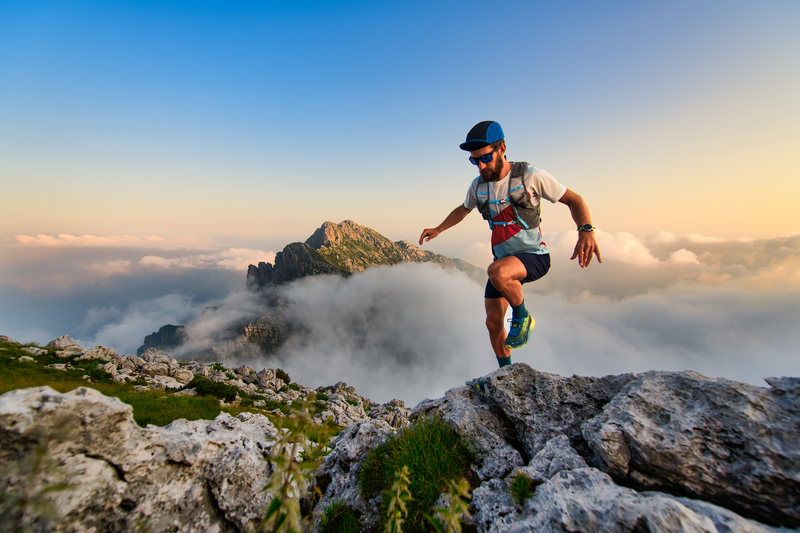
95% of researchers rate our articles as excellent or good
Learn more about the work of our research integrity team to safeguard the quality of each article we publish.
Find out more
ORIGINAL RESEARCH article
Front. Commun. Netw. , 06 December 2024
Sec. Signal Processing for Communications
Volume 5 - 2024 | https://doi.org/10.3389/frcmn.2024.1477270
This article is part of the Research Topic Emerging Optimization, Learning and Signal Processing for Next Generation Wireless Communications and Networking View all articles
The integration of terahertz (THz) communication with cell-free massive multiple-input multiple-output (CFMM) systems presents a promising strategy to enhance energy efficiency and reduce system complexity in future wireless networks. However, this integration faces significant challenges, such as dynamic and unpredictable channel behavior. Traditional channel estimation techniques are inadequate for handling these dynamic conditions. To address these issues, a convolutional neural network (CNN)-based hybrid precoding scheme is proposed for CFMM systems operating at THz frequencies. This method leverages CNN to predict optimal precoding weights, significantly improving the adaptability of hybrid precoding. The CNN-based model not only mitigates pilot contamination (PC) but also enhances channel estimation by capturing temporal and spatial dynamics. Simulation results indicate that the CNN-based approach achieves superior energy efficiency and lower system complexity compared to conventional techniques. At a signal-to-noise ratio (SNR) of 30 dB, it achieves 1.2 bits per joule and reduces system complexity to 1,400 FLOPs, demonstrating better scalability and resource optimization. These findings highlight the potential of CNN-based hybrid precoding to revolutionize THz communication in next-generation wireless networks by optimizing energy efficiency and system complexity.
1. The paper developed a novel hybrid precoding scheme using convolutional neural networks (CNNs) for dynamic terahertz communication channel conditions.
2. The proposed CNN-based approach significantly improves energy efficiency and reduces system complexity compared to state-of-the-art systems.
3. The proposed CNN-based hybrid precoding scheme improves adaptability and mitigates pilot contamination in a cell-free massive MIMO (CFMM) framework operating at THz frequencies.
The rapid evolution of wireless communication technologies has propelled the demand for higher data rates, lower latency, and increased energy efficiency. As we transition into the era of 5G and beyond, terahertz (THz) communication emerges as a pivotal technology due to its potential to provide unprecedented bandwidths and data rates. Concurrently, cell-free massive multiple-input multiple-output (CFMM) systems represent a significant advancement in wireless networks, promising to enhance coverage and capacity by leveraging a large number of distributed antennas (Zhao et al., 2024). The integration of THz communication with CFMM systems is anticipated to meet the escalating requirements of future wireless networks. However, this integration introduces formidable challenges, particularly in terms of energy efficiency and system complexity (Gnanaprakash and Perarasi, 2023).
A fundamental advancement in this domain is hybrid precoding, a technique that optimizes the number of radio frequency (RF) chains while preserving the achievable sum-rate performance. Hybrid precoding has gained prominence, especially in high-frequency massive MIMO systems, as it strikes a balance between performance and resource utilization. However, the conventional hybrid precoding methods encounter limitations due to the high hardware complexity and power consumption associated with large-scale antenna arrays. These limitations necessitate the development of more efficient and adaptive precoding techniques (Apiyo and Izydorczyk, 2024). The computational complexity of hybrid precoding is notably high, owing to the vast number of antennas and the intricacies of the high-dimensional channel. Addressing these complexities is fundamental to realizing the full potential of hybrid precoding in enabling efficient and robust communication networks for the future (Ozen and Guvensen, 2023).
CFMM systems have emerged as a revolutionary architectural solution in the field of wireless communication over the past few years. These systems are characterized by their distributed antenna configurations, which enable simultaneous connections to mobile stations from a multitude of nearby access points (APs). This unique feature enhances end-to-end connectivity, thereby improving the overall performance. Furthermore, these systems offer significant advantages, such as improved signal reception, reduced latency, and minimized power consumption. The latter is particularly beneficial as it results from the shorter transmission distances of the active links in the wireless orientation, leading to a more energy-efficient network (Ng and Raju, 2024).
The role of machine learning (ML), specifically convolutional neural network (CNN), in addressing the challenges associated with these systems cannot be overstated. CNNs have proven to be highly effective in handling sequential data and capturing temporal dependencies. This makes them an ideal choice for predicting and adapting to varying channel conditions in real time. The integration of CNNs into the hybrid precoding process is a significant advancement in this field. It allows for the dynamic adjustment of the precoding weights based on the current channel conditions. This not only enhances the energy efficiency of the system but also minimizes its complexity, making it a viable solution even in the challenging THz band. This integration of ML with CFMM systems represents a significant stride in the evolution of wireless communication systems (Sun et al., 2023).
Hybrid precoding is essential to maximizing the performance of CFMM systems in THz communication. In massive MIMO systems, this technology effectively manages the large array of antennas by combining digital and analog precoding in a way that balances hardware complexity, power consumption, and overall performance. However, the fast-varying channel conditions typical of THz frequencies cause standard hybrid precoding techniques to fail (Ali K et al., 2023).
CNNs are particularly well-suited for real-time prediction and response to dynamic channel circumstances because they can analyze sequential input and capture temporal dependencies. By using real-time data to train and update precoding algorithms dynamically, CNNs can improve system robustness and flexibility in contrast to previous methods that rely on static models and cannot handle the high fluctuation of THz channels (Jayarin and Sekar, 2023).
The main contributions of the paper are summarized as follows:
• Development of novel hybrid precoding scheme: This paper introduces a novel hybrid precoding scheme utilizing CNNs tailored for THz communication. The scheme exploits the adaptive capabilities of CNNs to manage precoding weights effectively, optimizing performance amid dynamic channel conditions inherent in THz environments.
• Performance optimization and energy efficiency: The CNN-based hybrid precoding method markedly improves energy efficiency and reduces system complexity. Comprehensive analysis and extensive simulations reveal that this innovative approach significantly outperforms traditional methods in terms of performance and efficiency within a CFMM framework.
• Validation and superior performance in a CFMM framework: Extensive simulations conducted within a CFMM framework substantiate the effectiveness of the proposed hybrid precoding scheme. The results demonstrate its superior performance compared to state-of-the-art systems, highlighting its potential to advance THz communication technologies by enhancing both efficiency and reliability.
The organization of the paper is as follows: Section 2 offers an exhaustive survey of the current literature. Section 3 delves into the intricacies of the system model and the formulation of the problem. Section 4 provides a detailed explanation of the design of MMSE-based hybrid precoding for downlink multi-user massive MIMO systems. Sections 5, 6, 7, 8 delve into the pilot assignment algorithm, which is designed to mitigate pilot contamination (PC) in cell-free networks, the application of ML techniques, complexity analysis, and the presentation of results and discussions, including the outcomes of simulations, respectively, and Section 9 concludes the paper.
A considerable body of research has been dedicated to exploring the performance of ML-based hybrid precoding, with a particular focus on enhancing energy efficiency and reducing system complexity in wireless communication. This has been especially prevalent in the study of CFMM systems integrated with THz communication. Recent advancements have led to the introduction of various innovative strategies aimed at optimizing these systems (Mohammadi et al., 2023). A substantial number of these studies have honed in on evaluating the effectiveness of CNN-based hybrid precoding. They have compared it with traditional methods and analyzed its performance under different channel conditions and system parameters (Gao et al., 2017). This section aims to examine existing research, identify challenges, and set the stage for targeted exploration. The study also delves into the development and optimization of hybrid precoding algorithms using CNNs, investigating their effectiveness in addressing energy efficiency and system complexity challenges.
The authors in (Ozgur Gurbuz, 2021) explored the defining features of THz wireless systems, shedding light on the transformative potential of cell-free massive MIMO in enhancing THz links. Their study emphasized the intrinsic challenges associated with channel estimation in THz communication while exploring the mitigative potential of hybrid precoding strategies in reducing system complexity.
The authors in (Elbir, 2019) introduced a CNN framework for designing precoders and combiners designs specifically for massive MIMO downlink transmissions in beyond 5G networks, which have been evaluated for energy efficiency and system simplicity. These designs show significant promise for practical implementation in advanced communication networks, underscoring their real-world applicability and paving the way for seamless integration into the evolving landscape of wireless communication technologies. The findings suggest that CNNs can significantly enhance hybrid beamforming performance, improving communication efficiency and reducing computational demands.
The authors in Tentu et al. (2020) presented how CNNs can be used for adaptive hybrid precoding in millimeter wave MIMO systems, which are a forerunner to the more complex THz communication systems. In order to improve signal transmission efficiency, their study focuses on employing CNNs to dynamically modify hybrid precoding matrices and balance digital and analog components. Their study, which extensively evaluated the framework’s performance concerning energy efficiency and system complexity, showcased its remarkable scalability and adaptability.
The authors in Abugubba et al. (2022) presented CNN-based hybrid precoding model for mmWave massive MIMO systems, achieving performance comparable to fully-digital precoders while reducing computational complexity. It demonstrates the effectiveness of deep learning in optimizing precoding strategies and validates the model against traditional algorithms through simulations. However, the reliance on simulation data raises concerns about generalizability, and the study does not address varying channel conditions. Future research should explore the model’s robustness in real-world scenarios and integrate other deep learning techniques to enhance performance.
The authors in Biswas and Vijayakumar (2021) conducted Two Deep Learning (DL) methods were proposed and evaluated for channel estimation in massive MIMO configurations. The first method employed a neural network composed of fully connected layers. Conversely, the second method utilized a CNN to extract spatial information from the channel covariance matrix, which was subsequently used to eliminate intercell interference. The study also accounted for factors such as imperfect synchronization and channel aging.
The authors in Nguyen et al. (2020) explored the maximization of energy efficiency in two-way amplify-and-forward half-duplex MIMO relaying. In this scenario, multiple user pairs exchange information through a shared relay. Unlike conventional iterative optimization methods, the authors introduced a Deep Neural Network (DNN) in the uplink (UL) mode. This approach demonstrated optimal performance, distinguishing itself from existing iterative optimization methods.
The authors in Khan et al. (2020) introduce the concept of CFMM systems. In this context, they present closed-form expressions. The energy efficiency is maximized through a two-step optimization approach. The first step involves formulating the convex problem, while the second step solves it in a decentralized manner using the method of multipliers.
Despite significant advancements in hybrid precoding techniques utilizing neural networks within wireless communications, the majority of contemporary research remains concentrated on traditional cellular architectures and hybrid precoding for 5G technologies. Such methodologies fall short in addressing the intricate and dynamic channel characteristics inherent to THz communication. This oversight has led to a discernible void in literature pertaining to CNN-based solutions tailored for THz CFMM systems. Our research endeavors to bridge this gap by pioneering CNN-based hybrid precoding specifically designed for CFMM systems operating within the THz spectrum. We introduce innovative algorithmic enhancements aimed at mitigating system complexity and augmenting energy efficiency, which dynamically adapt to the volatile and unpredictable channel conditions characteristic of THz frequencies. The results highlight the profound influence of this approach in optimizing THz communication for CFMM systems, thereby setting the stage for more sophisticated and dependable wireless technologies in the forthcoming era.
This review of the literature highlights significant developments and points out persistent gaps, providing an overview of the state of existing research. The problem formulation and system model will be discussed in the upcoming section 3.
This research work focuses on a massive MIMO system with a fully integrated hybrid precoding structure that is intended for multiple users. In order to enable the deployment of several distributed APs throughout the coverage area, the system adopts a cell-free architecture, as shown in Figure 1.
In the context of CFMM systems, each access point is equipped with NTR antennas and KRF RF chains to serve K mobile stations (MS). Conversely, each MS is equipped with NRT antennas and an RF chain. Within CFMM, which eliminates traditional cell boundaries, each AP employs a digital baseband precoder represented by
The elements of the analog precoding matrix
Let
The signal after combining at the ktℎ user terminal is modeled as Equation 4 (Section 3.1).
where
In the context of CFMM, the signal-to-interference-plus-noise ratio (SINR) is calculated by considering the power of the desired signal received at the user terminal normalized by total interference and noise power (Zhong et al., 2021).
The sum rate
Energy efficiency in a cell-free massive MIMO system can indeed be calculated based on the Shannon capacity formula, which considers the achievable data rate and the total power.
The power consumption model, derived from hybrid precoder studies (Rekkas et al., 2021) and (Zhang et al., 2021), accounts for the total power usage of the system architecture. The total power consumption is denoted as
In Equation 9, typical power consumption values are used, reported in existing THz studies (Zhang et al., 2021), typically around 0.1 THz, measured in mill watts. where
Owing to the short wavelength of THz signals, their transmission is marked by pronounced attenuation and limited scattering, leading to a predominantly line of sight propagation. This research introduces a THz-specific clustered channel model to navigate the distinct propagation challenges at THz frequencies, such as acute path loss and marked absorption. The model incorporates scattering clusters, each consisting of
In this model,
At THz frequencies, where traditional estimate methods fail due to the dynamic and sparse nature of the channels, effective channel state information (CSI) generation is essential for accurate precoding in Cell-Free Massive MIMO systems. To address this problem, we propose a CNN-based method trained on a dataset of channel realizations, leveraging temporal and spatial correlations in the THz channel. This model adapts to rapid changes in the channel, reduces estimation errors, and boosts overall system efficiency. As a result, it offers precise, real-time CSI estimates that significantly improve precoding performance.
In multi-user massive MIMO systems, hybrid precoding is typically approached as an optimization problem, with the key objective being the maximization of the total sum-rate across all users. This involves finding a balanced synergy between digital and analog precoding to ensure efficient data flow across the network, as explained in Hojatian et al. (2021). To that aim, an optimization model has been developed that combines both analog and digital precoding strategies with the intention of maximizing the system’s sum-rate.
This optimization process is formalized through Equation 11, which decomposes the hybrid precoding task into three distinct components: the analog precoder (
The decomposition of Equation 11 into analog and digital parts allows the system to divide complex tasks between two domains, optimizing resource usage. In massive MIMO systems, directly solving nonconvex optimization challenges can be computationally prohibitive. The hybrid precoding scheme employs both analog and digital components to optimize performance while managing complexity, particularly in terahertz communication environments. The analog part, represented by the analog precoder
The direct derivation from the nonconvex optimization challenge does not yield a straightforward resolution explained in Ammar et al. (2021). However, by decomposing the problem into its analog and digital components, a systematic approach can be developed. In the context of enhancing the antenna array gain, the effective channel
The optimization constraints for the analog precoder are described by Equation 12 (Section 3.2).
Here, the analog precoder and combiner handle most beamforming tasks that are computationally intensive but essential for optimizing overall signal gain.
The optimization problem for the digital precoder is captured by Equation 13 (Section 3.2).
This allows the digital precoding component to focus on optimizing sum-rate for all users, ensuring that each user receives the signal based on real-time conditions. The hybrid precoding scheme in cell-free massive MIMO systems optimizes performance and energy efficiency by decomposing Equation 11 into analog and digital components. Analog precoding, operating at the RF level, manages beamforming and reduces power consumption by limiting the number of RF chains needed, while digital precoding fine-tunes signals at the baseband to maximize signal-to-interference-plus-noise ratio and enhance data rates. This decomposition simplifies computational complexity, making the system easier to implement and scale, especially in high-frequency THz communication environments.
We presented the hybrid precoding optimization problem in the context of THz communication in Section 3, along with the system model for our CFMM. A hybrid precoding architecture based on minimum mean squared error (MMSE) will be presented and developed in Section 4, which builds on this foundation and is specifically designed for the downlink multi-user massive MIMO system.
For data streams sent to mobile stations, the mean squared error (MSE) is minimized by applying the MMSE criterion. This criterion seeks to minimize
The MMSE-based analog precoder relies on channel knowledge,
Considering the channel
The RF combiner Gk is designed based on the MMSE criterion as shown in Equation 17 (Section 4.1).
The analog combiner can possibly be expressed as:
where
We can obtain the effective channel after determining the analog precoder and channel matrix and designing the analog combiner. The channel matrix and analog precoder and combiner work together to create the effective channel.
It considerably simplifies the system model, allowing for more controllable digital precoding and combining in subsequent stages. The effective channel after analog precoding and combining is expressed as Equation 19 (Section 4.1).
The goal of the analog precoder is to maximize the antenna array gain. Based on the analog precoder
The power constraints can be satisfied by normalizing the digital precoder
Algorithm 1.MMSE Based Hybrid Precoding Require: H_k and P.
For k = 1,K Do
Calculate Analog Precoder
End for
Calculate effective channel
Compute Digital Precoder
Normalize Digital Precoder
This section has discussed the MMSE based hybrid precoding design for downlink MU massive MIMO system, highlighting its significance in our study. Next, we will proceed with the pilot assignment algorithm for cell-free networks.
This research investigates a cell-free network configuration comprising “M” APs with “N” antennas and “K” user equipment’s (UEs), each equipped with multiple antennas, distributed randomly within a specified coverage area. Despite the expected scenario where M significantly exceeds K in CFMM systems, the architecture maintains efficiency across all values due to its scalable design. Each AP is connected to a central processing unit (CPU) via a backhaul network. Channel modeling follows the Rayleigh fading model, describing the channel between UE “k” and AP “m” as:
Here,
PC is a situation where interference is caused due to the usage of identical pilot sequences by different user equipments (UEs). To address this issue, a unique pilot assignment strategy is suggested (Gkonis, 2023). In this strategy, each UE is assigned a pilot depending on its proximity to the closest AP. If there are multiple APs at the same distance, the pilot is allocated based on the large-scale fading coefficient, denoted as β. The assignment algorithm involves three key steps. Firstly, UE selection prioritizes UEs with lower channel quality for pilot assignment by calculating and sorting the large-scale fading coefficients, β, in descending order. Secondly, during AP selection, UEs use synchronization signals to connect with nearby APs, with the closest AP serving the UE. If multiple APs are equidistant, the AP with the highest β is chosen. Lastly, in pilot allocation, the serving AP assigns an unused pilot to the UE and informs neighboring APs to avoid using the same pilot, thereby reducing PC and enhancing system performance.
When UEs send out pilot signals, the signal that is picked up by the AP at the m-th position is:
Here
Algorithm 2.Optimal Pilot Allocation Algorithm. Algorithm 2 outlines the optimal pilot allocation strategy used for mitigating pilot contamination (Section 5.3).
Input:
Output: optimal
1. Initialize:
2. Compute: Calculate β for all users and sort into s
3. Pilot Assignment Condition:
• if
• if
4. Optimal Pilot Selection: Choose the pilot with minimal interference impact on
5. Return: Optimal ∅_k
To optimize pilot allocation, we utilize the optimal pilot allocation algorithm tailored to mitigate PC and improve CE accuracy in CFMM systems. It aims to mitigate PC and improve channel estimation (CE) accuracy. The algorithm prioritizes UEs with poor channel quality for network access, identifies the most suitable AP to serve the UE, and assigns pilots in a way that minimizes PC. It iteratively selects the best AP and assigns the optimal pilot to reduce interference. The effectiveness of this algorithm is demonstrated by its ability to strategically assign orthogonal pilot sequences, thereby reducing PC, enhancing CE accuracy, optimizing resource allocation, decreasing channel correlation, minimizing mutual interference between UEs, and ultimately improving overall system performance.
By presenting a novel pilot assignment algorithm in Section 5, we addressed the important problem of PC in cell-free networks. Building upon this advancement, Section 6 investigates the integration of ML techniques aimed at significantly enhancing the performance of CFMM systems. The received pilot signal model and its noise-reduction mechanism are detailed in Equations 24, 25 (Section 5.2).
Machine learning has significantly transformed wireless communication by facilitating adaptive, data-driven enhancements, particularly in the complex domain of THz communication. Convolutional Neural Networks are particularly adept at predicting channel state information by identifying spatial features within data. Their implementation in THz systems contributes to enhanced energy efficiency, reduced system complexity, and improved accuracy in channel estimation. These advancements collectively lead to superior overall performance, addressing the increasing demands for higher data rates, lower latency, and greater energy efficiency in future wireless networks (Nguyen et al., 2020).
While traditional mathematical models provide a foundational understanding of communication systems, they often depend on assumptions that may not be applicable in real-world scenarios—especially in intricate environments such as cell-free massive MIMO systems operating within THz frequencies. In contrast, CNNs excel at learning complex, non-linear relationships from data, enabling them to adapt effectively to variations in channel conditions, noise levels, and other dynamic factors that traditional models may struggle to accommodate.
A proposed CNN neural network architecture, shown in Figure 2, predicts the precoding and combining vectors for a hybrid system directly from the received CSI.
The neural network consists of eight layers: an input layer, two convolutional layers, a pooling layer, three fully connected layers, and a regression output layer. Each layer contains multiple units, generating outputs using activation functions. The input includes three channels: the element-wise absolute value and the real and imaginary parts of the channel matrix. The convolutional layers, using ReLU activation, extract and select data features. After feature extraction, the maximum pooling layer compresses the parameters, and the data is rearranged into a one-dimensional vector. Fully connected layers then perform weighted sums and map features to the sample space. A dropout layer is added to prevent overfitting during training. Finally, the regression layer outputs result by computing mean squared error loss.
CNNs were chosen for this research despite their limitations in handling temporal data because their strengths lie in capturing spatial and spectral dependencies within each coherence time frame. While channel data in massive MIMO systems exhibit temporal correlations, the CNN-based approach focuses on extracting spatial patterns that are crucial for hybrid precoding and resource allocation. This eliminates the need for memory units, such as recurrent neural networks. Furthermore, by continuously updating the model with real-time CSI, the CNN adapts dynamically to temporal variations, thereby minimizing the need for handling long-term sequences. This design choice achieves a balance between reducing computational complexity and optimizing system performance, making CNNs a suitable solution for real-time, energy-efficient cell-free massive MIMO systems under THz communication conditions.
Max pooling was employed in the CNN architecture due to its ability to retain critical features while reducing computational complexity, which is essential for real-time hybrid precoding in massive MIMO systems. Max pooling extracts the dominant spatial characteristics by selecting the maximum value within a pooling window. Although this process may result in the loss of some high-frequency details, the trade-off is justified, especially given the shallow nature of the CNN architecture, which only comprises two convolutional layers. Experiments show that max pooling allows the system to maintain good generalization across diverse channel conditions while significantly reducing system complexity. In this study, max pooling was specifically chosen to preserve the most important features required for the CNN-based hybrid precoding system, striking an effective balance between feature extraction and computational efficiency.
Residual connections were not utilized in this research, as the primary objective was to reduce system complexity and enhance energy efficiency for real-time hybrid precoding tasks in THz communication systems. Typically, residual connections are employed in deeper networks to address vanishing gradient issues. However, since our CNN model is relatively shallow comprising only two convolutional layers the architecture was considered sufficient for effective feature extraction and system performance without adding unnecessary computational overhead. Prior works, such as those referenced in Elbir (2019); Abugubba et al. (2022), have shown that a similar structure without residual connections was effective for feature extraction while maintaining computational efficiency. Moreover, the use of ReLU, suitable activation function and Adam optimizer further enhances the learning process in this simpler architecture. The model’s training on a substantial dataset also aids in learning robust features critical for hybrid precoding. While residual connections could be explored in future research for deeper architectures, the current design strikes an optimal balance between model complexity and computational efficiency, making it ideal for real-time deployment in CFMM systems.
The proposed framework utilizes a CNN to optimize the hybrid precoding process in cell-free massive MIMO systems at THz communication frequencies. As shown in Figure 3, the hybrid precoding structure enabled by CNN processes various factors, including the channel matrix, spatial correlation, mobility, channel phase noise, power fading, and shadowing, along with analog and digital precoder information. The CNN outputs parameters for the analog precoder, digital precoder, and analog combiner, which are crucial for optimizing precoding and enhancing throughput, energy efficiency, and reliability. This approach makes the hybrid precoder system adaptive, allowing it to optimize beamforming strategies based on learned information and adjust parameters over time in response to changing channel conditions.
In this architecture, CNNs are used at both the transmitter and receiver ends, processing beamforming matrices by extracting key features that improve overall communication efficiency. The independent design of the transmitter and receiver allows for separate optimization, simplifying the design process while maintaining effective performance tuning for different operational environments.
Performance evaluation of the CNN-based approach is conducted using metrics like energy efficiency and system complexity. Our results show that CNN-driven methods achieve comparable performance to traditional optimization techniques, while significantly lowering computational overhead.
The process begins by initializing the CNN with dynamic channel data and precoding information. The CNN then generates analog and digital precoders, as well as analog combiner parameters to optimize system performance. These parameters are continuously updated by the CNN to adapt to changing channel conditions. During transmission and reception, signals are processed using the hybrid precoder parameters to achieve efficient communication. This adaptive approach enables enhanced throughput, energy efficiency, and reliability in cell-free massive MIMO systems operating at THz communication frequencies.
The essential data generation parameters for CNN-based hybrid precoding in cell-free massive MIMO at THz frequencies encompass real and imaginary channel gains, channel phases, spatial correlation among antennas, noise power, transmitted signal power, path loss, shadowing, and fading effects. These parameters are reflected in metrics such as signal strength, phase shifts, correlation levels, noise levels, signal attenuation, and environmental variations. Moreover, the weights for digital and analog precoding, along with the analog combining matrix, play a pivotal role in controlling the hybrid precoding process. This diversity in data is vital for effectively training and testing the CNN model, ensuring robust and reliable performance in THz communication environments.
This research involves the implementation and evaluation of a CNN model intended for hybrid precoding weight prediction in dynamic wireless communication contexts. The actual implementation involves training the CNN on relevant datasets, including synthetic data designed to simulate various channel conditions. Once trained, the CNN can be integrated into both transmitter and receiver hardware for real-time operation. This integration facilitates efficient processing of beamforming tasks, leading to reduced computational overhead and improved energy efficiency.
To determine the model’s dependability and effectiveness, we use 70% of the dataset for training, followed by extensive testing and validation phases. Under THz communication conditions, the main goal is to maximize energy efficiency and reduce system complexity in CFMM systems.
• Training Phase: The CNN is trained with 70% of the dataset, which includes dynamic channel data and precoding features such as real and imaginary channel gains, channel phases, and noise power. To reduce the mean squared error (MSE) loss function, the ADAM optimizer is used with a learning rate of 0.001 and a batch size of 137. Throughout the training procedure, the CNN’s weights are continually modified to improve predicting accuracy. The model is trained across 250 epochs, with the fit method and the provided batch size used.
• Testing Phase: After training, the performance of the CNN is evaluated on 15% of an independent dataset. In this stage, the model’s ability to generalize and accurately predict hybrid precoding weights across a range of channel conditions is assessed.
• Validation Phase: To ensure optimal model performance and reduce the possibility of overfitting, 15% of the dataset is set aside for validation during the training phase. In order to optimize hyperparameters and guarantee efficient learning, the CNN’s performance on the validation set is continuously observed. During this validation stage, CNNs in CFMM systems operating in THz communication settings can best respond to real-time channel fluctuations while preserving high energy efficiency and reducing system complexity. This systematic procedure ensures that the CNN model is properly evaluated, validated, and rigorously trained, resulting in dependable performance in scenarios involving dynamic wireless communication.
The training data are shuffled to improve the model’s generalization across various channel conditions and to prevent over fitting. The focus is on capturing spatial and spectral features rather than temporal dependencies, as the hybrid precoding system operates within the short coherence time of THz channels. While not shuffling could preserve temporal dependencies, it would necessitate more complex models like attention mechanisms or RNNs, increasing computational complexity. This research aims to balance performance and system efficiency, with the CNN-based approach optimizing spatial and spectral patterns to achieve energy efficiency and reduced complexity in real-time massive MIMO systems at THz frequencies.
To address the complex demands of CFMM systems in THz communication, a specialized CNN configuration is designed. This setup features the Adam optimizer, selected for its adaptability in adjusting learning rates, which facilitates efficient convergence and robust performance across diverse scenarios. The ReLU activation function is employed to capture the nonlinear relationships crucial for hybrid precoding. Additionally, the Mean Squared Error (MSE) loss function is utilized, prioritizing precise channel estimation by minimizing the average squared difference between predicted and actual values, thus enhancing regression performance (Ammar et al., 2021). This configuration aims to improve performance and reliability by efficiently managing large datasets, ensuring compatibility with real-world requirements, and minimizing errors in predicted channel characteristics. Consequently, it enables accurate precoding decisions in dynamic communication scenarios.
In Section 6, we delve into the integration of ML techniques with MMSE-based hybrid precoding to augment the performance of CFMM systems. Having established these methodologies, we proceed to Section 7, where we analyze the system complexity of the proposed CNN-based hybrid precoder.
The complexity of the CNN-based precoding method is primarily determined by the convolutional layers used in the neural network. The key components of the CNN model include a convolutional layer, a pooling layer, and a dense layer. The input to the convolutional layer is channl matrix H with 64 filters and a kernel size of 1. The pooling layer has a pooling size of 2, and the dense layer comprises 128 units.
Table 1 compares the computational complexity of different precoding schemes, including the proposed CNN-based method (Section 7). To understand the complexity of the convolutional layer, we can approximate it by calculating the number of operations required to convolve the input feature map with the filters. The operations per filter can be represented as
For 64 filters, the total number of operations for the convolutional layer is approximately
The complexity of the pooling layer, particularly a max pooling layer with a pool size of 2, is significantly lower because it involves taking the maximum of every 2 elements. The total operations for the pooling layer can be approximated as
For the dense layer with 128 units, the input to this layer is from the previous layer’s output. Assuming the output dimension from the previous layer is proportional to
Complexity of fully connected layers.
Consider a fully connected layer where each neuron in the layer connects to every input feature. The complexity arises from the number of multiplications and additions required. The input to the fully connected layer be a matrix where dimensions involve
Total possible pairs of inputs.
Considering all interactions, the complexity term for the fully connected layers that account for these pairs is:
where
The value of β was selected as 55 by benchmarking against related works in Zhong et al. (2021). Various research papers that analyze the complexity of fully connected layers in neural networks were reviewed to determine an appropriate scaling factor. By comparing the computational loads and performance metrics reported in Zhang et al. (2021). This benchmarking ensures that the chosen β value is representative of typical computational demands in similar contexts and provides a reliable estimate for modeling the complexity of fully connected layers in CNN-based precoding methods.
Then fully connected layers complexity become
Combining all the terms, we get the overall complexity:
In Section 7, we analyze the complexity of the proposed CNN-based hybrid precoder. We now advance to Section 8, where we present the results of our simulation evaluations and engage in a comprehensive discussion of the findings.
The simulation parameters for the hybrid precoder research encompasses several critical components. The setup includes 256 transmit antennas and 64 receive antennas. The simulation models 5 paths and 10 clusters, operating at a frequency of 0.1 THz. Arrival and departure angles for paths are randomly generated between 0 and 2π. Channel gains for clusters and paths follow a complex normal distribution with a mean of 0 and variance of 1. The channel matrix is derived based on the numbers of transmitter and receiver antennas, as well as the channel gains. The noise variance is set to 10 dB. Additionally, the simulation involves 50 users and utilizes a transmit power of 30 dB.
Figure 4 presents the energy efficiency achieved by the CNN-based hybrid precoding method compared to the MMSE, regularized zero-forcing (RZF), and zero-forcing (ZF) hybrid precoding methods. The Figure clearly demonstrates the proposed CNN-based hybrid precoding method significantly outperforms traditional methods such as MMSE, RZF, and ZF in terms of energy efficiency, particularly at higher signal-to-noise ratio (SNR) levels. Energy efficiency is computed using Equation 8, which is based on Shannon’s capacity formula. At −20 dB, all techniques exhibit similar energy efficiencies near zero. However, as the SNR increases, the performance distinctions become marked. The CNN-based approach achieves approximately 1.2 bits per joule at 30 dB, compared to about 1.0 bits per joule for MMSE, 0.8 bits per joule for RZF, and 0.6 bits per joule for ZF. This superior performance is attributed to the CNN’s enhanced capability to exploit high-quality signal environments. The integration of a CNN-trained model, using parameters from Table 2 and hybrid precoding parameters illustrated in Figure 3, further optimizes system performance, highlighting the robustness and potential of the CNN-based method for energy efficiency optimization.
Figure 5 illustrates the energy efficiency performance of various precoding schemes as the number of transmitter antennas increases. The CNN-based hybrid precoding method demonstrates superior energy efficiency as the number of transmitter antennas increases from 0 to 250, starting at around 0.1 bits per joule and rising to approximately 0.7 bits per joule. The MMSE method also shows high efficiency, peaking at about 0.6 bits per joule before flattening. RZF and ZF methods exhibit more moderate increases, reaching 0.45 bits per joule and 0.4 bits per joule, respectively. These findings highlight the CNN-based method’s superior performance in achieving higher energy efficiency, especially with an increasing number of transmitter antennas, demonstrating its potential in massive MIMO systems. The critical role of Equations 15, 18, which depend heavily on the number of transmitter antennas, underscores the impact of antenna count on energy efficiency. The CNN-based method’s advanced feature learning capabilities, robustness to noise and interference, and computational efficiency enable it to overcome the challenges faced by traditional methods, showcasing its potential for enhancing energy efficiency in large antenna systems.
Figure 6 demonstrates the energy efficiency of various transmission schemes in a massive MIMO system as the number of users increases from 0 to 50. The CNN-based hybrid precoding method consistently outperforms traditional methods (MMSE, RZF, and ZF) across this range. Starting at around 0.1 bits per joule, the CNN-based method’s efficiency rises steadily, peaking at approximately 0.8 bits per joule at 40 antennas before experiencing a slight decline. MMSE shows high efficiency, reaching about 0.6 bits per joule before flattening. RZF and ZF exhibit moderate increases, peaking at 0.5 and 0.45 bits per joule, respectively. This performance highlights the CNN-based method’s superior capability to achieve high energy efficiency, especially as the number of users increases, validating its effectiveness in massive MIMO systems. The increase in energy efficiency aligns with theoretical expectations based on the signal-to-interference-plus-noise ratio (SINR) as outlined in Equation 6. However, at higher user densities, the efficiency increase diminishes due to growing interference and limited channel resources, illustrating the practical limits of energy efficiency in densely populated user scenarios.
Figure 7 illustrates the CNN-based method’s higher energy efficiency over a variety of RF chains. As the number of RF chains increases, all approaches become less efficient due to increased system complexity and power consumption. The CNN-based technique is more efficient than MMSE, RZF, and ZF, especially when there are a few RF chains (2–4). Despite a general fall in efficiency with more RF chains, the CNN method’s decrease is more gradual, indicating superior scalability and resource optimization. These findings are in line with the mathematical model described in Section 3, which describes the performance limits of fully connected RF chain designs. Algorithm 1 describes in detail the formulas used for hybrid precoding, which are crucial to understanding the efficiency of these systems.
Figure 8 compares the computational complexity, measured in FLOPs, of four signal processing algorithms: MMSE, RZF, ZF, and CNN, across a range of SNR values from −20 dB to 30 dB. The MMSE algorithm exhibits the highest complexity, starting around 1,600 FLOPs and increasing to approximately 1,800 FLOPs as SNR rises. The RZF algorithm follows a similar trend, with complexity increasing from slightly above 1,400 FLOPs to around 1,600 FLOPs. In contrast, the ZF algorithm maintains a small increase in complexity of about 1,400 FLOPs. The CNN algorithm shows the lowest initial complexity at around 1,000 FLOPs, gradually increasing to approximately 1,400 FLOPs as SNR increases. These results validate the theoretical and mathematical analysis presented in Section 7.
Figure 9 shows the comparison of computational complexity of RZF, MMSE, ZF, and CNN as the number of antennas increases from 0 to 250. The MMSE algorithm exhibits the highest complexity, starting around 1,000 FLOPs and exceeding 2,400 FLOPs at 250 antennas, due to its reliance on complex matrix operations. RZF shows a similar trend, with complexity rising steeply from 1,000 to 2,200 FLOPs. The ZF algorithm demonstrates a more moderate increase in complexity, from 1,000 to 2000 FLOPs, indicating its simpler computational requirements. The CNN algorithm maintains the lowest initial complexity, starting at 1,000 FLOPs and increasing steadily to 1,600 FLOPs, showcasing its efficient scalability. The critical role of Equation 26, which depend heavily on the number of transmitter antennas, underscores the impact of antenna count on system complexity. These results align with the theoretical and mathematical analysis presented in Section 7, confirming the high computational demands of MMSE and RZF in large antenna systems, the moderate complexity of ZF, and the efficient scalability of CNN, providing a realistic assessment of computational requirements for these algorithms.
The CNN-based precoding method for THz communication presents a new way to handle THz channel challenges, but it has limitations that future research needs to address.
• Dependence on Accurate CSI: The CNN-based precoding relies heavily on accurate CSI, and any inaccuracies can degrade performance. Future work could explore advanced CSI techniques to address this issue.
• Computational Complexity: The CNN model introduces computational overhead, which may challenge real-time applications. Optimizing the model or using more efficient algorithms could help balance performance and computational demands.
• Limited Adaptability to Dynamic Channels: The CNN may struggle with rapidly changing channel conditions in THz communication. Research into adaptive mechanisms or enhanced training strategies could improve its adaptability.
The research examined CNN-based hybrid precoding in CFMM systems operating in the THz band. It found that using CNNs to dynamically configure analog and digital precoders improves energy efficiency and reduces system complexity, offering advantages over traditional precoding methods. The research findings highlight the superiority of CNN-based hybrid precoding in CFMM systems operating in the THz band under challenging conditions. The CNN-based method significantly enhances energy efficiency compared to traditional precoding techniques like MMSE, RZF, and ZF. At a high SNR of 30 dB, the CNN-based approach achieves approximately 1.2 bits per joule, outperforming MMSE at 1.0 bits per joule, RZF at 0.8 bits per joule, and ZF at 0.6 bits per joule. This superior performance extends across varying numbers of antennas and receiver chains. With increasing antenna counts, the CNN-based method maintains higher energy efficiency, achieving around 0.8 bits per joule with 32 antennas, while MMSE, RZF, and ZF lag behind at approximately 0.52, 0.45, and 0.42 bits per joule, respectively. Additionally, the CNN-based approach exhibits better scalability and resource optimization, particularly evident with fewer RF chains where it shows a more gradual decline in efficiency compared to traditional methods. Furthermore, the method demonstrates robustness in managing challenges posed by THz frequencies and system complexity effectively. These findings underscore the potential of CNN-based precoding to meet the demands of high data rates and low latency in next-generation wireless networks, suggesting avenues for future research into its scalability across diverse communication environments and integration with advanced technologies.
The original contributions presented in the study are included in the article/supplementary material, further inquiries can be directed to the corresponding authors.
TA: Supervision, Writing–original draft, Writing–review and editing. YM: Writing–original draft, Writing–review and editing. BA: Writing–original draft, Writing–review and editing. NG: Writing–original draft, Writing–review and editing.
The author(s) declare that no financial support was received for the research, authorship, and/or publication of this article.
The authors declare that the research was conducted in the absence of any commercial or financial relationships that could be construed as a potential conflict of interest.
All claims expressed in this article are solely those of the authors and do not necessarily represent those of their affiliated organizations, or those of the publisher, the editors and the reviewers. Any product that may be evaluated in this article, or claim that may be made by its manufacturer, is not guaranteed or endorsed by the publisher.
Abugubba, M. A., Gaboua, N. M., Elganimi, T. Y., and Rabie, K. M. (2022). “CNN-based hybrid precoding design with geometric mean decomposition,” in IEEE vehicular technology conference. doi:10.1109/VTC2022-Fall57202.2022.10013036
Ali K, S., Khan, A. A., Ur Rehman, A., and Ouahada, K. (2023). Learned-SBL-GAMP based hybrid precoders/combiners in millimeter wave massive MIMO systems. PLoS One 18 (9), e0289868. doi:10.1371/journal.pone.0289868
Ammar, H. A., Adve, R., Shahbazpanahi, S., Boudreau, G., and Srinivas, K. V. (2021). User-centric cell-free massive MIMO networks: a survey of opportunities, challenges and solutions. IEEE Commun. Surv. Tutorials 24 (1), 611–652. doi:10.1109/comst.2021.3135119
Apiyo, A., and Izydorczyk, J. (2024). A survey of NOMA-aided cell-free massive MIMO systems. Electronics 13 (1), 231. doi:10.3390/electronics13010231
Biswas, S., and Vijayakumar, P. (2021). “AP selection in cell-free massive MIMO system using machine learning algorithm,” in 2021 sixth international conference on wireless communications, signal processing and networking (WiSPNET) (IEEE).
Elbir, A. M. (2019). CNN-based precoder and combiner design in mmWave MIMO systems. IEEE Commun. Lett. 23 (7), 1240–1243. doi:10.1109/LCOMM.2019.2915977
Gao, X., Dai, L., Sun, Y., Han, S., and Chih-Lin, I. (2017). “Machine learning inspired energy-efficient hybrid precoding for mmWave massive MIMO systems,” in 2017 IEEE international conference on communications (ICC) (IEEE), 1–6.
Gkonis, P. K. (2023). A survey on machine learning techniques for massive MIMO configurations: application areas, performance limitations and future challenges. IEEE Access 11 (January), 67–88. doi:10.1109/ACCESS.2022.3232855
Gnanaprakash, V., and Perarasi, T. (2023). “Performance analysis of deep learning based hybrid precoder/combiner in millimeter wave massive MIMO architecture,” in 2023 international conference on evolutionary algorithms and soft computing techniques (EASCT) (IEEE), 1–5.
Hojatian, H., Nadal, J., Frigon, J.-F., and Leduc-Primeau, F. (2021). Unsupervised deep learning for massive MIMO hybrid beamforming. IEEE Trans. Wirel. Commun. 20 (11), 7086–7099. doi:10.1109/twc.2021.3080672
Khan, L. U., Yaqoob, I., Imran, M., Han, Z., and Hong, C. S. (2020). 6G wireless systems: a vision, architectural elements, and future directions. IEEE access 8, 147029–147044. doi:10.1109/access.2020.3015289
Liu, F., Zhang, L., Yang, X., Li, T., and Du, R. (2023). DL-based energy-efficient hybrid precoding for mmWave massive MIMO systems. IEEE Trans. Veh. Technol. 72 (5), 6103–6112. doi:10.1109/TVT.2022.3230931
Mohammadi, M., Vu, T. T., Ngo, H. Q., and Matthaiou, M. (2023). Network-assisted full-duplex cell-free massive MIMO: spectral and energy efficiencies. IEEE J. Sel. Areas Commun. 41 (9), 2833–2851. doi:10.1109/JSAC.2023.3287613
Ng, G. K., and Raju, M. N. (2024). Design and optimization of hybrid precoders in massive MIMO systems: leveraging low-resolution ADCs/DACs, reconfigurable intelligent surfaces, and deep learning algorithms. Int. J. Intell. Eng. Syst. 17 (1). doi:10.22266/ijies2024.0229.63
Nguyen, H. V., Nguyen, V. D., Dobre, O. A., Sharma, S. K., Chatzinotas, S., Ottersten, B., et al. (2020). On the spectral and energy efficiencies of full-duplex cell-free massive MIMO. IEEE J. Sel. Areas Commun. 38 (8), 1698–1718. doi:10.1109/JSAC.2020.3000810
Ozen, H., and Guvensen, G. M. (2023). Interference and beam squint aware TTD-aided beamforming for dual wideband massive MIMO. IEEE Wirel. Commun. Lett. doi:10.1109/LWC.2023.3319575
Ozgur Gurbuz, A. O. (2021). Akhtar saeed, “special issue terahertz communications,”. Int. Telecommun. Union 2 (7), 1–4.
Rajatheva, N., and Latva-Aho, M. (2021). Deep learning for cell-free massive MIMO networks. 6 (4), 1–7. arXiv.2102.10366v1. doi:10.48550/arXiv.2102.10366
Rekkas, V. P., Sotiroudis, S., Sarigiannidis, P., Wan, S., Karagiannidis, G. K., and Goudos, S. K. (2021). Machine learning in beyond 5G/6G networks—state-of-the-art and future trends. Electronics 10 (22), 2786. doi:10.3390/electronics10222786
Jayarin, P. J. S. R, and Sekar, A. C. (2023). “Effective hybrid precoder logic with mm wave massive MIMO using novel deep learning scheme,” in 2023 eighth international conference on science technology engineering and mathematics (ICONSTEM), 1–8. doi:10.1109/ICONSTEM56934.2023.10142733
Sun, M., Wu, S., Wang, H., and Yang, S. (2023). “Adaptive massive MIMO hybrid precoding based on meta learning,” in 2023 international conference on wireless communications and signal processing (WCSP), 189–194. doi:10.1109/WCSP58612.2023.10404732
Tentu, V., Amudala, D. N., Rajoriya, A., Sharma, E., and Budhiraja, R. (2020). “Energy efficient multi-pair massive MIMO two-way AF relaying: a deep learning approach,” in 2020 international conference on COMmunication systems and NETworkS (COMSNETS) (IEEE), 440–445.
Zhang, Y., Tang, J., Pang, L., Guo, Y., Chen, Y., and Li, J. (2021). Energy efficiency optimization for compact massive MIMO wireless systems. IEEE Trans. Wirel. Technol. 71 (3), 3303–3308. doi:10.1109/tvt.2021.3138802
Zhao, L., Li, J., Huang, S., Wu, X., and Jiang, M. (2024). Low-complexity hybrid precoding for sub-connected millimeter wave massive MIMO systems. Signal Process. 219, 109381. doi:10.1016/j.sigpro.2023.109381
Keywords: cell-free massive MIMO, convolutional neural networks, energy efficiency, hybrid precoding, terahertz communication
Citation: Abose TA, Mekonen YA, Assefa BG and Gudeta NW (2024) Energy efficiency and system complexity analysis of CNN based hybrid precoding for cell-free massive MIMO under terahertz communication. Front. Comms. Net 5:1477270. doi: 10.3389/frcmn.2024.1477270
Received: 07 August 2024; Accepted: 04 November 2024;
Published: 06 December 2024.
Edited by:
Athina Petropulu, Rutgers, The State University of New Jersey - Busch Campus, United StatesReviewed by:
Cheng Wang, Beijing University of Posts and Telecommunications (BUPT), ChinaCopyright © 2024 Abose, Mekonen, Assefa and Gudeta. This is an open-access article distributed under the terms of the Creative Commons Attribution License (CC BY). The use, distribution or reproduction in other forums is permitted, provided the original author(s) and the copyright owner(s) are credited and that the original publication in this journal is cited, in accordance with accepted academic practice. No use, distribution or reproduction is permitted which does not comply with these terms.
*Correspondence: Tadele A. Abose, dGFkZW5lZ25AZ21haWwuY29t; Yitbarek A. Mekonen, eWl0YmFyZWthbmJlc2VAZ21haWwuY29t
Disclaimer: All claims expressed in this article are solely those of the authors and do not necessarily represent those of their affiliated organizations, or those of the publisher, the editors and the reviewers. Any product that may be evaluated in this article or claim that may be made by its manufacturer is not guaranteed or endorsed by the publisher.
Research integrity at Frontiers
Learn more about the work of our research integrity team to safeguard the quality of each article we publish.