- Department of Ports Management and Shipping, National and Kapodistrian University of Athens, Euboea, Greece
The maritime domain is a major driver of economic growth with emerging services, comprising intelligent transportation systems (ITSs), smart ports, security and safety, and ocean monitoring systems. Sixth generation (6G) mobile networks will offer various technologies, paving the way for reliable and autonomous maritime communication networks (MCNs), supporting these novel maritime services. This review presents the main enabling technologies for future MCNs and relevant use cases, including ITSs with reduced carbon footprint, ports and maritime infrastructure security, as well as fault detection and predictive maintenance. Moreover, the current trends in integrated satellite-aerial-terrestrial-maritime network architectures are discussed together with the different network segments and communication technologies, and machine learning integration aspects.
1 Introduction
Maritime activities hold the key for economic development at a global scale. Relevant figures highlight that shipping transports around 90% of goods while the deployment of offshore wind energy has been increasing with Europe alone targeting at least 42.5% renewable energy by 2030, requiring a massive increase in installed wind capacity from 204 GW in 2022 to more than 500 GW in 2030 (Commission, 2024). Currently, the maritime industry, which encompasses shipping and logistics, fishing, offshore energy, tourism and underwater applications, is experiencing unprecedented expansion, as countries across the globe look to exploit their maritime resources and strengthen their trade connections.
In this context, maritime communication networks (MCNs) support services related to maritime transportation, smart ports, ocean monitoring, tourism, as well as search and rescue (SAR) operations (Guan et al., 2021; Haidine et al., 2021). This service mixture is based on heterogeneous networks nodes, including satellites, unmanned aerial vehicles (UAVs), shore base stations (BSs), buoys, platforms, ships, unmanned surface vehicles (USVs), unmanned underwater vehicles (UUVs), sensors, and actuators (Wang et al., 2021). Furthermore, maritime applications have varying QoS requirements, as, for example, services for cruise ships demand broadband connectivity, SAR operations are based on transmitting real-time video, while maritime IoT traffic must adhere to ultra-reliable and ultra-low latency (URLLC) constraints (Alqurashi et al., 2022). Towards this end, novel communications algorithms should satisfy reliability and latency requirements by design (Nomikos et al., 2018). Current maritime networking architectures fall short of supporting the increasing number of vessels, USVs, UUVs, sensors and novel maritime services, due to unreliable connectivity, low data rates, insufficient spectrum, and increased delays. Even though various industrial initiatives have been deployed to offer broadband satellite coverage and long-distance shore-to-vessel communications (Seo et al., 2017; Huo et al., 2020; SpaceX, 2021), further research on flexible and smart MCNs is needed.
Another critical factor for the success of MCNs in the 6G era is their deep integration with machine learning (ML). In maritime environments with geographically dispersed nodes, ML training relies on data collection and processing from distributed sources, experiencing varying propagation conditions. Thus, conventional centralized ML might not be feasible, especially for URLLC services. Likewise, proper data manipulation is needed to mitigate the impact of diverse propagation conditions. Finally, in several cases, training data might include sensitive information, that should be stored locally and not transmitted to centralized premises (Trakadas et al., 2022). Thus, to deal with these issues, decentralized learning solutions have recently emerged to accelerate ML execution times and ensure data gravity (Aledhari et al., 2020; Wahab et al., 2021). Here, federated learning (FL) is a popular solution where maritime nodes exploit shared models, trained from excessive amounts of data, without the need to centrally store it. Moreover, to enable edge maritime nodes to participate in the ML process, over-the-air/coded computing (AirComp)-based schemes have been proposed, supporting communication and computation procedures via the multiple-access channels’ superposition property (Yang et al., 2020a).
As it is evident, the maritime domain will continue growing and the current communication infrastructure cannot cope with future service requirements. In this review, we present the different MCN architecture segments and communication technologies that are currently proposed by academic and industrial stakeholders. Moreover, we discuss enabling 6G technologies for enhanced MCN deployments. In this context, various challenges that must be addressed are highlighted and a discussion on key opportunities for MCNs to support novel maritime use cases is included.
2 Maritime communication networks
MCNs have evolved from topologies mainly supported by satellite constellations and shore BSs to heterogeneous networks, where numerous UAVs UUVs, USVs, sea vessels, buoys, platforms, and sensors cooperate to enable reliable communications in challenging and volatile environments.
2.1 MCN segments
Figure 1 depicts four main segments in the MCN architecture. More specifically, a maritime segment supporting underwater and sea surface activities, a shore segment, an aerial segment and a space segment.
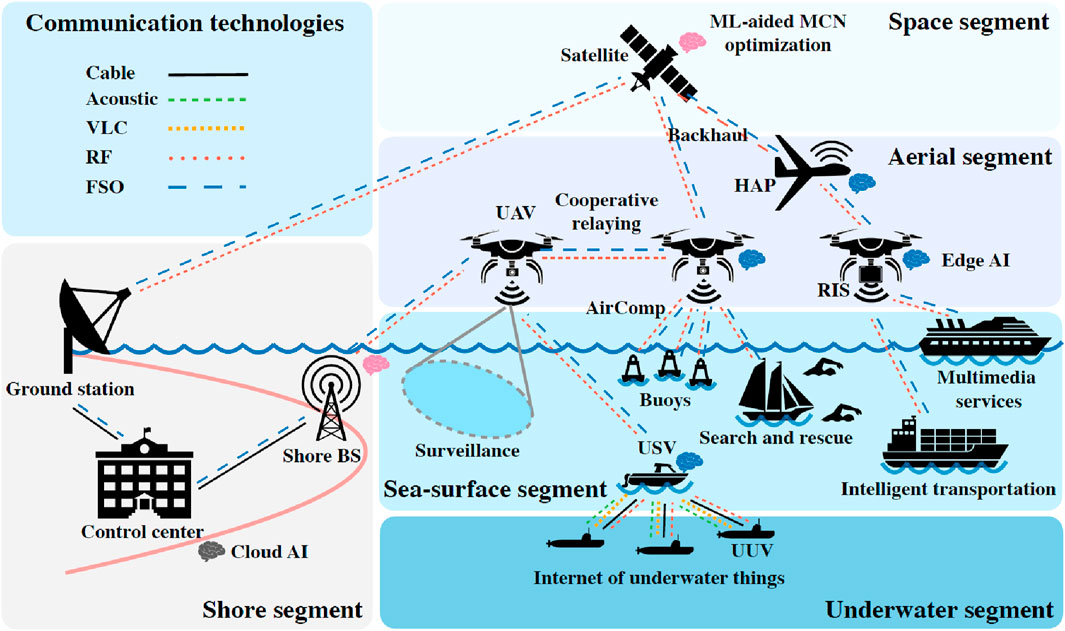
Figure 1. The different segments of a maritime communication network adapted from (Nomikos et al., 2023).
2.1.1 Maritime segment
In this segment, edge MCN nodes are present, allowing for surface and underwater data acquisition, data transmission with multiple communication technologies, and edge computing tasks.
2.1.1.1 Underwater
The underwater segment includes sensors, underwater buoys and UUVs, enabling data acquisition and forwarding to other MCN nodes, such as UUVs, ships or UAVs. Here, electromagnetic waves suffer from high attenuation in seawater when signals propagate over longer distances, and acoustic communications might be preferred.
2.1.1.2 Sea surface
On the sea surface, ships, USVs, and buoys exist, facilitating intelligent maritime transportation, environmental observation, data exchange with the other segments, and maritime SAR. Also, novel networking solutions include giant cells, in the form of seaborne floating towers, in the form of semi-submersible steel reinforced concrete platforms (Guan et al., 2021).
2.1.2 Shore segment
The shore segment mainly host BSs, providing connectivity to nearby maritime nodes and UAVs, using cellular standards. Moreover, ground stations communicating with the space segment exist. Other MCN elements include control centers and cloud infrastructure to enable the processing of more demanding computing tasks.
2.1.3 Aerial segment
Shore BSs and satellites provide extended coverage in MCNs but they might hinder the performance of URLLC or broadband services (Li et al., 2021). Thus, UAV-aided MCNs have emerged, including aerial segments, offering dynamic radio-resource provisioning to remote areas, reduced-latency compared to satellite links and increased reliability (Ait Allal et al., 2022; Nomikos et al., 2023). In addition to UAVs, the aerial segment can employ high-altitude platforms (HAPs) at the stratosphere.
2.1.4 Space segment
The space segment hosts various satellite systems, such as geostationary earth orbit (GEO)-based INMARSAT and low-earth orbit (LEO) constellations, such as Starlink. Apart from wide coverage across MCNs, this segment is also employed as a back-up when shore BSs and UAVs/HAPs fail to provide coverage to MCN nodes.
2.2 Communication technologies
A number of different communication technologies are employed in MCNs to adjust to varying environmental and propagation conditions. This heterogeneous networking paradigm should seamlessly exploit the capabilities of its different segments, in terms of radio- and computing resources to go beyond current 5G capabilities, also offering in-network cloud and edge AI.
2.2.1 Radio frequency (RF) communications
In traditional maritime communication systems, RF transmissions operate on the very high frequency (VHF) band between 156 and 174 MHz, offering radio services and SAR support. In 6G, the use of the sub-6 GHz (Frequency Range 1–FR1) band will be adopted, following its use in terrestrial deployments. Moreover, other spectrum bands, employed in terrestrial networks will be considered, including the millimeter wave band (FR2) between 24.25 GHz and 52.6 GHz and the upper-mid band (FR3) between 7.125 and 24.25 GHz, providing broadband communication and highly complementary radio characteristics among the three bands.
2.2.2 Free space optical (FSO) communications
The desire for high-throughput services, low-cost set-up and operation, increased security, and license-free use has motivated researchers to introduce free space optical (FSO) communication, under LoS conditions (Kaushal and Kaddoum, 2017). On the downside, FSO transmissions are affected by atmospheric effects, i.e., absorption, scattering, and turbulence (Lionis et al., 2021).
2.2.3 Visible-light communications (VLC)
Another attractive MCN technology is VLC, using light-emitting diodes (LEDs) for data transmission, avoiding impairments of the RF band, such as interference and signal leakage. In maritime environments, VLC is especially useful in underwater communications (Huang et al., 2020).
2.2.4 Acoustic communications
In underwater communications, long-range transmission can be achieved through acoustic waves. In various works on underwater channel characteristics, the main challenges include poor quality and highly dynamic nature of acoustic channels, smaller channel capacity over RF channels, multi-path-rich environment, and larger propagation delay (Bouvet and Auffret, 2020; Cao et al., 2021).
3 Enabling 6G technologies and key challenges
This section provides an overview of enabling technologies for 6G MCNs.
3.1 Cooperative communications with relays and RISs
The use of cooperative communications is critical in enhancing MCNs, as the heterogeneous network nodes should work in tandem to satisfy service requirements, also exploiting all the available duplexing modes of the relays (Nomikos et al., 2014). Nonetheless, the development of robust cooperative algorithms, exploiting the heterogeneous MCN segments requires that coordination and signalling overheads will be taken into consideration. Cooperative communications can support high data rates, URLLC, and enhanced security, which are essential for 6G MCNs. Here, UAVs play a significant role by serving as BSs or relays, offering coverage extension and improving wireless connectivity for nodes positioned on or below the sea surface. In greater detail, UAVs can provide multi-hop transmissions to other UAVs, and establish connectivity between underwater sensor nodes, sea surface sink nodes, and shore BSs. Recent studies highlight the advantages of UAV-assisted communications in maritime contexts. For instance, the work in (Chen et al., 2020) demonstrated that UAVs, acting as relay nodes, can significantly enhance the minimum throughput of ocean surface drifting buoys. Another study, (Nomikos et al., 2024), explored the role of opportunistic relaying with UAV swarms in MCNs, revealing that deployments with multiple UAVs can better support MCN services, compared to conventional communication with shore BSs. Furthermore, in integrated satellite-aerial-maritime communication networks, low earth orbit (LEO) satellites can cooperate with UAVs to provide reliable backhauling and increased data rates without significantly degrading the latency performance. This ensures the robust support of heterogeneous services, including augmented and virtual reality, and telemedicine. In this way, data can reach other parts of the MCN or shore-based infrastructure to support cloud and edge AI services. Another technology with tremendous potential in cooperative communications settings is reflecting intelligent surfaces (RIS). RISs consist of multiple antenna patches that actively or passively control the redirection of electromagnetic waves incident on them, adapting their phase and amplitude to enhance signal strength and reduce fading effects (Ramezani et al., 2022). Integrating RISs with UAVs and satellites enhances data rates and reliability, efficiently supporting IoT and smart shipping applications. The main challenge in this area corresponds to the development of robust cooperative algorithms with minimized coordination and signaling overheads.
3.2 Machine learning-aided MCN optimization
In the majority of related works on ML-aided MCN optimization and performance evaluation, simple network topologies have been considered (i.e., with reduced number of UAVs, USVs, UUVs, etc.) in order to reduce the computational complexity of the proposed optimization approaches. To address the complexity of practical scenarios, advanced ML techniques must be employed to handle large-scale deployments and the diverse requirements of IoT devices, UAVs, and vessels. Likewise, how to jointly address different sub-problems of MCNs, such as data relaying, end-to-end communication among satellites/UAVs/USVs/UUVs, and edge AI maritime service requirements have not been investigated. In such large-scale deployments, employing a realistic number of active IoT devices, UAVs and vessels, efficient ML-aided MCN optimization is necessary. Thus, as a result of the multi-parameter MCN aspects, advanced ML algorithms, such as DRL can be very efficient, due to their ability to adapt to various network conditions and provide appropriate modifications (Lei et al., 2021). Another important enabler for 6G MCNs is distributed ML optimization, since MCN operation is threatened by excessive communication and energy costs, as well as latency and privacy requirements that cannot be supported by centralized ML. Towards this end, transfer learning and FL correspond to promising solutions to address these issues (Qu et al., 2021), especially in edge AI scenarios. Transfer learning initially extracts features, that, for example, in content caching scenarios can correspond to file popularity on a base network, relying on a generalized dataset. Then, these features are leveraged by DRL agents on edge UAVs to derive the optimal caching policy, thus reducing their energy consumption. Moreover, FL exploits observations from multiple DRL agents at different edge nodes to train a shared model. The main advantage of FL in MCNs is communication cost reduction, as model updates are only computed to update the global shared model at a coordinating node and explicit data sharing is avoided. In this manner, privacy is ensured, since sensitive information remains at the edge nodes. Here, the main challenges are related to the computational complexity of ML optimization for large-scale deployments, ensuring data privacy in distributed ML frameworks.
3.3 Edge AI with over-the-air computation
The vessel-to-shore communications in an MCN using cellular standards involves multiple transceivers mounted on heterogeneous nodes that require simultaneous spectrum access. The propagation conditions of maritime wireless channels often experience severe signal degradation, resulting in an increased rate of transmission errors and underutilized channels. Although the increase of the transmission power in these channels minimizes the errors and the frequency of re-transmission, it further compromises the spectrum efficiency of the overall system due to co-channel interference, while also escalating the energy demands of the nodes (Wang et al., 2018). To this end, multiple access techniques that provide enhanced spectrum utilization and energy efficiency are expected to play a vital role in the 6G-enabled MCN architecture that consist of multiple heterogeneous nodes (Zetas et al., 2024). In this context, Over-the-Air Computation (AirComp) emerges as the most promising technique for integrated 6G networks within a heterogeneous IoT ecosystem, optimizing both energy efficiency and spectrum utilization (Liu et al., 2020). AirComp leverages the temporal superposition of multiple signals from diverse sources, enhancing communication efficiency and data privacy. In an AirComp system, communication nodes within the network transmit their data simultaneously over a common Multiple Access Channel (MAC). The pre-processed signals from all transmitters are transmitted through the individual channels and superposed in the receiver in the time domain. The receiver gathers the aggregated signal (each individual signal is scaled by the transmission power and the channel state information) and post-processes it using a nomographic function (Yang et al., 2020b; Tsinos et al., 2023). Therefore, AirComp encompasses both spectrum and energy efficiency principles, since it is based on non-orthogonal multiple access techniques and the transmission power of the nodes are optimized to comply with strict power budget limitations (Cao et al., 2020). In conclusion, AirComp can be useful for environmental monitoring, sustainability, and advanced maritime services such as real-time data analysis and emergency response. Still, managing signal degradation in maritime channels and optimizing power allocation to reduce co-channel interference remains an open issue.
4 Discussion
In this section, the role of MCNs in important maritime service domains are discussed.
4.1 Sustainable maritime transportation and supply chain
Sustainable maritime transportation is a critical aspect of addressing global environmental challenges. The sector has been increasingly adopting innovative technologies to reduce its carbon footprint and enhance operational efficiency (Giannopoulos et al., 2023). In this domain, efficient FL operation towards reduced green house gas emissions and optimized supply chain operation must be supported. FL facilitates the training of ML models across multiple decentralized devices or servers without requiring the exchange of raw data (Skianis et al., 2023). In the maritime sector, FL is particularly useful for optimizing routes, predicting maintenance needs, and enhancing fuel efficiency while ensuring data privacy and security (Giannopoulos et al., 2024). By leveraging data from various ships and ports, FL enables the development of robust predictive models that can significantly reduce greenhouse gas emissions. Studies have shown that FL can effectively enhance the performance of predictive maintenance systems and optimize fuel consumption, contributing to more sustainable operations (Zhang et al., 2021; Angelopoulos et al., 2024). Additionally, digital twin (DT) technologies can be useful for investigating how adjustments to MCNs can impact their performance and improving maritime services. DTs create virtual replicas of physical assets, allowing real-time monitoring and predictive maintenance. In maritime transportation, DTs can simulate and predict ship performance under various conditions, optimizing routes and improving fuel efficiency, thereby reducing emissions (Madusanka et al., 2023).
4.2 Fault detection and predictive maintenance
The maritime industry currently employs two main maintenance strategies, namely, reactive and preventive maintenance. Reactive maintenance entails the direct response to machinery failures, leading to costly unplanned downtime events (Swanson, 2001). In contrast, preventive maintenance, is a schedule-based intervention strategy, relying on manufacturer-defined projections of component usage, aiming to minimize unexpected downtime events. However, despite its goal to minimize the non-operational vessel periods, it may still result in significant costs due to unnecessary and excessive repairs (Basri et al., 2017).
The introduction of predictive maintenance offers maritime organizations a proactive approach to identify potential machinery issues (Kalafatelis et al., 2023). Extensive research in the literature focuses on real-time failure detection and proactive prediction for machinery maintenance in maritime environments (Berghout et al., 2021; Gribbestad et al., 2021; Liu et al., 2022). However, real-world applications of predictive maintenance in maritime settings are heavily relied on effective MCNs, as vessels often operate in remote areas, far from terrestrial communication infrastructures, necessitating global coverage. Aligned with the Shipping 4.0 paradigm, predictive maintenance requires continuous monitoring of key vessel components, enabling maritime organizations, such as vessel owners or operators to perform accurate and timely interventions, preventing costly breakdowns and enhancing operational efficiency. MCNs are crucial the secure transmission of real-time data supporting centralized aggregations to onshore centers as well as decentralized workflows such as FL, including, peer-to-peer, hierarchical, asynchronous, and cross-silo communication models (Han and Yang, 2021; Giannopoulos et al., 2024).
4.3 Increased security of ports and maritime infrastructure
In order to guarantee uninterrupted maritime activities, MCNs must provide reliable networking towards open and secure seas, allowing free trade, transport, energy security and tourism by protecting maritime infrastructure, offshore wind farms, and sea harbours (Bueger and Liebetrau, 2023). Nonetheless, various security challenges, stemming from advanced threats in the sea surface and underwater domain have been identified, including, sabotages, terrorist attacks, drug trafficking and smuggling. In this area, MCNs must support reliable operation of remote inspection and patrolling procedures. For example, ship hull anomaly detection is required for vessel screening in port areas, often in regions where the volume of shipping is large. These hull inspections are typically executed with divers, or in some cases ROVs, using manual visual inspection methods for navigation, imaging and assessment. These manual processes are time-consuming and are often not very robust, due to the turbidity of the operational environments (Lin and Dong, 2023). MCNs can enable automated inspection by ensuring timely data collection and computations which in-turn reduces the time to perform underwater inspections.
5 Conclusion
This review presented important communication and networking technologies that will drive the development of 6G MCNs. Also, various MCN-related challenges for providing highly demanding and distributed maritime services were identified. Finally, key domains where MCNs have the opportunity to thrive, including maritime transportation and supply chains with reduced carbon footprint, ports and maritime infrastructure security, and fault detection and predictive maintenance were discussed. Overall, the potential of MCNs to accelerate the growth of the maritime domain is tremendous and exciting opportunities arise for interested stakeholders to develop 6G innovations.
Author contributions
GX: Conceptualization, Investigation, Writing–original draft, Writing–review and editing. NN: Conceptualization, Investigation, Project administration, Writing–original draft, Writing–review and editing. AK: Investigation, Writing–original draft, Writing–review and editing. AG: Investigation, Writing–original draft, Writing–review and editing. SS: Investigation, Writing–original draft, Writing–review and editing. PT: Resources, Supervision, Writing–original draft, Writing–review and editing.
Funding
The author(s) declare that no financial support was received for the research, authorship, and/or publication of this article.
Conflict of interest
The authors declare that the research was conducted in the absence of any commercial or financial relationships that could be construed as a potential conflict of interest.
The author(s) declared that they were an editorial board member of Frontiers, at the time of submission. This had no impact on the peer review process and the final decision.
Publisher’s note
All claims expressed in this article are solely those of the authors and do not necessarily represent those of their affiliated organizations, or those of the publisher, the editors and the reviewers. Any product that may be evaluated in this article, or claim that may be made by its manufacturer, is not guaranteed or endorsed by the publisher.
References
Ait Allal, A., Amrani, L. E., Haidine, A., Mansouri, K., and Youssfi, M. (2022). “Towards deployment of UAV’s for autonomous ships’ 5G mobile communication,” in Advanced intelligent systems for sustainable development. Editors J. Kacprzyk, V. E. Balas, and M. Ezziyyani AI2SD’2020 (Cham: Springer International Publishing), 944–956.
Aledhari, M., Razzak, R., Parizi, R. M., and Saeed, F. (2020). Federated learning: a survey on enabling technologies, protocols, and applications. IEEE Access 8, 140699–140725. doi:10.1109/ACCESS.2020.3013541
Alqurashi, F. S., Trichili, A., Saeed, N., Ooi, B. S., and Alouini, M.-S. (2022). Maritime communications: a survey on enabling technologies, opportunities, and challenges. IEEE Internet Things J. 10, 3525–3547. doi:10.1109/JIOT.2022.3219674
Angelopoulos, A., Giannopoulos, A., Nomikos, N., Kalafatelis, A., Hatziefremidis, A., and Trakadas, P. (2024). Federated learning-aided prognostics in the shipping 4.0: principles, workflow, and use cases. IEEE Access 12, 6437–6454. doi:10.1109/access.2024.3350777
Basri, E. I., Razak, I. H. A., Ab-Samat, H., and Kamaruddin, S. (2017). Preventive maintenance (pm) planning: a review. J. Qual. maintenance Eng. 23, 114–143. doi:10.1108/JQME-04-2016-0014
Berghout, T., Mouss, L.-H., Bentrcia, T., Elbouchikhi, E., and Benbouzid, M. (2021). A deep supervised learning approach for condition-based maintenance of naval propulsion systems. Ocean. Eng. 221, 108525. doi:10.1016/j.oceaneng.2020.108525
Bouvet, P.-J., and Auffret, Y. (2020). On the achievable rate of multiple-input–multiple-output underwater acoustic communications. IEEE J. Ocean. Eng. 45, 1126–1137. doi:10.1109/JOE.2019.2916120
Bueger, C., and Liebetrau, T. (2023). Critical maritime infrastructure protection: what’s the trouble? Mar. Policy 155, 105772. doi:10.1016/j.marpol.2023.105772
Cao, X., Zhu, G., Xu, J., and Huang, K. (2020). Optimized power control for over-the-air computation in fading channels. IEEE Trans. Wirel. Commun. 19, 7498–7513. doi:10.1109/twc.2020.3012287
Cao, Y., Shi, W., Sun, L., and Fu, X. (2021). Channel state information-based ranging for underwater acoustic sensor networks. IEEE Trans. Wirel. Commun. 20, 1293–1307. doi:10.1109/TWC.2020.3032589
Chen, H., Yin, F., Huang, W., Liu, M., and Li, D. (2020). Ocean surface drifting buoy system based on UAV-enabled wireless powered relay network. Sensors 20, 2598. doi:10.3390/s20092598
Commission, E. (2024). Commission sets out immediate actions to support the european wind power industry.
Giannopoulos, A., Gkonis, P., Bithas, P., Nomikos, N., Kalafatelis, A., and Trakadas, P. (2024). Federated learning for maritime environments: use cases, experimental results, and open issues. J. Mar. Sci. Eng. 12 (6), 1034. doi:10.3390/jmse12061034
Giannopoulos, A., Nomikos, N., Ntroulias, G., Syriopoulos, T., and Trakadas, P. (2023). “Maritime federated learning for decentralized on-ship intelligence,” in IFIP international conference on artificial intelligence applications and innovations (Springer), 195–206.
Gribbestad, M., Hassan, M. U., and Hameed, I. A. (2021). Transfer learning for prognostics and health management (phm) of marine air compressors. J. Mar. Sci. Eng. 9, 47. doi:10.3390/jmse9010047
Guan, S., Wang, J., Jiang, C., Duan, R., Ren, Y., and Quek, T. Q. S. (2021). MagicNet: the maritime giant cellular network. IEEE Commun. Mag. 59, 117–123. doi:10.1109/MCOM.001.2000831
Haidine, A., Aqqal, A., and Dahbi, A. (2021). “Communications backbone for environment monitoring applications in smart maritime ports-case study of a moroccan port,” in 2021 IEEE asia-pacific conference on geoscience, electronics and remote sensing technology (AGERS), 136–140. doi:10.1109/AGERS53903.2021.9617440
Han, C., and Yang, T. (2021). “Privacy protection technology of maritime multi-agent communication based on part-federated learning,” in 2021 IEEE/CIC international conference on communications in China ICCC workshops (IEEE), 266–271.
Huang, G., Zhang, L., Jiang, Y., and Wu, Z. (2020). A general orthogonal transform aided MIMO design for reliable maritime visible light communications. J. Light. Technol. 38, 6549–6560. doi:10.1109/JLT.2020.3016662
Huo, Y., Dong, X., and Beatty, S. (2020). Cellular communications in ocean waves for maritime internet of things. IEEE Internet Things J. 7, 9965–9979. doi:10.1109/JIOT.2020.2988634
Kalafatelis, A. S., Nomikos, N., Angelopoulos, A., Trochoutsos, C., and Trakadas, P. (2023). “An effective methodology for imbalanced data handling in predictive maintenance for offset printing,” in International conference on mechatronics and control engineering (Springer), 89–98.
Kaushal, H., and Kaddoum, G. (2017). Optical communication in space: challenges and mitigation techniques. IEEE Commun. Surv. Tutorials 19, 57–96. doi:10.1109/COMST.2016.2603518
Lei, L., Shen, G., Zhang, L., and Li, Z. (2021). Toward intelligent cooperation of UAV swarms: when machine learning meets digital twin. IEEE Netw. 35, 386–392. doi:10.1109/MNET.011.2000388
Li, Y., Huang, J., Sun, Q., Sun, T., and Wang, S. (2021). Cognitive service architecture for 6G core network. IEEE Trans. Industrial Inf. 17, 7193–7203. doi:10.1109/TII.2021.3063697
Lin, B., and Dong, X. (2023). Ship hull inspection: a survey. Ocean. Eng. 289, 116281. doi:10.1016/j.oceaneng.2023.116281
Lionis, A., Peppas, K., Nistazakis, H. E., Tsigopoulos, A., and Cohn, K. (2021). Statistical modeling of received signal strength for an FSO link over maritime environment. Opt. Commun. 489, 126858. doi:10.1016/j.optcom.2021.126858
Liu, B., Gan, H., Chen, D., and Shu, Z. (2022). Research on fault early warning of marine diesel engine based on cnn-bigru. J. Mar. Sci. Eng. 11, 56. doi:10.3390/jmse11010056
Liu, W., Zang, X., Li, Y., and Vucetic, B. (2020). Over-the-air computation systems: optimization, analysis and scaling laws. IEEE Trans. Wirel. Commun. 19, 5488–5502. doi:10.1109/twc.2020.2993703
Madusanka, N. S., Fan, Y., Yang, S., and Xiang, X. (2023). Digital twin in the maritime domain: a review and emerging trends. J. Mar. Sci. Eng. 11, 1021. doi:10.3390/jmse11051021
Nomikos, N., Charalambous, T., Krikidis, I., Vouyioukas, D., and Johansson, M. (2014). “Hybrid cooperation through full-duplex opportunistic relaying and max-link relay selection with transmit power adaptation,” in 2014 IEEE international conference on communications (ICC), 5706–5711. doi:10.1109/ICC.2014.6884231
Nomikos, N., Giannopoulos, A., Kalafatelis, A., Özduran, V., Trakadas, P., and Karagiannidis, G. K. (2024). Improving connectivity in 6g maritime communication networks with uav swarms. IEEE Access 12, 18739–18751. doi:10.1109/access.2024.3360133
Nomikos, N., Gkonis, P. K., Bithas, P. S., and Trakadas, P. (2023). A survey on uav-aided maritime communications: deployment considerations, applications, and future challenges. IEEE Open J. Commun. Soc. 4, 56–78. doi:10.1109/OJCOMS.2022.3225590
Nomikos, N., Poulimeneas, D., Charalambous, T., Krikidis, I., Vouyioukas, D., and Johansson, M. (2018). Delay- and diversity-aware buffer-aided relay selection policies in cooperative networks. IEEE Access 6, 73531–73547. doi:10.1109/access.2018.2883894
Qu, Y., Dong, C., Zheng, J., Dai, H., Wu, F., Guo, S., et al. (2021). Empowering edge intelligence by air-ground integrated federated learning. IEEE Netw. 35, 34–41. doi:10.1109/MNET.111.2100044
Ramezani, P., Lyu, B., and Jamalipour, A. (2022). Toward RIS-enhanced integrated terrestrial/non-terrestrial connectivity in 6G. IEEE Netw. 37, 178–185. doi:10.1109/MNET.116.2200060
Seo, H., Shim, J., Ha, S., Kim, Y.-S., and Jeong, J. (2017). “Ultra long range LTE ocean coverage solution,” in 2017 24th international conference on telecommunications (ICT), 1–5. doi:10.1109/ICT.2017.7998246
Skianis, K., Giannopoulos, A., Gkonis, P., and Trakadas, P. (2023). Data aging matters: federated learning-based consumption prediction in smart homes via age-based model weighting. Electronics 12, 3054. doi:10.3390/electronics12143054
Swanson, L. (2001). Linking maintenance strategies to performance. Int. J. Prod. Econ. 70, 237–244. doi:10.1016/S0925-5273(00)00067-0
Trakadas, P., Masip-Bruin, X., Facca, F. M., Spantideas, S. T., Giannopoulos, A. E., Kapsalis, N. C., et al. (2022). A reference architecture for cloud–edge meta-operating systems enabling cross-domain, data-intensive, ml-assisted applications: architectural overview and key concepts. Sensors 22, 9003. doi:10.3390/s22229003
Tsinos, C., Spantideas, S., Giannopoulos, A., and Trakadas, P. (2023). Over-the-air computation with quantized csi and discrete power control levels. Wirel. Commun. Mob. Comput. 2023, 1–8. doi:10.1155/2023/8559701
Wahab, O. A., Mourad, A., Otrok, H., and Taleb, T. (2021). Federated machine learning: survey, multi-level classification, desirable criteria and future directions in communication and networking systems. IEEE Commun. Surv. Tutorials 23, 1342–1397. doi:10.1109/COMST.2021.3058573
Wang, J., Zhou, H., Li, Y., Sun, Q., Wu, Y., Jin, S., et al. (2018). Wireless channel models for maritime communications. IEEE Access 6, 68070–68088. doi:10.1109/ACCESS.2018.2879902
Wang, Y., Feng, W., Wang, J., and Quek, T. Q. S. (2021). Hybrid satellite-UAV-terrestrial networks for 6G ubiquitous coverage: a maritime communications perspective. IEEE J. Sel. Areas Commun. 39, 3475–3490. doi:10.1109/JSAC.2021.3088692
Yang, K., Jiang, T., Shi, Y., and Ding, Z. (2020a). Federated learning via over-the-air computation. IEEE Trans. Wirel. Commun. 19, 2022–2035. doi:10.1109/TWC.2019.2961673
Yang, K., Jiang, T., Shi, Y., and Ding, Z. (2020b). Federated learning via over-the-air computation. IEEE Trans. Wirel. Commun. 19, 2022–2035. doi:10.1109/twc.2019.2961673
Zetas, M., Spantideas, S., Giannopoulos, A., Nomikos, N., and Trakadas, P. (2024). Empowering 6g maritime communications with distributed intelligence and over-the-air model sharing. Front. Commun. Netw. 4, 1280602. doi:10.3389/frcmn.2023.1280602
Keywords: 6G, cooperative networks, maritime communication networks, machine learning, unmanned aerial vehicle (UAV), wireless networks
Citation: Xylouris G, Nomikos N, Kalafatelis A, Giannopoulos A, Spantideas S and Trakadas P (2024) Sailing into the future: technologies, challenges, and opportunities for maritime communication networks in the 6G era. Front. Comms. Net 5:1439529. doi: 10.3389/frcmn.2024.1439529
Received: 28 May 2024; Accepted: 25 June 2024;
Published: 24 July 2024.
Edited by:
Phil Yeoh, University of the Sunshine Coast, AustraliaReviewed by:
Gerald Ijemaru, University of the Sunshine Coast, AustraliaCopyright © 2024 Xylouris, Nomikos, Kalafatelis, Giannopoulos, Spantideas and Trakadas. This is an open-access article distributed under the terms of the Creative Commons Attribution License (CC BY). The use, distribution or reproduction in other forums is permitted, provided the original author(s) and the copyright owner(s) are credited and that the original publication in this journal is cited, in accordance with accepted academic practice. No use, distribution or reproduction is permitted which does not comply with these terms.
*Correspondence: Nikolaos Nomikos, bm9taWtvc25AcG1zLnVvYS5ncg==