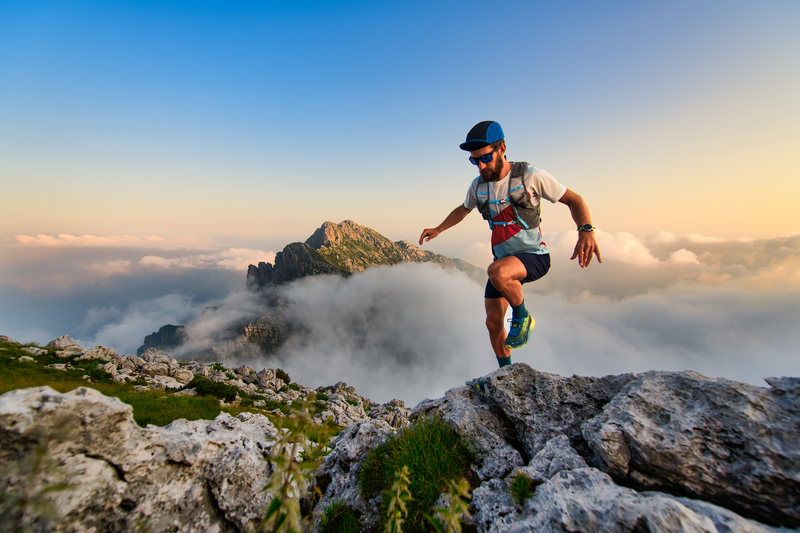
94% of researchers rate our articles as excellent or good
Learn more about the work of our research integrity team to safeguard the quality of each article we publish.
Find out more
REVIEW article
Front. Commun. Netw. , 07 October 2024
Sec. Aerial and Space Networks
Volume 5 - 2024 | https://doi.org/10.3389/frcmn.2024.1286073
This article is part of the Research Topic An Aerial and Space Networks Perspective on SDG 9: Industry, Innovation and Infrastructure View all 4 articles
Unmanned Aerial Vehicles (UAVs), commonly known as drones, show great potential in advancing the United Nations (UN) Sustainable Development Goals (SDGs), a collection of 17 worldwide targets outlined by the United Nations (UN) to tackle diverse social, economic, and environmental issues and foster sustainable development by 2030. As per the Federal Aviation Administration (FAA), UAVs can significantly improve areas of sustainability like wildlife and wildfire monitoring, precision agriculture, as well as healthcare. Furthermore, drones with communication capabilities are seen as key elements of non-terrestrial networks, and as enabling technologies for future 6G communication networks. This systematic literature review focuses on the role of UAV-enabled communications to achieve the SDGs. Our study is based on a thorough selection and analysis of scientific articles. Initially, we performed a broad search across three major databases: IEEE Xplore, Web of Science, and Scopus, yielding a set of 10,499 papers. Following a strict selection methodology (Preferred Reporting Items for Systematic Review and Meta-Analysis - PRISMA), we narrowed this set down to 28 key journal articles. Our analysis highlights six global targets that greatly benefit from UAV-enabled aerial communication. In order of impact, these are: “Sustainable Cities and Communities,” where UAVs improve security and disaster management; “Life on Land,” where drones help with wildlife monitoring and reforestation; “Industry, Innovation, and Infrastructure,” where UAVs (Unmanned Aerial Vehicles) aid in infrastructure inspection; “No Hunger,” where drones improve agricultural practices; “‘Good Health and Wellbeing,” where UAVs assist with disease prediction and monitoring; and “Clean water and sanitation,”where drones play a crucial role in efficiently monitoring water quality and distribution. In summary, this paper offers a detailed look at the applications of UAVs in achieving the SDGs through aerial communications. By identifying areas of major impact and spotting gaps and weaknesses in current research, this review article aims to guide future research and policy-making in sustainable drone applications.
Keeping economic growth without compromising the environment’s wellbeing is the essence of long-term sustainability Policy and Staff (1990). Yet, sustainability encompasses more than preserving nature; it implies fostering a harmonious coexistence between businesses, government entities, and society. Sustainable Development Goals (SDGs), refer to a set of 17 global objectives established by the United Nations (UN) in September 2015. Part of the 2030 Agenda for Sustainable Development, these goals tackle social, economic, and environmental challenges worldwide. The objectives aim to promote prosperity, protect the planet, and ensure universal wellbeing, designed inclusively for both developed and developing countries. Adopted by all 193 UN member states, the SDGs form a comprehensive framework for global sustainable development (United Nations Development Programme, 2023). These SDGs are built upon five core pillars: people, planet, prosperity, peace, and partnership. Each pillar embodies multiple goals, reflecting distinct sectors. A summary of the 17 SDGs is provided in Table 1.
Table 1. A brief overview of the 17 SDGs (Nations, 2018).
In this global journey, Goal 9 plays a pivotal role, with around 70% of the world’s SDGs targets potentially achieved through advancements aligned with this Goal (World Economic Forum, 2020). One of the cornerstones of innovative infrastructures is the telecommunications sector, underlining its significance in propelling sustainable development (European Commission Decision C, 2023). However, achieving the many targets set by the SDGs requires not just established technologies but also innovative solutions for communication, infrastructure, and inclusion.
Within this context, the emergence of non-terrestrial networks (Geraci et al., 2022; Azari et al., 2022) becomes notably significant. These networks, relying on aerial communication technologies, are proving to be catalysts in shaping the future of telecommunications (Javaid et al., 2023; Akyildiz et al., 2020; Tataria et al., 2021). Moving beyond conventional limitations, these networks offer ingenious solutions to some of the most pressing global sustainability challenges. By extending the network coverage, and by interconnecting devices seamlessly and flexibly (Vinogradov et al., 2018; Akhtar and Saeed, 2023; Giordani and Zorzi, 2021), they have the potential to not only transform industries and economies but also contribute significantly to the realization of sustainable development goals. Hence, this study embarks on a systematic literature review to unveil how aerial communication technology and non-terrestrial networks contribute to achieving sustainable development.
There have been some earlier studies in the general area of this topic. For instance, (Wu et al., 2018), outlined the important initiatives and research activities while introducing the history of sustainable development. Additionally, the authors looked into how Information and Communications Technologies (ICTs) can help achieve the SDGs. Following thorough reviews of the literature on SDG-related research initiatives and activities, they have concluded that the vast majority of contributions to SDGs have mainly focused on the technical aspects, lacking comprehensive social good perspectives. Furthermore, the same paper only took into account research acknowledged by the IEEE and ACM communities during 2015 and 2016, which cannot represent all studies available. The promise of Internet of Things (IoT) with integrated sensing and communication capabilities for effective, sustainable, and knowledgeable resource management in urban and rural areas was demonstrated in a study by Abdul Salam (Abdul, 2020). This study explored the fundamental ideas of sustainable community development with a focus on communication technologies and SDGs. Nevertheless, the work was limited to the linkages between IoT and sustainability. Moreover, both previously mentioned studies do not fall under the category of a systematic literature review, nor do they conform to any known reporting guidelines, and have not examined the advantages offered by UAV-assisted communications.
On the other hand, (Saini and Jain, 2023), employed a bibliometric method to explore the widespread impact of unmanned aerial vehicles on various aspects of social life, particularly focusing on their role in sustainable development, revealing 278 relevant articles from 2014 to 2023 through the Scopus database and identifying current trends, key contributors, and emerging subjects in the field. Both systematic literature reviews and bibliometric analysis are well-known and pertinent research techniques, yet they accomplish different goals and involve different procedures. The quantitative examination of bibliographic data, publication patterns, citation counts, and authorship trends are the main focus of bibliometric analysis. Evaluating the field’s impact, influence, and trends is frequently the aim. SLRs, on the other hand, are a qualitative research technique that seeks to thoroughly assess, summarize, and evaluate the body of existing literature. The purpose is to provide an overview of the current state of knowledge, identify gaps, and make evidence-based conclusion.
Our systematic literature review aims to fill these gaps by analyzing recent studies on the applications of aerial communication technologies to help build resilient infrastructure, promote inclusive and sustainable industrialization, and foster innovation. Different types of UAV-enabled communication scenarios, that have been applied in different countries and to different fields are covered in this paper. This systematic review is distinguished from other traditional or normal reviews in several key ways:
The remainder of the paper is structured as follows. Materials and methods including research questions, data sources, and inclusion criteria are presented in Section 2. A summary of the findings of the review, as well as a detailed comparison of the methods deployed in the included literature, are synthesized in Section 3. Identified gaps and future trends are presented in Section 4, and conclusions are drawn in Section 5.
This study consists of a Systematic Literature Review (SLR), conducted in accordance with PRISMA’s preferred reporting items statement (Prisma Checklist).
The SLR process (Figure 1) is comprised of three main phases: 1) Identification, 2) Screening, and 3) Eligibility. Upon the formulation of research questions, the identification phase defines the search strategy, including the choice of data sources and extraction methods to be used for the collection of relevant papers. Inclusion and exclusion criteria aligned with the specific requirements and scope of the review are outlined in the screening and eligibility phases where papers are filtered based on titles and abstracts (screening) and full-text (eligibility). Figure 2 details the results of each phase of the PRISMA process. The answers to the research questions are then synthesized, while the challenges, opportunities, and limitations are highlighted. These steps are defined in the subsequent sections below.
Progress made through the various aerial communication technologies shapes all sectors and has made a profound impact on all facets of society today. The development of such technologies can revolutionize the industry, result in new products and services, and contribute to a more sustainable society. Therefore, these technologies must be embraced and studied for their future impact on a sustainable future.
This systematic review identified essential research questions and, through a methodological study, tried to find relevant answers. Therefore, the research questions devised for this systematic review are:
These key questions have been used to achieve the main contribution of our paper, namely, to conduct a systematic review of recent studies on the applications of aerial communication technologies in order to support the further development of resilient infrastructure, promote inclusive and sustainable industrialization, and foster innovation. Moreover, this paper seeks to present a thorough summary of the many forms of aerial communication technologies, the domains to which they contribute, as well as the sustainable development objectives addressed by each publication. Additionally, this paper discusses the current state of research in this field as well as its primary obstacles and opportunities.
To address the research questions, the authors have used three databases: IEEE Xplore, Web of Science, and Scopus. The search for relevant publications was initiated on the
Using the research questions, keywords and queries were formulated according to the requirements of each scientific database used in the search. A combination of the keywords “aerial communication,” “sustainable,” “sustainability,” “UAV,” and “applied” was employed. All searches used the parameters “full-text,” “all metadata,” and “Document type: Journal article” when such specifications were supported by the search database. After completing the first draft of the search strings, we examined the results of each search string against each database to check the effectiveness of the search strings. The search time frame covered publications spanning the February 2013 - March 2023 period.
The initial search (Table 2) has identified 10,499 papers, 5449 of which were listed in the IEEE Xplore library, 4259 in Web of Science, and 791 in Scopus. Figure 2 illustrates the progression of studies through the screening phases. The abstract screening process resulted in 312 studies identified for a detailed review of full-text articles. After this review, we further excluded studies that did not meet the inclusion criteria, or that did not answer the research questions. We identified a total of 28 studies that met our eligibility criteria and addressed the research questions.
The collected papers from the initial search were screened according to the preset inclusion and exclusion criteria (Table 3). The paper selection process consisted of 2 phases. First, based on the inclusion and exclusion criteria, the papers were independently screened by two researchers through title and abstract screening. The publications selected during this phase were then independently assessed by four authors of our paper through full-text screening. The authors cross-checked the selection results and resolved any disagreement on the selection decisions. All disagreements in either the first or the second phase were resolved by consensus, and a third (identical to the first phase) or fifth (identical to the second phase) researcher was consulted to finalize the decision.
The authors formulated the inclusion and exclusion criteria to effectively select relevant papers. The documents were analyzed to assess the latest advancements and different applications of aerial communication for sustainable development. Journal papers written in English and within the scope of the research questions were included. Commercial papers, conferences, letters to the editor, e-books, books, and Ph.D. dissertations were excluded. Papers were excluded if they were review papers or only presented a theoretical framework without a tangible application. Papers not related to sustainable uses of aerial communications were removed, papers focusing only on energy performance, and energy consumption minimization were excluded, and papers presenting only trajectory design and optimization as well as resource allocation were also excluded. The authors deemed that the aim of the review was to identify applications focusing on building resilient infrastructure, promoting inclusive and sustainable industrialization, and fostering innovation. Relevant data were extracted from the selected publications for further analysis. In order to conduct this systematic literature review, the following data was retrieved: Authors list, Titles and abstracts, Year of publication, Technical characteristics, Type and network of aerial communication used, Methods and tools applied, Country where the study was conducted, Field of contribution covered, Sustainable development goal tackled.
This systematic literature review followed the PRISMA guidelines to screen and select the relevant literature. The selection of keywords to use in the initial query search can be influenced by bias. Additionally, the eligibility criteria defined by the authors are prone to subjectivity and thus increase the risk of bias. However, based on the PRISMA guidelines, the authors followed the best possible criteria to complete this systematic literature review. Independent selection processes and disagreement resolution techniques were utilized to ensure that the selection of publications is both transparent and relatively objective. A quality assessment system based on consensus between authors was used to ensure that publications of high quality and substantial contributions were included. This system was based on a checklist of the following criteria:
The search process represented by the flowchart in Figure 2 resulted in a total of 10,499 articles from all databases used. After the removal of duplicates, 10,129 papers remained. Out of which, 9817 studies were excluded after the title and abstract screening, as they did not fulfill the inclusion criteria. Out of the 312 studies that were full-text screened, 284 did not meet the inclusion criteria. Thus, finally, only 28 studies have been selected for inclusion in the current review and will be summarized in the following sections. The selection process took about 4 months to be completed and covered the 2013–2023 period span.
Nevertheless, papers using UAVs for sustainable development have been published from 2019 and onwards, as can be seen in Figure 3. The highest number of papers was published in 2022, while so far there has been a relatively large number of related publications for 2023. This number will significantly increase by the end of 2023 and it is very likely that it will surpass the year 2022.
In order to answer the formulated research questions posed by the literature review, a goal-based classification of the applications of aerial communication technologies was conducted. Thereafter, we evaluated the tools and methods used for the selected publications.
This section maps the selected papers to the SDGs, beginning with the one that garnered the least attention and progressing to the one that drew the most attention. Table 4 displays the number of publications on each SDG.
Goal 6 strives for universal access to clean water and sanitation, alongside sustainable water resource management. It targets challenges such as water scarcity, pollution, inadequate sanitation, and unsustainable water practices globally. Achieving Goal 6 leads to improved health, economic growth, ecosystem protection, and enhanced wellbeing within communities. Among the surveyed literature, only one paper tackled this goal, introducing an energy-efficient communication design in UAVs for water distribution.
In (Radhakrishnan and Wu, 2022), the authors introduced a UAV-enabled wireless-powered communication network, capable of beamforming radio signals to a target location and using it for both communication and sensors’ battery charging. The UAV energy consumption was minimized by integrating optimization and dome packing methods, which can find an optimal position and trajectory where the UAV will be hovering to direct wireless power transfer signals, as well as collect real-time data of water quality monitoring from ground sensors. This technology improves energy efficiency and security, minimizes communication costs, and reduces human interventions for battery replacements.
SDG 2 aspires to eradicate all types of malnutrition, including reaching the goals of stunting and wasting in children under the age of five, achieving food security, and promoting sustainable agriculture. This goal also aims to take care of the nutritional requirements of adolescent girls, pregnant and lactating mothers, as well as older people.
Few works covered the No Hunger sustainable development goal, they were all devoted to agriculture for different purposes. Singh and Sharma (2022), discussed the challenges climate change poses on agriculture and emphasized adapting to changing climatic conditions to increase food productivity. For that, it explored the potential of Wireless Sensor Networks (WSN) and UAVs in remote sensing to provide information about soil, water requirements, crop health, and the atmosphere. The authors suggested a four-layer architecture where the first layer is a set of small WSN clusters comprised of temperature, humidity, soil moisture, and water level sensors for data acquisition. Each cluster consists of a Cluster Head (CH) that communicates and transfers sensing data to the second layer comprising UAV. The second layer was also responsible for trajectory planning to minimize energy and time consumption. The third and fourth layers processed the data and provided analytical results to improve crop quality. Closely, an IoT and UAV-based crop health monitoring system for Saudi Arabian agriculture was presented in (Ammad Uddin et al., 2019). The suggested solution focused on resource optimization and clustering heterogeneous IoT devices for effective data collection. Several factors, including UAV trajectories, communication costs, weather, sensor heterogeneity, and variations, were taken into account when forming each cluster. The authors also introduced a virtual antenna array and dynamic clustering for data collection. The proposed system demonstrated its ability to collect data under challenging conditions and to be deployed in remote, rural locations. (Pamuklu et al., 2023). focus on enhancing smart farms through advanced task scheduling targeting processing of data collected by IoT devices (e.g., pest detection cameras) with limited energy and computing resources. To ensure timely task execution, the processing was distributed between Aerial Base Stations (ABS), acting as both task executors and data relays, and Multi-access Edge Computing (MEC) devices. Their findings highlight the reinforcement learning (RL) approach’s potential to improve smart farm operations by optimizing ABS usage for guaranteed IoT task processing, thereby increasing ABS hovering time and contributing to more sustainable agricultural practices.
SDG 3 aims to ensure everyone’s health and wellbeing, by providing universal healthcare and giving everyone access to medications and vaccinations that are both safe and effective. This SDG also shows a strong commitment to eradicating communicable diseases and epidemics of Acquired Immune Deficiency Syndrome (AIDS), tuberculosis, malaria, and others by the year 2030.
A total of three papers dealt with the goal of good health, with two of them addressing the COVID pandemic. In the first paper (Gupta et al., 2022), 6G-enabled services were deployed in a multi-UAV ecosystem together with 3 Fold Functions (3FFs), namely: social distancing, proper sanitization, and monitoring of affected areas, to tackle the COVID-19 pandemic and set the stage for any future cases. To that end, the authors proposed a layered and connected architecture. Initially, the governance layer sets guidelines for FFs monitoring using UAVs, and the communication layer allows real-time monitoring and information transfer using 6G, while the third layer tracks violations of 3FFs and allows remote monitoring and thermal inspections. Sanitization is accomplished by a swarm of hexacopter drones. An evaluation of 6G-based UAV channels was carried out versus traditional 5G-based UAV channels, where the 6G-based UAV channels were proven to be more effective. Similarly, Almalki and Alotaibi, 2022 in (Almalki et al., 2022a) included AI in a multi-functional drone to provide wireless services to combat the COVID pandemic. The proposed UAV system utilized thermal imaging cameras and a Modified Artificial Neural Network (MANN) for detecting face masks worn by individuals in public. Furthermore, their proposed system was able to perform basic diagnostic functions such as identifying elevated body temperatures to reduce the risk of infection transmission through close contact. Moreover, GPS transceivers were deployed for tracking people with elevated body temperatures to ensure they did not violate curfews. Lastly, the AI framework optimized the drone’s elevation angle altitude to enhance wireless connectivity with a ground station, resulting in improved throughput and reduced power consumption. The research in (Yang et al., 2019) proposed a three-layer design for deploying UAVs to assist medical patients. Base stations with limited bandwidth were covered by the first layer. The second layer was made up of UAVs that act as relay nodes and transmit data to other relay nodes, while the third layer is made up of non-relay UAVs that collaborate with the relay UAVs to transmit data to neighboring base stations. The authors created a multi-hop cooperative data routing mechanism inside the same framework to address the latency and energy efficiency problems associated with data transmission from UAVs to distant data centers while maintaining a high level of service. This was based on a genetic algorithm and a mixed-integer nonlinear programming paradigm.
Here, we demonstrate how SDG 9 can serve as both a goal and a tool. SDG 9 has a goal of building innovative environments, advancing sustainable industrialization, and creating resilient infrastructure. Three articles on the use of UAVs in business, innovation, and infrastructure covered a wide range of topics, including drone mobility, transportation, and tracking in coal mines. In (Aftab et al., 2019), the authors suggested an interface architecture between industrial Wireless Sensor Networks (WSN) and the Internet of Drones (IoD) used for WSN data transfer. IoD nodes togology and clustering are defined based on the bio-inspired dragonfly algorithm where drone and insect behavior features were mapped to each other (e.g., drones gathering sensor data while avoiding collisions with ech other imitate a swarm of dragonflies looking for food). Additionally, the topology was optimized for effective communication by choosing the optimum cluster head based on the location of the drone, its connectivity to the base station, and its remaining battery.
In (Aftab et al., 2019), the authors suggested an interface architecture between industrial Wireless Sensor Networks (WSN) and the Internet of Drones (IoD) used for WSN data transfer. IoD nodes topology and clustering are defined based on the bio-inspired dragonfly algorithm where drone and insect behavior features were mapped to each other (e.g., drones gathering sensor data while avoiding collisions with each other imitating a swarm of dragonflies looking for food). Additionally, the topology was optimized for effective communication by choosing the optimum cluster head based on the location of the drone, its connectivity to the base station, and its remaining battery.
The work in (Nikooghadam et al., 2021) focused on creating UAV trajectories that carry out many jobs simultaneously while offering consistent coverage over a targeted area, including delivery and transportation. This was accomplished by applying algorithms for last-mile delivery and uniform coverage applications, which have been proved through simulations to be effective while costing a minor increase in energy consumption. These methods were also proven to cover both circular and arbitrarily shaped regions. An Inertial Measurement Unit (IMU) and Ultra Wideband (UWB) were used in (Zhang et al., 2022) to provide accurate tracking of UAVs in coal mines. The suggested solution offered a trade-off between energy and accuracy in UAV placement through the use of an adaptive UWB signal transmission adjustment mechanism. Additionally, in order to guarantee data transmission to the ground server for remote tracking, the authors designed an on-demand trigger algorithm.
Life on Land (SDG 15) is one of the most important sustainable development goals, as it seeks to protect, restore and encourage the sustainable use of terrestrial ecosystems, manage forests sustainably, fight desertification, and halt and reserve land deterioration. Floods, wildlife monitoring, and wildfires have been investigated in numerous works related to the Life on Land goal.
Floods are one of the unforeseen natural catastrophes that are challenging to predict and monitor due to the possibility that sensor nodes may be washed up and get damaged. To solve this problem and provide continuous connectivity even during crucial flood times, the authors of (Goudarzi et al., 2022) presented a UAVs and WSN-based platform for real-time data collection and prediction. In the event of a flood, the architecture’s multi-hop WSN enables the deployment of UAVs as routers to close the network connection gap generated by idle nodes. This makes flood monitoring possible at all times. Additionally, a software-defined network (SDN) was used to control network connectivity and reliably detect and find abnormal events.
Regarding wildlife monitoring, it was suggested in (Chaudhry and Phillips, 2021) to use a UAV that gathers data and transmits it to and from a base station as an alternative to creating a routing protocol on a mobile ad hoc network. As a result, both when the UAV interacted directly with sensor nodes and when it passively collected data from waypoints, data was accessed more quickly and the percentage of nodes encountered rose. The Levy walk model of foraging by wild animals was imitated by the many mobile sensor nodes in a communication architecture where the UAV moved regularly around the field to collect data from them.
In terms of wildfire monitoring and detection, (Arafat and Moh, 2021), introduced Bio-Inspired Localization (BIL) and Bio-Inspired Clustering (BIC) algorithms in UAVs for monitoring and detecting wildfires. The authors used a Hybrid Gray Wolf Optimization (HGWO) technique to reduce localization errors and obtain high accuracy. By selecting the appropriate CH based on the UAV’s fitness rating, BIC based on the HGWO contributes to extending the network lifetime. The authors also proposed a Compressive Sensing (CS-GWO) method based on GWO for efficient data transmission from the CH to the base station, resulting in reduced routing delay and transmissions. In order to quickly identify bushfires, (Zadeh et al., 2022), built a multi-agent Mission Coordinating Architecture (MCA) on UAVs that provides continuous Situational Awareness (SA). A UAV-based fire spread probability map that is based on the amount of vegetation cover was employed by the MCA. The authors used the Fuzzy C-means method to define a distinct operation zone for each UAV, which helped to save battery life by preventing UAVs from colliding and collecting redundant data. A framework for synchronized communication that enables UAVs to work together adaptively to maximize total flight time was also incorporated into the MCA. Finally, the study used GA for mission planning and monitoring in order to pinpoint fire areas. (Zhang et al., 2021). examined the monitoring of forest fires using a fixed-wing UAV. Various WSNs were deployed across sensitive locations in the proposed strategy, and a UAV flew to each node to gather environmental data from each node. The research suggested a Bi-Level Hybridization-based Metaheuristic Algorithm (BLHMA) to make sure that the UAV and nodes in the mountain forest areas could effectively communicate with one another. In order to reduce the number of blind searches in the mixed-variable space and improve convergence, the method also employed a constructive heuristic based on Self-Organized Multi-Agent Competition (SOMAC). The positions and headings were then refined using a local search based on a multistage approximate gradient, which enhances the BLHMA’s convergence. In (Burhanuddin et al., 2022), to enhance the Quality of Experience (QoE) of UAV-users by optimizing the positions for all UAVs, the authors of this work devised a reinforcement learning strategy for mobile UAV-to-UAV (U2U) communication to aid rescue teams in fighting wildfires. In order to stream videos from other flying UAV users, a UAV at a high altitude served as a mobile base station (UAV-BS). Deep Q-Network and Actor-Criric were then used to optimize QoE video transmission to base station ones, define their ideal positions, and learn the dynamic fire areas and communication environment. The QoE, latency, and video smoothness of the suggested method were found to be superior to those of the Greedy algorithm. Lastly, the authors of (Panahi et al., 2022) wanted to put an end to forest fires rather than just detecting and warning people about them. Thus, they presented a system that, in contrast to others, combines two different UAV types: a group of ground-based fire-fighting UAVs (FUAV) that fly while carrying fire distinguishers and an Energy Harvesting Aided Surveillance UAV (EH-SUAV) with mounted cameras. If a fire was found when the SUAV was continuously scanning the target area for them, the SUAV would immediately send a fire alarm to the ground-based FUAVs, which would react fast to put it out and extinguish it.
More than half of the world’s population already lives in cities, and by the year 2050, it’s expected that 7 out of 10 people will live in metropolitan regions (The World Bank, 2022). Making cities and human settlements inclusive, resilient, and sustainable is the focus of Goal 11. This will be accomplished by ensuring access for all to safe and affordable housing with basic services and protecting urban areas from natural disasters.
In terms of interest and articles, UAV applications for sustainable cities and communities have drawn the most attention. In the first publication, the security component was of the utmost significance. Nikooghadam et al. (2021) developed a secure authentication system based on the elliptic curve for drones to secure safe and intelligent living for residents of smart cities. The strategy takes into account users, drones, and a control server, allowing users and drones to sign up for the control server and get the required credentials without compromising security. This system was demonstrated to be secure and impervious to known assaults in the random oracle paradigm while incurring low computation and communication costs.
In (Alam and Moh, 2022), a UAV swarm was used to efficiently monitor crowds. The authors suggested a two-phase architecture and routing control to achieve this goal by balancing the mission and communication performance. They proposed a Joint Trajectory Character Recognition (JTCR) that outperformed existing routing protocols in terms of tracking coverage rate, connectivity rate, the number of re-transmissions, packet delivery ratio, end-to-end delay, and energy consumption. The realistic mobility control of the UAV swarm at a reasonable cost to control overhead is principally responsible for making this achievable. The major aim of (Oubbati et al., 2019) is to exploit UAVs’ ability to see from above to enhance emergency response in metropolitan environments. The authors recommended UAVs to track down accidents on the road and concurrently give rescue crews precise position data. The suggested study calculates the shortest path for emergency vehicles while taking into account traffic conditions. Additionally, in light of their high mobility, it made use of a reliable routing system to reduce UAV energy consumption and guarantee stable communication amongst UAVs. With various obstructing impediments including underground garages, shopping centers, and high-rise buildings, the urban tracking problem in (Deng et al., 2021) is made slightly more difficult. In order to increase the accuracy of target tracking, this study introduced edge computing, UAV, and a network of security cameras. The tracking was then accomplished by suggesting an air-ground surveillance sensor network, which enhances reliability and responsiveness while minimizing the delay and the shortest path problem.
The post-disaster and catastrophe scenarios were handled by different works. In (Faraci et al., 2023), the authors combined edge intelligence and UAVs to support post-disaster rescue operations with disrupted ground communication infrastructure in emergency scenarios. In the proposed method, the UAVs autonomously provided edge computing services as a Flying Ad-Hoc network (FANET). At the same time, renewable-energy generators charged the batteries of UAVs. The authors used model-based reinforcement learning to determine the optimal number of UAVs that should take off based on current edge-computing service requests to conserve power and resources. Furthermore, the study used optimal management policy to ensure sufficient edge computing while minimizing satellite channel usage during periods of low green energy generation and high service requests. In (Minhas et al., 2021), the effectiveness of multihop routing in a man-made catastrophe scenario was assessed in relation to several clustering techniques and the presence of a UAV. The authors provided a reinforcement learning strategy to construct the routing topology and so enhance the energy efficiency while minimizing the immediate energy expenses because in such cases the energy dynamics are what’s most influenced and it becomes difficult to reach end-to-end connectivity.
The study presented in (Almalki F. A. et al., 2022) proved that real-time reporting is essential in the case of natural or man-made disasters, to reiterate. Since Aerial journalism is crucial in those urgent situations, Almalki emphasizes that investigative reporting and the rapid creation and dissemination of media content on a big scale are made possible by the incorporation of drones with integrated AI. For that reason, an improved propagation model that combined drones and AI was developed to support smart journalism and make the process more environmentally friendly and sustainable. In order to improve accuracy, connectivity, and media coverage, the authors assessed a number of optimal propagation models that integrate drones with their payloads, the ground control unit that supports their operation, as well as an AI framework that makes use of a Neural Network-Radial Base Function Network (NN-RBFN).
In (Varghese et al., 2022), the authors’ aim was to address the issue of broken communication in a disaster-affected area, whether it be man-made or natural. They introduced a terrain-ware algorithm and re-established a communication network, in the whole catastrophe area affected. The algorithm determined the smallest number of drones required to completely cover the affected area while maximizing the system’s average energy, allowing the drones to operate for longer periods of time. Similarly, (Hydher et al., 2020), addressed the problem of ongoing network connectivity in serious emergency situations. However, they took a different approach, suggesting the best location for the UAVs to serve as aerial base stations rather than recommending a framework based on WSN and UAVs for real-time data gathering and prediction. With this method, users’ equipment is allocated to each aerial base station in an effort to increase the wireless network’s overall spectral efficiency in disaster-affected areas. With the use of renewable energy sources including wind and solar energy, the work under (Donevski et al., 2022) intends to deliver UAV swarm-based communication in off-grid circumstances with restricted access to power grids. Therefore, to evaluate the effect of wind on UAV energy consumption, the authors included a realistic model. They also included energy generation modules for photovoltaic (PV) and wind turbines (WT). The ultimate objective was to assess the viability of self-sustaining energy systems for long-term, uninterrupted operation of UAV swarms in infrastructure-less, that is, disaster situations. To identify the best configuration based on covered area, WTs, PV panels, ground battery cells, and UAV swarm, they, therefore, offer a computationally efficient technique based on greedy sampling and binary search.
Last but not least, a sustainable city must ensure the safety of transmission lines to ensure human safety because manual inspection requires personnel to climb towers and measure physically with tools, which is exceedingly unsafe. The research in (Deng et al., 2022) provided a novel method for developing a mobile edge computing system and an improved lightweight object detection algorithm for an insulator online defect detection system. Insulate failure detection may be carried out in real time by utilizing a deep neural network partition method based on binary particle swarm optimisation. This intelligent recognition method can recognize insulator self-explosion at the terminal level and detect problems with an accuracy of 94.5%.
A summary of all the SDGs that greatly benefit from UAV-enabled aerial communication, geographical sources, year of publication, the type of UAV network, and the field of contribution used in the included literature is illustrated in Table 5.
A wide spectrum of methods is used to provide solutions for the various problems identified when UAVs are used for communication purposes. From the relevant research works selected, three major categories were distinguished, namely, Heuristic, AI/ML (Artificial Intelligence/Machine Learning), and Optimization, whose distribution is shown in Figure 4A. These techniques are utilized to improve numerous design and functional elements of UAV-based communications, such as channel modeling, resource management, positioning, and security. A per-paper summary of the utilized methods and techniques is presented in Table 6. In the following, we put the techniques in the context of investigated research problems.
Figure 4. UAV-based communication methods used in the included publications. (A) Distribution of included papers by category of methods. (B) Evolution of the methods used per year.
The heuristic solutions rely on various Bio-inspired designs which are used to effectively address optimization issues in a wide range of application domains by drawing on physical principles, and specific behaviors of living things (Darwish, 2018). The Genetic Algorithm (GA), based on biologically inspired operators like mutation, crossover, and selection (Beasley et al., 1993), is often used for optimization and search tasks. In Aftab et al. (2019), the authors used a Bio-inspired clustering scheme using the Dragonfly algorithm, a method based on the swarming behavior of dragonflies in nature (Mirjalili, 2016), to manage communication among drones in an industrial wireless sensor network. The research in Arafat and Moh (2021), also used bio-inspired localization, bio-inspired clustering as well as compressive gray wolf optimization; an algorithm that simulates grey wolf leadership hierarchy and hunting mechanism to detect and monitor wildlife. In Zadeh et al. (2022), the Genetic Algorithm was also merged with Fuzzy C-Mean, a data clustering technique that provides solutions for search and optimization problems in machine learning. This combination of methods enabled the authors to improve bushfire detection and response efficiency in bushfire-prone areas.
Optimization methods have been consistently used through the years and have been applied in various studies. These methods were mostly used to optimize UAV’s hovering positions for efficient communication with ground users Radhakrishnan and Wu (2022); Pamuklu et al. (2023); Deng et al. (2021); improve energy and cost efficiency Alam and Moh (2022); Yang et al. (2019); Zhang et al. (2022); Donevski et al. (2022); optimize and ensure a good network coverage, and a minimum QoS for users Hydher et al. (2020); Varghese et al. (2022); Khosravi et al. (2021), and predict future events using data acquired through WSN and UAVs Goudarzi et al. (2022). Particle Swarm Optimisation (PSO), was the technique that was most frequently utilized. This method models the social behavior of birds or fish, where particles (solutions) move around a solution space, modifying their placements based on their own best-known solution and the swarm’s collective knowledge. The shortest pathways were found using Dijkstra’s algorithm and the Travelling Salesman Problem (TSP). Dijkstra uses a weighted graph to find the shortest path between nodes, as opposed to TSP, which determines the shortest path between a set of points and locations that must be visited Dahiya and Sangwan (2018); Javaid (2013). Other algorithms, such as the Terrain-Aware Algorithm and Topology Aware Q-routing (TAQR), were utilized for the same goal.
Furthermore, the selected publications shed light on how the integration of AI techniques can be leveraged for various purposes in UAV-based communications. As shown in Figure 4B, beginning with one paper in 2021, then six in 2022, and three in the first 3 months of 2023, the inclusion of AI has been gaining a lot of traction in recent years.
The AI-based solutions mainly relied on machine learning techniques, such as Deep Neural Networks (DNN), which mimics how the brain processes information, and Reinforcement Learning, which is a training method based on rewarding desired behaviors and punishing undesired ones. These techniques enable the model to perceive and interpret its environment, take action, and learn through trial and error. Moreover, different iterations of YOLO were used, an algorithm that aims to predict an object’s class and the bounding box that defines the object location on the input image Han (2017); Jiang et al. (2022).
In Almalki et al. (2022a), the use of Aerial smart journalism was supported by a technology that combines drones with a Neural Network-Radial Base Function Network (NN-RBFN) to provide an accurate channel propagation model. This promotes sustainable and eco-efficient media and journalism services with high data rates and broad coverage footprints, whether in normal or hazardous situations. In the case of dangerous conditions indeed, Panahi et al. (2022) and Deng et al. (2022) respectively used reinforcement learning and deep neural networks to identify and put out forest fires and self-explosion defects in insulators in the presence of hazardous situations. AI-based systems were also used in pandemic scenarios, specifically COVID, where Faster Region-based Convolutional Neural Networks (F-RCNN), Modified Artificial Neural Networks (MANN), and Convolutional Neural Networks (CNN) were used in Gupta et al. (2022) and Almalki F. A. et al. (2022) to monitor social distancing and help reduce the risk of infection spread. In Minhas et al. (2021), Faraci et al. (2023) and Burhanuddin et al. (2022), Reinforcement Learning and Deep Reinforcement Learning based on Actor-Critic (AC) and Deep Q Network (DQN) were used for optimized multipath routing to ensure public safety and provide edge computing services in emergencies. In addition, Pamuklu et al. (2023) used Multi-agent Reinforcement Learning techniques to schedule tasks in smart agriculture using air base stations with limited energy capacities.
Few publications, namely, Nikooghadam et al. (2021); Chaudhry and Phillips (2021); Oubbati et al. (2019); Minhas et al. (2021), did not use any of the previously listed categories. These papers focused mainly on secure authentification schemes and routing protocols.
This systematic literature review formulated two research questions in order to identify the latest advancements and applications in sustainable and efficient aerial communication technologies and the key enablers and tools to achieve that. First, an SDG-based analysis was undertaken based on the review’s findings to distinguish between the applications employed in various studies. This distinction was observed to be related to the areas that each research has focused on, its fields of contribution, and its interests.
There are 17 goals and several targets in the SDGs of the United Nations. Yet, our findings demonstrate the narrow emphasis on just six of those, namely, SDGs 6, 2, 3, 9, 15, and 11.
In the second phase, findings were analyzed based on a methodological approach. An examination of the major categories of methods was conducted, namely, heuristics, optimizations, and AI/ML. The findings indicated that optimization methods had the highest frequency of publications (46,2%), followed by a recent emergence of AI/ML methods (38,5%) in solving UAV-based communications. This recent trend has only started emerging in 2021. It was shown that while UAVs were originally designed to be handled manually, the introduction of AI has enabled them to be controlled by machines, letting them perform a variety of duties more sustainably and efficiently. The least used category identified in the selected articles was heuristic approaches; however, they appear to be frequently included as early comparators to other methods or employed for simple tasks alongside optimization and AI methods.
Geographically, the United Kingdom, Saudi Arabia, China, and the USA registered the largest number of publications (six). While Romania, Pakistan, Estonia, Tunisia, Sri Lanka, Russia, Japan, Sweden, Algeria, and France all were part of one publication only (see Figure 5). It is noteworthy that the majority of works had contributions from different countries.
Figure 5. Geographical distribution of included papers. The smallest triangles represent one publication, while the largest ones represent five to six publications.
In fact, the highest contributions covering SDGs were found to be more used in developed and rich countries than in developing countries. We realized that contributions from South America were completely absent, and only two works from Africa; Algeria (Oubbati et al., 2019) and Tunisia (Almalki et al., 2022a) were registered collaboratively with UAE, France, USA, Australia, and Saudi Arabia. This can be explained by either a lack of understanding of the global sustainable development goals, a lack of resources and infrastructure to work on this issue, or a dearth of peer-reviewed journal publications. It is worth mentioning that multiple studies using UAV technologies for transportation in emergency situations were found in developing countries, but these publications were not included in the full-text screening because there was no communication involved.
Despite the screening process not focusing on papers that had novel ideas in communication. The majority of contributions were made in the telecommunications line, with a percentage of 76.7%, followed by contributions on Security and robotics, with a percentage of 6.7% on each, and only one contribution on each of the following lines: Transportation, Image Processing, and Environmental Sciences. (See Figure 6).
Although the findings allowed for a detailed synthesis of applications of sustainable aerial communication technologies, a few limitations and gaps were found, revealing topics for additional research and chances for practical applications. UAV-enabled communications, a critical element in non-terrestrial networks, have the potential to significantly advance SDGs which were not mentioned in the articles included in this SLR. Therefore, we offer a few concepts that can be covered in upcoming research-related activities.
Further, this extended coverage can be instrumental in promoting SDG 8 (Good Jobs and Economic Growth) and SDG 1 (No Poverty) by connecting remote areas to economic opportunities, thereby supporting local businesses and creating job prospects. It also enhances SDG 3 (Good Health and Wellbeing), and SDG 10 (Reduced Inequalities) by providing the necessary on-demand connectivity for telemedicine services in remote and disadvantaged areas. Lastly, this broad coverage promotes SDG 17 (Partnerships for the Goals) by fostering global partnerships, enabling connectivity in remote and underprivileged areas, and allowing these communities to participate in the global exchange of ideas and collaborative problem-solving.
Since 2015, member states of the United Nations have vowed to make the world a better and safer environment for everyone, everywhere by 2030. To that end, they created a roadmap and issued an urgent call to action for both rich and developing nations to work together in a global partnership. At its core are the 17 Sustainable Development Goals, which are intended to balance the economic, social, and environmental aspects of sustainable development.
This systematic review incorporates the latest scientific evidence and methods that focus on the importance of aerial communication in achieving these SDGs. Our study is based on a thorough selection and analysis of 28 scientific articles. This helped us identify six SDGs that gained the most from the published research on aerial communication facilitated by UAVs. In order of impact, these were: “Sustainable Cities and Communities,” where UAVs improve security and disaster management; “Life on Land,” where drones help with wildlife monitoring and reforestation; “Industry, Innovation, and Infrastructure,” where UAVs aid in infrastructure inspection; “No Hunger,” where drones improve agricultural practices; “Good Health and Wellbeing,” where UAVs assist with disease prediction and monitoring; and “Clean water and sanitation,” where drones ensure water distribution efficiently. The key tools and methodology used to achieve the goals targeted in each study were heuristic methods, optimization tactics, and more novel artificial intelligence techniques. It was also demonstrated that in many of the selected papers, a rise in the popularity of AI across several fields of application on UAV-based communications has been noticed.
This review also sheds light on the major gaps in the literature and proposes directions for future research as well as sustainable drone application in every area that is underrepresented, including “Gender Equality,” “Clean Water and Sanitation,” “Quality Education,” “Peace and Justice,” and others.
IG: Conceptualization, Data curation, Formal Analysis, Investigation, Methodology, Validation, Writing–original draft, Writing–review and editing. EV: Data curation, Methodology, Validation, Writing–original draft. AS: Data curation, Methodology, Writing–original draft. PB: Data curation, Methodology, Writing–review and editing. PM: Data curation, Methodology, Writing–review and editing. SP: Conceptualization, Supervision, Writing–review and editing.
The author(s) declare that no financial support was received for the research, authorship, and/or publication of this article.
We thank our Wavecore group colleagues for the insightful suggestions that helped us generate fresh concepts for the discussion section.
The authors declare that the research was conducted in the absence of any commercial or financial relationships that could be construed as a potential conflict of interest.
The author(s) declared that they were an editorial board member of Frontiers, at the time of submission. This had no impact on the peer review process and the final decision.
All claims expressed in this article are solely those of the authors and do not necessarily represent those of their affiliated organizations, or those of the publisher, the editors and the reviewers. Any product that may be evaluated in this article, or claim that may be made by its manufacturer, is not guaranteed or endorsed by the publisher.
Abdul, S. (2020). Internet of things for sustainable community development: introduction and overview. Cham: Springer International Publishing, 1–31. doi:10.1007/978-3-030-35291-2_1
Aftab, F., Khan, A., and Zhang, Z. (2019). Bio-inspired clustering scheme for Internet of Drones application in industrial wireless sensor network. SAGE Publ. doi:10.1177/1550147719889900
Akhtar, M. W., and Saeed, N. (2023). UAVs-enabled maritime communications: UAVs-enabled maritime communications: opportunities and challenges. IEEE Syst. Man, Cybern. Mag. 9, 2–8. doi:10.1109/MSMC.2022.3231415
Akyildiz, I. F., Kak, A., and Nie, S. (2020). 6G and beyond: the future of wireless communications systems. IEEE Access 8, 133995–134030. doi:10.1109/ACCESS.2020.3010896
Alam, M. M., and Moh, S. (2022). Joint topology control and routing in a UAV swarm for crowd surveillance. J. Netw. Comput. Appl. 204, 103427. doi:10.1016/j.jnca.2022.103427
Alkaabi, K., and Abuelgasim, A. (2017). Applications of unmanned aerial vehicle (uav) technology for research and education in uae. Int. J. Soc. Sci. Arts Humanit. 5 (1).
Almalki, A. A. A. M. C., Alotaibi, F. A., and Angelides, M. C. (2022a). Coupling multifunction drones with AI in the fight against the coronavirus pandemic. Computing 104, 1033–1059. doi:10.1007/S00607-021-01022-9
Almalki, F. A., Aljohani, M., Algethami, M., and Soufiene, B. O. (2022b). Incorporating drone and AI to empower smart journalism via optimizing a propagation model. Sustainability 14, 3758. doi:10.3390/su14073758
Ammad Uddin, M., Ayaz, M., Aggoune, E.-H. M., Mansour, A., and Le Jeune, D. (2019). Affordable broad agile farming system for rural and remote area. IEEE Access 7, 127098–127116. doi:10.1109/ACCESS.2019.2937881
Arafat, M. Y., and Moh, S. (2021). Bio-inspired approaches for energy-efficient localization and clustering in UAV networks for monitoring wildfires in remote areas. IEEE Access 9, 18649–18669. doi:10.1109/access.2021.3053605
Azari, M. M., Solanki, S., Chatzinotas, S., Kodheli, O., Sallouha, H., Colpaert, A., et al. (2022). Evolution of non-terrestrial networks from 5G to 6G: a survey. IEEE Commun. Surv. and Tutorials 24, 2633–2672. doi:10.1109/COMST.2022.3199901
Beasley, D., Bull, D. R., and Martin, R. R. (1993). An overview of genetic algorithms: Part 1, fundamentals. Univ. Comput. 15, 56–69.
Burhanuddin, B., Adilla, L., Liu, X., Deng, Y., Challita, U., and Zahemszky, A. (2022). QoE optimization for live video streaming in UAV-to-UAV communications via deep reinforcement learning. IEEE Trans. Veh. Technol. 71, 5358–5370. doi:10.1109/tvt.2022.3152146
Chaudhry, U., and Phillips, C. (2021). UAV aided data collection for wildlife monitoring using cache-enabled mobile ad-hoc wireless sensor nodes. Int. J. Adv. Comput. Sci. Appl. 12. doi:10.14569/IJACSA.2021.0121002
Dahiya, C., and Sangwan, S. (2018). Literature review on travelling salesman problem. Int. J. Res. 5, 1152–1155.
Darwish, A. (2018). Bio-inspired computing: algorithms review, deep analysis, and the scope of applications. Future Comput. Inf. J. 3, 231–246. doi:10.1016/j.fcij.2018.06.001
Deng, F., Xie, Z., Mao, W., Li, B., Shan, Y., Wei, B., et al. (2022). Research on edge intelligent recognition method oriented to transmission line insulator fault detection. Int. J. Electr. Power and Energy Syst. 139, 108054. doi:10.1016/j.ijepes.2022.108054
Deng, X., Liu, Y., Zhu, C., and Zhang, H. (2021). Air–ground surveillance sensor network based on edge computing for target tracking. Comput. Commun. 166, 254–261. doi:10.1016/j.comcom.2020.10.012
Donevski, I., Virgili, M., Babu, N., Nielsen, J. J., Forsyth, A. J., Papadias, C. B., et al. (2022). Sustainable wireless services with UAV swarms tailored to renewable energy sources. IEEE Trans. Smart Grid 14, 3296–3308. doi:10.1109/tsg.2022.3224517
European Commission Decision C (2023). Horizon europe - work Programme 2023-2024, digital, industry and spac.
Faraci, G., Rizzo, S. A., and Schembra, G. (2023). Green edge intelligence for smart management of a FANET in disaster-recovery scenarios. IEEE Trans. Veh. Technol. 72, 3819–3831. doi:10.1109/tvt.2022.3217331
Geraci, G., Garcia-Rodriguez, A., Azari, M. M., Lozano, A., Mezzavilla, M., Chatzinotas, S., et al. (2022). What will the future of UAV cellular communications Be? A flight from 5G to 6G. IEEE Commun. Surv. and Tutorials 24, 1304–1335. doi:10.1109/COMST.2022.3171135
Giordani, M., and Zorzi, M. (2021). Non-terrestrial networks in the 6G era: challenges and opportunities. IEEE Netw. 35, 244–251. doi:10.1109/MNET.011.2000493
Goudarzi, S., Soleymani, S. A., Anisi, M. H., Ciuonzo, D., Kama, N., Abdullah, S., et al. (2022). Real-time and intelligent flood forecasting using UAV-assisted wireless sensor network. Comput. Mater. and Continua 70, 715–738. doi:10.32604/cmc.2022.019550
Gupta, R., Bhattacharya, P., Tanwar, S., Sharma, R., Alqahtani, F., Tolba, A., et al. (2022). Fight against future pandemics: UAV-based data-centric social distancing, sanitizing, and monitoring scheme. Drones 6, 381. doi:10.3390/drones6120381
Han, S. (2017). Efficient methods and hardware for deep learning. Stanford University. Ph.D. thesis.
Hydher, H., Jayakody, D. N. K., Hemachandra, K. T., and Samarasinghe, T. (2020). Intelligent UAV deployment for a disaster-resilient wireless network. Sensors 20, 6140. doi:10.3390/s20216140
Javaid, S., Saeed, N., Qadir, Z., Fahim, H., He, B., Song, H., et al. (2023). Communication and control in collaborative UAVs: recent advances and future trends. Future Trends 24, 5719–5739. doi:10.1109/TITS.2023.3248841
Jiang, P., Ergu, D., Liu, F., Cai, Y., and Ma, B. (2022). A Review of YOLO algorithm developments. Procedia Comput. Sci. 199, 1066–1073. doi:10.1016/j.procs.2022.01.135
Khosravi, M., Enayati, S., Saeedi, H., and Pishro-Nik, H. (2021). Multi-purpose drones for coverage and transport applications. IEEE Trans. Wirel. Commun. 20, 3974–3987. doi:10.1109/TWC.2021.3054748
Laws, D. (2024). Drone laws for every country in the world. u.s.a. states and major cities. Available at: https://drone-laws.com/
Minhas, H. I., Ahmad, R., Ahmed, W., Waheed, M., Alam, M. M., and Gul, S. T. (2021). A reinforcement learning routing protocol for UAV aided public safety networks. Sensors 21, 4121. doi:10.3390/s21124121
Mirjalili, S. (2016). Dragonfly algorithm: a new meta-heuristic optimization technique for solving single-objective, discrete, and multi-objective problems. Neural Comput. Appl. 27, 1053–1073. doi:10.1007/s00521-015-1920-1
Nations, U. (2018). The 2030 Agenda and the sustainable development goals: an opportunity for Latin America and the caribbean,(LC/G.2681-P/Rev.3). Santiago. Tech. rep.
Nikooghadam, M., Amintoosi, H., Islam, S. H., and Moghadam, M. F. (2021). A provably secure and lightweight authentication scheme for Internet of Drones for smart city surveillance. J. Syst. Archit. 115, 101955. doi:10.1016/j.sysarc.2020.101955
Oubbati, O. S., Lakas, A., Lorenz, P., Atiquzzaman, M., and Jamalipour, A. (2019). Leveraging communicating UAVs for emergency vehicle guidance in urban areas. IEEE Trans. Emerg. Top. Comput. 9, 1070–1082. doi:10.1109/tetc.2019.2930124
Pamuklu, T., Nguyen, A. C., Syed, A., Kennedy, W. S., and Erol-Kantarci, M. (2023). IoT-aerial base station task offloading with risk-sensitive reinforcement learning for smart agriculture. IEEE Trans. Green Commun. Netw. 7, 171–182. doi:10.1109/TGCN.2022.3205330
Panahi, F. H., Panahi, F. H., and Ohtsuki, T. (2022). A reinforcement learning-based fire warning and suppression system using unmanned aerial vehicles. IEEE Trans. Instrum. Meas., 1. doi:10.1109/tim.2022.3227558
Policy, T. W. B. S., and Staff, R. (1990). The ecological economics of sustainability: making local and short-term goals consistent with global and long-term goals. Tech. Rep. Robert costanza, ben haskell, laura cornwell, herman daly, and twig johnson.
Radhakrishnan, V., and Wu, W. (2022). Energy efficient communication design in UAV enabled WPCN using dome packing method in water distribution system. Energies 15, 3844. doi:10.3390/en15103844
Saini, H. K., and Jain, K. (2023). Past, present, and future of UAV in sustainable development: a bibliometric analysis: bibliometric analysis of UAVs in sustainability. New York, NY, USA: Association for Computing Machinery. doi:10.1145/3607947.3608074
Singh, P. K., and Sharma, A. (2022). An intelligent WSN-UAV-based IoT framework for precision agriculture application. Comput. Electr. Eng. 100, 107912. doi:10.1016/j.compeleceng.2022.107912
Tataria, H., Shafi, M., Molisch, A. F., Dohler, M., Sjöland, H., and Tufvesson, F. (2021). 6G wireless systems: vision, requirements, challenges, insights, and opportunities. Proc. IEEE 109, 1166–1199. doi:10.1109/JPROC.2021.3061701
United Nations Development Programme (2023). United nations development Programme, 7355–7357. doi:10.1007/978-3-031-17299-1_3097
Varghese, B. V., Kannan, P. S., Jayanth, R. S., Thomas, J., and Shibu Kumar, K. M. B. (2022). Drone deployment algorithms for effective communication establishment in disaster affected areas. Computers 11, 139. doi:10.3390/computers11090139
Vinogradov, E., Sallouha, H., De Bast, S., Azari, M., and Pollin, S. (2018). Tutorial on UAVs: a blue sky view on wireless communication. Netw. Internet Archit. 14, 395–468. doi:10.13052/jmm1550-4646.1443
Wu, J., Guo, S., Huang, H., Liu, W., and Xiang, Y. (2018). Information and communications technologies for sustainable development goals: state-of-the-art, needs and perspectives. IEEE Commun. Surv. and Tutorials 20, 2389–2406. doi:10.1109/COMST.2018.2812301
Yang, Y., Qiu, X., Li, S., Wang, J., Chen, W., Hung, P. C. K., et al. (2019). Energy-efficient data routing in cooperative UAV swarms for medical assistance after a disaster. Chaos Interdiscip. J. Nonlinear Sci. 29, 063106. doi:10.1063/1.5092740
Zadeh, R. B., Zaslavsky, A., Loke, S. W., and MahmoudZadeh, S. (2022). A multiagent mission coordination system for continuous situational awareness of bushfires. IEEE Trans. Automation Sci. Eng. 20, 1275–1291. doi:10.1109/tase.2022.3183233
Zhang, H., Dou, L., Xin, B., Chen, J., Gan, M., and Ding, Y. (2021). Data collection task planning of a fixed-wing unmanned aerial vehicle in forest fire monitoring. IEEE Access 9, 109847–109864. doi:10.1109/access.2021.3102317
Keywords: aerial communication, drones, industry, innovation and infrastructure, sustainable cities, sustainable development goals, unmanned aerial vehicles
Citation: Gryech I, Vinogradov E, Saboor A, Bithas PS, Mathiopoulos PT and Pollin S (2024) A systematic literature review on the role of UAV-enabled communications in advancing the UN’s sustainable development goals. Front. Comms. Net 5:1286073. doi: 10.3389/frcmn.2024.1286073
Received: 30 August 2023; Accepted: 24 September 2024;
Published: 07 October 2024.
Edited by:
Di Zhang, Zhengzhou University, ChinaReviewed by:
Angelo Trotta, University of Bologna, ItalyCopyright © 2024 Gryech, Vinogradov, Saboor, Bithas, Mathiopoulos and Pollin. This is an open-access article distributed under the terms of the Creative Commons Attribution License (CC BY). The use, distribution or reproduction in other forums is permitted, provided the original author(s) and the copyright owner(s) are credited and that the original publication in this journal is cited, in accordance with accepted academic practice. No use, distribution or reproduction is permitted which does not comply with these terms.
*Correspondence: Ihsane Gryech, aWhzYW5lLmdyeWVjaEBrdWxldXZlbi5iZQ==
Disclaimer: All claims expressed in this article are solely those of the authors and do not necessarily represent those of their affiliated organizations, or those of the publisher, the editors and the reviewers. Any product that may be evaluated in this article or claim that may be made by its manufacturer is not guaranteed or endorsed by the publisher.
Research integrity at Frontiers
Learn more about the work of our research integrity team to safeguard the quality of each article we publish.