- 1Flowers Team Inria and Ensta ParisTech, Bordeaux, France
- 2Philosophy Department University of Kentucky, Lexington, KY, United States
- 3International Labour Organisation, Research Department, Switzerland and Geneva Macro Labs, Geneva, Switzerland
- 4Peregrine Data Inc., Mumbai, India
- 5Corteva Agriscience (US), Indianapolis, IN, United States
- 6Boston College, Chestnut Hill, MA, United States
- 7Avista Therpeutics, Pittsburgh, PA, United States
- 8UK Centre for Ecology and Hydrology Lancaster, Lancaster, United Kingdom
- 9Max Planck Institute for Evolutionary Biology, Plön, Schleswig-Holstein, Germany
- 10Programa de Doctorado en Genómica Integrativa Vicerrectoría de Investigación GEMA Center for Genomics, Ecology & Environment Universidad Mayor Santiago, Santiago, Chile
- 11Konrad Lorenz Institute for Evolution and Cognition Research, Klosterneuburg, Austria
The term “resilience” has risen in popularity following a series of natural disasters, the impacts of climate change, and the Covid-19 pandemic. However, different disciplines use the term in widely different ways, resulting in confusion regarding how the term is used and difficulties operationalising the underlying concept. Drawing on an overview of eleven disciplines, our paper offers a guiding framework to navigate this ambiguity by suggesting a novel typology of resilience using an information-theoretic approach. Specifically, we define resilience by borrowing an existing definition of individuals as sub-systems within multi-scale systems that exhibit temporal integrity amidst interactions with the environment. We quantify resilience as the ability of individuals to maintain fitness in the face of endogenous and exogenous disturbances. In particular, we distinguish between four different types of resilience: (i) preservation of structure and function, which we call “strong robustness”; (ii) preservation of function but change in structure (“weak robustness”); (iii) change in both structure and function (“strong adaptability”); and (iv) change in function but preservation in structure (“weak adaptability”). Our typology offers an approach for navigating these different types and demonstrates how resilience can be operationalised across disciplines.
1 Introduction
Resilience has received renewed attention, particularly since the outbreak of the COVID-19 pandemic and the rising impact of climate change (see Figure 1, also Mcaslan, 2010). However, the varying use of the term “resilience” across academic disciplines has defied a unifying approach despite shared features. This paper approaches resilience from a complex adaptive systems point of view in order to 1) clarify the meaning of resilience across disciplines, 2) operationalise insights across disciplines that help identify and address common features of dynamic systems and 3) develop a typology of different forms of resilience to facilitate interdisciplinary collaboration on real-world problems.
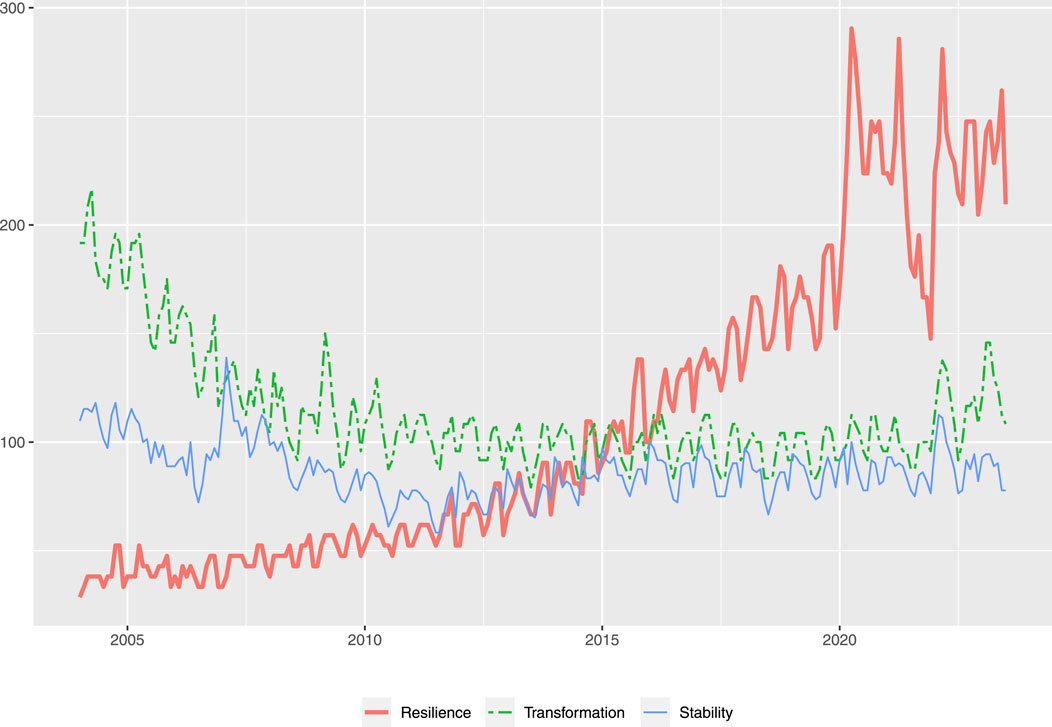
FIGURE 1. Rising interest in resilience.Note: Based on Google Trends monthly data between January 2004 and July 2023. Comparing the appearance of the search terms “resilience” as opposed to “transformation” and “stability” in worldwide Google searches. Values have been indexed w.r.t. January 2016 [Accessed: 27 July 2023].
To develop our typology, we conducted an exploratory literature review across 11 disciplines—agriculture; ecology and evolutionary biology; economics and finance; education and psychology; engineering; health systems; international development; international trade; physiology and cell biology; policy design; sustainability science—with a focus on the dynamic properties that each discipline emphasizes in its study of resilience. Our study reveals that disciplines vary widely in the features they choose to analyse and the weight they give to them (see Figure 2). At one extreme, for instance, engineering emphasizes maintenance of function, predictability, defined interactions, responses to shocks, and equilibrium dynamics. Social disciplines tend to use a broader application of resilience with an emphasis on transformation, unpredictability, adaptability and non-equilibrium dynamics. Across disciplines, terms related to stability—adaptability, structure, and feedback—are considered the most relevant to resilience, while concepts related to the fluidity or dynamic behavior of systems—multiple equilibria, transformativity, modularity, and self-organization—are considered to receive the least attention or only be relevant for certain disciplines. Without a clear definition of the term ‘resilience’ and considering the variety of viewpoints, opening up cross-disciplinary dialogues between these fields seems difficult.
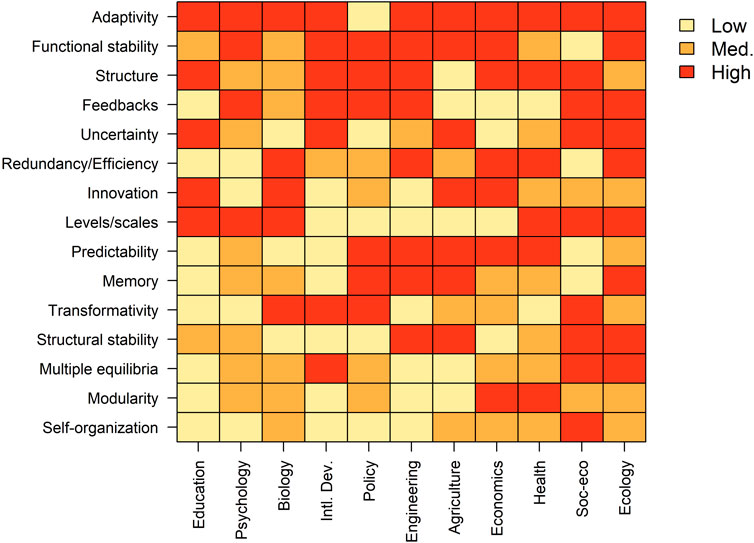
FIGURE 2. Resilience across disciplines. Note: The relative importance or centrality of concepts related to resilience in a number of disciplines. Ratings are based on a structured elicitation of the authors after reviewing non-systematically selected example studies in each discipline. Disciplines are ordered left to right in increasing average importance across concepts. Concepts are ordered bottom to top in increasing prevalence among disciplines.
Yet our review has also revealed that, despite their differences, there are recurring patterns across disciplines. In each area of study, processes of recognizing the object of resilience, understanding its structure and its interaction with its environment, and analyzing its ability to react to change are present, albeit formalized to different extents. A definition of resilience that abstracts away domain-specific particularities could be versatile and useful enough to benefit future studies and cross-disciplinary dialogue. We recognize that some of these domain-specific particularities are rooted in epistemological differences that defy a fully unifying typology. Thus, we offer our definition as a way to operationalize resilience as a boundary concept in cross-disciplinary dialogue by finding common yet differentiated features.
We consider that a certain number of key characteristics of resilient systems—adaptability, functional and structural stability—seems to be retained across disciplines (see Figure 2). These can be used to deduct some general principles regarding the behaviour of dynamical systems to understand their evolution over time and their reaction vis-à-vis exogenous and endogenous shocks. In particular, our approach aims at categorising and measuring system-wide properties that characterise a system’s behaviour in reaction to a shock. At the same time, we do not attempt to give in to a reductionist view of resilience by making it a single metric that fully characterises a complex system (Morin, 2008). Rather, our main objective is to establish a cross-disciplinary dialogue around the notion of “resilience” by providing elements of an abstract conceptualisation without giving it a detailed mathematical treatment as in Krakovská et al. (2023). In this regard, we consider our formalisation of resilience as context-specific, a point that we will develop further below in the section ‘Discussion’.
Our approach is inspired by an information-theoretic perspective of systems dynamics (Krakauer et al., 2020). In general, an information-theoretic approach studies processes of information exchange to characterize systems in terms of their entropy, i.e., the uncertainty over the states they can adopt. By doing so it emphasises the dynamic nature of the system, in contrast to static approaches that focus on properties of systems that remain stable over time. In particular, we build upon the information-theoretic formalisation of individuality suggested by Krakauer et al. (2020), and associate resilience with the ability of the system to maintain its fitness, characterised as a system’s capacity to maintain the transmission of information within specified bounds in the face of disturbance. Our conceptualisation relies on a system’s structure and function: structure is a model of the internal components of a system and their interactions, while function is the process by which the system interacts with its environment and determines its fitness. In this respect, a system that falls below a certain fitness threshold lacks resilience as it loses its function.
The structure of complex systems, such as biological organisms, socio-ecological systems and human cities, is multi-layered and encompasses multiple scales across space and time (Flack, 2017; Krakauer et al., 2020). Changes in function, and thus fitness, may require a change in structure. Transformation in the system’s structure seems to be fundamental for its resilience, yet not all transformation is considered to be a sign of resilience. For example, the appearance of new levels of organization that aggregate information from lower levels (such as proteins arising after RNA) can improve an organism’s ability to predict its environment and, thus, increase its fitness (Flack et al., 2013). In contrast, ecosystems can lose resilience and experience significant reductions in biodiversity but still survive, albeit at a lower level of “fitness” when assessed against criteria such as biomass, capacity to absorb carbon emissions or other. By quantifying resilience in terms of fitness and allowing for changes in structure and function, our definition enables the study of such systems, while distinguishing it from other concepts such as transformation or adaptability.
We begin with a general information-theoretic definition of resilience. However, as our multi-disciplinary review suggests, practitioners from different disciplines face different constraints, so that operationalizing resilience under a single discipline-independent metric will prove of little practical value. For this reason we propose four types of resilience that differ in the constraints on change in structure and function they allow. Specifically, a dynamic system that maintains a minimum level of fitness over a certain time period is said to be:
1) Strongly robust when it maintains both its structure and function. This is the most conservative type of resilience;
2) Weakly robust when it allows change in structure but not in function;
3) Strongly adaptable when it allows change in both structure and function;
4) Weakly adaptable when it allows change in function but not in structure.
In the remainder of the paper, we provide a broad overview of the disciplines, studying each one in isolation to explain how our literature review led to the meta-analysis in Figure 2. Then, we provide our information-theoretic definition of resilience and formulate the four types of resilience. To get an intuition of how these types relate to real-world studies, in the Supplementary Material we provide illustrative examples of how different disciplines study resilience, this time through the lens of our own definition.
2 Overview of concepts of resilience in different fields
2.1 Agriculture
Resilience in farm-level agriculture is commonly used in the sense of improving the stability of production. Examples include excess application of fertilizer to guard against loss in case of heavy precipitation (Smith and Goodwin, 1996) or the use of cover crops for conservation of soil moisture under intermittent drought (Basche and Edelson, 2017). Social and economic studies of agriculture are more likely to use the concept of resilience in complex systems terms. For instance, farm size affects access to capital, which in turn affects adaptive capacity and the ability to take advantage of policy incentives for agricultural innovation (Kuhmonen, 2020).
2.2 Ecology and evolutionary biology
Resilience in ecology describes the ecosystem’s ability to absorb or mitigate environmental perturbations such as nutrient enrichment, natural disturbances, species introductions, and land use conversion. Ecosystem science is fundamentally about the flux of matter and energy through natural systems. As such, resilience is about the maintenance of flux rates. The field also investigates the link between ecosystem structure—types and number of species—and its functionality to address the question of whether preservation of system composition is essential (Holling, 1973). Population and community ecology fields are less likely to use resilience as a concept, but are nonetheless concerned with its components, including stable equilibria (Chesson, 2000) and emergent stability in disequilibrium systems (MacArthur and Wilson, 1967; Levins, 1970).
In evolutionary biology, robustness, a concept similar to resilience, is used to define the persistence of a specific phenotypic trait under perturbation. The phenotypic trait in question may be a gene function, physiological or anatomical trait, behaviour, or any other trait influenced by genetics. Perturbations might occur at the level of individual genes, through a recombination of different genes or driven by environmental changes. The robustness of developmental pathways to develop the same phenotype (i.e., function) regardless of the genetic background or environmental conditions (i.e., structure) is known as canalization (Waddington, 1942).
Terms have emerged to distinguish types of resilience. For instance, “mutational resilience” indicates a protein can accumulate mutations without losing function (Tenthorey et al., 2020). Resilience is also explicitly used in describing the insensitivity of protein interaction networks to node removal (gene loss) (Zitnik et al., 2019). In this sense, robustness and resilience are understood as the ability of an entity to tolerate endogenous changes and maintain the same output. Resilience through adaptability is also a theme, wherein exposure to an external stressor (such as low doses of antibiotics) can facilitate the evolution of novel functionality (e.g., antibiotic resistance).
2.3 Economics and finance
In the economics and finance literature, resilience is a term used to describe the robustness to shocks and fast recovery of the market. A related concept is anti-fragility, which assumes a creative destruction of the previous state that allows for a new, better state to establish itself. An example would be the cleansing effect of recessions whereby resources are transferred from unprofitable to more profitable firms during economic downturns (Caballero and Hammour, 1994) or the process of firm entry and exit through innovation (Aghion and Howitt, 1992).
Economists have started looking into the role of redundancy in economic systems as a shock absorber, which can help the system to rebound more rapidly (“potential capital,” see Eberly et al., 2021; Hynes et al., 2022). By and large, however, resilience is only understood as the extent to which a system can withstand a shock, and the speed with which a system returns to the status-quo-ante. As such, it is defined with respect to a presumed steady-state of the system at which equilibrium is obtained but not as a systemic feature of the equilibrium itself (e.g., Brunnermeier, 2021).
2.4 Education, neuroscience and psychology
Resilience is the ability of learners, schools, communities and institutions to identify, absorb, and adapt to challenges, and to transform in the face of adversity, with the functional standard of sustained, measurable improvements and equitable access to all learners. For instance, the United States Agency for International Development (USAID) defines resilience in its Policy and Program Guidance on Building Resilience to Recurrent Crisis (USAID, 2012, p. 5) as the “ability of people, households, communities, countries, and systems to mitigate, adapt to, and recover from shocks and stresses in a manner that reduces chronic vulnerability and facilitates inclusive growth.”
Similarly, in neuroscience, resilience is seen as “the ability to achieve a successful outcome in the face of adversity” (Hunter et al., 2018) while in psychology resilience is the capacity and dynamic process of adaptively overcoming stress and adversity while maintaining normal psychological and physical functioning.
2.5 Engineering
In engineering, resilience is mostly used in the context of critical infrastructure systems. Here, it is regarded as the ability of such systems to resist—either prevent or withstand—any possible hazards, absorb the initial damage, and recover to normal operation (Smith et al., 2011). In other words, a resilient infrastructure is expected to return to equilibrium following a disturbance. In the context of networks, like the Internet, resilience is the ability of a network to defend itself and maintain an acceptable level of service in the presence of exogenous challenges.
Resilience cuts through many thematic areas, such as information and network security, fault tolerance, software dependability, operating costs, and network survival. The focus has been on building an operational framework which will help the system become more resilient with respect to specific failure scenarios. One type of scenario that has received a lot of attention has been malicious attacks on communication networks. There have been broadly two approaches to making engineering systems more resilient. One approach is to incorporate resilience as a design goal. This includes changing the topology of a network or creating redundancy of infrastructure. The other approach is to add specific sub-systems to increase resilience. These could be security mechanisms like secure VPN connections between sites, authentication protocols and firewalls. It also includes mechanisms that aid recovery in the event of failure.
2.6 Health systems
Health systems include a large set of actors and actions working towards health improvement, including and beyond the provision of health care services. This leads to a diversity of views on resilience (Turenne et al., 2019). In its broadest sense, resilience in health systems refers to its ability to prepare for, manage (absorb, adapt and transform), withstand and learn from shocks, sudden and extreme changes to the context (Thomas et al., 2020). This includes the capacity to maintain essential functions during a crisis. Essential functions include governance, financing, resource generation, and service delivery according to the Health System Performance Assessment Framework for universal Healthcare supported by the World Health Organization (Papanicolas et al., 2022). The framework may be used to measure resilience indirectly, by assessing a health system’s performance and goal attainment before and after a shock (Sagan et al., 2021).
Health systems are often characterised by path-dependence in their organization and financing, leading to a shift in resilience over time. Early political choices of health financing and/or health delivery options tend to “lock-in” the country on a path that resists major change (e.g., the single-payer/provider system in the United Kingdom vs market-based hybrid system in the United States). Also, the resilience of health systems likely needs to evolve over time to reflect the rising needs of populations as a country develops (Bhandari and Alonge, 2020).
2.7 International development
Development resilience is the “capacity over time of a person, household or other aggregate unit to avoid poverty in the face of various stressors or shocks. If and only if that capacity is and remains high over time, then the unit is resilient.” (Barrett and Constas, 2014). Resilience in international development focuses on the evolution of individual and collective human wellbeing, in particular the capacity to avoid and escape from unacceptably low standards of living (poverty, for short) over time and in the face of stressors and shocks.
International development was more narrowly focused on economic development in the 20th century when the post-world-war decolonization took place. Over time the focus was broadened to other aspects of wellbeing, and a more multi-dimensional metric—the Human Development Index—was adopted by the United Nations Development Program (Stanton, 2007). However, it was realized that pervasive external shocks like climate change have a different impact on different countries not only because of the differences in physical changes but also due to the differences in resilience of the political systems of nations. The term climate coloniality is often used to denote the differential impact on post-colonial nations (located primarily in the tropics and subtropics where climate-induced disasters and shifts have been prevalent for some time). The Sustainable Development Goals (SDGs), also known as the Global Goals, were adopted by the United Nations in 2015 as a universal call to action to end poverty, protect the planet, and ensure that by 2030 all people enjoy peace and prosperity.1 These goals cannot be met without changing global social and economic relations to address climate change and mitigate its impacts. There is a robust discussion going on in this field on how to increase resilience and how much structural change is required. This leads to an interesting debate on the relationship between structural change or transformation and its relationship to resilience.
2.8 International trade
The disruption in international trade during and in the aftermath of the COVID-19 pandemic has produced some reflection on resilience in global supply chains. One of the pervasive features of international trade is the tightly knit chain of suppliers that can produce ripple effects even when only one supplier fails to deliver (on time). Public-health-related restrictions on activity and mobility during the pandemic both depressed the output of individual suppliers and prevented timely transportation of goods. Researchers in this area distinguish between resilience as “the ability to return to normal operations over an acceptable period of time, post-disruption” from robustness as “the ability to maintain operations during a crisis” (Brandon-Jones et al., 2014; Miroudot, 2020). This trade-off between resilience and robustness can be understood as the difference between the structure of the network of global suppliers (“resilience”) vs. their individual capacity to maintain a certain level of output, for instance as part of their commitment to a long-term supply relationship (Jain et al., 2021). In other words, different forms of resilience/robustness involve adjustments at different levels of the hierarchy of global supply chains. This conceptualisation implies an equilibrium state of affairs with resilience defined as a swift return to this steady state.
2.9 Physiology and cell biology
In physiology and cell biology, the term resilience is not often used, but a strong analog of it, homeostasis, is ubiquitous. Homeostasis is defined as the stable range of internal physical and chemical conditions maintained by regulatory mechanisms within the organism in face of environmental changes (Billman, 2020). It encompasses regulatory mechanisms at the cell, tissue, organ and the organism level. Implicitly, homeostasis assumes the organism returns to the basal level of internal conditions after dealing with an exogenous shock, i.e., the system returns to its previous state and does not change or improve its function.
In immunology, “resilience is the process that allows individuals to adapt to adverse conditions and recover from them,” for instance through exposing the immune system to pathogens (Dantzer et al., 2018). This improved function of the immune system exposed to pathogens compared to a naive immune system stems from immunologic memory. Antigens that previously activated the immune system are remembered and a more intense immune reaction is launched the second time the antigen is encountered. This is an example of an adaptable system, which improves its function after being subjected to a shock irrespective of changes in system structure.
2.10 Policy design
In policy design, resilience is a successful transition to a new, desired steady state. The key issue here is that the transition often involves the change in several components of the existing system due to policy complementarities (Roland, 2002). Only once all changes have been made will the system acquire a new functionality. Often, policy makers try to solve problems by implementing several changes at the same time (“revolution,” large reform packages). In most cases, this might not be feasible, or it fails because resistance against change builds up against some of the sub-components. In this case, the reform gets only partially implemented or not at all. In other situations, the order in which policies are implemented will determine the success of the reform process. In this case, resilience does not stem from the internal structure of the system but rather from the type and timing of policy (shocks) (Bednar, 2016; Capano and Woo, 2017).
2.11 Sustainability science
The social-ecological systems (SES) lens has become prominent in sustainability science, which views linked human and natural systems as complex adaptive systems. From this lens, resilience is the capacity of a system to absorb disturbance and reorganize while undergoing change so as to retain essentially the same function, structure, identity, and feedbacks. In other words, resilience is the capacity of a system, be it an individual, a forest, a city or an economy, to “cope with shocks and to keep functioning in much the same kind of way” (Walker, 2020). Sustainability scientists use the metaphor of a stability landscape with multiple basins of attraction to further characterize the dynamics of change in SESs. More recently, the lens of ‘resilience thinking’ focuses on three central aspects of the dynamics of change in SESs: resilience, adaptability, and transformability. Here, resilience is the capacity of an SES to continuously change and adapt to remain within these “critical thresholds.” Adaptability contributes to such resilience, while transformability represents the capacity to cross thresholds (Folke et al., 2010). Amid calls for transformative change to address the root cause of environmental crises like biodiversity loss and climate change, emerging research focuses on characterizing the transformative capacities of system agents and structures (Wilson et al., 2013). This perspective stands apart from less formal applications of the resilience concept for its focus on continuous rather than episodic change.
2.12 Summary
Overall, the concept of resilience links to complex system analysis, but different disciplines and sectors have developed their own interpretations according to their own needs and conventions. As a result, “resilience” has become a catch-all term to explain how or why states of affairs return to “normalcy” after a disturbance occurs. The imprecision and lack of overarching definitions of the term creates challenges communicating across fields and presenting the risk that the term resilience becomes less meaningful. Our summary overview of different disciplines attempts to explore this ambiguity surrounding the term “resilience” to provide a rough and ready playbook for our own conceptualisation that we present in the next section.
3 Modeling a resilient system
To define resilience, we first need to define the system whose resilience we are interested in studying. To do so, we consider systems as multi-level individuals that maintain integrated information flows over time, a conceptualisation inspired by recent work by Krakauer et al. (2020) in which individuality can be continuous, emerge at any level of organisation and can be nested.
In a more concise way, Krakauer et al. (2020) define individuals as “aggregates that ‘propagate’ information from the past to the future and have temporal integrity.” Individuals, in this regard, are characterised by their capacity to transmit information over time while interacting with their environment. Moreover, individuals transmit information that evolve at different speeds and are updated at different frequencies, which gives rise to the concept of “complex time,” (Flack et al., 2013) and to multi-level information dynamics. In the following we refer to such systems as multi-layered individuals.
We identify resilience with the capacity of a system to maintain integrity of the various levels of information flows when interacting with its environment. In other words, a resilient system is one that is able to survive, i.e., to maintain a minimum fitness level, while being exposed to new information arising from the environment (see Figure 3 that presents the main components of our mathematical model). The degree to which it is capable of doing so defines its resilience.
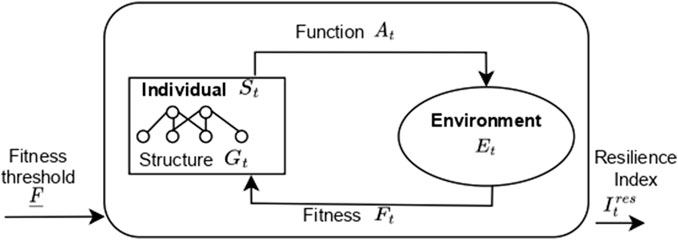
FIGURE 3. Modeling resilience. Note: The environment and individual are characterized by their states Et and St, which are time-dependent, multi-dimensional random variables. We model the state of the system as a joint function of two parameters: its structure G, which determines how the dimensions of the state interact with each other and has a hierarchical form, and function A, which captures the behavior of the system. The structure G is internal to the system and often has a multi-layered form, as the individual is processing information at multiple spatial and temporal scales. The function A represents an information flow from the system to its environment. This flow incurs another flow in the opposite direction: a function applied on the environment affects the fitness F of the system, which is associated with the ability of the system to predict its future state.
In order to survive, systems in this model can make use of the information they receive to predict their environment and their future self. To enable transmission of information, the system needs to be equipped with mechanisms that ensure the fidelity of transmission. Examples of such mechanisms in the real world are the DNA replication mechanism employed by biological organisms and an institution’s norms and protocols. In this work we view such mechanisms as the means of resilience and the survival of the individual as the objective of resilience. We proceed with a formal definition of the components of this model and then provide our definition of resilience.
We consider a system and an environment that share a boundary with each other and evolve over time.2 The state of the system and the environment can be modelled as time-dependent, multi-dimensional, real-valued random variables St and Et, respectively. In what follows, we consider that a random variable X takes values xi with probability p (xi).
3.1 Background
In the rest of our analysis we will make use of four information-theoretic measures.
• H(X) is the entropy of random variable X which quantifies our uncertainty about its state. Formally, H(X) = ∑ip(xi) log2p(xi) where we sum over all possible states xi;
• H (X; Y) is the joint entropy between variables X and Y, which is at maximum when there is no relationship between the two. Formally, H (X; Y) = ∑i ∑jp (xi, yj) log2p (xi, yj);
• H(Y|X) is the conditional entropy between variables X and Y, which captures the amount of information in Y that is not in X. Formally, H(Y|X) = ∑i ∑jp (xi, yj) log2p (xi, yj)/p(xi);
• I (X; Y) is the mutual information between random variables X and Y and captures the information shared between the two. Formally, I (X; Y) = H(X) + H(Y) − H (X; Y) or I (X; Y) = H(Y) − H(Y|X).
Following Krakauer et al. (2020), we define the predictability of a system as the reduction in our uncertainty about its future assuming knowledge of its current state and the environment. Thus, we can quantify this variable using mutual information:
Equation 1 does not help us disentangle the effect of the environment from the effect of the system to its future state, as these are both captured by the last term. To solve this, Krakauer et al. (2020) further decompose Eq. 1 as:
The decomposition shifts focus to the influence of the system upon itself. The first term on the right side of Eq. 2 is referred to as the autonomy of the system as it quantifies its ability to control its future state without interacting with its environment. The second is referred to as non-closure as it captures the information flow between the environment and the state.
To provide our definition of resilience, we need some additional concepts. We augment the model above by considering that the system St is characterized by a structure G: M → M, which can be conceptualized as a graph that models the components, M, of the system and how they interact with each other to deliver the function At of the system.3 Typically complex systems have a multi-layered structure: information is processed at different temporal and spatial scales (‘complex time’, see above). The function is a behavior of the system in its environment, i.e., A (S (M, G), E).
We refer to this predictability as fitness, i.e., F = I(St, Et; St+1). We define disturbance D as an information flow from the environment to the state that is not already predicted by the state. Thus, the size of a disturbance is equal to I(St+1; Et|St). We can evaluate the merit of a fitness function by measuring how much it contributes to an increase in the predictability of the system:
We now have the necessary ingredients to provide a definition of resilience.
Definition 1:. Resilience is the ability of a system to maintain its fitness (F = I(St, Et; St+1) above a desirable threshold
Note that here we attributed low values of I(St+1; St) to endogenous disturbances. However, a low value of autonomy could also be attributed to the system not correctly predicting its environment using its current state. As explained in Krakauer et al. (2020), autonomy is a detector of individuality and one needs to draw the environment-state boundary in such a way that autonomy is high enough to be able to acknowledge the presence of an individual. This brings us back to our initial argument: you can only define resilience once you have defined an individual. Thus, our definition is most informative once one is certain that the individual is correctly identified; nevertheless, it is still useful if not: extreme endogenous disturbance can be seen as lack of individuality.
Our proposed model can be framed mathematically as a set of difference equations that capture the dynamics and relationships between the different variables.
where f, g, h, i, j are non-linear functions. Such non-linear dynamical models allow us to capture the “descent-with-modification” nature of adaptive systems, because current values of state variables are conditioned not only on potential causal factors (“modification”), but also on their own past values (“descent”). For example, a system’s function (At) is affected both by its past values and by the current state. Similarly, the state of the environment (Et) may be a result of complex processes of a system’s niche construction and its internal dynamics, while the fitness of the system (Ft) may depend on its past values. Equation 5 features our assumption that only function and not structure per se influences fitness. We also see that the resilience index (Ires) does not participate in the equations of other variables: Resilience is an emergent property of the individual-environment system, which is useful for describing a system but not a mechanism driving its future state.
Our definition is purposely broad enough to account for many approaches to studying resilience, while recognizing that a universal definition will always remain elusive. Indeed, the literature has talked about robustness, resilience, adaptability, and other relevant concepts, in a way that it is infeasible for different works to agree on which mechanisms they consider. Thus, this definition serves as a boundary objective to stimulate cross-disciplinary dialogue. In some cases, that means that particular disciplines may disagree with elements of our definition, which in itself is a useful finding.
4 Types of resilience
Based on our definition 1 of resilience, a system can make use of two of its properties to ensure resilience: its structure G and function A. As we have shown in our review of the different disciplines, however, different studies of resilience may assume that change in one of these properties is not allowed for a system. Here, we introduce four different types of resilience depending on which of these two properties one is allowed to change. In the Supplementary Material, we demonstrate how these types can be mapped to studies of different disciplines. For each type we also introduce a corresponding Ires, which describes the set of criteria studies are interested in evaluating when considering this type.
We can visualize the types of resilience as logical sub-spaces of the space of solutions that a study of resilience can consider (see Venn diagram in Figure 4). In particular, we can define the following spaces:
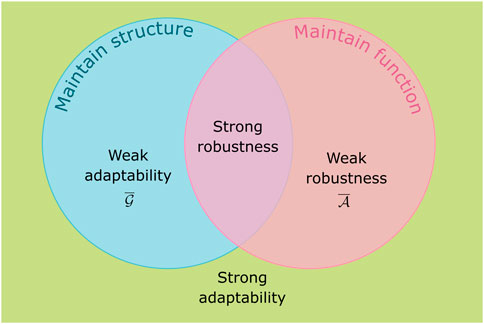
FIGURE 4. Resilience types. Note: Categorising approaches to resilience into four different sub-types based on whether changes on the structure or the function of the individual are allowed. Approaches in
4.1 Type I: Strong robustness
Definition 2:. Strong robustness.
A system is strongly robust if it can maintain its fitness above a desirable threshold
This is the strongest type of resilience where a system fully absorbs and withstands shocks, changing neither function nor structure. Examples of this type can be found in many human-engineered systems: buildings, communication systems and factories are designed with expectations about the worst-case conditions they will encounter. At times of distress, we do not expect the components of the system to reorganize or change their functionality, but we rely on existing safety measures, which absorb the disturbance as long as it persists and enable the return to its previous state. This type of resilience relies on the gradual dissipation of the shock or the existence of certain safety margins that allow the system to operate within a, potentially large, range of external parameters.
Resilience here is quantified with respect to the size of the shock, taking into account both a (temporary) reduction in the fitness of the system due to the disturbance and the duration of the shock. Formally, we denote as td the time step at which the disturbance appears, TD the time at which the disturbance ends,
Thus, the resilience index captures the temporally-extended fitness cost caused by the disturbance and is considered zero if the fitness falls below a certain threshold.
4.2 Type II: Weak robustness
Definition 3:. Weak robustness.
A system is weakly robust if it can keep its fitness above a desirable threshold
This type of resilience is still interested in the ability of the system to return to its previous function, so the fitness before and after the disturbance is the same. However, the system is allowed to change its structure G: it can delete/add dimensions and/or it can change the connectivity of existing dimensions.
To quantify resilience in this type we augment the resilience index of strong robustness defined in Eq. 8 with a measure of the structural change:
where
Some authors denominate weak robustness as robustness (Ay, 2020), for others it is the typical form of resilience (Walker, 2020), depending on how much emphasis different authors lay on the maintenance of structural integrity in comparison to preservation of fitness. This form of resilience stresses the adaptability and transformability of a system to shocks in contrast to the previous type of strong robustness which stresses immutability (Folke et al., 2010).
Weak robustness as one form of resilience implies G ≠ G′ → A = A′. This is also known as functional equivalence and arises when certain functions in a system can be performed by more than one way of structuring the elements of the system. In economics, for instance, policy makers often recur to legal constraints to protect jobs (“employment protection legislation”) by restricting individual lay-offs. Alternatively they can use economic incentives by penalising employers with high labour turnover. In the United States, for instance, this is being implemented through a mechanism called “experience rating” whereby the insurance premium an employer has to pay to the unemployment insurance system is determined on the basis of its historical layoff records. Both approaches, therefore, can strengthen a labour market’s resilience to shocks through very different policy structures.
4.3 Type III: Strong adaptability
Definition 4:. Strong adaptability.
A system is strongly adaptable if it can maintain its fitness above a desirable threshold
By allowing a change in function, this type of resilience allows the system to not only absorb a shock but also to adapt its function. Our definition here assumes that a change in fitness is only possible under a change in function but does not necessarily require a change in structure. Indeed, a change in structure alone is not sufficient to affect fitness, unless it also changes a system’s function. For completeness we also consider the following sub-type.
4.4 Type IV: Weak adaptability
Definition 5:. Weak adaptability.
A system is weakly adaptable if it can maintain its fitness above a desirable threshold
Indeed, a change in function does not necessarily require a change in structure. For example, biological organisms possess behavioral plasticity mechanisms that enable adaptation to environmental conditions without requiring genomic adaptation (Kim et al., 2020). But changes in function that come about due to a change in structure can be amongst the most interesting phenomena to study. To induce a change in function, a functional change may entail the emergence of a new organizational level in a hierarchical complex system, such as the emergence of proteins from RNA and the emergence of cells from proteins. Our definition of resilience does not change in the face of such profound transformations of a system: if the practitioner considers that this emergence is an acceptable structural change, then we can still characterize the system as resilient.
We note that our resilience type II (weak robustness) does not include approaches that allow this kind of functional change. Equivalently, we can say that a change in structure can keep the system in the same equilibrium state (weak robustness) but can also lead to a new equilibrium state (strong adaptability).
To the extent that a system can significantly increase its level of fitness following a functional change, resilience of type III is also called anti-fragility, often encountered in economics (e.g., through technical innovations) or immunology (e.g., through vaccines). Here, the disturbance that a system is subject to is instrumental in allowing it to evolve a new structure and function that provides a strictly better fitness than the one the system had before the disturbance (Taleb, 2012).
This conceptualisation of anti-fragility implies that a system’s fitness function can have multiple equilibria, which will be visited only after a perturbation. Anti-fragility has an explicit normative meaning in that only improvements in a system’s fitness are considered to be anti-fragile whereas reductions are not, making it a special case of strong adaptability. In the next section, we will discuss the implications for complete system failure and breakdown.
To measure resilience of either type III or type IV, the improvement in fitness achieved by the change needs to be added to the considerations of the previous resilience types:
where wIII ∈ [0, 1] determines the contribution of the new term. In case of type IV, in Eq. 9 the first term is replaced by IResI as no change in structure is taking place. In case of type IV, in Eq. 9 the first term is replaced by IResI as no change in structure is taking place.
Summing up, to measure resilience of a system we have defined multiple resilience indexes. Each index builds upon the previous one to integrate overlapping considerations, giving rise to a multi-dimensional measure that involves weighting schemes (wI, wII, wIII). In this regard, our four types of resilience help clarify the circumstances under which change in function, change in structure or both can be identified as resilience (Walker et al., 2004). Hence, to compute a resilience index one needs to determine the relevant type of resilience that one is interested in. As such, resilience is an inherently normative approach that involves trading-off different systemic features. S1 illustrates our different resilience types for selected disciplines (telecommunications, ecology, economics, cell biology).
5 Discussion and extension
Our four types of resilience allow for cross-disciplinary links to discussions in other fields, including integrated information theory (IIT), multi-layered networks, and criticality in systems theory.
5.1 Resilience and the integrated information theory
Integrated Information Theory (IIT) has become a lead contender to formalise consciousness (Tononi, 2004). While the conceptual framework of IIT goes beyond our definition of resilience, the mathematical formulation of integrated information ϕE as defined by Barrett and Seth (2011) provides a measure of the complexity of a minimally irreducible system, which can be linked to our resilience measures. In particular, we can demonstrate that the following relationship holds:
where we follow Engel and Malone (2018) and define:
where
Note that
5.2 Hierarchy, panarchy, and transformation
The information-theoretic definition of the individual (Krakauer et al., 2020) that inspired our resilience definition explicitly parsed information flows at different scales within a system, conferring individuality at the lowest level exhibiting persistence of information flows. Holling’s introductory paper on the resilience concept (Holling, 1973) defined persistence as the ultimate measure of resilience. It thus offers a means of identifying the type and degree of resilience of a particular system that satisfy the condition of an information-theoretic individual I(St, Et; St+1). Importantly, each system is composed of smaller partitions and can be part of larger partitions that define information processes at different scales. Socio-ecological systems theory captures the interplay among scales in the concept of panarchy, noting that elements outside the focal scale can provide memory or alter thresholds for regime change (Walker et al., 2006). More generally, the existence of multiple scales in complex systems is implicit in the notion of emergent properties, which are subject to changes in structure and function across the system.
Our approach also includes the idea of system transformation. We conceive, transformation as linked to a system’s structure whereas its function needs to be kept at least at the same fitness level as at the initial state. To the extent that the system undergoes a transformative change leading to a higher fitness level, an “anti-fragile” transformation has taken place. In contrast, if the fitness falls below its prior level, a system is not considered to be resilient. Note that such a transformation can still lead to a new system that is resilient but at a lower fitness level (such as if an ecosystem loses a large degree of its bio-diversity but continues to exist). Transformation can be driven by processes acting outside the system under consideration, including at other scales. Folke et al. (2010) give the example of the transformation of row crop agriculture in Argentina, Paraguay, and Brazil to reduced tillage practices, which was driven by experimentation and adoption by individual farmers before precipitating system-wide changes in farming practices and equipment. We can equate such transformation with regime shifts as we discuss next.
5.3 Regime shifts and resilience
Finally, our definition of resilience facilitates the understanding of system criticality to analyse cases of regime shifts. In particular, when changes in the environment occur that lower a system’s fitness below its minimum viable level
This can be illustrated in times of major societal changes and breakdowns of political regimes, such as in the case of the fall of the Roman Empire that gave place to smaller, less economically and socially powerful regional and city powers. In other cases, a cascading breakdown of the structure can lead to the total collapse of the system (see Bardi (2017) who discusses these cascading dynamics). This was presumably the case with the environmental disaster on the Easter Islands that led to their abandonment (see Diamond, 2005). A systematic overview of socio-ecological regimes is provided by Biggs et al. (2018).
These considerations of regime shifts are closely related to the interpretation of resilience as multiple equilibria and system bifurcations in the presence critical thresholds. Dynamic systems at critical thresholds can quickly shift between neighbouring equilibria, which is what would explain that these systems keep their functionality and hence are resilient. Larger equilibrium shifts (catastrophic events à la René Thom (1975) with significant changes in equilibrium behaviour), however, increase the risk of reaching parts of the state-space that are not feasible, for instance because certain dynamic systems can exist only in discrete entities (see also Prigogine and Nicolis, 1971).
6 Conclusion and outlook
The paper presents a succinct definition of four types of resilience, using an information-theoretic approach. Starting from an overview of how the concept of resilience is being used in 11 different academic disciplines, we aim at reflecting the key ingredients shared by the various resilience concepts. In particular, our definition focuses on how functional stability is being maintained under the impact of environmental fluctuations. We illustrate how functional stability can lead to different forms of resilience, depending on whether a system’s function or structure are allowed to change. Our notion of resilience encompasses concepts of robustness—no change in structure or function—as well as adaptive systems where both structure and function can change, provided a minimum level of fitness is being maintained. We also illustrate how functional improvements under the impact of environmental variation together with system-internal restructuring can lead to increases in fitness, a case of type III resilience that has been dubbed anti-fragility in the literature.
Our approach highlights that there is a continuum of forms of resilience, from the most robust form—total immutability—to a fully transformed system that displays changes in structure and function to yield improved fitness. Depending on a researcher’s object of analysis and research question, some forms will be more appropriate than others. Researchers in sustainability research, for instance, will put more emphasis on a system’s adaptability whereas engineers might be more interested in strong robustness to be able to identify safety margins. Hence, before employing our proposed resilience types to quantify resilience, the practitioner needs to identify the resilient system, putting boundaries between it and the environment, define its structure and understand how its function and fitness arise as it is interacting with the environment. Our objective here is to provide a cross-disciplinary definition of resilience but any practical resilience study would need to rely on a deep understanding of a complex system’s multi-scale nature in space and time, and the function of information flows within the system and between the system and the environment. Further, we recognize that any broad typology of resilience can never be fully universal, but that attempting a convergence in language and structure of the term ‘resilience’ can stimulate cross-disciplinary dialogue through its use as a boundary object.
Our definition also has a strong normative element to it, as it links resilience to structure, function and fitness, three concepts closely related to the informational integrity of a system. According to our definition, a system is considered to be resilient only to the extent that it can maintain or increase its fitness. However, different forms of resilience put more or less emphasis on maintenance of structure and function. Finally, our definition makes explicit reference to adaptability to the extent that shifts in the environment are being absorbed in such a way that fitness is being maintained above a minimum threshold
Finally, our definition acknowledges the existence of conditions under which a system might break down or significantly lose its resilience. This will happen when a system’s fitness falls below a certain fitness threshold that no longer allows it to keep afloat its current functionality, regardless of whether a system restructuring has happened or not.
Our approach does not claim to be an exhaustive answer to the question of what constitutes resilience. Rather, it offers an information-theoretic proposal to facilitate inter-disciplinary exchange and dialogue around a notion that has gained relevance in recent times. Moreover, it aims at disentangling different forms of resilience that can help in better understanding system performance and possibilities for intervention to improve a system’s resilience. One stream of anticipated future work will be applications of our definitions in different domains. Another stream would consider the philosophical underpinnings of various conceptualisations of resilience and their normative implications, which will influence any cross-disciplinary dialogue about such a common but contentious term.
7 Significance statement
This paper presents an inter-disciplinary review of the conceptualisation and use of the term “resilience” in eleven different disciplines and suggests a rigorous definition, taking an information-theoretic complex-systems perspective. To distinguish resilience from robustness and anti-fragility, three concepts often used interchangeably, we define resilience as the persistence of key system functions with respect to a specific hierarchical level. Robustness, on the other hand, refers to a system that persists in its entirety whereas anti-fragility to a system that reaches a different level of functionality. The paper discusses the implication of this concept, possible ways of measuring the degree of resilience as well as possible extensions to include instances of systemic failure (catastrophes) and multi-system competition.
Data availability statement
The original contributions presented in the study are included in the article/Supplementary Material, further inquiries can be directed to the corresponding author.
Author contributions
EN, supported by EE, formulated the information-theoretic definition of resilience. EE, KD, NF and NP contributed with examples on how to apply our definition to their respective fields. All authors contributed to the article and approved the submitted version.
Acknowledgments
The authors are grateful for the intellectual support received from the Santa Fe Institute, in particular during the Complex Interactive Summer School in June 2021. Discussions with SFI faculty proved extremely helpful in setting up and designing this project. Comments from two anonymous referees are gratefully acknowledged. Contributions to an earlier draft on the discussion of resilience in health care by Osondu Ogbuoji (Duke Center for Policy Impact in Global Health) are gratefully acknowledged.
Conflict of interest
KD is the founder of Peregrine Data Inc.
The remaining authors declare that the research was conducted in the absence of any commercial or financial relationships that could be construed as a potential conflict of interest.
Publisher’s note
All claims expressed in this article are solely those of the authors and do not necessarily represent those of their affiliated organizations, or those of the publisher, the editors and the reviewers. Any product that may be evaluated in this article, or claim that may be made by its manufacturer, is not guaranteed or endorsed by the publisher.
Supplementary material
The Supplementary Material for this article can be found online at: https://www.frontiersin.org/articles/10.3389/fcpxs.2023.1236406/full#supplementary-material
Footnotes
1https://www.undp.org/sustainable-development-goals
2Note that we start from the premise that we have identified a system in contrast to Krakauer et al. (2020) who aim to identify what an individual constitutes. We discuss the implications of this regarding our assumptions about multi-level hierarchy in the section on panarchy
3Although we could assume that the environment also has a structure, we do not find this necessary to formalize the resilience of the system
References
Aghion, P., and Howitt, P. (1992). A model of growth through creative destruction. Econometrica 60 (2), 323–351. doi:10.2307/2951599
Ay, N. (2020). Ingredients for robustness. Theory Biosci. 139, 309–318. doi:10.1007/s12064-020-00332-4
Bardi, U. (2017). The Seneca effect. Why growth is slow but collapse is rapid. Cham, Switzerland: Springer.
Barrett, A. B., and Seth, A. K. (2011). Practical measures of integrated information for time-series data. PLOS Comput. Biol. 7 (1), e1001052. doi:10.1371/journal.pcbi.1001052
Barrett, C. B., and Constas, M. A. (2014). Toward a theory of resilience for international development applications. Proc. Natl. Acad. Sci. 111 (40), 14625–14630. doi:10.1073/pnas.1320880111
Basche, A. D., and Edelson, O. F. (2017). Improving water resilience with more perennially based agriculture. Agroecol. Sustain. Food Syst. 41, 799–824. doi:10.1080/21683565.2017.1330795
Bednar, J. (2016). “Robust institutional design: what makes some institutions more adaptable and resilient to changes in their environment than others?,” in Complexity and evolution: A new synthesis for economic. Editors D. S. Wilson, and A. Kirman (Cambridge, MA: MIT Press), 167–184.
Bhandari, S., and Alonge, O. (2020). Measuring the resilience of health systems in low- and middle-income countries: A focus on community resilience. Health Res. Policy Syst. 18, 81. doi:10.1186/s12961-020-00594-w
Biggs, R., Peterson, G. D., and Rocha, J. C. (2018). The regime shifts database: A framework for analyzing regime shifts in social-ecological systems. Ecol. Soc. 23 (3), 9. doi:10.5751/es-10264-230309
Billman, G. E. (2020). Homeostasis: the underappreciated and far too often ignored central organizing principle of physiology. Front. Physiology 11, 200. doi:10.3389/fphys.2020.00200
Brandon-Jones, E., Squire, B., Autry, C. W., and Petersen, K. J. (2014). A contingent resource-based perspective of supply chain resilience and robustness. J. Supply Chain Manag. 50 (3), 55–73. doi:10.1111/jscm.12050
Brunnermeier, M. (2021). The resilient society. Lessons from the pandemic for recovering from the next major shock. Princeton, NJ.: Princeton University Press.
Caballero, R. J., and Hammour, M. L. (1994). The cleansing effect of recessions. Am. Econ. Rev. 84 (5), 1350–1368.
Capano, G., and Woo, J. J. (2017). Resilience and robustness in policy design: A critical appraisal. Policy Sci. 50, 399–426. doi:10.1007/s11077-016-9273-x
Chesson, P. (2000). Mechanisms of maintenance of species diversity. Annu. Rev. Ecol. Syst. 31, 343–366. doi:10.1146/annurev.ecolsys.31.1.343
Dantzer, R., Cohen, S., Russo, S. J., and Dinan, T. G. (2018). Resilience and immunity. Brain, Behav. Immun. 74, 28–42. doi:10.1016/j.bbi.2018.08.010
Diamond, J. (2005). Collapse: How societies choose to fail or succeed. New York, NY: Viking Penguin.
Eberly, J. C., Haskel, J., and Mizen, P. (2021). ’Potential capital’, working from home, and economic resilience. Cambridge, MA: National Bureau of Economic Research.
Engel, D., and Malone, T. W. (2018). Integrated information as a metric for group interaction. PLOS One 13 (10), e0205335. doi:10.1371/journal.pone.0205335
Flack, J. C., Erwin, D., Elliot, T., and Timescales, D. C. K. (2013). “Symmetry, and uncertainty reduction in the origins of hierarchy in biological systems,” in Cooperation and its evolution. Editors K. Sterelny, R. Joyce, B. Calcott, B. Fraser, and R. Joyce (Cambridge, MA: MIT Press). doi:10.7551/mitpress/9033.003.0004
Flack, J. (2017). “Life’s information hierarchy,” in From matter to life: information and causality. Editors S. I. Walker, P. C. W. Davies, and G. F. R. Ellis (Cambridge University Press), 283–302. doi:10.1017/9781316584200.012
Folke, C., Carpenter, S. R., Walker, B., Scheffer, M., Chapin, T., and Rockstrom, J. (2010). Resilience thinking: integrating resilience, adaptability and transformability. Ecol. Soc. 15 (4), 20. doi:10.5751/es-03610-150420
Holling, C. S. (1973). Resilience and stability of ecological systems. Annu. Rev. Ecol. Syst. 4, 1–23. doi:10.1146/annurev.es.04.110173.000245
Hunter, R. G., Gray, J. D., and McEwen, B. S. (2018). The neuroscience of resilience. J. Soc. Soc. Work Res. 9 (2), 305–339. doi:10.1086/697956
Hynes, W., Trump, B. D., Kirman, A., Haldane, A., and Linkov, I. (2022). Systemic resilience in economics. Nat. Phys. 18, 381–384. doi:10.1038/s41567-022-01581-4
Jain, N., Girotra, K., and Netessine, S. (2022). Recovering global supply chains from sourcing interruptions: the role of sourcing strategy. Manuf. Serv. Operations Manag. 24 (2), 846–863. doi:10.1287/msom.2021.0967
Kim, H., Muñoz, S., Osuna, P., and Gershenson, C. (2020). Antifragility predicts the robustness and evolvability of biological networks through multi-class classification with a convolutional neural network. Entropy 22 (6), 986. doi:10.3390/e22090986
Krakauer, D. C., Bertschinger, N., Olbrich, E., Flack, J. C., and Ay, N. (2020). The information theory of individuality. Theory Biosci. 139, 209–223. doi:10.1007/s12064-020-00313-7
Krakovská, H., Kuehn, C., and Longo, I. P. (2023). Resilience of dynamical systems. Eur. J. Appl. Math., 1–46. doi:10.1017/s0956792523000141
Kuhmonen, I. (2020). The resilience of Finnish farms: exploring the interplay between agency and structure. J. Rural Stud. 80, 360–371. doi:10.1016/j.jrurstud.2020.10.012
Levins, R. (1970). “Extinction,” in Some mathematical problems in biology. Editor M. Gertenhaber (Providence, RI: American Mathematical Society), 75–107.
MacArthur, R. H., and Wilson, E. O. (1967). The theory of island biogeography. Princeton, NJ: Princeton University Press.
Mcaslan, A. (2010). The concept of resilience. understanding its origins, meaning and utility. Technical report. Adelaide, Australia: Torrens Resilience Institute.
Miroudot, S. (2020). Resilience versus robustness in global value chains: Some policy implications Technical report. London: CEPR VoxEU column. Avaliable At: https://cepr.org/voxeu/columns/resilience-versus-robustness-global-valu e-chains-some-policy-implications.
Papanicolas, I., Rajan, D., Karanikolos, M., Soucat, A., and Figueras, J. (2022). Health system performance assessment. a framework for policy analysis Technical report. WHO.
Prigogine, I., and Nicolis, G. (1971). Biological order, structure and instabilities. Q. Rev. Biophysics 4 (2-3), 107–148. doi:10.1017/s0033583500000615
Roland, G. (2002). The political economy of transition. J. Econ. Perspect. 16 (1), 29–50. doi:10.1257/0895330027102
Sagan, A., Webb, E., Azzopardi-Muscat, N., de la Mata, I., McKee, M., and Figueras, J. (2021). “Health systems resilience during covid-19: lessons for building back better,” in WHO regional office for europe on behalf of the European observatory on health systems and policies (Brussels: WHO).
Smith, P., Hutchison, D., Sterbenz, J. P. G., Schöller, M., Fessi, A., Karaliopoulos, M., et al. (2011). Network resilience: A systematic approach. IEEE Commun. Mag. 49 (7), 88–97. doi:10.1109/MCOM.2011.5936160
Smith, V. H., and Goodwin, B. K. (1996). Crop insurance, moral hazard, and agricultural chemical use. Am. J. Agric. Econ. 78, 428–438. doi:10.2307/1243714
Stanton, E. A. (2007). The human development index: A history. Working paper 127. Amherst, MA: Political Economy Research Institute.
Tenthorey, J. L., Young, C., Sodeinde, A., Emerman, M., and Malik, H. S. (2020). Mutational resilience of antiviral restriction favors primate TRIM5α in host-virus evolutionary arms races. eLife 9, e59988. doi:10.7554/eLife.59988
Thom, R. (1975). Structural stability and morphogenesis. Reading, MA: W. A. Benjamin Advanced Book Program.
Thomas, S., Sagan, A., Larkin, J., Cyles, J., Figueras, J., and Karanikolos, M. (2020). “Strengthening health systems resilience. key concepts and strategies,” Technical report No. 36, WHO regional office for europe on behalf of the European observatory on health systems and policies (Brussels: WHO).
Tononi, G. (2004). An information integration theory of consciousness. BMC Neurosci. 5, (42). doi:10.1186/1471-2202-5-42
Turenne, C. P., Gautier, L., Degroote, S., Guillard, E., Chabrol, F., and Ridde, V. (2019). Conceptual analysis of health systems resilience: A scoping review. Soc. Sci. Med. 232, 168–180. doi:10.1016/j.socscimed.2019.04.020
USAID (2012). “Building resilience to recurrent crisis,” in USAID policy and program guide (Washington, DC: USAID).
Waddington, C. H. (1942). Canalization of development and the inheritance of acquired characters. Nature 150 (3811), 563–565. doi:10.1038/150563a0
Walker, B., Gunderson, L., Kinzig, A., Folke, C., Carpenter, S., and Schultz, L. (2006). A handful of heuristics and some propositions for understanding resilience in social-ecological systems. Ecol. Soc. 11 (1), 13. doi:10.5751/es-01530-110113
Walker, B., Holling, C. S., Carpenter, S. R., and Kinzig, A. (2004). Resilience, adaptability and transformability in social-ecological systems. Ecol. Soc. 9 (2), 5. doi:10.5751/es-00650-090205
Walker, B. (2020). Resilience: what it is and is not. Ecol. Soc. 25 (2), art11. doi:10.5751/es-11647-250211
Wilson, S., Pearson, L. J., Kashima, Y., Lusher, D., and Pearson, C. (2013). Separating adaptive maintenance (resilience) and transformative capacity of social-ecological systems. Ecol. Soc. 18 (1), 22. doi:10.5751/es-05100-180122
Keywords: resilience, complex-systems theory, interdisciplinary review, dynamic systems, information theory
Citation: Nisioti E, Clark C, Das KK, Ernst E, Friedenberg NA, Gates E, Lambros M, Lazurko A, Puzović N and Salas I (2023) Resilience—Towards an interdisciplinary definition using information theory. Front. Complex Syst. 1:1236406. doi: 10.3389/fcpxs.2023.1236406
Received: 07 June 2023; Accepted: 24 August 2023;
Published: 25 September 2023.
Edited by:
Marco Villani, University of Modena and Reggio Emilia, ItalyReviewed by:
Louise Comfort, University of Pittsburgh, United StatesDavid Sidney Byrne, Durham University, United Kingdom
Copyright © 2023 Nisioti, Clark, Das, Ernst, Friedenberg, Gates, Lambros, Lazurko, Puzović and Salas. This is an open-access article distributed under the terms of the Creative Commons Attribution License (CC BY). The use, distribution or reproduction in other forums is permitted, provided the original author(s) and the copyright owner(s) are credited and that the original publication in this journal is cited, in accordance with accepted academic practice. No use, distribution or reproduction is permitted which does not comply with these terms.
*Correspondence: Ekkehard Ernst, ernste@ilo.org