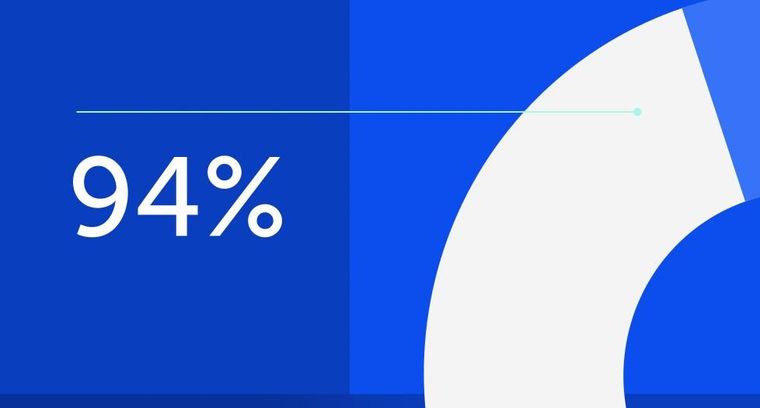
94% of researchers rate our articles as excellent or good
Learn more about the work of our research integrity team to safeguard the quality of each article we publish.
Find out more
ORIGINAL RESEARCH article
Front. Commun. Netw.
Sec. IoT and Sensor Networks
Volume 6 - 2025 | doi: 10.3389/frcmn.2025.1575120
The final, formatted version of the article will be published soon.
Select one of your emails
You have multiple emails registered with Frontiers:
Notify me on publication
Please enter your email address:
If you already have an account, please login
You don't have a Frontiers account ? You can register here
The exponential growth of connected devices on the Internet of Things (IoT) has transformed multiple domains, from industrial automation to smart environments. However, this proliferation introduces complex challenges in efficiently managing limited resources—such as bandwidth, energy, and processing capacity, especially in dynamic and heterogeneous IoT networks. Existing optimization methods often fail to adapt in real-time or scale adequately under variable conditions, exposing a critical gap in resource management strategies for dense deployments. The present study proposes a granular computing framework designed for dynamic resource optimization in IoT environments to address this. The methodology comprises three key stages: granular decomposition to divide tasks and resources into manageable grains, granular aggregation to reduce computational load through data fusion, and adaptive granular selection to refine resource allocation based on current system states. These techniques were implemented and evaluated in a controlled industrial IoT testbed comprising over 80 devices. Comparative experiments against heuristic and AI-based baselines revealed statistically significant improvements: a 25% increase in processing throughput, a 20% reduction in energy consumption, and a 60% decrease in error rate. Additionally, quality of service (QoS) reached 95%, and latency was reduced by 25%, confirming the effectiveness of the proposed model in ensuring robust and energy-efficient performance under varying operational loads.
Keywords: Granular Computing, Resource optimization in IoT, Energy efficiency in networks, Internet of the Things, networks
Received: 13 Feb 2025; Accepted: 07 Apr 2025.
Copyright: © 2025 Villegas, Govea and Gutiérrez. This is an open-access article distributed under the terms of the Creative Commons Attribution License (CC BY). The use, distribution or reproduction in other forums is permitted, provided the original author(s) or licensor are credited and that the original publication in this journal is cited, in accordance with accepted academic practice. No use, distribution or reproduction is permitted which does not comply with these terms.
* Correspondence: William Villegas, University of the Americas, Quito, Ecuador
Disclaimer: All claims expressed in this article are solely those of the authors and do not necessarily represent those of their affiliated organizations, or those of the publisher, the editors and the reviewers. Any product that may be evaluated in this article or claim that may be made by its manufacturer is not guaranteed or endorsed by the publisher.
Supplementary Material
Research integrity at Frontiers
Learn more about the work of our research integrity team to safeguard the quality of each article we publish.