- 1Department of Electronics, Information and Bioengineering (DEIB), Politecnico di Milano, Milan, Italy
- 2Department of Biological and Environmental Sciences and Technologies (DiSTeBA), University of Salento, Lecce, Italy
In this Perspective article we intend to focus on the opportunity of modelling Shannon information and/or “semantic” information in the field originated by the convergence of bottom-up synthetic biology (in particular, the construction of “synthetic cells”) and the engineering approaches to molecular communication. In particular we will argue that the emerging technology of synthetic cell fabrication will allow novel opportunities to study nano-scale communication and manipulation of information in unprecedented manner. More specifically, we will discuss the possibility of enquiring on the transfer and manipulation of information in the chemical domain, and interpreting such a dynamics according to Shannon or to MacKay-Bateson (“semantic” information).
1 Synthetic Biology and Molecular Communication: Two Growing and Potentially Converging Fields
Synthetic Biology (SB) applies engineering principles to biology, aiming at designing, constructing and assembling biological parts, devices, systems, and processes that do not exist in nature. The latter sentence can be considered as a definition of SB, even though SB encompasses a plurality of goals and approaches. Applications of SB are though for practical purposes, like the production of biofuel and fine chemicals, the development of biosensors or microorganisms for bioremediation. SB, then, faces with the issues of design and optimization of living organisms by operations like adding, removing, substituting, modifying, hybridizing, their parts, and it is then a typical bioengineering practice. The natural system is “re-wired” for controlling its behavior, with a pre-defined goal in mind.
However, SB also plays a role in basic science, according to the motto “What I cannot create, I do not understand” (R. Feynman) and contributes, with robotics and artificial intelligence (AI), to the constructive or synthetic approaches to life and cognition (Damiano and Stano (2018)). In this arena, SB needs to identify the minimal (necessary and sufficient) elements that generate a desired pattern, looking for the Graal of minimal complexity. Two very relevant research lines are : 1) the “minimal cell/genome” one (Gil et al. (2004); Forster and Church (2006); Hutchison et al. (2016)), and 2) the construction of cell-like systems from scratch (Luisi (2002); Luisi et al. (2006); Ichihashi et al. (2010); Nourian et al. (2014); Schwille et al. (2018); Göpfrich et al. (2018); Deshpande and Dekker (2018); Friddin et al. (2019); Berhanu et al. (2019)).
With the term “molecular communication” (MC) a quite innovative field is indicated, belonging to the macro-area of non-conventional communication and networks engineering (Farsad et al. (2016); Nakano (2017); Akyildiz et al. (2019a)). It refers to the courageous approach of studying and exploiting chemical communications instead of the well-known electromagnetic ones. While biologists know well what chemical signals are, and that they exert bioactivities at different scales and in a very unique way, the novelty in the MC approach is that the entire phenomenology of chemical signalling is encoded and formalized according to rigorous information and communication theories (ICT) in order to achieve a successful control of them, and thus foresee radically new technologies capable of manipulating biological systems from the sub-cellular to the whole-body levels. The cultural roots of MC practitioners lie, then, in communication and network engineering principles, and the resulting approaches deploy well-established conceptual and mathematical tools that have successfully contributed to the modern hyper-connected world (Akyildiz et al. (2015)). MC, in this respect, promises analogous progresses in the bio-chem domains and the consequent development of bio-chem-ICTs. Potential applications range from smart drug delivery systems to bio-inspired nano-robots, from programmed nanodevices to nanonetworks, from brain-machine interfaces to hybrid bio-nanomachine/silicon-based systems (Akyildiz et al. (2019b)).
It seems clear, to us, that these two fields—SB and MC—despite the fact that speak different languages, have many common features (the main ones: adopting an engineering approach, sticking to the synthetic methodologies) and there are several possibilities for a convergence and a synergical growth. A frontier research can be envisaged, where nano-bio-info-cogno-devices are designed, built, and operated in an unprecedented manner. It is worth mentioning that there are already existing works in the literature that investigate MC using SB, as discussed in the recent surveys (Bi et al. (2021); Söldner et al. (2020)). In these works the biological components that can potentially serve as the main building blocks, i.e., transmitter, receiver, and signaling particles, for the design and implementation of synthetic MC systems are defined. For example, experimental works were made based on calcium signalling and bacteria (Martins et al. (2018); Amerizadeh et al. (2021)).
Here, we would like to comment on the ongoing progress in bottom-up SB, in particular on the construction of the so-called “synthetic cells” (SCs, also known as “artificial cells”, ACs). Such systems, indeed, have been recently developed for being engaged in MC. This will provide us with an opportunity for presenting new experimental approaches for enquiring about “information” with different perspectives, hoping that the present discussion will inspire future investigations.
2 Bottom-Up Synthetic Cells Engaged in Molecular Communication
A quite radical route to SB refers to building cell-like systems from scratch (Luisi (2002); Luisi et al. (2006)). The so-called bottom-up approach aims at constructing SCs capable of living-like behavior. Clearly, bottom-up SCs have very low complexity when compared to biological cells or to SCs built by genome synthesis and transplantation (Gibson et al. (2010)), nevertheless they are interesting because they address very fundamental questions, such as how the transition from non-life to life occurs, and allow the design of alternative (and sometimes “orthogonal”) cell-like systems from artificial and/or allegedly primitive molecules (Stano (2019b)). Bottom-up SCs are modular and programmable, and represent a totally new technology.
Bottom-up SCs (Figure 1A) are generally built by directed-assembly procedures, whereby molecules of different types are included inside or on the surface of cell-like semipermeable microcompartments (vesicles, coacervates, emulsions, gels) and establish a chemical system whose behavior resembles, at different degrees, what living cells do. The ultimate goal is the construction of SCs capable of self-fabricating all their molecular components (boundary included) via internal metabolic reactions, thus being “autopoietic” (Varela et al. (1974); Maturana and Varela (1980)). Current research is progressing rapidly, but autopoietic SCs are still out of reach. On the other hand, an increasing number of reports has dealt with the exchange of chemical signals and communication either between SCs either between SCs and biological cells.
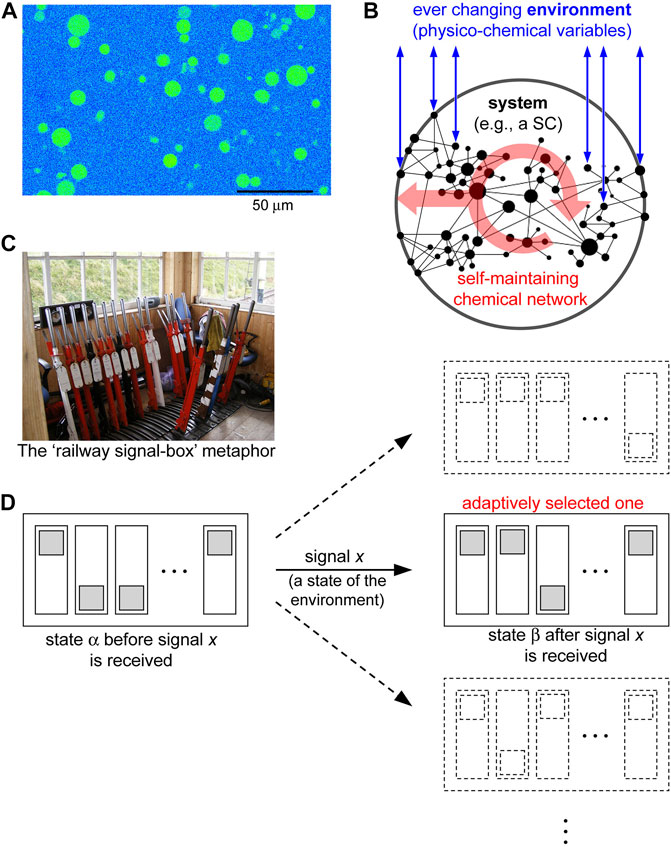
FIGURE 1. Synthetic cells (SCs) and semantic information. (A) Confocal fluorescence image of SCs producing a fluorescent protein. Size bar measures 50 μm. (B) SCs can be considered as chemical systems, whose dynamics is determined by an internal chemical network, surrounded by an ever changing (physico-chemical) environment. Complex SCs function thanks to a self-maintaining network, which is perturbed by the variations of the environmental variables (e.g., by the presence of chemicals which directly interact with the network, affecting its dynamics). The red arrows inside the system represent the self-production of components, included the ones which constitute the boundary. (C) MacKay used the “railway signal-box” metaphor for the changes that messages cause to the organization states of a receiver system, which he called “states of conditional readiness” (image in the public domain, https://www.publicdomainpictures.net/en/view-image.php?image=2462&picture=signal-box). (D) According to the “railway signal-box” metaphor, it is possible to draw a very schematic representation of the selecting role a signal x exerts on the states of a receiver system, here indicated by a transition between the states α and β. The systems states, for simplicity, have been represented by an array of binary system’s variables. In the case of biological cells, such a transition rule (α to β caused by x) was evolutionary “assigned” to the signal x adaptively, aiming at increasing the system fitness (e.g., the cell viability in the environmental conditions represented by the signal x), and it clearly depends on the initial state too.
Very simple SCs, built in 2009, were able to produce a sugar derivative that activated a bioluminescence response in the bacterium Vibrio harveyi (Gardner et al. (2009)). By employing gene expression strategy (Stano et al. (2012); Stano (2019a)), a number of bioinspired SCs were reported later. Examples are variegated, ranging from SCs acting as translators for Escherichia coli (activated by theophylline and sending isopropyl β-d-1-thiogalactopyranoside, IPTG) (Lentini et al. (2014)), to SC/SC signalling employing theophylline or arabinose signals that trigger the release of IPTG or doxycycline (Adamala et al. (2017)), and also from two-ways molecular communication between SCs and V. fischeri based on N-acyl-homoserine lactones, AHLs (Lentini et al. (2017)), to SCs that activate the expression of several genes in Pseudomonas aeruginosa again by AHLs (Rampioni et al. (2018)). Many other examples have been reported (reviewed in (Rampioni et al. (2019))), including a recent report about SCs/neuron communication (Toparlak et al. (2020)), and quite interesting cases where chemical communication eventually led to programmed operations like the production of a toxin to kill a biological cell. The latter example comes full circle as it brings us back to the concept of SCs as information-processing machines (i.e., not necessarily alive), and thus to the need a cybernetic control by applying the principle of ICT in the chemical domain. As mentioned, communicating SCs have relevance for the construction of programmable micro- and nano-devices for “smart” drug delivery, whereby—as already proposed—the MC engineering principles exquisitely match with the requirements of such future technology.
3 Information and Communication Theory Applied to Molecular Communication
The field of MC is quite young too. The main idea was introduced in the early 2000s by a group of communication engineers, with Tadashi Nakano being one of the most active proponents, as an “unexplored research area at the intersection of computer networks and biology” or even as a “paradigm shifting research” (Nakano (2017)). Several scholars have already established a theoretical framework to model the exchange of signals in the chemical domain, not in the electromagnetic one. This implies the adaptation of the ICT to the intrinsic stochasticity of molecular-level patterns, due to the granularity of matter and to the incessant background thermal motion (the “molecular storm” (Hoffmann (2012))), which very peculiarly affects all chemical systems. In contrast to communications based on electronic devices and electromagnetic signals, MC are generally characterized by low speeds, short range, high specificity, low energy needs, and massive parallelism. MC is typically stochastic in nature, due to the erratic movements of molecules and to their potential degradation.
MC accurate modeling requires mathematical tools that describe discrete molecular diffusion and Brownian motion, the first arrival time, and the dual-description embracing matter granularity and average concentrations (Kuran et al. (2020)). It is relevant to highlight, however, that despite the need of specific modelling tools, MC explains the phenomenology of interest by adopting the usual Shannon ICT as it is general enough and can be successfully adapted to the specific features of chemical signals (Nakano et al. (2013)). In particular, we refer to the full arsenal of concepts such as the modeling of a communication channel in terms of the same abstract layers that are employed in electromagnetic-based communication (encoding, transmitting, propagating, receiving, decoding elements), the information content of a source expressed in bits per emitted symbol, which relies on the concept of Shannon entropy, or the reliable transfer of information, which is based on the concept of Shannon channel capacity.
The Shannon capacity is obtained from the maximization of the mutual information (MI), which is a measure of the mutual dependence between the two random variables. More specifically, it quantifies the amount of information expressed in bits obtained about one random variable, through the other random variable. The MI is calculated as (Cover and Thomas (2012))
where H(D) is the entropy of the destination, i.e. the output, which is described by the random variable D, and H(D|S) is the conditioned entropy of the output D given the input, i.e. the source, which is described by the random variable S. The two entropies on the RHS of (Eq. 1) depend on the statistics of the specific channel.
The maximization of information transfer through molecule diffusion and chemical reactions is one of the leading research directions in MC theory. The approaches so far proposed to evaluate the capacity in MC mainly consider the diffusive channel, where signaling molecules are released in a fluid medium to transfer information between transmitting and receiving nanomachines (Farsad et al. (2016)). In this type of MC systems, the number of received molecules is statistically described by a Binomial random variable that, under some circumstances, can be well approximated as a Poisson random variable, i.e. the number of trials and the probability of success, are, respectively, sufficiently large and small (Jamali et al. (2019)). The Poisson approximation allows the application of analytical methods to derive tight upper and lower bounds of the channel channel capacity (Ratti et al. (2021)). Considering the exact Binomial channel, the capacity-achieving discrete input distribution can be efficiently obtained by applying numerical approaches based on the Blahut-Arimoto (BA) method (Farsad et al. (2020)). In the context of a biochemical scenario, a variant of the BA has been proposed in (Jetka et al. (2019)) with application to cellular signaling.
From the point of view of information content and processing, the use of biological materials and processes make MC to exhibit unique features that distinguish it from the current telecommunication technologies (Table 1). To achieve a more in depth knowledge of the mechanisms at the basis of information exchange between cells, it is important to take into account the autoregulation effect introduced by the feedback (Ratti et al. (2020)). This effect, known as retroactivity, is among the most important differences between the classical model of a digital communication system and the MC one. Therefore, the main goal is to consider the variations of the channel that are induced by interference due to nearby cells that subtract molecules from the environment, thus modifying the channel seen by the target cell, which is the final destination of the transmitted information. This knowledge makes possible the implementation of adaptation mechanisms to maximize the capacity of the MC channel that are inspired by those used in classical digital communication systems. Clearly, as the research moves on, researchers discover several important and stimulating open problems to address, but essentially—and this is the key point for our discussion—MC builds on Shannon ICT.
4 MacKay-Bateson Semantic Information
On the other hand, it is well known that Shannon information does not deal with the meaning of messages, i.e., with semantic aspects of information.1 It is then an intriguing open question whether or not the design and the construction of SCs capable of exchanging chemical signals can be an unprecedented experimental model to investigate, in an innovative manner, semantic information, and how to build rigorous theories for it. Such a possibility leads, when properly developed, to novel directions that could contribute to embody AI and cognition,2 according to the above-mentioned synthetic approach (Damiano and Stano (2018)).
Semantic theories of information were already present in the early cybernetics (Hayles (1999)). Donald M. MacKay, for instance, was one of the principal proponents of semantic information (MacKay (1969)). He pointed out that Shannon information has a “selective” nature (i.e., dealing with the selection of one possible message from the set of possible messages), while semantic information is essentially related to what information “does” when perceived by the receiver (Logan (2012)). Other authors refer to semantic information as “instructional” (Kauffman et al. (2008)), or “meaningful”, “functional”, “relevant”, “pragmatic” (see references in (Kolchinsky and Wolpert (2018))). Semantic information, then, is a concept related to the changes that a message causes in the internal organization of the receiver, with the consequence of modifying its internal dynamics (i.e., its behavior, its states), and essentially is a further instance of self-organization patterns in natural biological contexts. We refer to the changes that environmental physico-chemical variables cause on the dynamics of the chemical network, which constitutes the system we are interested in (e.g., a biological or a synthetic cell), Figure 1B. According to the MacKay “railway signal-box” metaphor, a working definition of a signal meaning could be “its selective function on the range of the recipient’s states of conditional readiness for goal-directed activity” [MacKay (1969), p. 35], see Figures 1C,D (details in the caption). MacKay semantic information, as evidenced by (Floridi (2011, 2015)) has interesting connections with the systemic approaches (situation logic), proposed by (Barwise and Perry (1983)). In the context of biological cells, the goal-directed activity can be identified with the processes assuring cell existence and adaptation to the ever-changing environment. However, it will be possible to identify beneficial processes, e.g., stabilizing ones, and especially goal-directed ones, even in non-living SCs. Therefore, while comparing semantic information with the Shannon approach, the focus shifts from the message itself (related to the probability of successfully sending, transmitting, receiving a specific message) to the mechanisms determining the dynamical patterns of the receiver, and to the reasons why these mechanisms have been established. It can be realized that this is how the reception of a certain message becomes a perception, associated to certain adaptive patterns.
Semantic information mottoes read that information is “the distinction that makes the difference” (MacKay) or “the difference that makes a difference” (Bateson) (Floridi (2010)). These expressions indicate that distinct (distinguishable) data/messages, for the receiver, functionally cause (are related to) different operations or transitions between states—within the receiver. While this sort of analysis is quite complex when it refers to human communication and behavior, it seems to us that enquiring on minimal semantic information might become an interesting and viable topic when studied by the SC model, especially because SCs can be constructed according to precise designs.
Looking at the semantic aspects of information means comparing and relating the environment and its variations (the presence/absence of chemical signals can be interpreted as a variation of the environment composition) with the internal states of a system—e.g., a SC—and their variations. These two entities (environment and system) co-variate in time. The system is blind to those variations that do not perturb its dynamical state. The “meaning” of such messages, instead, does not reside in the message itself, but is embodied in the organizational pattern (the network of transformations occurring within the system) that governs the system dynamics, which resulted as an adaptation to the perturbation. In this sense, the meaning depends on the context. A message (for example, a chemical compound), per se, has no meaning but the one that it possibly carries to the system because of the history of past adaptations in the case of natural cells (these relations have been shaped by evolution). The meaning, then, is generated, or constructed, by the system itself, within the relational boundaries of its own self-maintenance operations.
In SCs, meanings can be engineering because they are embodied in the internal molecular mechanisms that one can devise and construct by SB methods, being just a mapping of the effects of perturbation on the system internal dynamical organization. For the first time, then, the relations embodied in the molecules and their functional relations within a responsive intra-SC networks can be re-designed, constructed, combined, and hybridized in order to engineer meanings in a programmable fashion. Intriguingly, an example of messages that trigger different behaviour in the recipient system has been provided, in a purely MC modeling scenario, by (Felicetti et al. (2014)), who proposed a connection-oriented protocol, based on chemical exchanges, that realizes programmed transitions in a finite-state machine.
5 Concluding Remarks
The meaningless (Shannon) and the meaningful (MacKay-Bateson) facets of information complement each other, answering different questions. To parallel the established MC quantitative and formal approaches to Shannon information in the chemical domain, we call for similar efforts, as done recently by Kolchinsky and Wolpert (2018), to face semantic information. The synergy between SB and MC can provide an insightful platform for accomplishing this task. Besides this new application field that merge MC and SB, the idea of including semantic and goal-oriented aspects is a new paradigm that is currently under investigation also in more traditional ICT systems for the development of future 6G wireless networks (Strinati and Barbarossa (2021)).
Data Availability Statement
The original contributions presented in the study are included in the article/Supplementary Material, further inquiries can be directed to the corresponding author.
Author Contributions
All authors listed have made a substantial, direct, and intellectual contribution to the work and approved it for publication.
Conflict of Interest
The authors declare that the research was conducted in the absence of any commercial or financial relationships that could be construed as a potential conflict of interest.
Publisher’s Note
All claims expressed in this article are solely those of the authors and do not necessarily represent those of their affiliated organizations, or those of the publisher, the editors, and the reviewers. Any product that may be evaluated in this article, or claim that may be made by its manufacturer, is not guaranteed or endorsed by the publisher.
Acknowledgments
We thank the members of the European CIRCLE project for organizing The 3rd Workshop on Molecular Communications (Ghent, 4–6 April 2018), which fostered the collaboration between the two Authors.
Footnotes
1Here we do not refer to the semantics of intentional communication typical of higher organisms (in particular semantic aspects of linguistics), but to the very basic “signs” and “meanings” that evolution has embodied in the cell dynamics in response to chemicals. This approach is resonant with the “protosemiosis” corner of biosemiotics (Sharov and Vehkavaara (2015)). For example, environmental molecules such as nutrients or poisons cause different consequences on the cell behavior (moving toward a nutrient, or stepping away from a poison), but both the responses are adaptive and increases the cell fitness. For an observer, a nutrient and a poison have different “meanings” for the cell. Semantic information refers to what a message causes once received, i.e., how it is perceived by the receiver, how its “meaning” emerges.
2As highlighted by Dennett, “the term ‘information’, which is ubiquitous in cognitive science (and elsewhere), does not refer to Shannon information”, and “the concept of information we use in cognitive science is semantic information”, that is, information identified as being about something specific: faces, or places, or glucose, for instance (Dennett (2017)).
References
Adamala, K. P., Martin-Alarcon, D. A., Guthrie-Honea, K. R., and Boyden, E. S. (2017). Engineering Genetic Circuit Interactions within and between Synthetic Minimal Cells. Nat. Chem. 9, 431–439. doi:10.1038/nchem.2644
Akyildiz, I. F., Pierobon, M., and Balasubramaniam, S. (2019a). Molecular Communications and Networking [scanning the Issue]. Proc. IEEE 107, 1227–1229. doi:10.1109/jproc.2019.2927797
Akyildiz, I. F., Pierobon, M., and Balasubramaniam, S. (2019b). Moving Forward with Molecular Communication: From Theory to Human Health Applications [point of View]. Proc. IEEE 107, 858–865. doi:10.1109/jproc.2019.2913890
Akyildiz, I., Pierobon, M., Balasubramaniam, S., and Koucheryavy, Y. (2015). The Internet of Bio-Nano Things. IEEE Commun. Mag. 53, 32–40. doi:10.1109/mcom.2015.7060516
Amerizadeh, A., Mashhadian, A., Farahnak-Ghazani, M., Arjmandi, H., Rad, M. A., Shamloo, A., et al. (2021). Bacterial Receiver Prototype for Molecular Communication Using Rhamnose Operon in a Microfluidic Environment. IEEE Trans. NanoBioscience 21. doi:10.1109/TNB.2021.3090761
Berhanu, S., Ueda, T., and Kuruma, Y. (2019). Artificial Photosynthetic Cell Producing Energy for Protein Synthesis. Nat. Commun. 10, 1325. doi:10.1038/s41467-019-09147-4
Bi, D., Almpanis, A., Noel, A., Deng, Y., and Schober, R. (2021). A Survey of Molecular Communication in Cell Biology: Establishing a New Hierarchy for Interdisciplinary Applications. IEEE Commun. Surv. Tutorials 23 (3), 1494–1545. doi:10.1109/comst.2021.3066117
Calvanese Strinati, E., and Barbarossa, S. (2021). 6g Networks: Beyond shannon towards Semantic and Goal-Oriented Communications. Computer Networks 190, 107930. doi:10.1016/j.comnet.2021.107930
Damiano, L., Stano, P., and Stano, P. (2018). Synthetic Biology and Artificial Intelligence: Grounding a Cross-Disciplinary Approach to the Synthetic Exploration of (Embodied) Cognition. ComplexSystems 27, 199–228. doi:10.25088/ComplexSystems.27.3.199
Dennett, D. C. (2017). The Evolution of Minds. W. W. Norton & Company.From Bacteria to Bach and Back.
Deshpande, S., and Dekker, C. (2018). On-chip Microfluidic Production of Cell-Sized Liposomes. Nat. Protoc. 13, 856–874. doi:10.1038/nprot.2017.160
Farsad, N., Chuang, W., Goldsmith, A., Komninakis, C., Médard, M., Rose, C., et al. (2020). Capacities and Optimal Input Distributions for Particle-Intensity Channels. IEEE Trans. Mol. Biol. Multi-scale Commun. 6, 220–232. doi:10.1109/tmbmc.2020.3035371
Farsad, N., Yilmaz, H. B., Eckford, A., Chae, C.-B., and Guo, W. (2016). A Comprehensive Survey of Recent Advancements in Molecular Communication. IEEE Commun. Surv. Tutorials 18, 1887–1919. doi:10.1109/comst.2016.2527741
Felicetti, L., Femminella, M., Reali, G., Nakano, T., and Vasilakos, A. V. (2014). TCP-like Molecular Communications. IEEE J. Select. Areas Commun. 32, 2354–2367. doi:10.1109/JSAC.2014.2367653
Floridi, L. (2015). “Semantic Conceptions of Information,” in Stanford Encyclopedia of Philosophy (Winter 2019 Edition). Editor E. N. Zalta. Available at: https://plato.stanford.edu/entries/information-semantic/ (Accessed September 7, 2021).
Forster, A. C., and Church, G. M. (2006). Towards Synthesis of a Minimal Cell. Mol. Syst. Biol. 2, 45. doi:10.1038/msb4100090
Friddin, M. S., Elani, Y., Trantidou, T., and Ces, O. (2019). New Directions for Artificial Cells Using Prototyped Biosystems. Anal. Chem. 91, 4921–4928. doi:10.1021/acs.analchem.8b04885
Gardner, P. M., Winzer, K., and Davis, B. G. (2009). Sugar Synthesis in a Protocellular Model Leads to a Cell Signalling Response in Bacteria. Nat. Chem. 1, 377–383. doi:10.1038/nchem.296
Gibson, D. G., Glass, J. I., Lartigue, C., Noskov, V. N., Chuang, R.-Y., Algire, M. A., et al. (2010). Creation of a Bacterial Cell Controlled by a Chemically Synthesized Genome. Science 329, 52–56. doi:10.1126/science.1190719
Gil, R., Silva, F. J., Peretó, J., and Moya, A. (2004). Determination of the Core of a Minimal Bacterial Gene Set. Microbiol. Mol. Biol. Rev. 68, 518–537. doi:10.1128/MMBR.68.3.518-537.2004
Göpfrich, K., Platzman, I., and Spatz, J. P. (2018). Mastering Complexity: Towards Bottom-Up Construction of Multifunctional Eukaryotic Synthetic Cells. Trends Biotechnol. 36, 938–951. doi:10.1016/j.tibtech.2018.03.008
Hayles, N. K. (1999). How We Became Posthuman. Virtual Bodies in Cybernetics, Literature, and Informatics. Chicago & London: The University of Chicago Press.
Hoffmann, P. M. (2012). Life’s Ratchet. How Molecular Machines Extract Order from Chaos. 1st edn. New York: Basic Books. A member of the Perseus Books Group.
Hutchison, C. A., Chuang, R.-Y., Noskov, V. N., Assad-Garcia, N., Deerinck, T. J., Ellisman, M. H., et al. (2016). Design and Synthesis of a Minimal Bacterial Genome. Science 351, aad6253. doi:10.1126/science.aad6253
Ichihashi, N., Matsuura, T., Kita, H., Sunami, T., Suzuki, H., and Yomo, T. (2010). Constructing Partial Models of Cells. Cold Spring Harbor Perspect. Biol. 2, a004945. doi:10.1101/cshperspect.a004945
Jamali, V., Ahmadzadeh, A., Wicke, W., Noel, A., and Schober, R. (2019). Channel Modeling for Diffusive Molecular Communication-A Tutorial Review. Proc. IEEE 107, 1256–1301. doi:10.1109/jproc.2019.2919455
Jetka, T., Nienałtowski, K., Winarski, T., Błoński, S., and Komorowski, M. (2019). Information-theoretic Analysis of Multivariate Single-Cell Signaling Responses. Plos Comput. Biol. 15, e1007132. doi:10.1371/journal.pcbi.1007132
Kauffman, S., Logan, R. K., Este, R., Goebel, R., Hobill, D., and Shmulevich, I. (2008). Propagating Organization: an Enquiry. Biol. Philos. 23, 27–45. doi:10.1007/s10539-007-9066-x
Kolchinsky, A., and Wolpert, D. H. (2018). Semantic Information, Autonomous agency and Non-equilibrium Statistical Physics. Interf. Focus. 8, 20180041. doi:10.1098/rsfs.2018.0041
Kuran, M. Ş., Yilmaz, H. B., Demirkol, I., Farsad, N., and Goldsmith, A. (2020). A Survey on Modulation Techniques in Molecular Communication via Diffusion. IEEE Commun. Surv. Tutorials 23, 7–28. doi:10.1109/COMST.2020.3048099
Lentini, R., Martín, N. Y., Forlin, M., Belmonte, L., Fontana, J., Cornella, M., et al. (2017). Two-Way Chemical Communication between Artificial and Natural Cells. ACS Cent. Sci. 3, 117–123. doi:10.1021/acscentsci.6b00330
Lentini, R., Santero, S. P., Chizzolini, F., Cecchi, D., Fontana, J., Marchioretto, M., et al. (2014). Integrating Artificial with Natural Cells to Translate Chemical Messages that Direct E. coli Behaviour. Nat. Commun. 5, 4012. doi:10.1038/ncomms5012
Logan, R. K. (2012). What Is Information?: Why Is it Relativistic and what Is its Relationship to Materiality, Meaning and Organization. Information 3, 68–91. doi:10.3390/info3010068
Luisi, P. L., Ferri, F., and Stano, P. (2006). Approaches to Semi-synthetic Minimal Cells: a Review. Naturwissenschaften 93, 1–13. doi:10.1007/s00114-005-0056-z
Luisi, P. L. (2002). Toward the Engineering of Minimal Living Cells. Anat. Rec. 268, 208–214. doi:10.1002/ar.10155
Martins, T. V., Hammelman, J., Marinova, S., Ding, C. O., and Morris, R. J. (2018). An Information-Theoretical Approach for Calcium Signaling Specificity. IEEE Trans. Nanobioscience 18, 93–100. doi:10.1109/TNB.2018.2882223
Maturana, H. R., and Varela, F. J. (1980). Autopoiesis and Cognition: The Realization of the Living. 1st edn. D. Reidel Publishing Company.
Nakano, T., Eckford, A. W., and Haraguchi, T. (2013). Molecular Communications. Cambridge UK: Cambridge University Press.
Nakano, T. (2017). Molecular Communication: A 10 Year Retrospective. IEEE Trans. Mol. Biol. Multi-scale Commun. 3, 71–78. doi:10.1109/TMBMC.2017.2750148
Nourian, Z., Scott, A., and Danelon, C. (2014). Toward the Assembly of a Minimal Divisome. Syst. Synth. Biol. 8, 237–247. doi:10.1007/s11693-014-9150-x
Rampioni, G., D'Angelo, F., Leoni, L., and Stano, P. (2019). Gene-Expressing Liposomes as Synthetic Cells for Molecular Communication Studies. Front. Bioeng. Biotechnol., 7. 1, doi:10.3389/fbioe.2019.00001
Rampioni, G., D’Angelo, F., Messina, M., Zennaro, A., Kuruma, Y., Tofani, D., et al. (2018). Synthetic Cells Produce a Quorum Sensing Chemical Signal Perceived byPseudomonas Aeruginosa. Chem. Commun. 54, 2090–2093. doi:10.1039/C7CC09678J
Ratti, F., Magarini, M., Vecchio, D. D., et al. (2020). “The Impact of Retroactivity on Information Exchange in Molecular Communications,” in 7th ACM International Conference on Nanoscale Computing and Communication (New York, NY: Association for Computing Machinery), 1–2. doi:10.1145/3411295.3411311
Ratti, F., Vakilipoor, F., Awan, H., and Magarini, M. (2021). Bounds on the Constrained Capacity for the Diffusive Poisson Molecular Channel with Memory. IEEE Trans. Mol. Biol. Multi-Scale Commun., 1. doi:10.1109/tmbmc.2021.3054917
Schwille, P., Spatz, J., Landfester, K., Bodenschatz, E., Herminghaus, S., Sourjik, V., et al. (2018). MaxSynBio: Avenues towards Creating Cells from the Bottom up. Angew. Chem. Int. Ed. 57, 13382–13392. doi:10.1002/anie.201802288
Sharov, A. A., and Vehkavaara, T. (2015). Protosemiosis: Agency with Reduced Representation Capacity. Biosemiotics 8, 103–123. doi:10.1007/s12304-014-9219-7
Söldner, C. A., Socher, E., Jamali, V., Wicke, W., Ahmadzadeh, A., Breitinger, H.-G., et al. (2020). A Survey of Biological Building Blocks for Synthetic Molecular Communication Systems. IEEE Commun. Surv. Tutorials 22, 2765–2800. doi:10.1109/comst.2020.3008819
Stano, P. (2019a). Gene Expression inside Liposomes: From Early Studies to Current Protocols. Chem. Eur. J. 25, 7798–7814. doi:10.1002/chem.201806445
Stano, P. (2019b). Is Research on “Synthetic Cells” Moving to the Next Level? Life 9, 3. doi:10.3390/life9010003
Stano, P., Rampioni, G., Carrara, P., Damiano, L., Leoni, L., and Luisi, P. L. (2012). Semi-synthetic Minimal Cells as a Tool for Biochemical ICT. BioSystems 109, 24–34. doi:10.1016/j.biosystems.2012.01.002
Toparlak, Ö. D., Zasso, J., Bridi, S., Serra, M. D., Macchi, P., Conti, L., et al. (2020). Artificial Cells Drive Neural Differentiation. Sci. Adv. 6, eabb4920. doi:10.1126/sciadv.abb4920
Keywords: synthetic biology, synthetic cells, molecular communication, Shannon information, MacKay-Bateson information, semantic information
Citation: Magarini M and Stano P (2021) Synthetic Cells Engaged in Molecular Communication: An Opportunity for Modelling Shannon- and Semantic-Information in the Chemical Domain. Front. Comms. Net 2:724597. doi: 10.3389/frcmn.2021.724597
Received: 13 June 2021; Accepted: 31 August 2021;
Published: 21 September 2021.
Edited by:
Mauro Femminella, University of Perugia, ItalyReviewed by:
Lokendra Chouhan, King Abdullah University of Science and Technology, Saudi ArabiaMichael Taynnan Barros, University of Essex, United Kingdom
Luca Felicetti, Consorzio Nazionale Interuniversitario Per Le Telecomunicazioni, Italy
Copyright © 2021 Magarini and Stano. This is an open-access article distributed under the terms of the Creative Commons Attribution License (CC BY). The use, distribution or reproduction in other forums is permitted, provided the original author(s) and the copyright owner(s) are credited and that the original publication in this journal is cited, in accordance with accepted academic practice. No use, distribution or reproduction is permitted which does not comply with these terms.
*Correspondence: Pasquale Stano, cGFzcXVhbGUuc3Rhbm9AdW5pc2FsZW50by5pdA==