Number of FCs in italics on top, with number of individuals on bottom (bolded if >1M). Number is shown in red (or green) if there is a prevalence of anti-vaccination (or neutral) communities. Only regions with >3% of total FCs have labels.
Thus Figures 3A, B highlight the significance of topic combinations in shaping public messaging. In total, an audience of 77.3 million individuals has engaged with one or more of these topics. The group of FCs discussing all five topics ranks as the second largest, reaching a staggering 35.5 million individuals. Neutral communities primarily drive this discourse, however in their absence, anti-vaccination communities would dominate the narrative. Out of 29 potential topic combinations, only three would be led by pro-vaccination communities without the influence of neutral voices.
A noticeable trend is the limited number of FCs focusing solely on topics like mpox, abortion, or elections. Meanwhile, discussions often intersect between COVID-19 and climate change, especially in global FCs. For locally oriented FCs, the pairing of elections and climate change emerges as a combination. Despite the participation of pro-vaccination communities, the discourse is frequently steered by FCs whose perspectives diverge from the prevailing scientific consensus—and in many cases, these are FCs that actively oppose it, especially at the local level. A case in point: while global FCs discussing all five topics are majority neutral, their local counterparts are majority anti-vaccination. Moreover, if neutral communities were excluded, six topic combinations for global FCs would be led by pro-vaccination voices. In contrast, this number shrinks to merely three for local FCs.
Overall, our comprehensive analysis of the Facebook vaccine-view network highlights its complexity and adaptability. In particular, our updated analysis of the original 2019 Facebook vaccine-view network reveals intricate patterns of interconnectedness, robustness, and thematic diversity. As global and local narratives intertwine, it emphasizes the platform’s complex web of voices, underscoring the importance of understanding online discourse at scale as a complex system and hence its broader implications.
4 Discussion
Our analysis of the evolving dynamics within the Facebook community network paints a vivid picture of the intricate and robust nature of online discourse, particularly concerning the 5 topics of COVID-19, mpox, abortions, elections, and climate change. The results underscore the network’s adaptive capacity, a trait that conventional mitigation strategies might struggle to address effectively.
The robustness observed in the network’s core structure, even amid external interventions, suggests a robust system that challenges traditional approaches to information dissemination and correction. Such robustness is emblematic of ecosystems that possess an inherent ability to maintain equilibrium, even when certain nodes are altered or removed. This robustness implies that singular, node-centric interventions, like the removal of certain Facebook communities, may only offer short-term solutions. For lasting impact, a broader, system-level approach is imperative. Specifically, our study reveals the multifaceted, interconnected structure of the online discourse on Facebook around topics that generate mis/disinformation and distrust. Its system-wide interconnected structure and robustness—despite Facebook’s many interventions and mitigation policies, particularly during COVID-19, e.g., around November 2020—suggest that traditional, isolated mitigation strategies to address mis/disinformation and distrust of medical expertise across Facebook may fall short. These findings also serve to confirm and extend our analyses that appeared in 2022 which showed that Facebook’s mitigations and policies during COVID-19 were inefficient in terms of disrupting this network of distrust (Illari et al., 2022; Johnson et al., 2022; Restrepo et al., 2022a; Restrepo et al., 2022b). This earlier finding (Restrepo et al., 2022a; Johnson et al., 2022) was subsequently confirmed by a later independent study (Broniatowski et al., 2023). Overall, our findings indicate a robust network structure resilient to external interventions. This observation challenges the efficacy of traditional information dissemination and correction strategies, emphasizing the need for more comprehensive, system-level approaches.
A significant takeaway from our research is the importance of geographic and thematic diversity in shaping online narratives. While global themes dominate the overarching discourse, local narratives play a crucial role in driving grassroots sentiments and actions. This “glocal” blend of global and local perspectives hints at the need for more nuanced and diversified messaging strategies. Rather than narrowly focusing on singular topics or scales, a combination of strategic topics, both local and global, might be more effective in addressing misinformation and fostering constructive discourse.
Furthermore, our analysis of topic combinations in shaping public messaging provides invaluable insights into the dynamics of online discussions. The intertwining of discussions, such as those between COVID-19 and climate change, suggests that FCs are not confined to siloed narratives. Instead, they weave multiple themes, drawing parallels or contrasts, a trait that mis/disinformation mitigation strategies should consider. The importance of geographic and thematic diversity in shaping online narratives is a key insight from our study. The interplay of local and global perspectives suggests strategic opportunities for nuanced and diversified messaging strategies.
While our dataset is robust, it is worth noting that it primarily captures select languages prevalent on Facebook. However, the diverse locations of page administrators suggest a broader geographic representation. Future research could delve deeper into sentiment analysis or employ natural language processing techniques for richer insights. Moreover, while Facebook is a significant platform, understanding discourse dynamics on other social platforms would provide a more holistic view of the online vaccine distrust ecosystem.
Many other studies being published on similar themes, use CrowdTangle which is a commercial application tool owned by Facebook, and hence have claims and findings that rely entirely on the hope that CrowdTangle is accurate [e.g., (Broniatowski et al., 2023)]. However, the researchers in such studies sit outside Facebook’s CrowdTangle team and hence have little knowledge or quantitative explanation of how and why this tool returns the results that it does. In other words, it is effectively a black-box tool, which makes it unacceptable for academic science research in our opinion. Worse still, it is widely reported that the CrowdTangle tool is no longer being properly maintained and hence any accuracy that it may arguably have had in the past has now likely degraded significantly. It may still be the best tool available, but the lack of such tools means that simply being best has no bearing on whether it is sufficiently reliable and accurate for scientific research–and hence whether the often subtle conclusions from such studies carry any weight. We further expand on this in the Supplementary Material [see also (Restrepo et al., 2022b) for our original critique]. In addition, we stress that our study builds and analyses a follower/like/social network based on the formal page follow/like connections on Facebook itself, whereas those other studies rely on a mention network in which the links are fleeting URLs of unknown relevance or importance at the scale of the Facebook community. Technically, we also note that it is not obvious how to create our kind of network using third-party tools such as CrowdTangle as used by these other studies.
Finally, we comment on how our study’s findings might help solve the problem of online distrust and its associated mis/disinformation without having to rely on social media platforms to finance costly human mitigation teams. It involves the novel idea proposed and later tested and presented by Restrepo et al. (2022a) (and earlier online public talks). In this scheme, the complex dynamical network of interlinked FCs that is presented in this current paper, can be used to guide the formation of small anonymous deliberation groups–with the remarkable result that softening of the extremes within such groups is observed to arise in the empirical studies (Restrepo et al., 2022a). Specifically, the extremes of the deliberation group get softened organically as long as the group contains individuals drawn fairly evenly from across the anti-, neutral and pro-spectrum. Moreover, this scheme could easily be automated at scale across social media platforms in a rapid and cost-effective way—which allows platforms to avoid engaging in top-down removal of FCs, or providing preventative messaging, or censorship. We refer to Ref. (Restrepo et al., 2022a) for full details, empirical and theoretical validation, and the core social science theory underpinning it. Hence the cost to social media platforms will be minimal—just the time cost of inviting individuals into new Facebook groups, for example, from the anti, neutral and pro communities. Importantly, this cost can be a minimal one since this process can be completely automated given the network map that we provide in this paper. We note that this remarkable effect appears to have been re-discovered and hence verified to some degree in a subsequent independent study by Abroms et al. (2023) in which Facebook groups effectively act as online deliberation groups.
In conclusion, our study offers a complex systems understanding of the Facebook distrust ecosystem and its post-pandemic shifts. Our findings underline the need to rethink current mitigation strategies, advocating instead for a more holistic approach that considers the network’s diverse and interconnected nature. Harnessing these insights could pave the way for more effective interventions.
Data availability statement
The original contributions presented in the study are included in the article/Supplementary Material, further inquiries can be directed to the corresponding author.
Author contributions
LI: Conceptualization, Formal Analysis, Investigation, Methodology, Resources, Software, Validation, Visualization, Writing–original draft, Writing–review and editing. NR: Data curation, Methodology, Validation, Visualization, Writing–original draft. NJ: Conceptualization, Formal Analysis, Funding acquisition, Investigation, Methodology, Project administration, Resources, Supervision, Validation, Visualization, Writing–original draft, Writing–review and editing.
Funding
The author(s) declare financial support was received for the research, authorship, and/or publication of this article. NJ is supported by U.S. Air Force Office of Scientific Research awards FA9550-20-1-0382 and FA9550-20-1-0383, and by The John Templeton Foundation in collaboration with S. Gavrilets.
Conflict of interest
The authors declare that the research was conducted in the absence of any commercial or financial relationships that could be construed as a potential conflict of interest.
Publisher’s note
All claims expressed in this article are solely those of the authors and do not necessarily represent those of their affiliated organizations, or those of the publisher, the editors and the reviewers. Any product that may be evaluated in this article, or claim that may be made by its manufacturer, is not guaranteed or endorsed by the publisher.
Supplementary material
The Supplementary Material for this article can be found online at: https://www.frontiersin.org/articles/10.3389/fcpxs.2023.1329857/full#supplementary-material
References
Abroms, L. C., Koban, D., Krishnan, N., Napolitano, M., Simmens, S., Caskey, B., et al. (2023). Empathic engagement with the COVID-19 vaccine hesitant in private Facebook groups: a randomized trial. Health Educ. Behav. 2023, 10901981231188313. doi:10.1177/10901981231188313
Ammari, T., and Schoenebeck, S. (2016). ““Thanks for your interest in our Facebook group, but it’s only for dads”: social Roles of Stay-at-Home Dads,” in Proceedings of the 19th ACM conference on computer-supported cooperative work & social computing CSCW ’16 (New York, NY, USA: Association for Computing Machinery), 1363–1375. doi:10.1145/2818048.2819927
Ardia, D. S., Ringel, E., Ekstrand, V., and Fox, A. (2020). Addressing the decline of local news, rise of platforms, and spread of mis- and disinformation online: a summary of current research and policy proposals. UNC Legal Studies Research Paper. doi:10.2139/ssrn.3765576
Arriagada, A., and Ibáñez, F. (2020). “You need at least one picture daily, if not, you’re dead”: content creators and platform evolution in the social media ecology. Soc. Media + Soc. 6, 205630512094462. doi:10.1177/2056305120944624
Boyle, P. (2022). Why do so many Americans distrust science? AAMC. Available at: https://www.aamc.org/news/why-do-so-many-americans-distrust-science (Accessed October 26, 2023).
Broniatowski, D. A., Simons, J. R., Gu, J., Jamison, A. M., and Abroms, L. C. (2023). The efficacy of Facebook’s vaccine misinformation policies and architecture during the COVID-19 pandemic. Sci. Adv. 9, eadh2132. doi:10.1126/sciadv.adh2132
Calleja, N., AbdAllah, A., Abad, N., Ahmed, N., Albarracin, D., Altieri, E., et al. (2021). A public health research agenda for managing infodemics: methods and results of the first WHO infodemiology conference. JMIR Infodemiology 1, e30979. doi:10.2196/30979
Centola, D., Becker, J., Brackbill, D., and Baronchelli, A. (2018). Experimental evidence for tipping points in social convention. Science 360, 1116–1119. doi:10.1126/science.aas8827
Chen, E., Lerman, K., and Ferrara, E. (2020). Tracking social media discourse about the COVID-19 pandemic: development of a public coronavirus twitter data set. JMIR Public Health Surveillance 6, e19273. doi:10.2196/19273
Chen, X., Sin, S.-C. J., Theng, Y.-L., and Lee, C. S. (2015a). “Why do social media users share misinformation?,” in Proceedings of the 15th ACM/IEEE-CS joint conference on digital libraries JCDL ’15 (New York, NY, USA: Association for Computing Machinery), 111–114. doi:10.1145/2756406.2756941
Chen, X., Sin, S.-C. J., Theng, Y.-L., and Lee, C. S. (2015b). Why students share misinformation on social media: motivation, gender, and study-level differences. J. Acad. Librariansh. 41, 583–592. doi:10.1016/j.acalib.2015.07.003
Czaplicka, A., Charalambous, C., Toral, R., and San Miguel, M. (2022). Biased-voter model: how persuasive a small group can be? Chaos, Solit. Fractals 161, 112363. doi:10.1016/j.chaos.2022.112363
Diaz-Diaz, F., San Miguel, M., and Meloni, S. (2022). Echo chambers and information transmission biases in homophilic and heterophilic networks. Sci. Rep. 12, 9350. doi:10.1038/s41598-022-13343-6
DiResta, R. (2018). The digital maginot line ribbonfarm. Available at: https://www.ribbonfarm.com/2018/11/28/the-digital-maginot-line/(Accessed March 28, 2023).
Douek, E. (2022). Content moderation as systems thinking. Harvard law review 136. Available at: https://harvardlawreview.org/print/vol-136/content-moderation-as-systems-thinking/(Accessed August 10, 2023).
EU (2023). Beyond disinformation – EU responses to the threat of foreign information manipulation. Available at: https://www.youtube.com/watch?v=YJf2pZGe36Q (Accessed March 28, 2023).
Freiling, I., Krause, N. M., Scheufele, D. A., and Brossard, D. (2023). Believing and sharing misinformation, fact-checks, and accurate information on social media: the role of anxiety during COVID-19. New Media & Soc. 25, 141–162. doi:10.1177/14614448211011451
Gelfand, M. J., Harrington, J. R., and Jackson, J. C. (2017). The strength of social norms across human groups. Perspect. Psychol. Sci. 12, 800–809. doi:10.1177/1745691617708631
Get the facts on coronavirus (2023). Full fact. Available at: https://fullfact.org/health/coronavirus/(Accessed March 14, 2023).
Green, Y., Gully, A., Roth, Y., Roy, A., Tucker, J. A., and Wanless, A. (2023). Evidence-based misinformation interventions: challenges and opportunities for measurement and collaboration carnegie endowment for international peace. Available at: https://carnegieendowment.org/2023/01/09/evidence-based-misinformation-interventions-challenges-and-opportunities-for-measurement-and-collaboration-pub-88661 (Accessed August 10, 2023).
Hootsuite (2021). Digital trends - digital marketing trends 2022 the global state of digital 2021. Available at: https://www.hootsuite.com/resources/digital-trends?utm_campaign=all-tier_1_campaigns-digital_2021-glo-none----smw_landing_page-en--q1_2021&utm_source=third_party&utm_medium=directory&utm_content=smw (Accessed March 14, 2023).
Illari, L., Restrepo, N. J., and Johnson, N. F. (2022). Losing the battle over best-science guidance early in a crisis: COVID-19 and beyond. Sci. Adv. 8, eabo8017. doi:10.1126/sciadv.abo8017
Jacomy, M., and Bastian, M. (2011). ForceAtlas2, A graph layout algorithm for handy network visualization. Available at: https://www.semanticscholar.org/paper/ForceAtlas2%2C-A-Graph-Layout-Algorithm-for-Handy-Jacomy-Bastian/ac53ade1902cb209493b541a29e65fab95bee497 (Accessed December 3, 2023).
Jacomy, M., Heymann, S., Venturini, T., and Bastian, M. (2012). ForceAtlas 2, A continuous graph layout algorithm for handy network visualization. Available at: https://www.semanticscholar.org/paper/ForceAtlas-2-%2C-A-Continuous-Graph-Layout-Algorithm-Jacomy-Heymann/6241886034d9a8cec981f6857a1dd1066b8290a0 (Accessed December 3, 2023).
Jacomy, M., Venturini, T., Heymann, S., and Bastian, M. (2014). ForceAtlas2, a continuous graph layout algorithm for handy network visualization designed for the Gephi software. PLOS ONE 9, e98679. doi:10.1371/journal.pone.0098679
Jilani, Z. (2022). AAAS 2022 annual meeting: how to tackle mis- and dis-information | American association for the advancement of science (AAAS). Available at: https://www.aaas.org/news/aaas-2022-annual-meeting-how-tackle-mis-and-dis-information (Accessed March 28, 2023).
Johnson, N. F., Jefferies, P., and Hui, P. M. (2003). Financial market complexity: what physics can tell us about market behaviour. Oxford, New York: Oxford University Press.
Johnson, N. F., Restrepo, E. M., and Illari, L. (2022). Focus on science. Available at: http://aps.org/publications/apsnews/202203/misinformation.cfm (Accessed December 4, 2023).
Johnson, N. F., Velásquez, N., Restrepo, N. J., Leahy, R., Gabriel, N., El Oud, S., et al. (2020). The online competition between pro- and anti-vaccination views. Nature 582, 230–233. doi:10.1038/s41586-020-2281-1
Kim, Y. A., and Ahmad, M. A. (2013). Trust, distrust and lack of confidence of users in online social media-sharing communities. Knowledge-Based Syst. 37, 438–450. doi:10.1016/j.knosys.2012.09.002
Klein, S., and Hostetter, M. (2023). Restoring trust in public health. New York, NY: Commonwealth Fund. doi:10.26099/j7jk-j805
Kleineberg, K.-K., and Boguñá, M. (2016). Competition between global and local online social networks. Sci. Rep. 6, 25116. doi:10.1038/srep25116
Lappas, G., Triantafillidou, A., Deligiaouri, A., and Kleftodimos, A. (2018). Facebook content strategies and citizens’ online engagement: the case of Greek local governments. Rev. Socionetwork Strat. 12, 1–20. doi:10.1007/s12626-018-0017-6
Larson, H. J. (2020). Blocking information on COVID-19 can fuel the spread of misinformation. Nature 580, 306. doi:10.1038/d41586-020-00920-w
Laws, R., Walsh, A. D., Hesketh, K. D., Downing, K. L., Kuswara, K., and Campbell, K. J. (2019). Differences between mothers and fathers of young children in their use of the internet to support healthy family lifestyle behaviors: cross-sectional study. J. Med. Internet Res. 21, e11454. doi:10.2196/11454
Lazer, D. M. J., Baum, M. A., Benkler, Y., Berinsky, A. J., Greenhill, K. M., Menczer, F., et al. (2018). The science of fake news. Science 359, 1094–1096. doi:10.1126/science.aao2998
Lewandowsky, J., Stephan, C., Ecker, U., Albarracín, D., Amazeen, M. A., Kendeou, P., et al. (2020). Debunking handbook 2020. Fairfax, VA: George Mason University. Available at: https://www.climatechangecommunication.org/wp-content/uploads/2020/10/DebunkingHandbook2020.pdf (Accessed March 28, 2023).
Madhusoodanan, J. (2022). Safe space: online groups lift up women in tech. Nature 611, 839–841. doi:10.1038/d41586-022-03798-y
Mazzeo, V., and Rapisarda, A. (2022). Investigating fake and reliable news sources using complex networks analysis. Front. Phys. 10, 886544. doi:10.3389/fphy.2022.886544
Mazzeo, V., Rapisarda, A., and Giuffrida, G. (2021). Detection of fake news on COVID-19 on web search engines. Front. Phys. 9, 685730. doi:10.3389/fphy.2021.685730
Meta (2020). Keeping our services stable and reliable during the COVID-19 outbreak meta. Available at: https://about.fb.com/news/2020/03/keeping-our-apps-stable-during-covid-19/(Accessed March 14, 2023).
Meyers, C. (2022). American physical society takes on scientific misinformation. Available at: http://aps.org/publications/apsnews/202203/misinformation.cfm (Accessed March 14, 2023).
Mirza, S., Begum, L., Niu, L., Pardo, S., Abouzied, A., Papotti, P., et al. (2023). “Tactics, threats & targets: modeling disinformation and its mitigation,” in Proceedings 2023 network and distributed system security symposium (San Diego, CA, USA: Internet Society). doi:10.14722/ndss.2023.23657
Moon, R. Y., Mathews, A., Oden, R., and Carlin, R. (2019). Mothers’ perceptions of the internet and social media as sources of parenting and health information: qualitative study. J. Med. Internet Res. 21, e14289. doi:10.2196/14289
NewsDirect (2021). TRUST science pledge calls for public to engage in scientific literacy news direct. Available at: https://newsdirect.com/news/trust-science-pledge-calls-for-public-to-engage-in-scientific-literacy-737528151 (Accessed March 14, 2023).
Nobel Prize Summit (2023). NobelPrize.org. Available at: https://www.nobelprize.org/events/nobel-prize-summit/2023/(Accessed March 28, 2023).
Pérez-Silva, J. G., Araujo-Voces, M., and Quesada, V. (2018). nVenn: generalized, quasi-proportional Venn and Euler diagrams. Bioinformatics 34, 2322–2324. doi:10.1093/bioinformatics/bty109
Pertwee, E., Simas, C., and Larson, H. J. (2022). An epidemic of uncertainty: rumors, conspiracy theories and vaccine hesitancy. Nat. Med. 28, 456–459. doi:10.1038/s41591-022-01728-z
Plique, G. (2021). Graphology ForceAtlas2 graphology. Available at: https://graphology.github.io/standard-library/layout-forceatlas2.html (Accessed December 3, 2023).
RAND Corporation (2019). What’s being done to fight disinformation online. Available at: https://www.rand.org/research/projects/truth-decay/fighting-disinformation.html (Accessed March 28, 2023).
Rao, A., Morstatter, F., and Lerman, K. (2022). Partisan asymmetries in exposure to misinformation. Sci. Rep. 12, 15671. doi:10.1038/s41598-022-19837-7
Ravenelle, A. J., Newell, A., and Kowalski, K. C. (2021). “The looming, crazy stalker coronavirus”: fear mongering, fake news, and the diffusion of distrust. Socius 7, 237802312110247. doi:10.1177/23780231211024776
Restrepo, E. M., Moreno, M., Illari, L., and Johnson, N. F. (2022a). Softening online extremes organically and at scale. arXiv. doi:10.48550/arXiv.2207.10063
Restrepo, N. J., Illari, L., Leahy, R., Sear, R. F., Lupu, Y., and Johnson, N. F. (2022b). How social media machinery pulled mainstream parenting communities closer to extremes and their misinformation during covid-19. IEEE Access 10, 2330–2344. doi:10.1109/ACCESS.2021.3138982
Roozenbeek, J., van der Linden, S., Goldberg, B., Rathje, S., and Lewandowsky, S. (2022). Psychological inoculation improves resilience against misinformation on social media. Sci. Adv. 8, eabo6254. doi:10.1126/sciadv.abo6254
San Miguel, M., and Toral, R. (2020). Introduction to the chaos focus issue on the dynamics of social systems. Chaos (Woodbury, N.Y.) 30, 120401. doi:10.1063/5.0037137
Semenov, A., Mantzaris, A. V., Nikolaev, A., Veremyev, A., Veijalainen, J., Pasiliao, E. L., et al. (2019). Exploring social media network landscape of post-soviet space. IEEE Access 7, 411–426. doi:10.1109/ACCESS.2018.2885479
Smith, R., Cubbon, S., and Wardle, C. (2020). Under the surface: Covid-19 vaccine narratives, misinformation and data deficits on social media First Draft. Available at: https://firstdraftnews.org/long-form-article/under-the-surface-covid-19-vaccine-narratives-misinformation-and-data-deficits-on-social-media/(Accessed March 28, 2023).
Society of Editors (2023). Government to relaunch ‘Don’t Feed the Beast’ campaign to tackle Covid-19 misinformation. Available at: https://www.societyofeditors.org/soe_news/government-to-relaunch-dont-feed-the-beast-campaign-to-tackle-covid-19-misinformation/(Accessed March 14, 2023).
Song, H., So, J., Shim, M., Kim, J., Kim, E., and Lee, K. (2023). What message features influence the intention to share misinformation about COVID-19 on social media? The role of efficacy and novelty. Comput. Hum. Behav. 138, 107439. doi:10.1016/j.chb.2022.107439
Starbird, K., Spiro, E. S., and Koltai, K. (2020). Misinformation, crisis, and public health—reviewing the literature. Social Science Research Council, MediaWell. https://mediawell.ssrc.org/literature-reviews/misinformation-crisis-and-public-health doi:10.35650/MD.2063.d.2020
Taylor, T. E., Rhodes, H., Garcilazo Green, L., and Ryan, L. (2023). “Understanding and addressing misinformation about science A public workshop,” in National academy of sciences building 2101 constitution ave NW (Washington DC 20418 USA: The National Academies). Available at: https://www.nationalacademies.org/event/04-19-2023/understanding-and-addressing-misinformation-about-science-a-public-workshop (Accessed March 28, 2023).
The National Academies (2023). Navigating infodemics and building trust during public health emergencies A workshop. Available at: https://www.nationalacademies.org/event/04-10-2023/navigating-infodemics-and-building-trust-during-public-health-emergencies-a-workshop (Accessed March 28, 2023).
The Rockefeller Foundation (2021). New USD10 million project launched to combat the growing mis- and disinformation crisis in public health the rockefeller foundation. Available at: https://www.rockefellerfoundation.org/news/new-usd10-million-project-launched-to-combat-the-growing-mis-and-disinformation-crisis-in-public-health/(Accessed March 14, 2023).
Tram, K. H., Saeed, S., Bradley, C., Fox, B., Eshun-Wilson, I., Mody, A., et al. (2022). Deliberation, dissent, and distrust: understanding distinct drivers of coronavirus disease 2019 vaccine hesitancy in the United States. Clin. Infect. Dis. 74, 1429–1441. doi:10.1093/cid/ciab633
Tria, F., Loreto, V., and Servedio, V. D. P. (2018). Zipf’s, heaps’ and taylor’s Laws are determined by the expansion into the adjacent possible. Entropy (Basel) 20, 752. doi:10.3390/e20100752
van der Linden, S., Leiserowitz, A., Rosenthal, S., and Maibach, E. (2017). Inoculating the public against misinformation about climate change. Glob. Challenges 1, 1600008. doi:10.1002/gch2.201600008
VQF (2023). Github. Available at: https://github.com/vqf/nVennR (Accessed October 27, 2023).
Wardle, C. (2023). Getting ahead of misinformation democracy journal. Available at: https://democracyjournal.org/magazine/68/getting-ahead-of-misinformation/(Accessed March 28, 2023).
WHO (2020). Managing the COVID-19 infodemic: promoting healthy behaviours and mitigating the harm from misinformation and disinformation. Available at: https://www.who.int/news/item/23-09-2020-managing-the-covid-19-infodemic-promoting-healthy-behaviours-and-mitigating-the-harm-from-misinformation-and-disinformation (Accessed March 14, 2023).
Wone, A. (2020). VERIFIED: UN launches new global initiative to combat misinformation Africa Renewal. Available at: https://www.un.org/africarenewal/news/coronavirus/covid-19-united-nations-launches-global-initiative-combat-misinformation (Accessed March 14, 2023).
Keywords: betweenness centrality, complex adaptive systems, digital discourse dynamics, holistic strategies, network analysis, online misinformation, robustness, social media dynamics
Citation: Illari L, Restrepo NJ and Johnson NF (2024) Complexity of the online distrust ecosystem and its evolution. Front. Complex Syst. 1:1329857. doi: 10.3389/fcpxs.2023.1329857
Received: 30 October 2023; Accepted: 22 December 2023;
Published: 09 January 2024.
Edited by:
Francesca Tria, Sapienza University of Rome, ItalyReviewed by:
János Török, Budapest University of Technology and Economics, HungarySalvatore Micciche', University of Palermo, Italy
Copyright © 2024 Illari, Restrepo and Johnson. This is an open-access article distributed under the terms of the Creative Commons Attribution License (CC BY). The use, distribution or reproduction in other forums is permitted, provided the original author(s) and the copyright owner(s) are credited and that the original publication in this journal is cited, in accordance with accepted academic practice. No use, distribution or reproduction is permitted which does not comply with these terms.
*Correspondence: Neil F. Johnson, neiljohnson@gwu.edu