- 1Computer Electrical, and Mathematical Science and Engineering (CEMSE) Division, King Abdullah University of Science and Technology (KAUST), Thuwal, Makkah Province, Saudi Arabia
- 2School of Electrical, Electronic and Mechanical Engineering, University of Bristol, Bristol, United Kingdom
- 3School of Electrical Engineering, Chongqing University, Chongqing, China
The continual progress pace of the development of the Wi-Fi generations may not fulfill the expected tremendous data growth of future smart-home network devices and applications. Throughout this article, we show a comprehensive design of the futuristic smart-home network that distributes massive data for indoor devices while fully supporting intelligent functions. We start by drawing a vision for various application scenarios of information and communication technologies in smart-home networks beyond the 2030s. Then, we appraise the technical specifications of the candidate intelligent home network. To achieve these technological speculations, we propose a novel holistic networking solution that is efficient and intelligent from service and operation perspectives. Specifically, we detail the proposed network architecture, elements, and enabling technologies. To accelerate the research progress and implementation, we further point out the future research directions. Finally, we discuss the non-technological factors that could affect the future deployment of the proposed network solution.
1 Introduction
A smart home has been one of the essential people-centric Internet of Things (IoT) systems that attracted a tremendous amount of attention in academia and industry (Feng et al., 2017). The smart home reaps the progress in information and communications technologies (ICT) to adapt living environments and conditions to drastically improve residential quality, services, comfort, and safety. Moreover, motivated by new residential requirements during the COVID-19 pandemic, the smart home should support several remote daily activities, such as education, healthcare, work, and entertainment. To this end, the capability, reliability, and security of data distribution are of paramount importance and need to be accomplished by efficient smart home network solutions (Xu et al., 2016).
Nowadays, the Wi-Fi systems supported by IEEE 802.11 standards provide widely applied solutions for home networks with growing coverage and data rate. Undoubtedly, Wi-Fi has significantly accelerated the informatization progress and has profoundly impacted human society in the past two decades (Pahlavan and Krishnamurthy, 2020). Today, the latest system, Wi-Fi 6E, offers a tri-band solution at frequencies 2.4 GHz, 5 GHz, and 6 GHz with bandwidths of 60 MHz, 500 MHz, and 1,200 MHz, respectively, c.f. Figure 1. The Wi-Fi systems adopt several powerful communication techniques, such as multiple-input and multiple-output (MIMO), orthogonal frequency-division multiplexing (OFDM), and higher modulation orders, to boost the throughput and coverage performance (Hardani and Hayat, 2022). Despite the commendable services Wi-Fi achieved in the past few years, it is questionable if the capabilities of Wi-Fi systems can accommodate the ambitions of future smart home networks (He et al., 2020; Bandyopadhyay et al., 2023). Further improvement on throughput without going beyond the current Wi-Fi bands would be marginal owing to hitting the theoretical performance wall of the Shannon-Hartley theorem (Dohler et al., 2011).
Numerous research efforts have been dedicated to developing smart-home networks, which aim to connect various types of automated devices to the core network. This intelligent network can learn and adapt its performance to accomplish required tasks with minimal human involvement (Brush et al., 2018; Yan et al., 2022). The field of smart-home research can be broadly categorized into three areas: 1) network deployment and configuration, 2) monitoring and adaptation, and 3) developing multi-service networks (Brush et al., 2018; Ye et al., 2018; Namboodiri et al., 2013; Collotta and Pau, 2015; Ishikawa, 2013; Pinheiro et al., 2020; Yu et al., 2019). In the realm of network deployment and configuration, innovative approaches have been proposed to support intelligent services, such as a service-aware wireless heterogeneous network. This network utilizes a mega service-aware network controller to achieve service adaptation, network cognition, and resource provisioning (Ye et al., 2018). A significant amount of research has also been dedicated to intelligent energy metering and management, with a particular focus on privacy and security constraints (Namboodiri et al., 2013; Collotta and Pau, 2015). These studies explore the control and management of home appliances over heterogeneous networks, offering solutions to optimize energy consumption. User privacy in smart-home networks has received considerable attention. For instance, Pinheiro et al. (2020) proposed a packet padding mechanism that uses supervised learning mechanisms to monitor the network and apply appropriate padding, ensuring user privacy. Furthermore, deep reinforcement learning has been explored as a method for managing energy consumption in smart homes. A notable example is the work of Yu et al. (2019), who proposed an approach for task offloading to efficiently manage energy consumption.
Smart home networks are a critical component of the broader smart cities paradigm, which integrates a massive number of Internet of Things (IoT) devices, machines, and users, facilitating the control and optimization of a multitude of tasks with minimal human intervention. As explored in the comprehensive work by Song et al., smart cities encompass concepts such as smart governance, economy, mobility, environment, people, and living (Song et al., 2017). These principles are enabled by advancements in IoT technologies, which have been widely reviewed in recent literature (Zanella et al., 2014; Al-Fuqaha et al., 2015; Mehmood et al., 2017; Qian et al., 2019; Syed et al., 2021; Talebkhah et al., 2021). IoT applications extend across various areas such as transportation, healthcare, and public services, and play a crucial role in realizing the futuristic visions of smart cities. For instance, in the context of smart homes, IoT technologies enable advanced monitoring, automation, and enhance the interaction between homes and residents. By incorporating such works on overarching smart city principles and IoT applications, we provide a more complete view of the state of the art and motivate continued research in smart homes. However, it is important to note the ongoing challenges posed by the need to support the immense number of anticipated future smart devices (Naik et al., 2021). As urban landscapes continue to evolve towards smart cities, we recognize the crucial role smart homes play in this transformation (El-Azab, 2021).
Future smart-home networks are expected to support data-hungry and low-latency applications, such as mixed reality (MR), three-dimensional (3D) holography, and tactile internet. The current and coming Wi-Fi systems are neither suitable for such applications nor simultaneous services, such as accurate localization, sensing, and tracking. It is worthy to emphasize that one of the smart-home goals is to construct smart cyberspace allowing various location-based services (Ahmad et al., 2019). Therefore, the integration of data communications and a set of sensing functions with a much higher level of autonomy becomes a necessity (Huang et al., 2020; Bahadori et al., 2022). Although researchers attempt to achieve this goal by proposing the concept of radio frequency (RF) cloud by the Wi-Fi technology (Pahlavan and Krishnamurthy, 2020), the received signal strength (RSS) and time of arrival (TOA) of Wi-Fi signals give insufficient information to construct the futuristic cyberspace. Furthermore, it is difficult and even not possible to eliminate electromagnetic interference by using Wi-Fi in dense networks. Also, due to the openness and interoperability brought by Wi-Fi standards, smart home networks’ digital security and privacy are under threat of cyberattacks, which are challenging to be managed by current Wi-Fi technology (Sivaraman et al., 2018).
Given the unique challenges associated with Wi-Fi and the specific needs of smart home networks, it is reasonable to question the effectiveness of Wi-Fi solutions in supporting future smart home networks. To identify a network solution that is well-suited to the requirements of a smart home, we conducted an extensive review of the literature and identified unique features of smart home networks. These features align with three key research directions:
1) Network deployment and configuration
2) Monitoring and adaptation
3) Developing multi-service networks
To realize the above-mentioned features, we propose a novel smart home network solution that relies on the following key enablers:
In the following sections, we delve deeper into the interplay between the crucial technologies that form the backbone of our proposed smart home network solution: Beyond Wi-Fi Bands, Smart Communication Surfaces, Federated Learning, and DAG-based blockchain. These technologies are not stand-alone elements but tightly interwoven to enhance the network’s deployment, multi-service development, monitoring, adaptation, security, and privacy. We aim to provide a comprehensive understanding of their relationships and collective contributions to the proposed smart home network.
The remainder of the article is organized as follows. We first present the typical application scenarios of smart-home networks in the 2030s and the corresponding technical specifications. Then, we propose the network specifications, including the architecture, key enablers, and system setups, that can achieve the expected requirements. Based on the proposed solution, we discuss the future research directions and analyze the non-technological issues that might hinder the implementation of the proposed solution.
2 Application scenarios of smart home
The future smart-home network requires many sensors with new combined technologies to provide innovative applications that improve household residents’ life quality and working efficiency (Rault et al., 2014; Abo-Zahhad et al., 2014). This section envisions five representative application scenarios of the futuristic smart home that can be achieved and extended in the next decade. Figure 2 shows a pictorial illustration of these five application scenarios.
2.1 Intelligent indoor environment control
Indoor environmental intelligent monitoring and control are necessary for smart-home networks to ensure safe and comfortable living conditions (Kolokotsa et al., 2010). Smart-home networks should monitor all household apparatus and infrastructures to supervise maintenance tasks automatically and avoid housing risks. For example, tens of thousands of people were hospitalized due to carbon monoxide poisoning at housing units per year (Organization WHO, 2011). The primary cause is the misuse of ovens for warming without alerting when the carbon monoxide concentration reaches a threshold level. Alternatively, these hospitalized cases can be averted by the capability of intelligent indoor environment control installed in smart-home units. In addition to safety, creating cozy and environmentally friendly households is also important and can be facilitated by various intelligent indoor environment control systems such as air humidity, temperature, illumination, and gas composition systems.
2.2 Surveillance and monitoring of residents safety
Monitoring personal safety is always a crucial issue at present and in the future and therefore should be given the highest priority. Observing the individual health status and alerting related personnel in time to any occurrence or risk of illness and accident are essential for smart-home residents, which is also known as e-health (Farahani et al., 2020). Therefore, smart-home networks should be equipped with many sensors and actuators to enable powerful surveillance and monitoring functions, ensuring the highest residential safety level. Precisely, the internal body health will be monitored by sensors to provide a complete medical file for each resident with hospitals or online medical centers. Such shared information can enable emergency preparation by doctors or urgent self-treatments suggested by artificial intelligence. Also, a remote physical check-up and minor treatments can be performed using a tactile internet systems (Fanibhare et al., 2021). Such a physical interaction is suitable for isolated areas, lock-down scenarios, and emergency scenarios.
2.3 Mixed reality
The concept of mixed reality (MR) encompasses the well-known virtual reality (VR) and augmented reality (AR) concepts. MR devices are believed to be the next-generation “killer app” in the next decades after the smartphone revolution (Speicher et al., 2019; Tepper et al., 2017). The futuristic three-dimensional virtual networks, or metaverse, will introduce a virtual life platform using MR technologies and combine physical and virtual spaces (Lee et al., 2021). At the very beginning, when the concept of MR is proposed, the first imagination coming to one’s mind is gaming. Unlike computer games, an MR game does not require a clumsy screen or bulky host machines. The vision of MR games can directly be projected onto the retina by putting an MR appliance as small as a smartphone on a player’s head. In this way, the players can access a human-machine interface (HMI) by the motions of hands or eyeballs without relying on other peripheral devices. Furthermore, MR appliances can also serve as user-friendly HMIs with various sensors for administrating the entire smart home and performing many jobs that rely on three-dimensional imaging, e.g., teaching, painting, and medical practicing. Besides, MR appliances can be essential and helpful for disabled residents to practice their lives normally. Therefore, MR applications will undoubtedly become crucial parts of the upcoming smart-home network.
2.4 Accurate indoor positioning and object tracking
Everyone might have obnoxious experiences to lose something tiny and inconspicuous at home, e.g., keys, controllers, and wallets, and spend much effort to find them. As a result of these obnoxious experiences, it would be attractive to enable the smart-home network to perform accurate indoor positioning and object tracking. Furthermore, such an ability of smart home is far more than a tool for finding lost items but can provide location reference information to help with the precise navigation of a variety of household robots working at future smart home (Piekarski et al., 2003; Mulloni et al., 2012). Besides, the wireless charging service at the smart-home network relying on precise beamforming techniques can also benefit from this attractive ability.
2.5 Work from smart home
Working from home is an inevitable trend with the developments of wireless communications and cloud computing (Randall, 2003; Kasmi et al., 2016). This trend has been recently accelerated after the COVID-19 pandemic, which prevented people from going to their workplaces. Therefore, work from a smart home in the future should also be expected and planned. To this end, we need to design a home working platform that shall support secure, reliable and vast-throughput connectivity for business data exchange and holographic display. The “work from smart home” conception will help maintain a better work-and-life balance for employees and improve their wellbeing. The concept of working shall be extended to teaching and learning where other technologies such as MR and tactile internet can participate.
All the above-envisioned application scenarios of smart homes involve emerging advanced devices that require special operating conditions. In the following section, we define the necessary technical specifications of the futuristic smart-home network to fulfill the required services and applications.
3 Specifications of smart home networks
The beyond Wi-Fi network solution should provide superior performance improvement to the Wi-Fi generations to support the required application as shown in Figure 1. Precisely, we expect extending the transmission bands of the beyond Wi-Fi network to capture the mmW, THz, and optical bands, as illustrated in Table 1. Thus, the transmission bandwidth can increase, hitting the multi GHz allowing for ultra latency performance and breaking the Tbps limit. We expect to see a significant improvement in the future technical specifications from five dimensions compared with the present specifications of Wi-Fi generations. Specifically, smart home networks will be able to support hundreds of connected nodes rather than only several connected devices in recent smart homes, bring in several-fold improvement in throughput and latency, as well as energy and efficiency, provide almost 100% secure network and keeping residents’ privacy and personal information from leakage, and equipped with more powerful predictability. In the following, we give detailed discussions about these five technical specifications.
3.1 Number of connected nodes
The continual progress in wireless communication technology connects the world more than ever. In 2008, connected devices exceeded the human population (6.8 billion) and continued to grow with an expectation to reach 41.6 billion by 2025 (Forum, 2020). With the maturity of the IoT system, it is envisioned that the heterogeneity becomes increasingly imperative over time, which results in the number of the connected smart home devices continuing to proliferate and surpassing the current one several-fold in the following decades (Cisco, 2020). There will be massive types of home applications, including but not limited to smart media devices, e.g., smart TVs, game consoles, novel network domestic appliances, such as smart light, smart window shutter, and intelligent washing machines, as well as intelligent surveillance cameras. Moreover, to realize the application scenarios mentioned in the last section, heterogeneous wireless sensors are going to be distributed in a smart home to collect data from the environment, residents, and machines. Therefore, the capability of connecting various things in hundreds or even thousands of magnitudes simultaneously with almost surely 100% connectivity is one of the basic specifications for future smart-home networks.
3.2 Throughput and latency
The expected running applications of the tremendous different connected devices will inevitably compete for the limited spectral resources, yielding a low transmission throughput without proper countermeasures. Therefore, there can be a spectrum contention issue when hundredfold or thousandfold devices request different rates in a limited legitimate bandwidth (Gupta et al., 2022). Consequently, the co-channel interference of spectrum sharing can degrade the information transmission quality (Ye et al., 2021), which is more critical for multimedia applications such as high-definition television streaming, MR, and real-time holographic display. Thus, the future home network should have an enhanced throughput of the current one (Oughton et al., 2021). The throughput also impacts another key network parameter signifying the system performance, termed as latency (Abderrahim et al., 2020). Latency determines if users can respond promptly within a certain time threshold when interacting with smart devices through the network (Showail and Shihada, 2018). Some of the existing literature already work on this field and assure that the transmission latency of sensors should be no more than 10 ms (Anwar et al., 2015; Shah et al., 2020; Ariyanti and Suryanegara, 2020). However, the multimedia applications mentioned above and tactile internet systems require less than a 1 ms latency service. Undoubtedly, physical-layer and network-layer technologies for high throughput and low latency are the premises for constructing smart-home networks.
3.3 Energy efficiency
Future smart homes with various appliances and sensors will be far more complicated energy systems and consume more energy than current homes without intelligent functionality. Therefore, energy efficiency is an essential issue that needs to be carefully managed and enhanced in smart-home networks (Zobel et al., 2021). Such a consideration is necessary when the number of connected nodes surges in the future (Turchet and Rinaldo, 2021; Wang et al., 2019). Over the past few years, there has been a growing demand to develop well-designed energy-saving strategies enabled by intelligent technologies, such as eliminating the energy drain caused by idling appliances (target wake time (TWT) in current Wi-Fi systems). Moreover, IoT applications are developed to harvest energy from a different source, such as sunlight, indoor optical and radio frequency (RF) signals (Sudevalayam and Kulkarni, 2010; Abdelhady et al., 2020). The smart-home environment is full of electromagnetic waves, which provide abundant energy to be reused as harvested and stored in batteries for IoT applications (Eltresy et al., 2020). It is expected that the energy-constrained nodes with limited battery capacities, e.g., wearable devices, enjoy a much longer lifetime of the replacement of the batteries in their structure, especially when they are difficult or even impossible to be charged. The more energy-efficient network can translate to more extended device battery life. On the other hand, more energy-efficient smart-home applications have a stronger attraction to people who want to enjoy the same user experience with lower energy costs to afford (AlQerm and Shihada, 2019). Overall, energy efficiency is an essential performance metric for future smart-home networks (Amin et al., 2015), and enhancing energy efficiency directly contributes to reducing the carbon footprint of smart home networks, aligning with global sustainability goals.
3.4 Security and privacy
In addition to enjoying the functionalities brought by the smart-home network, residents also expect their online experience at home to be always secure and their personal information and assets to be safe (Boutaba et al., 2003). The leading trends such as e-commerce, mobile payments, IoT, machine learning, and social media greatly ease users’ life, but also lead to a higher incidence of cyberattacks (Cisco, 2020). For instance, an eavesdropping adversary can sniff the wireless channel, infer the device execution states from wireless traffic, steal the users’ privacy by performing over-privileged execution, and even perform spoofing attacks on smart-home networks. The resource-constrained devices lack sufficient processing and computation capacity to engage in security operations. The centralized structure collecting massive data from users’ devices to provide personalized and customized services can worsen even the security. The information leakage when people work from home might cause catastrophic consequences to their companies, especially for those who work for banks and health centers. Therefore, the smart home should ensure that cyberattacks and information eavesdropping are financially unviable for the cybercriminals with almost 100% probability. Otherwise, without adequate security and privacy mechanisms, the development of the smart home will turn out to be futile.
3.5 Predictability
The ability to predict inhabitants’ requirements in advance and make proactive decisions on network resource allocation is a key for smart-home networks (Zhang et al., 2022c). From the users’ perspective, the success of prediction is significant for people who need living assistance, such as children, the elderly, and the disabled. Low prediction accuracy with many inopportune services could annoy users, lower their interest and trust in intelligent functionality, and eventually make them unsatisfied with smart-home services. From the network’s point of view, predicting the upcoming traffic and the transmission delay for different applications accurately can help allocate the limited spectrum resource more efficiently and provide a more fluent application experience for users (AlGhadhban and Shihada, 2018; Zhang et al., 2021). In addition, the smart home with predictable activity can also dynamically force some applications to sleep when they are not be utilized, which can save power throughout the system and leave more network capacity available for other time-critical traffic (Bouacida et al., 2017). Moreover, the battery life of different sized battery capacities for each device can be predicted by understanding its energy consumption, which assists residents in replacing the battery or recharging it at an appropriate time. We conclude that predictability will play an essential role in future smart homes, whose accuracy depends on the reliability and timeliness of data drawn from the actual living environment, the intelligent services provided, and user behaviors.
4 Proposed smart home network solution
This section proposes and details our smart home network solution in response to the evolving needs of smart home networks and the limitations of existing Wi-Fi systems. In particular, we expound on the distributed network architecture and elements, including management and orchestration aspects, network monitoring and adaptation strategies, and security and reliability assurance mechanisms. Our solution leverages advanced technologies including beyond Wi-Fi bands, smart communication surfaces, federated learning, and DAG-based blockchain. This section further details the implementation of these technologies and how they collectively contribute to the robustness, efficiency, and adaptability of our smart home network solution. Finally, we detail the versatile multi-service platform enabled by our proposed solution, demonstrating its ability to support a wide array of smart home functionalities.
4.1 Implementation of key technologies
We now turn our attention to how our solution’s essential technologies - Beyond Wi-Fi Bands, Smart Communication Surfaces, Federated Learning, and DAG-based Blockchain - are brought to life within our network infrastructure.
4.1.1 Beyond Wi-Fi bands
In our proposed solution, we extend the traditional Wi-Fi frequency bands to include mmW, THz, and optical bands for the purpose of network deployment and service provision. The use of these expanded frequency ranges significantly increases communication bandwidth and allows for effective spectral resource optimization.
4.1.2 Smart communication surfaces
In our network, we integrate smart communication surfaces - such as metasurfaces, programmable mirror arrays, and smart optical devices (such as optical shutters) - to effectively interact with the expanded frequency bands. These surfaces intelligently control the propagation of electromagnetic waves, thereby enhancing network coverage and data rate.
4.1.3 Federated learning
Our solution incorporates federated learning to improve network monitoring and adaptation. This machine learning approach allows edge devices in our network to collaboratively learn a shared prediction model, all while keeping the training data on the original device - a process that significantly enhances network intelligence and ensures data privacy.
4.1.4 DAG-based blockchain
We integrate DAG-based blockchain technology to bolster the security of our network. This decentralized technique provides an effective anti-tamper mechanism via a fast and green consensus process. It works in tandem with Federated Learning to maintain user privacy and ensures the secure operation of our smart home network.
The interplay between these technologies is vital to our proposed solution. Smart communication surfaces interact with the expanded frequency bands to enhance network efficiency, while federated learning and DAG-based Blockchain work together to ensure data privacy and network security. Collectively, these technologies form the foundation of our proposed smart home network solution, significantly enhancing network deployment, multi-service development, monitoring, adaptation, security, and privacy. It is important to note that our proposed solution not only addresses technological challenges but also contributes to resource management and carbon footprint reduction. The energy-saving strategies outlined in this section, including hybrid frequency band utilization, smart communication surfaces, and federated learning, collectively work towards creating a more sustainable and environmentally friendly smart home network infrastructure.
4.2 Network architecture and components
The proposed home network solution consists of a hardware basis constructed by several components and devices in the lower layer, three intermediate panels in the medium layer, and finally a user interface (UI) as depicted in Figure 3. Firstly, the hardware basis provides a platform for network components, sensors, and actuators to exchange processed data for fulfilling several required services efficiently. To this end, the network components enable essential communication services by sharing and exchanging operational data among several devices in the network. These components mainly encompass various transceivers relying on intelligent communication surfaces and multiple frequency bands. We classify the adopted frequency bands as low-frequency (LF) and high-frequency (HF) bands to support different services and applications. The LF band is an extended version of the classical Wi-Fi bands where the adopted frequency is less than the mmW band. On the other hand, the HF band captures the sub-THz (mmW), THz, and optical bands.
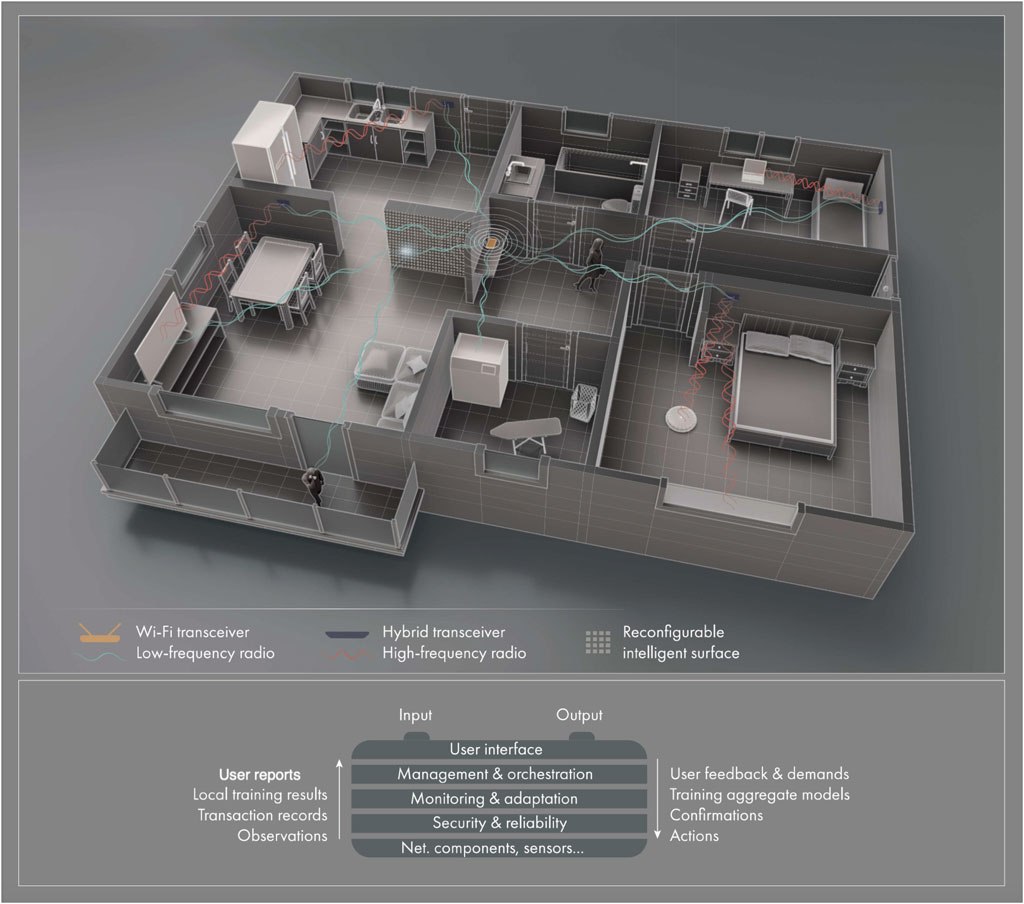
Figure 3. Holistic framework of the proposed smart home network solution with all panels, key components, and enabling technologies (Illustration created by Ivan Gromicho. Scientific Illustrator at Research Communication and Publication Services. Office of the Vice President for Research - King Abdullah University of Science and Technology).
Thanks to the broad coverage of the LF bands, the proposed intelligent network uses them to provide reliable transmission channels for control, coordinating, and confirmation signals. Such LF channels convey a small amount of information that are the pivots to ensure the secure and efficient operation of the entire home network. Unlike the LF bands, the HF bands are used to transmit data for both communications and sensing purposes due to their much higher bandwidths and the accompanying transmission capability. Technologies like massive MIMO beamforming, 3D beamforming, and spatial multiplexing are employed to further enhance the throughput into the Tbps range and beyond, increasing both the bandwidth and spatial streams (Shlezinger et al., 2021; Yang et al., 2020). Meanwhile, it is worth noting that the HF band’s short transmission range makes it not feasible for hybrid transceivers to obtain a complete picture of the indoor electromagnetic environment and deal with mobility-related issues. Therefore, the successful use of HF bands is highly dependent on the real-time and accurate coordination enabled by LF radios. In such a way, we adopt both RF bands in a hybrid and complementary manner to construct immersive and intelligent cyberspace. In addition, the LF bands can occasionally take the responsibility of raw data transmission as alternatives in exceptional circumstances when the HF bands cannot be accessed or are suffering from interference.
The LF access point (AP) utilizes frequency bands lower than 30 GHz, including the current WiFi bands 2.4 GHz, 5 GHz, and 6 GHz, which have good indoor penetration abilities allowing large covering areas (Muhammad et al., 2021). However, there might be some dead zones that the LF AP cannot cover due to the required coverage area or the complexity of the building structure (Lyu et al., 2019). To realize seamless coverage, we employ reconfigurable intelligent surfaces (RISs) to direct LF radio signals and focus them on those dead spots to ensure the reception of the control signaling by the network components and devices distributed over those areas (Chen et al., 2022; Ye et al., 2022; Boulogeorgos and Alexiou, 2021; Du et al., 2022; Zeng et al., 2020; Hedhly et al., 2023). Besides, employing RISs can form a geo-fence that only allows intended transceivers to access legal signals while preventing jamming radio from interfering with the legal signals and preventing the indoor radios from leaking out. Therefore, RISs can participate effectively in mitigating side-channel and jamming attacks, which further enhance the security and privacy of the home network from the physical-layer perspective. In addition to aiding in coverage and security, the RISs also facilitate network slicing, allowing us to dedicate network resources to latency-sensitive applications like AR/VR and autonomous vehicles, ensuring these services receive the required bandwidth and latency.
On the other hand, the HF APs are extensively distributed throughout the home, ensuring seamless coverage despite the short transmission range of the HF bands. With the dense deployment of these APs, each with inter-AP distances of just a few meters, we can mitigate the limited coverage range and ensure there are no dead zones within the network. Also, the network can support very high data rates thanks to the extremely available high bandwidth channels. Although the mmW and THz bands signals can provide longer ranges than the optical band and offer higher bandwidth than the optical devices, visible light communications (VLC) can provide an efficient solution (Abdelhady et al., 2019). The spatial distribution of illumination sources along the home guarantees good network connectivity and encourages the exploitation of optical energy harvesting (Abdelhady et al., 2020), improving energy efficiency and decreasing network deployment costs. Other HF-based energy-efficient intelligent surfaces such as optical shutters can be installed in the windows and walls to support passive optical modulation, which offer another HF communication solution (Abdelhady et al., 2022). For example, liquid crystal shutters can allow indoor sunlight illumination during daytime while modulating the passed sunlight conveying data along nearby indoor regions (Bloom et al., 2019; Ammar et al., 2022). Interestingly, the required energy needed by the LC shutters can be harvested by the sunlight; thus, a green, efficient communication link is established (Ammar et al., 2023).
Secondly, the intermediate panels collect the detected observation and data by various sensors and receivers through network components. Specifically, the security and reliability panel (SRP) is the responsible panel that connects directly to the hardware basis because of the paramount importance of security and reliability in smart-home networks. At the same time, the actuators receive action orders only from the SRP to adapt to the physical and electromagnetic environments of the smart home. The SRP manages a DAG-based blockchain and generates transaction records corresponding to the observations and actions. The SRP also receives feedback for confirmation from the monitoring and adaption panel (MAP). The MAP is responsible for monitoring the holistic status of the entire smart-home network via the transaction records, producing specific adaptation schemes by federated learning, and sending confirmations back to help the management of the DAG-based blockchain. It also dynamically allocates the use of HF and LF bands based on the current network conditions, user demands, and application requirements. After the MAP, the management and orchestration panel (MOP) connects as the higher-layer panel, directly reporting finally to the UI. The MOP functionality is more complex and abstract than the SRP and the MAP. In brief, the MOP manages various network resources and harmonizes all components and devices connected to the smart-home network.
The MAP is responsible for monitoring the holistic status of the entire smart-home network via the transaction records, producing specific adaptation schemes by federated learning, and sending confirmations back to help the management of the DAG-based blockchain. Unlike traditional single-chain blockchain technologies that rely on Proof of Work (PoW) or Proof of Stake (PoS) mechanisms, a DAG-based blockchain offers a unique approach facilitating concurrent transaction validation and consensus among distributed agents in a network. Consequently, this concurrency results in improved network scalability, faster request responses, and reduced energy consumption. When combined with federated learning, this integration enables a decentralized network architecture, enhancing security and privacy when network and user data is processed at the MAP.
The network architecture we propose, accompanied by the three panels, is engineered to facilitate the unique characteristics and application scenarios discussed in Sections 1, 2. Specifically, the network’s support for hybrid RF bands ensures reliable and efficient connectivity for machine-type communications across various signal types at high speeds. One key component in this architecture is the MOP, which plays a pivotal role in resource management and scheduling, ensuring optimal network performance. Moreover, leveraging advanced techniques like RIS and DAG-based blockchain enhances the security of these communications, providing a robust, efficient, and secure network environment.
It should be noted that the three panels mentioned above are simply the collective abstractions of the corresponding functions distributed over network components and devices rather than concrete panels in specific machinery. Therefore, these panel abstractions do not compromise the distributed nature of the solution in practice but ease the functional analysis, optimization, and upgrade for smart-home networks. In the following subsections, we will give the details of these three panels and the UI design.
4.3 Co-existence of multiple technologies and quality of service
The co-existence of multiple technologies in a common area, as illustrated in Figure 3, introduces unique management complexities. These technologies, hybrid transceivers, reconfigurable intelligent surfaces, LF radios, and HF radios, have varying communication requirements, necessitating careful frequency allocation.
LF bands, while providing long-range and reliable communications, are more susceptible to noise and interference than HF radio bands. Therefore, the adopted frequency should be carefully chosen for different transceivers with various communication targets. Designing a frequency allocation scheme to avoid scenarios where some frequency bands are busy while others are idle or unused is essential.
Despite the wide range of frequencies considered, it can still be overwhelmed as the number of devices increases (Pullmann and Macko, 2019). Therefore, careful frequency allocation is required to minimize the impacts of co-channel interference. The deployed reconfigurable intelligent surfaces can reduce the burden on interference management and frequency allocation. However, this introduces more complex system optimization problems requiring high-complexity computations.
Moreover, the performance gains introduced by reconfigurable intelligent surfaces heavily depend on the estimated channel state information, which is challenging to obtain accurately in passive-mode operation. Additionally, the applications of reconfigurable intelligent surfaces require additional control links, consuming limited channel resources.
The dynamically allocated frequency and reconfigurable intelligent surfaces optimization also increase the computational capabilities requirements, presenting additional challenges. Overall, the co-existence of multiple technologies in a common area results in complicated management and design problems, consuming more channel and computational resources.
However, smart homes constructed using these technologies are expected to enjoy much higher performance as given in Table 1. Therefore, the focus should not solely be on the advantages or disadvantages when these technologies work cooperatively. Instead, more attention should be paid to handle the trade-off problems to enjoy the highest performance gains with the lowest cost.
4.4 Security and reliability
Communications and networking research communities have widely agreed that well-designed distributed architectures with an appropriate consensus process are generally more secure and reliable than centralized architectures (Dang et al., 2020). Indeed, centralized servers, controllers, and databases are vulnerable; once hacked, all components and devices connected to the compromised network become threatened. Hence, while keeping the distributed design principle in mind, we resort to blockchain technologies to ensure the security and reliability of the proposed smart-home network are adequate (Fu et al., 2022).
The specific choice of a DAG-based blockchain for our smart home network stems from its unique advantages in addressing the demands of this environment. Unlike traditional linear blockchains, DAG-based architectures offer superior scalability, handling high transaction throughput from numerous interconnected devices without compromising network performance. Their parallel processing capabilities and forking topology enable efficient transaction confirmation, ensuring low latency crucial for real-time applications like security systems and appliance control.
While other blockchain technologies exist, their characteristics are less suitable for the unique demands of a smart home network. Traditional linear blockchains, with their sequential transaction processing, struggle to handle the high volume of transactions expected in a future smart home. Proof-of-Work based blockchains, while secure, are energy-intensive, making them less desirable for an environment where energy efficiency is paramount. In contrast, DAG-based blockchains consume significantly less energy, aligning with the need for sustainable smart home solutions. Proof-of-Stake alternatives, while more energy-efficient than Proof-of-Work, often centralize control within a smaller group of stakeholders, potentially compromising the desired decentralized security of our proposed network.
Given these advantages, we propose to employ a DAG-based blockchain to form an anti-tamper mechanism to enhance security and reliability further. By allowing a forking topology and concurrent processing of transactions, the DAG-based blockchain can deal with high-rate transaction flow and significantly reduce energy consumption for computation (Wang et al., 2020). In future Wi-Fi networks, where numerous devices may be transacting data simultaneously, this technology improves overall network efficiency and considerably enhances network scalability. More importantly, a decentralized network structure enabled by a DAG-based blockchain helps to improve network resilience and reduce vulnerability to single points of failure or attack. These unique features make the DAG-based blockchain an ideal candidate to decentralize the architecture of smart-home networks and build a trustworthy consensus against double-spending and spamming by a minority of nodes connected to the smart-home network.
Also, we can use a context-aware data-driven solution to decrease irrelevant and useless data traffic (Aslani et al., 2020). In addition, the plenty of HF communication bands with second-layer chain solutions allow partitioning the data traffic through different communication media and provide better scalability and enhanced resistance against security issues (Aslani et al., 2020). Throughout the DAG-based blockchain process, the connected components and devices generate a unique transaction record of each function/task, i.e., signal transmission, reception, and processing task. Then, they sign the transaction record with its private key and broadcast it to all other components and devices connected to the smart-home network. The uniqueness and unforgeability of the generated transaction record are guaranteed by its hash value. By adopting the Markov chain Monte Carlo (MCMC) algorithm for reaching consensuses, the ledger of a DAG-based blockchain becomes anti-tamper, especially when the number of connected components and devices is significant.
Our solution’s distributed architecture and edge computing enable localized processing power, optimizing routing and traffic flows based on real-time network insights, thus reducing latency. Artificial intelligence (AI) and machine learning technologies are integral in optimizing our network solution. They contribute to achieving high data rates and low latency through real-time traffic management, predictive maintenance, and intelligent resource allocation. Lastly, our solution utilizes federated learning for collaborative model training while preserving data privacy, leading to more efficient network operation and potentially increased data rates.
4.5 Monitoring and adaptation
The MAP performs several monitoring and adaptation functions on the transaction records received from the SRP. It extracts and interprets valuable information from these records, produces and sends local training model parameters to the MOP. In return, the MAP also feeds confirmations back to the SRP to confirm the transaction records and thereby helps the management of the DAG-based blockchain. In nature, the MAP serves as an agent to prevent access to sensitive data contained in transaction records.
Following the distributed architecture of the DAG-based blockchain, federated learning can be designed and implemented to play a crucial role in the MAP for producing local training model parameters. It is stipulated in our solution and shown in Figure 3 that the hybrid transceivers installed on the ceiling are capable of transmitting, receiving, and processing both LF and HF signals. As a fixed AP, hybrid transceivers are supposed to have a reliable power supply and be a facility with sufficient processing capability. Hence, the raw data from user equipment or sensors will be transmitted to the hybrid transceivers over HF bands. However, all raw data will be processed locally and distributed at the hybrid transceivers rather than sending them to a remote server for collective processing. Then, the processed outcomes, typically intermediate and local training model parameters used to build a global training model and much smaller in size than the raw data, will be sent to the Wi-Fi transceiver through LF bands and then uploaded to a powerful server for aggregation. In this way, all raw data can be kept locally and removed, after some time, to be inaccessible to third parties. With the help of the distributed architecture and federated learning, the MAP simultaneously achievable a high level of autonomy and complete privacy protection in the proposed smart home network.
4.6 Management and orchestration
The management and orchestration functions primarily involve the design and adjustments of resource allocation policies, which include hardware, energy, spectra, timeslots, space, and network element synergy schemes. The MOP panel, playing a key role in these processes, is responsible for performing these higher-layer functions that cater to the global environmental profile and user demands. Effective resource management and scheduling are crucial for ensuring high performance and efficient utilization of the available resources in a smart home network.
Two primary aspects should be considered in the resource management and scheduling process: 1) optimizing the allocation of resources to meet the diverse requirements of the smart home applications, and 2) minimizing the energy consumption and latency to ensure the sustainability and responsiveness of the network. To achieve these goals, we propose the deployment of advanced and adaptive scheduling algorithms that can dynamically allocate resources based on the current network conditions, user demands, and application requirements. These algorithms can be based on machine learning techniques that can learn from historical data and predict the future resource requirements for different applications. In this context, the MOP panel plays a crucial role in scheduling the use of different RF bands as part of its resource management responsibilities. The wide-coverage LF signal is utilized to assign the dense HF sources to different users, ensuring smooth data connections. Meanwhile, RIS installed on walls and ceilings redirect signals to extend their propagation range and fill any remaining coverage holes, thus allowing for reliable omni-directional coverage and seamless connectivity within the smart home network. The MOP panel could employ either a centralized or distributed approach for resource management and scheduling, depending on the specific network architecture and application requirements.
Apart from these functional designs and adjustments, the MOP also needs to summarize and send user-friendly reports and indicators to the UI that hide tedious technical details but show sufficient and explicit information for users’ attention and decision. The MOP aggregates local training model parameters by obtaining the local training results from the MAP and the user feedback and demands. It also rectifies them according to the user’s input and demands to produce global training models to cope with the heterogeneity of involved elements in the network. These global training models are broadcasted and shared among all active components, which govern the operation of the smart home network. Explicitly, these global training models are expected to determine.
Furthermore, the resource management and scheduling process should also incorporate fault tolerance and recovery mechanisms to ensure the reliability and robustness of the smart home network. These mechanisms can include the detection of faulty components or devices, reallocation of resources, and rescheduling of tasks to maintain the seamless operation of the smart home network.
4.7 User interface and anti-misoperation system
UIs form the bridge between human users and the smart home, facilitating interactions via input and output modules such as keyboards and display screens. Our UIs will be software-defined, integrated into mobile devices like smartphones or tablets as an app, or embedded in MR devices. The software-defined UIs include a range of interfaces like the graphical user interface, command-line interface, menu-driven user interface, and touch user interface. Further, our smart home UI will utilize AI algorithms and wireless sensors to incorporate natural language user and human movement detection interfaces. These modern UIs can facilitate technology usage by individuals who may not be tech-savvy, such as children, the elderly, or people with mobility impairments. A significant innovation in our proposed solution is an AI-based detection system, which will leverage machine learning techniques to detect and identify IoT devices in the network (Liu et al., 2021). The system will extract device-specific features from network traffic traces and wireless signals, using various learning algorithms to classify devices based on their radiometric signatures. In addition, our system will employ unsupervised learning and abnormality detection methods to discover unknown or compromised devices in the network. This approach provides an efficient solution to secure existing systems and offers additional protection to systems with cryptographic protocols. Our vision of the smart home UI is one that moves toward “No-UI,” where numerous intelligent sensors and the AI-based detection system monitor and detect the residents’ states. Consequently, the smart-home network will automatically adjust its configurations to suit the demands extracted from users’ movements, gestures, and expressions. Designers or residents of the smart home can opt for traditional or modern UIs, depending on their specific requirements.
Despite the convenience of interactions between humans and the smart home provided by the UI, an embedded anti-misoperation system is critical to act as a gateway between users’ inputs and the operation of the entire system. This anti-misoperation system, integrated with the AI-based detection system, can reduce abnormal and unsafe operations by children and novices, as well as thwart malicious processes. A typical anti-misoperation system should check the legality of demands received from residents through a UI and then parse them into commands interpretable by the MOP. The AI-based detection system will play a crucial role in strengthening the performance of the anti-misoperation system. By improving the detection of abnormal or unsafe operations, it will enhance the overall security and safety. Furthermore, machine learning can help recognize and learn from residents’ patterns and behaviors, allowing the network to provide personalized, context-aware services. The system will also enhance the user interface and anti-misoperation system of our smart home network solution by providing real-time feedback and alerts to the users about the status and behaviour of their IoT devices. The control data exchange between the anti-misoperation system and the MOP is relatively small, and hence, LF signals should be sufficient to support this exchange.
4.8 Multi-service platform
Our proposed smart home network solution provides a rich multi-service platform that goes beyond basic data transmission to meet the diverse needs of future smart homes. This platform enhances convenience, comfort, safety, sustainability and efficiency through services such as:
5 Non-technological issues
In this section, we talk about the issues beyond ICT and utilize the political, economic, social, technological, legal, and environmental (PESTEL) business model to analyze the potential non-technological merits and obstacles for implementing our proposed smart home network solution (Yüksel, 2012; Perera, 2017). The PESTEL strategic study is imperative to provide the ICT market decision-makers with a comprehensive picture of the future of the proposed technology (Zhang et al., 2022b; Zhang C. et al., 2022).
Political factors include political influences, commercial agreements, policies, laws, and regulations, affecting innovative home solutions differently. Strong and stable internal and external politics can support the stability and developments in different sectors, including ICT. For example, internal instabilities such as corruption and non-transparency can limit access to the networks reducing the need to use smart-home network technologies. On the other hand, the recent immigration towards electronic governments encourages adopting innovative ICT solutions such as our proposed one. Also, the current COVID-19 pandemic encourages people to perform their activities (working, education, shopping, medical testing, etc.) remotely. Thus, such a trend can boost the development of the proposed technology and encourage its deployment. Another severe political factor is the taxes, especially its increasing rate with the expected growth of the exchanged smart home data rate.
Economic factors include market size, labor costs, inflation, business cycle, interest, and growth rates. Investors are interested in evaluating the equity of various markets before making investment decisions. The market in different countries is classified based on the economic development, size, liquidity requirements, and market accessibility into developed, emerging, and frontier markets. Moreover, it is crucial to know the share of the ICT sector of the country in the gross domestic product (GDP) growth rate and the unemployment rate. Then, based on the previously mentioned criterion, the investors can decide on their investment contribution in the proposed smart-home network solutions. As we saw from the current remote working experience in the pandemic, several software platforms witness a considerable increase in their market value, such as Zoom. Thus, we expect a massive rise in smart-home economic factors starting from the development stage. It is worthy to highlight that the current global economic value of Wi-Fi is 995 billion USD, which is expected to grow to 1.58 trillion USD by 2025 (Kutz et al., 2021).
Social factors capture cultural norms, education, demography, population growth, and income distribution. Young population societies are more technology-oriented and thus can positively affect our adopted smart home networking solutions. However, poor education, skill mismatch, poverty, and income inequality are among the leading social factors that can impede the deployment of smart technologies. Thus, it is necessary to reduce the “neither employed nor educated” rate in countries and improve education and technology usage among people.
Technological factors include research and development efforts, innovation, emerging technologies, and technology transfer. Fortunately, all governments are encouraging the development and investment in the ICT sector in general, facilitating our smart home networking solutions. However, not all countries are managing and protecting intellectual property rights. Under such cases, these countries can suffer from the tax-loss problem that threatens developing innovative solutions. Also, the lack of reliable technology infrastructure can prevent deploying smart solutions and reaping all its benefits. Therefore, developing a strong infrastructure and improving the research commercialization to integrate innovative smart home networking solutions is necessary.
Legal factors include regulations, laws, court systems, and standards. The hierarchy of authorities connected to communication regulations, national law, and court systems significantly define the associated legislative framework. Although meeting the regulation and rules can be difficult in some countries, especially while launching new innovative services, other challenges come from the legislative misalignment between different countries, affecting technology deployment and investment. Therefore, it is necessary to encourage developing laws to protect ICT investors considering the alignment with various authorities.
Environmental factors include climate change, energy availability, and resource management. The increased ICT energy demand encouraged the research interest to develop friendly-environment solutions. The proposed smart-home networking solutions introduce practical ways to save energy and meet futuristic operational requirements with less wasting resources and less environmental harming than existing solutions. Our proposed smart-home networking solution contributes to resource management and carbon footprint reduction through its energy-efficient design and operation. By incorporating energy harvesting, adaptive resource allocation, and other power-saving technologies, the solution aims to minimize environmental impact while meeting the growing demands of smart home networks.
6 Research directions
This section unveils some prospective research territories for the future smart-home networks based on our beyond Wi-Fi proposal.
6.1 Trade-offs
The employment of a multitude of services in smart networks requires adaptive utilization of the same shared resources in terms of power, spectrum, time, and space for different operation objectives including both technological and non-technological metrics (Chen et al., 2011). The former includes several traditional and non-traditional design metrics such as achievable throughput, localization accuracy, charging time, latency, energy efficiency, and lighting uniformity; to name but a few. However, the latter should include factors or metrics related to the PESTEL business model; such as, capital expenditures, operational expenditures, frequency regulations, pricing, and carbon footprint. Hence, there are inevitable trade-offs for each indoor smart network scenario between different quality-of-services metrics that quantify the proposed system performance from different aspects. Consequently, the network design research efforts need to alleviate the associated trade-offs’ severity at reasonable implementation cost and operational complexity.
6.2 Network planning
Adopting multiple technologies with a wide frequency band access including both RF and optical bands adds nontraditional challenges on the beyond Wi-Fi network planning. The challenges includes both intelligent integration and automation of several network assets using ML and AI-based techniques adaptively configure the network connectivity based on a variable communication demand and available resources along with communication nodes capabilities. Hence, several network planning tasks need to be done on the fly in an intelligent way. For example, accurate models for the coverage prediction and traffic capacity should be created based on local network access information with regular updates during operation (Ak and Canberk, 2021). Moreover, the automated network radio planning should be able to include models for new services and forecast the traffic and coverage prior to actual implementation. The network optimization design should capture both technological and non-technological design metrics according to the user objectives, predefined regulations and operational conditions.
6.3 Inter-smart-home networking
One of the significant challenges and potential research directions in the realm of smart home networks is creating cooperative systems between different units and services to achieve both local and global performance objectives. This requires the development of synergistic strategies that can optimize the individual performance metrics of each home while also meeting the larger goals of the entire network. In this context, transfer learning is a promising approach that permits the application of learned knowledge from one domain to another related domain, which can be particularly beneficial as we consider the numerous degrees of freedom in futuristic smart-home networks (Niu et al., 2020). Efficient algorithms could exploit spatial and temporal localities to reduce computational costs of parameter optimization through the transfer of learned knowledge from one smart home network to another. Moreover, smart homes can be perceived as building blocks of indoor environment for the larger smart cities. Therefore, it is necessary to develop strategies that can achieve global performance objectives at various scales, from individual streets and districts to the entire city. Such global goals could encompass:
1. Efficient caching of digital content based on traffic demands, where knowledge from one smart home about popular content could be transferred to other homes to optimize their caching strategies.
2. Support for surrounding networks through coverage extension, where one smart home network’s understanding of coverage gaps could help inform the configuration of nearby networks.
3. Efficient usage of public network resources, such as electricity, water, and natural gas. Here, one network’s learning about optimal resource usage could be transferred to other networks to enhance overall efficiency.
The use of transfer learning in this context could enable smart home networks to learn from each other, creating a more efficient and effective overall system. Future research in this area might explore the best ways to apply transfer learning strategies in the context of inter-smart-home networking to achieve these objectives.
6.4 Optimizing co-existence of multiple technologies
The co-existence of multiple technologies in a common area presents an array of complex challenges, as we have discussed in the “Co-existence of Multiple Technologies and Quality of Service” subsection. Although we have proposed some solutions, these challenges open up opportunities for further exploration. One significant area for future research lies in the development of advanced interference management techniques. With multiple technologies operating concurrently within the same environment, interference becomes a pronounced issue. Techniques that go beyond traditional methods and employ artificial intelligence or machine learning algorithms for dynamic prediction and mitigation of interference could be an exciting frontier to explore.
Another crucial research direction is the design of smart resource allocation algorithms. As the number of devices and co-existing technologies increases, the demand for limited resources such as frequency bands and computational power escalates. Developing intelligent resource allocation algorithms that can handle increased demand more efficiently is essential. Leveraging predictive analytics to anticipate demand and proactively allocate resources could be a valuable approach.
As new communication and networking technologies emerge, their integration into existing network infrastructure presents another worthwhile research avenue. Studying how to incorporate these technologies effectively, with minimal disruption to existing services and maximum enhancement of network performance and quality of service, will be important. Moreover, in a network environment that includes multiple technologies, traditional quality of service metrics may not fully capture the dynamics. Thus, future studies could aim to develop new metrics to address the unique challenges and characteristics of such environments. This would assist network operators in better managing and optimizing their networks.
Finally, considering the security implications of multi-technology environments is vital. The integration of multiple technologies in a common area could potentially introduce new security vulnerabilities. Therefore, future research could focus on identifying these potential threats and developing robust security measures to counteract them.
6.5 Enhanced security by mechanisms DAG-Based blockchain
Future research in enhancing the security mechanisms provided by DAG-based blockchains for future Wi-Fi networks holds significant promise. One avenue of exploration could focus on developing novel consensus algorithms tailored to accommodate specific characteristics of Wi-Fi networks and user demands. In addition, investigating methods to integrate advanced encryption techniques within the DAG-based blockchain framework could significantly enhance data privacy and integrity in Wi-Fi networks. The integration of encryption techniques into the transaction validation process could ensure that even local model data remains encrypted while being processed at the MAP, making future Wi-Fi networks more resilient to various security threats and wireless attacks. These efforts have the potential to elevate security measures to a new height, ensuring that the benefits of the DAG-based blockchain technology are harnessed while safeguarding the data integrity, user privacy, and system reliability of Wi-Fi networks.
6.6 Backhauling
The foreseen excessive data traffic generated by futuristic smart homes raises concerns about the handling capability of the current backhauling techniques. The efficient connectivity of smart homes with the external world leaves the floor open for future research efforts. Towards this aim, optimal deployment and operation of terrestrial free-space optical communications to carry smart homes data traffic represent a good start point. Nonetheless, the continuous development and increasing interest in low-earth orbit satellite communications, high-altitude platforms, and drones increase their feasibility. Also, it encourages developing a hybrid vertical backhauling structure that complements the terrestrial horizontal fabric of cellular and public switching networks.
On a different scale, providing multiple options for indoor backhauling can improve reliability and enhance security. For example, considering indoor optical fiber, ethernet, and power line communication can create robust systems similar to the suggested architecture in the industrial internet of things systems (Aslani et al., 2020).
6.7 Robotics support
Futuristic smart-home networks umbrella should include the expected newly robotic services soon. Robotic participation in smart homes is expected to be reinforced quantitatively and qualitatively. Smart-home robotics are envisioned to provide healthcare, simple maintenance and manufacturing, and logistic services. To this end, the joint optimization of the localization and communication service provided to the robotic terminals under ultra-reliable low-latency constraints is indispensable to avoid potential materials damages, or even worse, personnel injuries. In addition, mobile robotic entities in intelligent homes can serve as secondary hotspots or relays for the primary smart-home network. Consequently, the operation optimization of robotics to achieve smart-home network communications objectives in terms of coverage and throughput enhancement and the goals of their primary services’ objectives create a rich ensemble of joint communications and control research problems.
6.8 Extreme radiated power profiling
The exploding data traffic demand, growth of the number of nodes within indoor environments, and the conflicting need to reduce systems energy consumption impose challenging requirements on the spatial distribution of radiated power. Hence, confining the radiated power towards the intended receivers represents a promising research area for futuristic wireless indoor networks. To achieve this aim, realizing tunable multi-pencil-shaped lobes radiation patterns at the transmitter side and amorphous power-focusing smart surfaces should receive more attention from academia and industry sides.
6.9 Sustainability and carbon footprint reduction
Future research should focus on quantifying and further reducing the carbon footprint of smart home networks. This could involve developing more accurate models for energy consumption in complex, multi-technology networks, exploring novel energy harvesting techniques, and investigating the long-term environmental impact of widespread smart home adoption. Additionally, research into lifecycle analysis of smart home devices and infrastructure could provide valuable insights for creating more sustainable network solutions.
6.10 Computer vision-aided smart home networks
Prospective futuristic home technologies encompass imaging and sensing features for security, object tracking, and environmental monitoring purposes. A cyber model for the smart-home residents and objects can be built in these setups while accounting for the dynamic behavior. Such cognitive capabilities need to be exploited to optimize the performance of intelligent homes on many frontiers, including energy expenditure, localization accuracy, communications channels estimation and modeling, and multi-agent cooperative robotic tasks. To this end, the design process of the imaging systems, the generated models, and the way they are used to optimize the smart-home network operation forms an unexplored territory to be explored by future research efforts.
6.11 AI-native Wi-Fi era
The advent of AI-driven WLANs (Coronado et al., 2021) has marked the beginning of the AI-native Wi-Fi era. In this era, Wi-Fi networks are designed and optimized using AI models that are trained and executed locally on APs. This approach facilitates fast, secure wireless networking with local intelligence, empowering APs to make real-time decisions that enhance network performance and security. In this new era, Wi-Fi networks are becoming increasingly self-learning and adaptive, continuously self-optimizing in response to changing network conditions. A key enabler of this evolution is federated learning, which allows multiple distributed Wi-Fi APs to jointly train a common model. Not only does this approach enhance predictive performance, but it also preserves user data privacy. This transition from centralized control to distributed, AI-driven decision-making at the network edge represents a significant shift in Wi-Fi network management and optimization. It opens an array of research directions, including the development of new AI models and algorithms to optimize Wi-Fi network performance and security. Additionally, the incorporation of contextual information into AI models can improve network performance and the user experience. Moreover, new applications for AI-native Wi-Fi, such as indoor positioning or IoT device management, are being explored. As we continue to advance in the AI-native Wi-Fi era, we can anticipate the emergence of even more research directions. These will pave the way for faster, more secure wireless networking with local intelligence, pushing the boundaries of what Wi-Fi networks can achieve.
7 Conclusion
This paper presents a comprehensive and forward-looking vision for smart-home networks that goes beyond current Wi-Fi capabilities. Our proposed solution addresses the anticipated challenges of future smart homes, including increased connectivity demands, enhanced security requirements, and the critical need for improved energy efficiency. By integrating advanced technologies and considering both technological and non-technological factors, we have laid the groundwork for the next-generation of smart-home networks, which we anticipate will be realized and commercialized in the beyond of 2030s. Key innovations in our solution include the hybrid utilization of Wi-Fi and beyond Wi-Fi frequency bands, the integration of intelligent communication surfaces, and the implementation of DAG-based blockchain and federated learning technologies. These advancements enable unprecedented data rates, enhance network security and privacy, and support a wide range of smart home services beyond basic data transmission. Our approach to energy management is multifaceted, incorporating energy harvesting techniques, efficient resource allocation strategies, and context-aware data-driven solutions. The hybrid frequency band utilization allows for more efficient energy use by allocating bandwidth according to specific needs. Smart communication surfaces help tailor network coverage, potentially reducing energy needed for signal transmission. The DAG-based blockchain and federated learning reduce energy consumption for computation and data transmission respectively. These strategies collectively address the increased power demands of high-bandwidth, multi-technology networks. By outlining future research directions and considering implementation through the PESTEL framework, we provide a roadmap for realizing this vision. In conclusion, this work represents a significant step towards conceptualizing and designing energy-efficient, secure, and high-performance smart home networks of the future. As we move towards the 2030s, the ideas presented here have the potential to shape the evolution of smart home technology, ultimately leading to more connected, efficient, and user-centric living environments.
Data availability statement
The original contributions presented in the study are included in the article/supplementary material, further inquiries can be directed to the corresponding author.
Author contributions
OA: Writing–original draft, Writing–review and editing. SD: Writing–original draft, Writing–review and editing. AA: Writing–original draft, Writing–review and editing. GM: Writing–original draft, Writing–review and editing. JY: Writing–original draft, Writing–review and editing. M-SA: Writing–original draft, Writing–review and editing. BS: Writing–original draft, Writing–review and editing.
Funding
The author(s) declare that financial support was received for the research, authorship, and/or publication of this article. This work is funded by KAUST.
Acknowledgments
Figure 3 was created by Ivan Gromicho. Scientific Illustrator at Research Communication and Publication Services, office of the Vice President for Research - King Abdullah University of Science and Technology.
Conflict of interest
The authors declare that the research was conducted in the absence of any commercial or financial relationships that could be construed as a potential conflict of interest.
Publisher’s note
All claims expressed in this article are solely those of the authors and do not necessarily represent those of their affiliated organizations, or those of the publisher, the editors and the reviewers. Any product that may be evaluated in this article, or claim that may be made by its manufacturer, is not guaranteed or endorsed by the publisher.
References
Abdelhady, A. M., Amin, O., Alouini, M.-S., and Shihada, B. (2022). Revolutionizing optical wireless communications via smart optics. IEEE Open J. Commun. Soc. 3, 654–669. doi:10.1109/ojcoms.2022.3164790
Abdelhady, A. M., Amin, O., Chaaban, A., Shihada, B., and Alouini, M.-S. (2019). Spectral-efficiency—illumination pareto front for energy harvesting enabled vlc systems. IEEE Trans. Commun. 67, 8557–8572. doi:10.1109/tcomm.2019.2944913
Abdelhady, A. M., Amin, O., Shihada, B., and Alouini, M.-S. (2020). Spectral efficiency and energy harvesting in multi-cell slipt systems. IEEE Trans. Wirel. Commun. 19, 3304–3318. doi:10.1109/twc.2020.2972314
Abderrahim, W., Amin, O., Alouini, M.-S., and Shihada, B. (2020). Latency-aware offloading in integrated satellite terrestrial networks. IEEE Open J. Commun. Soc. 1, 490–500. doi:10.1109/ojcoms.2020.2988787
Abo-Zahhad, M., Amin, O., Farrag, M., and Ali, A. (2014). A survey on protocols, platforms and simulation tools for wireless sensor networks. Int. J. Energy Inf. Commun. 5, 17–34. doi:10.14257/ijeic.2014.5.6.02
Ahmad, E., Alaslani, M., Dogar, F. R., and Shihada, B. (2019). Location-aware, context-driven qos for iot applications. IEEE Syst. J. 14, 232–243. doi:10.1109/jsyst.2019.2893913
Ak, E., and Canberk, B. (2021). Forecasting quality of service for next-generation data-driven wifi6 campus networks. IEEE Trans. Netw. Serv. Manag. 18, 4744–4755. doi:10.1109/tnsm.2021.3108766
Al-Fuqaha, A., Guizani, M., Mohammadi, M., Aledhari, M., and Ayyash, M. (2015). Internet of things: a survey on enabling technologies, protocols, and applications. IEEE Commun. Surv. and tutorials 17, 2347–2376. doi:10.1109/comst.2015.2444095
AlGhadhban, A., and Shihada, B. (2018). “Delay analysis of new-flow setup time in software defined networks,” in NOMS 2018-2018 IEEE/IFIP Network Operations and Management Symposium (IEEE), 1–7.
AlQerm, I., and Shihada, B. (2019). “Enhanced online q-learning scheme for energy efficient power allocation in cognitive radio networks,” in 2019 IEEE Wireless Communications and Networking Conference (WCNC) (IEEE), 1–6.
Amin, O., Bedeer, E., Ahmed, M. H., and Dobre, O. A. (2015). Energy efficiency–spectral efficiency tradeoff: a multiobjective optimization approach. IEEE Trans. Veh. Technol. 65, 1975–1981. doi:10.1109/tvt.2015.2425934
Ammar, S., Amin, O., Alouini, M.-S., and Shihada, B. (2022). Design and analysis of lcd-based modulator for passive sunlight communications. IEEE Photonics J. 14, 1–17. doi:10.1109/jphot.2022.3200833
Ammar, S., Amin, O., Alouini, M.-S., and Shihada, B. (2023). Sun-fi: architecting glass for sunlight data transmission. IEEE Commun. Mag. 61, 175–180. doi:10.1109/mcom.002.2200603
Anwar, M., Xia, Y., and Zhan, Y. (2015). TDMA-based IEEE 802.15. 4 for low-latency deterministic control applications. IEEE Trans. Industrial Inf. 12, 338–347. doi:10.1109/tii.2015.2508719
Ariyanti, S., and Suryanegara, M. (2020). “Visible light communication (vlc) for 6g technology: the potency and research challenges,” in 2020 Fourth world conference on smart trends in systems, security and sustainability (WorldS4) (IEEE), 490–493.
Aslani, M., Amin, O., Nawab, F., and Shihada, B. (2020). Rethinking blockchain integration with the industrial internet of things. IEEE Internet Things Mag. 3, 70–75. doi:10.1109/iotm.0001.1900079
Bahadori, N., Ashdown, J., and Restuccia, F. (2022). “Rewis: reliable wi-fi sensing through few-shot multi-antenna multi-receiver csi learning,” in 2022 IEEE 23rd International Symposium on a World of Wireless, Mobile and Multimedia Networks (WoWMoM) (IEEE), 50–59.
Bandyopadhyay, D., De, S., Roy, S. H., Biswas, D., Bhose, M., and Karmakar, R. (2023). “Network throughput improvement in wi-fi 6 over wi-fi 5: a comparative performance analysis,” in 2023 International Conference on Computer, Electrical and Communication Engineering (ICCECE) (IEEE), 1–6.
Bazzi, A., and Chafii, M. (2023). On outage-based beamforming design for dual-functional radar-communication 6g systems. IEEE Trans. Wirel. Commun. 22, 5598–5612. doi:10.1109/twc.2023.3235617
Bazzi, A., and Chafii, M. (2024). Secure full duplex integrated sensing and communications. IEEE Trans. Inf. Forensics Secur. 19, 2082–2097. doi:10.1109/tifs.2023.3346696
Bloom, R., Zamalloa, M. Z., and Pai, C. (2019). Luxlink: creating a wireless link from ambient light. Proc. 17th Conf. Embed. Networked Sens. Syst., 166–178. doi:10.1145/3356250.3360021
Bouacida, N., Alghadhban, A., Alalmaei, S., Mohammed, H., and Shihada, B. (2017). “Failure mitigation in software defined networking employing load type prediction,” in 2017 IEEE International Conference on Communications (ICC) (IEEE), 1–7.
Boulogeorgos, A.-A. A., and Alexiou, A. (2021). Coverage analysis of reconfigurable intelligent surface assisted thz wireless systems. IEEE Open J. Veh. Technol. 2, 94–110. doi:10.1109/ojvt.2021.3051209
Boutaba, R., Ishibashi, B., and Shihada, B. (2003). A network management viewpoint on security in e-services. Certif. Secur. E-Services E-Government E-Business, 17–45. doi:10.1007/978-0-387-35696-9_2
Brush, A., Hazas, M., and Albrecht, J. (2018). Smart homes: undeniable reality or always just around the corner? IEEE Pervasive Comput. 17, 82–86. doi:10.1109/mprv.2018.011591065
Cao, B., Li, Y., Zhang, L., Zhang, L., Mumtaz, S., Zhou, Z., et al. (2019). When internet of things meets blockchain: challenges in distributed consensus. IEEE Netw. 33, 133–139. doi:10.1109/mnet.2019.1900002
Chen, A., Chen, Y., and Wang, Z. (2022). Reconfigurable intelligent surface deployment for blind zone improvement in mmwave wireless networks. IEEE Commun. Lett. 26, 1423–1427. doi:10.1109/lcomm.2022.3161656
Chen, Y., Zhang, S., Xu, S., and Li, G. Y. (2011). Fundamental trade-offs on green wireless networks. IEEE Commun. Mag. 49, 30–37. doi:10.1109/mcom.2011.5783982
Collotta, M., and Pau, G. (2015). A novel energy management approach for smart homes using bluetooth low energy. IEEE J. Sel. areas Commun. 33, 2988–2996. doi:10.1109/jsac.2015.2481203
Coronado, E., Bayhan, S., Thomas, A., and Riggio, R. (2021). Ai-empowered software-defined WLANs. IEEE Commun. Mag. 59, 54–60. doi:10.1109/mcom.001.2000895
Dang, S., Amin, O., Shihada, B., and Alouini, M.-S. (2020). What should 6G be? Nat. Electron. 3, 20–29. doi:10.1038/s41928-019-0355-6
Dohler, M., Heath, R. W., Lozano, A., Papadias, C. B., and Valenzuela, R. A. (2011). Is the PHY layer dead? IEEE Commun. Mag. 49, 159–165. doi:10.1109/MCOM.2011.5741160
Du, H., Zhang, J., Guan, K., Niyato, D., Jiao, H., Wang, Z., et al. (2022). Performance and optimization of reconfigurable intelligent surface aided thz communications. IEEE Trans. Commun. 70, 3575–3593. doi:10.1109/tcomm.2022.3162645
Elayan, H., Amin, O., Shihada, B., Shubair, R. M., and Alouini, M.-S. (2019). Terahertz band: the last piece of rf spectrum puzzle for communication systems. IEEE Open J. Commun. Soc. 1, 1–32. doi:10.1109/ojcoms.2019.2953633
El-Azab, R. (2021). Smart homes: potentials and challenges. Clean. Energy 5, 302–315. doi:10.1093/ce/zkab010
Eltresy, N. A., Dardeer, O. M., Al-Habal, A., Elhariri, E., Abotaleb, A. M., Elsheakh, D. N., et al. (2020). Smart home IoT system by using RF energy harvesting. J. Sensors 2020, 1–14. doi:10.1155/2020/8828479
Fanibhare, V., Sarkar, N. I., and Al-Anbuky, A. (2021). A survey of the tactile internet: design issues and challenges, applications, and future directions. Electronics 10, 2171. doi:10.3390/electronics10172171
Farahani, B., Barzegari, M., Aliee, F. S., and Shaik, K. A. (2020). Towards collaborative intelligent IoT ehealth: from device to fog, and cloud. Microprocess. Microsystems 72, 102938. doi:10.1016/j.micpro.2019.102938
Feng, S., Setoodeh, P., and Haykin, S. (2017). Smart home: cognitive interactive people-centric internet of things. IEEE Commun. Mag. 55, 34–39. doi:10.1109/MCOM.2017.1600682CM
Fu, Y., Li, C., Yu, F. R., Luan, T. H., Zhao, P., and Liu, S. (2022). A survey of blockchain and intelligent networking for the metaverse. IEEE Internet Things J. 10, 3587–3610. doi:10.1109/jiot.2022.3222521
Gupta, S., Mehdizadeh, E., Cheema, K., and Shealy, J. (2022). “Miniaturized ultrawide bandwidth wifi 6e diplexer implementation using xbaw rf filter technology,” in 2022 IEEE/MTT-S International Microwave Symposium-IMS 2022 (IEEE), 880–882.
Hardani, D. N. K., and Hayat, L. (2022). Data communication performance of multi-node wifi and lora by testing range and packet delivery. AIP Conf. Proc. 2578, 040004. doi:10.1063/5.0106352
He, Y., Chen, Y., Hu, Y., and Zeng, B. (2020). Wifi vision: sensing, recognition, and detection with commodity mimo-ofdm wifi. IEEE Internet Things J. 7, 8296–8317. doi:10.1109/jiot.2020.2989426
Hedhly, W., Amin, O., Alouini, M.-S., and Shihada, B. (2023). Intelligent reflecting surfaces assisted hyperloop wireless communication network. IEEE Trans. Mob. Comput. 23, 4943–4955. doi:10.1109/tmc.2023.3299792
Hu, J., Bandyopadhyay, S., Liu, Y.-h., and Shao, L.-Y. (2021). A review on metasurface: from principle to smart metadevices. Front. Phys. 8, 502. doi:10.3389/fphy.2020.586087
Huang, Q., Chen, H., and Zhang, Q. (2020). Joint design of sensing and communication systems for smart homes. IEEE Netw. 34, 191–197. doi:10.1109/MNET.011.2000107
Ishikawa, N. (2013). PUCC activities on overlay networking protocols and metadata for controlling and managing home networks and appliances. Proc. IEEE 101, 2355–2366. doi:10.1109/jproc.2013.2274095
Jahid, A., Alsharif, M. H., and Hall, T. J. (2022). A contemporary survey on free space optical communication: potentials, technical challenges, recent advances and research direction. J. Netw. Comput. Appl. 200, 103311. doi:10.1016/j.jnca.2021.103311
Javed, S., Elzanaty, A., Amin, O., Shihada, B., and Alouini, M.-S. (2021). When probabilistic shaping realizes improper signaling for hardware distortion mitigation. IEEE Trans. Commun. 69, 5028–5042. doi:10.1109/tcomm.2021.3074978
Kasmi, M., Bahloul, F., and Tkitek, H. (2016). “Smart home based on internet of things and cloud computing,” in 2016 7th International Conference on Sciences of Electronics, Technologies of Information and Telecommunications (SETIT) (IEEE), 82–86.
Kolokotsa, D., Saridakis, G., Dalamagkidis, K., Dolianitis, S., and Kaliakatsos, I. (2010). Development of an intelligent indoor environment and energy management system for greenhouses. Energy Convers. Manag. 51, 155–168. doi:10.1016/j.enconman.2009.09.007
Kutz, R., Jung, J., and Callorado, F. (2021). The economic value of wi-fi: a global view (2021 – 2025). Telecom Advisory Services LLC.
Lamontagne, B., Fong, N. R., Song, I.-H., Ma, P., Barrios, P. J., and Poitras, D. (2019). Review of microshutters for switchable glass. J. Nanolithogr. MEMS, MOEMS 18, 040901. doi:10.1117/1.jmm.18.4.040901
Lee, L.-H., Braud, T., Zhou, P., Wang, L., Xu, D., Lin, Z., et al. (2021). All one needs to know about metaverse: a complete survey on technological singularity, virtual ecosystem, and research agenda. arXiv Prepr. arXiv:2110.05352. doi:10.13140/RG.2.2.11200.05124/8
Li, T., Sahu, A. K., Talwalkar, A., and Smith, V. (2020). Federated learning: challenges, methods, and future directions. IEEE Signal Process. Mag. 37, 50–60. doi:10.1109/msp.2020.2975749
Liu, Y., Wang, J., Li, J., Niu, S., and Song, H. (2021). Machine learning for the detection and identification of internet of things devices: a survey. IEEE Internet Things J. 9, 298–320. doi:10.1109/jiot.2021.3099028
Lu, X., Wang, P., Niyato, D., Kim, D. I., and Han, Z. (2014). Wireless networks with rf energy harvesting: a contemporary survey. IEEE Commun. Surv. and Tutorials 17, 757–789. doi:10.1109/comst.2014.2368999
Lyu, F., Fang, L., Xue, G., Xue, H., and Li, M. (2019). Large-scale full wifi coverage: deployment and management strategy based on user spatio-temporal association analytics. IEEE Internet Things J. 6, 9386–9398. doi:10.1109/jiot.2019.2933266
Mehmood, Y., Ahmad, F., Yaqoob, I., Adnane, A., Imran, M., and Guizani, S. (2017). Internet-of-things-based smart cities: recent advances and challenges. IEEE Commun. Mag. 55, 16–24. doi:10.1109/mcom.2017.1600514
Muhammad, S., Zhao, J., and Refai, H. H. (2021). “An empirical analysis of ieee 802.11 ax,” in 2020 International Conference on Communications, Signal Processing, and their Applications (ICCSPA) (IEEE), 1–6.
Mulloni, A., Seichter, H., and Schmalstieg, D. (2012). Indoor navigation with mixed reality world-in-miniature views and sparse localization on mobile devices. Proc. Int. Work. Conf. Adv. Vis. Interfaces, 212–215. doi:10.1145/2254556.2254595
Naik, G., Ogbe, D., and Park, J.-M. J. (2021). Can Wi-Fi 7 support real-time applications? on the impact of multi link aggregation on latency. IEEE Int. Conf. Commun. (ICC’21), 1–6. doi:10.1109/ICC42927.2021.9500256
Namboodiri, V., Aravinthan, V., Mohapatra, S. N., Karimi, B., and Jewell, W. (2013). Toward a secure wireless-based home area network for metering in smart grids. IEEE Syst. J. 8, 509–520. doi:10.1109/jsyst.2013.2260700
Niu, S., Liu, Y., Wang, J., and Song, H. (2020). A decade survey of transfer learning (2010–2020). IEEE Trans. Artif. Intell. 1, 151–166. doi:10.1109/tai.2021.3054609
Organization WHO (2011). Environmental burden of disease associated with inadequate housing. Copenhagen: WHO Regional Office for Europe.
Oughton, E. J., Lehr, W., Katsaros, K., Selinis, I., Bubley, D., and Kusuma, J. (2021). Revisiting wireless internet connectivity: 5G vs Wi-Fi 6. Telecommun. Policy 45, 102127. doi:10.1016/j.telpol.2021.102127
Pahlavan, K., and Krishnamurthy, P. (2020). Evolution and impact of Wi-Fi technology and applications: a historical perspective. Int. J. Wirel. Inf. Netw. 28, 3–19. doi:10.1007/s10776-020-00501-8
Piekarski, W., Avery, B., Thomas, B. H., and Malbezin, P. (2003). “Hybrid indoor and outdoor tracking for mobile 3D mixed reality,” in The Second IEEE and ACM International Symposium on Mixed and Augmented Reality, 2003 (Washington, DC, United States: IEEE Computer Society), 266.
Pinheiro, A. J., de Araujo-Filho, P. F., Bezerra, J. d. M., and Campelo, D. R. (2020). Adaptive packet padding approach for smart home networks: a tradeoff between privacy and performance. IEEE Internet Things J. 8, 3930–3938. doi:10.1109/JIOT.2020.3025988
Pullmann, J., and Macko, D. (2019). A new planning-based collision-prevention mechanism in long-range iot networks. IEEE Internet Things J. 6, 9439–9446. doi:10.1109/jiot.2019.2940994
Qian, Y., Wu, D., Bao, W., and Lorenz, P. (2019). The internet of things for smart cities: technologies and applications. IEEE Netw. 33, 4–5. doi:10.1109/mnet.2019.8675165
Randall, D. (2003). Living inside a smart home: a case study. Inside Smart Home, 227–246. doi:10.1007/1-85233-854-7_12
Rault, T., Bouabdallah, A., and Challal, Y. (2014). Energy efficiency in wireless sensor networks: a top-down survey. Comput. Net. 67, 104–122. doi:10.1016/j.comnet.2014.03.027
Shah, B. M., Murtaza, M., and Raza, M. (2020). “Comparison of 4g and 5g cellular network architecture and proposing of 6g, a new era of ai,” in 2020 5th International Conference on Innovative Technologies in Intelligent Systems and Industrial Applications (CITISIA) (IEEE), 1–10.
Shlezinger, N., Alexandropoulos, G. C., Imani, M. F., Eldar, Y. C., and Smith, D. R. (2021). Dynamic metasurface antennas for 6g extreme massive mimo communications. IEEE Wirel. Commun. 28, 106–113. doi:10.1109/mwc.001.2000267
Showail, A., and Shihada, B. (2018). Battling latency in modern wireless networks. IEEE Access 6, 26131–26143. doi:10.1109/access.2018.2836439
Sivaraman, V., Gharakheili, H. H., Fernandes, C., Clark, N., and Karliychuk, T. (2018). Smart IoT devices in the home: security and privacy implications. IEEE Tech. Soc. Mag. 37, 71–79. doi:10.1109/MTS.2018.2826079
Song, H., Srinivasan, R., Sookoor, T., and Jeschke, S. (2017). Smart cities: foundations, principles, and applications. John Wiley and Sons.
Speicher, M., Hall, B. D., and Nebeling, M. (2019). What is mixed reality? Proc. 2019 CHI Conf. Hum. Factors Comput. Syst., 1–15. doi:10.1145/3290605.3300767
Sudevalayam, S., and Kulkarni, P. (2010). Energy harvesting sensor nodes: survey and implications. IEEE Commun. Surv. and Tutorials 13, 443–461. doi:10.1109/surv.2011.060710.00094
Syed, A. S., Sierra-Sosa, D., Kumar, A., and Elmaghraby, A. (2021). Iot in smart cities: a survey of technologies, practices and challenges. Smart Cities 4, 429–475. doi:10.3390/smartcities4020024
Talebkhah, M., Sali, A., Marjani, M., Gordan, M., Hashim, S. J., and Rokhani, F. Z. (2021). Iot and big data applications in smart cities: recent advances, challenges, and critical issues. IEEE Access 9, 55465–55484. doi:10.1109/access.2021.3070905
Tepper, O. M., Rudy, H. L., Lefkowitz, A., Weimer, K. A., Marks, S. M., Stern, C. S., et al. (2017). Mixed reality with hololens: where virtual reality meets augmented reality in the operating room. Plastic Reconstr. Surg. 140, 1066–1070. doi:10.1097/prs.0000000000003802
Turchet, L., and Rinaldo, E. (2021). Technical performance assessment of the ableton link protocol over wi-fi. J. Audio Eng. Soc. 69, 748–756. doi:10.17743/jaes.2021.0014
Wang, Q., Yu, J., Chen, S., and Xiang, Y. (2020). Sok: diving into dag-based blockchain systems. arXiv Prepr. arXiv:2012, 06128. doi:10.1145/3576899
Wang, Z., Hong, T., Piette, M. A., and Pritoni, M. (2019). Inferring occupant counts from wi-fi data in buildings through machine learning. Build. Environ. 158, 281–294. doi:10.1016/j.buildenv.2019.05.015
Xu, K., Wang, X., Wei, W., Song, H., and Mao, B. (2016). Toward software defined smart home. IEEE Commun. Mag. 54, 116–122. doi:10.1109/MCOM.2016.7470945
Yan, W., Wang, Z., Wang, H., Wang, W., Li, J., and Gui, X. (2022). Survey on recent smart gateways for smart home: systems, technologies, and challenges. Trans. Emerg. Telecommun. Technol. 33, e4067. doi:10.1002/ett.4067
Yang, Z., Xu, W., Huang, C., Shi, J., and Shikh-Bahaei, M. (2020). Beamforming design for multiuser transmission through reconfigurable intelligent surface. IEEE Trans. Commun. 69, 589–601. doi:10.1109/tcomm.2020.3028309
Ye, F., Qian, Y., and Hu, R. Q. (2018). Smart service-aware wireless mixed-area networks. IEEE Netw. 33, 84–91. doi:10.1109/mnet.2018.1700399
Ye, J., Dang, S., Shihada, B., and Alouini, M.-S. (2021). Modeling co-channel interference in the thz band. IEEE Trans. Veh. Technol. 70, 6319–6334. doi:10.1109/tvt.2021.3089427
Ye, J., Kammoun, A., and Alouini, M.-S. (2022). Reconfigurable intelligent surface enabled interference nulling and signal power maximization in mmwave bands. IEEE Trans. Wirel. Commun. 21, 9096–9113. doi:10.1109/twc.2022.3172885
Yu, L., Xie, W., Xie, D., Zou, Y., Zhang, D., Sun, Z., et al. (2019). Deep reinforcement learning for smart home energy management. IEEE Internet Things J. 7, 2751–2762. doi:10.1109/jiot.2019.2957289
Yüksel, I. (2012). Developing a multi-criteria decision making model for PESTEL analysis. Int. J. Bus. Manag. 7, 52. doi:10.5539/ijbm.v7n24p52
Zanella, A., Bui, N., Castellani, A., Vangelista, L., and Zorzi, M. (2014). Internet of things for smart cities. IEEE Internet Things J. 1, 22–32. doi:10.1109/jiot.2014.2306328
Zeng, S., Zhang, H., Di, B., Han, Z., and Song, L. (2020). Reconfigurable intelligent surface (ris) assisted wireless coverage extension: ris orientation and location optimization. IEEE Commun. Lett. 25, 269–273. doi:10.1109/lcomm.2020.3025345
Zhang, C., Dang, S., Alouini, M.-S., and Shihada, B. (2022a). Big communications: connect the unconnected. Front. Commun. Netw. 3, 4. doi:10.3389/frcmn.2022.785933
Zhang, C., Dang, S., Shihada, B., and Alouini, M.-S. (2021). “Dual attention-based federated learning for wireless traffic prediction,” in IEEE INFOCOM 2021-IEEE conference on computer communications (IEEE), 1–10.
Zhang, L., Zhang, C., Dang, S., and Shihada, B. (2022b). Lessons from the commercial failure of project loon for 6g research roadmap design. Front. Commun. Netw. 11. doi:10.3389/frcmn.2022.867581
Zhang, L., Zhang, C., and Shihada, B. (2022c). Efficient wireless traffic prediction at the edge: a federated meta-learning approach. IEEE Commun. Lett. 26, 1573–1577. doi:10.1109/lcomm.2022.3167813
Zhou, X., Zhang, R., and Ho, C. K. (2013). Wireless information and power transfer: architecture design and rate-energy tradeoff. IEEE Trans. Commun. 61, 4754–4767. doi:10.1109/tcomm.2013.13.120855
Keywords: beyond Wi-Fi, distributed network architecture, Federated learning, indoor data distribution, multi-band transmission, smart communication surfaces, smart home network
Citation: Amin O, Dang S, Abdelhady AM, Ma G, Ye J, Alouini M-S and Shihada B (2024) Beyond The Wi-Fi Era. Front. Comms. Net 5:1486488. doi: 10.3389/frcmn.2024.1486488
Received: 26 August 2024; Accepted: 09 September 2024;
Published: 25 September 2024.
Edited by:
George Tsoulos, University of Peloponnese, GreeceReviewed by:
Ahmad Bazzi, New York University Abu Dhabi, United Arab EmiratesCopyright © 2024 Amin, Dang, Abdelhady, Ma, Ye, Alouini and Shihada. This is an open-access article distributed under the terms of the Creative Commons Attribution License (CC BY). The use, distribution or reproduction in other forums is permitted, provided the original author(s) and the copyright owner(s) are credited and that the original publication in this journal is cited, in accordance with accepted academic practice. No use, distribution or reproduction is permitted which does not comply with these terms.
*Correspondence: Basem Shihada, YmFzZW0uc2hpaGFkYUBrYXVzdC5lZHUuc2E=