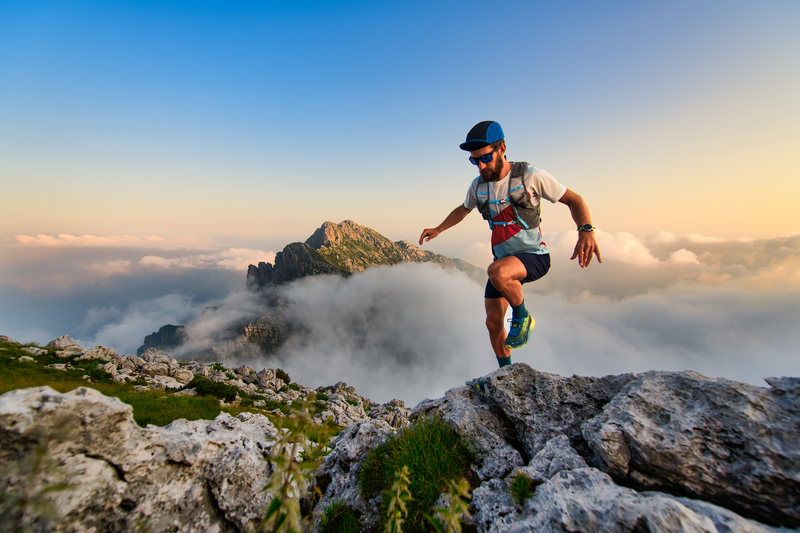
94% of researchers rate our articles as excellent or good
Learn more about the work of our research integrity team to safeguard the quality of each article we publish.
Find out more
SYSTEMATIC REVIEW article
Front. Commun. Netw. , 15 July 2021
Sec. IoT and Sensor Networks
Volume 2 - 2021 | https://doi.org/10.3389/frcmn.2021.679502
This article is part of the Research Topic AI-powered IoT for Intelligent Systems and Smart Applications View all 4 articles
Atrial fibrillation (AF) is one of the most common types of cardiac arrhythmia, with a prevalence of 1–2% in the community, increasing the risk of stroke and myocardial infarction. Early detection of AF, typically causing an irregular and abnormally fast heart rate, can help reduce the risk of strokes that are more common among older people. Intelligent models capable of automatic detection of AF in its earliest possible stages can improve the early diagnosis and treatment. Luckily, this can be made possible with the information about the heart's rhythm and electrical activity provided through electrocardiogram (ECG) and the decision-making machine learning-based autonomous models. In addition, AF has a direct impact on the skin hydration level and, hence, can be used as a measure for detection. In this paper, we present an independent review along with a comparative analysis of the state-of-the-art techniques proposed for AF detection using ECG and skin hydration levels. This paper also highlights the effects of AF on skin hydration level that is missing in most of the previous studies.
The main causes of the rise in the number of deaths in the European Union (EU) and the United States (US) can be attributed to a growing rate of heart diseases. It is estimated that around 47% of deaths annually are related to heart disorders that are usually ignored even after early warning signals (Alqarafi et al., 2017; Nisar et al., 2019). Most of these disorders are related to irregular atrial contraction causing abnormal heart rhythm or simply arrhythmia. Atrial fibrillation (AF) is the most common type of cardiac arrhythmia in which the heart beats faster than normal, and the number of patients suffering from AF has been growing over the past decade. An Electrocardiogram (ECG) is a popular test for diagnosing heart malfunctions including the detection of damaged heart muscle cells or conduction system. During the test, the ECG recording machine is connected through wires to several small sticky electrodes attached to the patient's body. Apart from the ECG machines installed at healthcare centers, various hand-held ECG devices are available to generate single-lead ECG, including WIWE®, AliveCor®, and OMRON HCG801 Heartscan®. Basically, ECG is a type of non-invasive testing that measures the electrical signals produced by heartbeats. There are various types of ECG machines having with different capacities for capturing data. For example, a 12-lead 300 Hz ECG monitor can generate hundreds of millions of points for each patient. The physicians analyze ECG data to identify different heart diseases such as AF, myocardial infarction, or acute hypotensive. The machine learning and deep learning approaches are employed to predict AF heart diseases. In this paper, we present an analysis along with a comparison of machine learning and deep learning based approaches for detection of these skin hydration and AF using ECG signals (Gogate et al., 2017b; Adeel et al., 2019b).
The A summary of the contributions of this study is given below.
• This paper outlines the AF and the skin hydration along with their mutual relationship.
• This study provides a survey of the state-of-the-art AF detection techniques based on machine learning to signify the need for interdisciplinary research in the field.
• The advantages and disadvantages of different AF detection schemes and skin hydration level measurement approach in the literature are highlighted that to provide a basis for further improvements research.
This paper is organized as follows. Section 2 provides an introduction to AF and its types. This section further presents a review of the machine learning-based AF detection methods. Section 3 introduces skin hydration and its importance in health condition diagnosis followed by a survey of the machine learning-based methods for skin hydration level determination. Section 4 highlights the effects of skin hydration or dehydration level on AF. Section 5 includes a comparison of AF detection schemes and skin hydration level measurement approaches. Finally, section 5 presents concluding remarks and potential future research directions.
Atrial fibrillation (AF) is a well-known cardiac arrhythmia that causes serious health concerns including mortality and can result in mortality. Nevertheless, the recent technological advancements in computing devices and algorithms can help in reducing the AF-related risks by detecting them in the early stages. In general, the machine learning and deep learning approaches extract useful knowledge from historical training data to make decisions in real-time applications. Furthermore, different types of sensors have been developed that can be used for the identification of cardiovascular, gait, and other activities of daily life (Shah et al., 2020). The presence of these computing devices, intelligent algorithms, and sophisticated sensors provide opportunities to develop systems that can reduce health hazards and improve quality of life, such as detecting AF in the early stage. In this section, we first present an overview of the common types of AF, and then discuss, the state-of-the-art AF detection approaches that are developed based on machine learning and deep learning.
Atrial fibrillation refers to abnormal heart behavior which can be symptomatic or asymptomatic and is caused by irregular patterns of atria. The common signs of symptomatic AF include shortening of breath, chest pain, faintness, and losing loss of consciousness (Maisel et al., 2001; Gogate et al., 2017a; Ullah et al., 2021). If these signs are ignored and if necessary actions are not taken in time, the AF may lead to morbidity or mortality. Broadly, the AF can be divided into two types, namely including aetiology and electrophysiology, based on symptoms (Kannel et al., 1982; Connolly et al., 2009). The American heart association recommends the classification of AF into first detection, paroxysmal, persistent, and permanently based on the temporal rhythm due to its' simplicity and clinical relevance (Sanna et al., 2014; Shah et al., 2021a).
The AF that is occurred for the first time to the patient. The first instance of AF detected in a patient regardless of the duration of arrhythmia is known as the first detection. There may be no presence of symptoms and this type of AF may or may not reoccur. For early detection of this incident, conventional event detection based on ECG signals is not preferred as this scheme is useful only when serious symptoms are seen. However, the first detection AF can be entirely silent and unnoticeable. Nevertheless, the presence of the first detection in the entire medical record of the patients is helpful to identify the preceding pattern which leads to the other types of AF. The data obtained from different sources and various patients at this stage can be combined to obtain high-quality data for maintaining the medical records of patients. Data analysis techniques can be used to identify the potential biomarkers from similar cases in such public healthcare databases. Subsequently, the patterns in the data can help achieve prediction or detection of AF that are recognized using machine learning approaches. Usually, the data from patients' bodies are collected using wearable devices such as smart watches equipped with sensors (1991; Wijffels et al., 1995).
Paroxysmal is a form of AF that stops on its own after a week. This is a recurring form of AF for which early identification or prediction is critical. There are two different approaches for the detection of Paroxysmal. The first method focuses on the AF episode detection from the sinus rhythm to distinguish the patients who have recently encountered AF episodes, while the second approach uses machine learning algorithms and bio-markers to detect any AF occurrences.
This type of AF commonly prolongs persists for more than a week and, therefore, the patients can be effectively diagnosed and treated. The machine learning techniques can be applied to predict the future episodes of AF to take necessary action in time.
The permanent AF is continuous in nature and does not disappear for a long period of time, usually more than a week. This type of AF may be developed from persistent AF that is not treated in time. Early detection of permanent AF can help provide better treatment to for the patient and delays in detection increase the risk to life. Moreover, in the case of permanent AF, it is easier to collect data as compared to other types of AF because of the availability of healthcare facilities for this case. This data can also be useful in the identification of different characteristics related to AF from the patterns that will help in the development of ML algorithms.
The most common type of an irregular heartbeat is AF and its symptoms include chest pain, palpitations, shortness of breath, fatigue, and even strokes in fatal cases. The AF can come as a silent stroke or asymptomatic AF where no symptoms appears before the AF. Unfortunately, it is difficult to detect a silent AF with such a short duration even using a 12-lead electrocardiogram because it requires longer monitoring intervals of the patient wearing devices, which can be troublesome (Jan and Koo, 2018). The diagnosis of patients with silent AF can be accelerated by improving the screening process in the detection of such unnoticed AF (Preethi and Sathiyakumari, 2016; Yu et al., 2020). Here, the machine learning approaches can be used to speed up the decision-making process while improving the reliability, efficiency, and accuracy of AF diagnosis. Furthermore, machine learning can be used to develop a risk model with the capabilities of identifying the patients at high risk of AF attacks (Liu et al., 2018).
The support vector machine (SVM) is a supervised machine learning algorithm that learns from examples with assigned labels. The range of applications of SVM includes recognizing different types of diseases such as AF, natural language processing (Dashtipour et al., 2020), human activity recognition (Taylor et al., 2020b), image processing (Jiang et al., 2021), fault detection, and diagnosis (Dashtipour et al., 2017; Jan et al., 2017; Jan and Koo, 2018; Asad et al., 2020).
The SVM is favored in scenarios where the volume of data is very big or the complexity of the data is very high (Gogate et al., 2019). This technique can also be used for AFf detection where complex test samples are obtained from patients suffering from the disease. The sample can be, for instance, a medical image compressed using wavelet transformation. The SVM classifier can achieve excellent unbiased excellent results in the training phase with a sensitivity of 99.2% and a specificity of 99.5% for AF detection problems. The performance further improves when the number of features is reduced from nine to four using linear discriminant analysis (LDA). The sensitivity reaches 99.07%, specificity to 100%, and positive prediction 100% positive prediction is achieved in this case (Jan et al., 2017).
The AF detection is based on two methods. The first method is to diagnose without considering the properties of waves, but this needs very high- resolution signals. The second method uses RR intervals as shown in Figure 1.
Figure 1. ECG waveform with R-R intervals (Sahoo et al., 2011).
The widely used classifiers in detection systems include SVM and linear discriminant analysis for ECG arrhythmic detection. For more reliable and accurate detection of AF, the RR interval of ECG signal is used as an input to an artificial neural network (ANN) for classification. R peak has strong robustness and the ANN classifier is good in at dealing with non-linear data. This classifier, when trained and tested on the AF Termination Challenge Database and MIT-BIH Arrhythmia Database, gave a sensitivity of 99.3%, specificity of 97.4%, and accuracy of 98.3%.
ECG signal-based classification techniques are composed of pre-processing, feature extraction, normalization, and classification steps. For pre-processing, a linear phase low pass filter, high pass filters, or median filters are used. For feature extraction, Discrete Wavelet transform, discrete cosine transform, Discrete Fourier transform, or continuous Wavelet transform, etc. are used. For feature normalization, Z-score and Unity Standard Deviation techniques are used. The classification techniques can be designed based on SVM, quantum neural network (QNN), ANN, or deep neural network (DNN). The AF detection needs long monitoring intervals of ECG signals. As a result, deep learning is used to identify different types of arrhythmia diseases and to achieve more precise outcomes when dealing with large amounts of data. Most researchers use the convolutional neural network (CNN) and Long short-term memory (LSTM) neural networks to eliminate feature extraction and achieve the best results, as shown in Table 1. This hybrid classifier performed much better than other machine learning algorithms. The CNN-LSTM gave 97.08% accuracy, 95.52% sensitivity, 98.57% specificity, and 98.46% precision.
Recently, the convolutional neural network (CNN) has gained a lot of attention due to its performance in various applications, image detection, time series analysis, and language processing. Time series analysis involves massive data, which is used in many health care scenarios. CNN consists of fully interconnected convolutional layers comprised of neurons. This proposed CNN-based method in (Xu et al., 2018) uses raw ECG signals. Another model is developed which comprises modified frequency slice wavelet transform (MFSWT) and CNN. The MFSWT performs better for low frequency ECG signals and its benefits are,include its precise time-frequency component position estimation and signal adaptiveness. MFSWT converts ECG signal into 2D space, then images were are given to 12-layered CNN which extract features of labeled images and calculate scores to sort the predicted image. For this system, the MIT-BIH AFDB database is used. This contains 25 ECG recordings collected from 25 subjects' data which is interpreted by a cardiologist. The time laps for each recording are 10 h and 15 min. Each ECG signal has 250 samples in 1 s and its resolution is 12-bits in a range of 10 mV. The results from the above setup give accuracy of up to 81.07% with five-fold cross validation, with sensitivity is at 74.96% and specificity is at 86.41%. The recurrent neural network (RNN) gives accuracy, sensitivity, and specificity of 97.8, 98.98, and 96.95% respectively, using five-fold cross-validation (Petrenas et al., 2012). CNN is a popular technique that combines extraction of features, reduction of features, and techniques of classification. It classifies the data with a fully connected multi-layer perceptron (MLP). The disadvantages of CNN include slow convergence speed and a lot of iterations. To overcome these drawbacks a combined CNN-SVM classifier is proposed which extracts features automatically from the CNN model. The result shows achieved accuracy is 96%, specificity is 96%, and sensitivity is 88%. There is another improved model for AF detection with an increased rate of data transmission and processing. This comprises 8-layer CNN with a shortcut connection and 1-layer LSTM.
The skin surface is an important parameter into the preparation of cosmetic products, image, and face recognition in security applications, and diagnosis of dermatological diseases like dryness, skin fungus, or allergic symptoms (Juyal et al., 2021). Nowadays, skin diseases are increasing, therefore, their diagnosis using proper analysis of skin texture is significant. Analysis of skin is based on different characteristics and features like dryness, pigmentation, skin oiliness, and allergic symptoms for the treatment of skin diseases (Preethi and Sathiyakumari, 2016). Patient's skin differentiation from others depends on skin measurements and then the an efficient algorithm is applied for the classification task (Ardali et al., 2019). Due to excess exercises, the human body undergoes dehydration. This affects the performance and efficiency of a person. The hydration level of the body is difficult to monitor on the market level. There are many methods to measure hydration levels using sensors. The most used method is Bio-electric Impedance Analysis (BIA), which measures the impedance of the body to the passage of low power and high frequency current. This method also estimates total body water, fat mass, intra and extra cellular water, and metabolic activity (Lapadula et al., 2020). BIA measures above mentioned water contents without using significant fluids and electrolytes. However, the segmental-BIA, multifrequency BIA, or bioelectrical spectroscopy in altered hydration states also requires further research (De Lorenzo, 2004). Body resistance has more value for dry skin like at 100 K ohms and its value is as low as 300 ohms beneath the skin. More than 99% of body resistance for current flow is at the skin (Fish and Geddes, 2009). The dehydration level in a person is the cause of many serious health conditions like diabetes, cancer symptoms, and cardiovascular abnormality etc.
The machine learning approaches are used in different applications such as cyber-security (Ieracitano et al., 2018), text sentiment analysis (Dashtipour et al., 2016, 2018, 2021; Hussien et al., 2018; Ahmed et al., 2021; Guellil et al., 2021), speech enhancement (Gogate et al., 2020a,b), speech recognition, IoT (Adeel et al., 2019a; Ozturk et al., 2019), health-care (Hussain et al., 2020; Taylor et al., 2020a), and posture detection (Liaqat et al., 2021).
Recently, machine learning has been used in skin hydration. The hydration levels are very important for all living organisms. Dehydration can be due to an extremely hot and dry environment, the sensation of thirst and drinking level there is low which increases levels of thirst and may cause a low hydration level. Overhydration occurs during dialysis of the kidney. Both dehydration and overhydration are fatal. There is a non-invasive method for the detection of hydration levels in living specimens. It consists of an RF transmitter that emits RF radiation signals ranging between 10 kHz and 2 GHz, consist of detecting the ions which are dissolved in the body water and pass through tissues and then are transmitted from the processor. The output signal represents the water level which is then compared with the reference signal of normal water level inside the tissue. The difference gives the indication of hydration level inside tissues.
Figure 2 shows a schematic diagram for skin hydration level detection which comprises of an RF generator and transmitter for RF signal. A set of RF signals of different frequencies is collected from a tissue of a living organism to be processed. This processed signal is then given to the indicator and compared to a set of reference signals collected when the subject has a normal hydration level. Then the difference between the received signal and reference signal is an the output signal, which indicates the degree of hydration level (Eyal-Bickels and Margaliot, 2005). Another method of detecting hydration level is to use water-permeable material. A sensing material that can be a disposable or reusable element that determines the amount of fluid of a subject in different conditions, for example, a blotting paper is sandwiched between two water-impermeable materials that can be as adhesive tapes and, placed inside the mouth of the user. The saliva of the user gets into the blotting paper through capillary action. The rate of flow of saliva on blotting paper helps to determine the hydration level. Different users need a different amount of liquid in maintaining hydration level, so the hydration sensor is calibrated according to the user in a specific condition. After calibration, the sensor becomes personalized to the user. In one idea an additional sensor is added along with a hydration sensor. This additional sensor measures the temperature or activity of the user and helps in the determination of hydration level. Dehydration may lead to a 10% decrease in performance of a subject and to headache, weakness, dizziness, cramps, nausea, and thirst. Not only dehydration but over-hydration can also be problematic. If a runner runs for 3 h and drinks a lot of water, but sodium and minerals are lost due to sweating, then sodium imbalance leads to seizure, pulmonary edema (lungs fluid), and respiratory arrest. To prevent both, amounts of fluid intake should be equal to sweat and urine losses (Howell et al., 2014; Gepperth et al., 2016).
There are many methods to measure hydration levels using sensors. The most used method is Bio-electric Impedance Analysis (BIA), which measures the impedance of the body to the passage of low power and high frequency currents. This method also estimates total body water, fat mass, intra and extra cellular water, and metabolic activity [3]. BIA measures above mentioned water contents without using significant fluids and electrolytes. There is a relationship between the electric resistance of the body and hydration level. The variation in electric resistance of the body is between a few ohms and thousands of ohms, and depends on the hydration level of the body. Electrodermal activity (EDA) sensor shows promise when used to detect hydration levels of subjects given the correct proportion of data varying over numerous subjects. The device has the potential to be used in a completely non-invasive and easy-to-deploy way, unlike current methods of determining hydration level (Kulkarni et al., 2021). EDA sensor is used for the measurement of galvanic skin resistance (GSR). GSR is sensitive to electric signals which are generated due to external or internal stimuli. The main impact of this research is to develop the model using machine learning for the assessment of hydration levels in the human body with the help of collected data. For better performance of HL detection, selection of window size, feature set, and algorithms are the main targets. In addition, a comparative study is done on GSR data which is collected in different body postures i.e., sitting, standing, and independent. The model for HL estimation is trained using different classifiers like SVM, logistic regression, KNN, Decision Tree (DT), Gaussian Naive Byes Classifier (NB), and Linear Discriminant Analysis (LDA).
KNN model obtained 95% of accuracy in the sitting posture of the dehydrated individual for the collected GSR data in a window size of 60 s (Rizwan et al., 2020). BITalino kit channel is used for the collection of data and its resolution is 16 bits. Supervised machine learning is applied because the state label is used for the hydration level of an individual. GSR data for the hydration and dehydration state of an individual is used for the training of the model. The hydration level estimator model is trained with a different types of classifiers i.e., random forest (RF), Naïve Bayes (NB), logistic regression (LR), decision tree (DT), LDA, Ada boost classifier (ABC), quadratic discriminant analysis (QDA), and KNN with all extracted features. In this experimentation, the training data set is 70% and the test data set are 30% of the model. This development of the model is done with three-fold cross-validation and gives an accuracy 91.3% with a random forest classifier among all other classifiers. (Liaqat et al., 2020). Figure 3 shows the main steps of hydration level estimation.
Earlier research explained that dehydration raises the risk of stroke in patients. Patients with dehydration have a 60% higher risk of ischemic stroke as compared to AF patients without dehydration (Swerdel et al., 2014).
There are different features that can be selected to identify skin hydration. For example, minimum, mean, variance, entropy, standard deviation, percentile, median, mode, and kurtosis (Ahmed et al., 2019). The importance of every feature is analyzed from different sizes of the window. For example, data is separated into non-overlapping segments and statistical features are analyzed for every 30 s of the chosen window size. However, most of the deep learning approaches do not require any feature engineering. It is worth mentioning that the finding of important features and selecting the correct combination of the features is an important task that can increase the performance of the machine learning approach. The main reason to use statistical features in most skin hydration state-of-the-art approaches is that they are easy to extract. The straightforward feature extraction technique is reducing the computational time and cost of processing, and in addition, it can be utilized for real-time models.
Most of the state-of-the-art approaches to evaluate the performance of the proposed approach used different evaluation metrics including accuracy, precision, recall, and f-measure are used (Shah et al., 2021b).
where TP denotes true positive, TN presents true negative, FP is false positive, and FN represents false negative, respectively.
The relationship between skin hydration and AF is not clear: either dehydration leads to AF or AF leads to dehydration. One hypothesis is that, due to dehydration, coagulability of blood is increased, which increases the risk of AF. Previous studies show that the risk of stroke is high with dehydration as compared to the hydrated person (Swerdel et al., 2014). Most people do not think that dehydration and AF have are linked with each other. However, the reality is that if a person is not properly hydrated then he can easily have AF. Due to changes in the level of fluids many functions of the body are affected, including heart function. Eating patterns, work stress, and exertion are all are reasons for dehydration, and this can increase the risk of AF.
In this section, we compare the state-of-the-art approaches proposed for AF detection and skin hydration level measurement. As shown in Table 1, the approaches which use the deep learning approaches can achieve better performance as compared to those approaches which utilize the conventional machine learning approaches.
In Table 2, we present a comparative analysis of the state-of-the-art AF detection and skin hydration level measurement approaches in terms of advantages and disadvantages.
Table 2. Advantages and disadvantages of different approaches for classification of AF and Skin Hydration Approaches.
In this paper, we present an overview of the state-of-the-art machine learning and deep learning approaches for AF detection and skin hydration level determination. There is a lack of available resources for the research community addressing such critical issues. Therefore, to cope with this scarcity problem, it is required necessary to collect a novel data set of patients suffering from AF and skin hydration abnormalities that can be used by researchers around the globe, which has been highlighted in this paper. As a future research direction, it is much needed to develop a hybrid machine learning and deep learning approaches to detect AF and determine the exact level of skin hydration from complex signals.
The original contributions presented in the study are included in the article/supplementary material, further inquiries can be directed to the corresponding author/s.
SL: methodology and conceptualization. KD, KAr, AZ, NR, and SU: formal analysis. KAs: investigation. All authors have read and agreed to the published version of the manuscript.
This research was funded under EPSRC DTA studentship which is awarded to AZ for his Ph.D. This research work was funded by EPSRC DTG EP/N509668/1 Eng. This work was supported in part by the Ajman University Internal Research Grant.
The authors declare that the research was conducted in the absence of any commercial or financial relationships that could be construed as a potential conflict of interest.
(1991). Stroke prevention in atrial fibrillation study: final results. Circulation 84, 527–539. doi: 10.1161/01.CIR.84.2.527
Adeel, A., Gogate, M., Farooq, S., Ieracitano, C., Dashtipour, K., Larijani, H., et al. (2019a). “A survey on the role of wireless sensor networks and IOT in disaster management,” in Geological Disaster Monitoring Based on Sensor Networks (Springer), 57–66. doi: 10.1007/978-981-13-0992-2_5
Adeel, A., Gogate, M., Hussain, A., and Whitmer, W. M. (2019b). Lip-reading driven deep learning approach for speech enhancement. IEEE Trans. Emerg. Top. Comput. Intell. 481–490. doi: 10.1109/TETCI.2019.2917039
Ahmed, R., Dashtipour, K., Gogate, M., Raza, A., Zhang, R., Huang, K., et al. (2019). “Offline Arabic handwriting recognition using deep machine learning: a review of recent advances,” in International Conference on Brain Inspired Cognitive Systems (Springer), 457–468. doi: 10.1007/978-3-030-39431-8_44
Ahmed, R., Gogate, M., Tahir, A., Dashtipour, K., Al-tamimi, B., Hawalah, A., et al. (2021). Deep neural network-based contextual recognition of Arabic handwritten scripts. Entropy 23:340. doi: 10.3390/e23030340
Alqarafi, A. S., Adeel, A., Gogate, M., Dashitpour, K., Hussain, A., and Durrani, T. (2017). “Toward's Arabic multi-modal sentiment analysis,” in International Conference in Communications, Signal Processing, and Systems (Springer), 2378–2386. doi: 10.1007/978-981-10-6571-2_290
Ardali, D. R., Rüether, L., Popov, V., Schlippe, G., and Vuksanovic, B. (2019). “Human skin profiling by physical skin biomarkers: a machine learning approach,” in Third International Congress on Information and Communication Technology (Springer), 151–160. doi: 10.1007/978-981-13-1165-9_14
Asad, S. M., Dashtipour, K., Hussain, S., Abbasi, Q. H., and Imran, M. A. (2020). “Travelers-tracing and mobility profiling using machine learning in railway systems,” in 2020 International Conference on UK-China Emerging Technologies (UCET), 1–4. doi: 10.1109/UCET51115.2020.9205456
Asgari, S., Mehrnia, A., and Moussavi, M. (2015). Automatic detection of atrial fibrillation using stationary wavelet transform and support vector machine. Comput. Biol. Med. 60, 132–142. doi: 10.1016/j.compbiomed.2015.03.005
Babaeizadeh, S., Gregg, R. E., Helfenbein, E. D., Lindauer, J. M., and Zhou, S. H. (2009). Improvements in atrial fibrillation detection for real-time monitoring. J. Electrocardiol. 42, 522–526. doi: 10.1016/j.jelectrocard.2009.06.006
Colloca, R., Johnson, A. E., Mainardi, L., and Clifford, G. D. (2013). “A support vector machine approach for reliable detection of atrial fibrillation events,” in Computing in Cardiology 2013, 1047–1050.
Cömert, Z., and Kocamaz, A. (2017). Comparison of machine learning techniques for fetal heart rate classification. Acta Phys. Pol. A 132, 451–454. doi: 10.12693/APhysPolA.132.451
Connolly, S. J., Ezekowitz, M. D., Yusuf, S., Eikelboom, J., Oldgren, J., Parekh, A., et al. (2009). Dabigatran versus warfarin in patients with atrial fibrillation. N. Engl. J. Med. 361, 1139–1151. doi: 10.1056/NEJMoa0905561
Dashtipour, K., Gogate, M., Adeel, A., Hussain, A., Alqarafi, A., and Durrani, T. (2017). “A comparative study of Persian sentiment analysis based on different feature combinations,” in International Conference in Communications, Signal Processing, and Systems (Springer), 2288–2294. doi: 10.1007/978-981-10-6571-2_279
Dashtipour, K., Gogate, M., Adeel, A., Ieracitano, C., Larijani, H., and Hussain, A. (2018). “Exploiting deep learning for Persian sentiment analysis,” in International Conference on Brain Inspired Cognitive Systems (Springer), 597–604. doi: 10.1007/978-3-030-00563-4_58
Dashtipour, K., Gogate, M., Cambria, E., and Hussain, A. (2021). A novel context-aware multimodal framework for Persian sentiment analysis. arXiv preprint arXiv:2103.02636. doi: 10.1016/j.neucom.2021.02.020
Dashtipour, K., Gogate, M., Li, J., Jiang, F., Kong, B., and Hussain, A. (2020). A hybrid Persian sentiment analysis framework: integrating dependency grammar based rules and deep neural networks. Neurocomputing 380, 1–10. doi: 10.1016/j.neucom.2019.10.009
Dashtipour, K., Poria, S., Hussain, A., Cambria, E., Hawalah, A. Y., Gelbukh, A., et al. (2016). Multilingual sentiment analysis: state of the art and independent comparison of techniques. Cogn. Comput. 8, 757–771. doi: 10.1007/s12559-016-9415-7
De Lorenzo, K. U. B. I. (2004). Ad deurenberg p. elia m. gómez jm heitmann bl kent-smith l. melchior j.-c. pirlich m. bioelectrical impedance analysis-part i: Review of principles and methods. Clin. Nutr. 23, 1226–1243.
Douglas, P. K., Harris, S., Yuille, A., and Cohen, M. S. (2011). Performance comparison of machine learning algorithms and number of independent components used in fMRI decoding of belief vs. disbelief. Neuroimage 56, 544–553. doi: 10.1016/j.neuroimage.2010.11.002
Eyal-Bickels, E., and Margaliot, S. (2005). System and Method for Detecting the State of Hydration of a Living Specimen. US Patent 6,849,046.
Fan, X., Yao, Q., Cai, Y., Miao, F., Sun, F., and Li, Y. (2018). Multiscaled fusion of deep convolutional neural networks for screening atrial fibrillation from single lead short ECG recordings. IEEE J. Biomed. Health Inform. 22, 1744–1753. doi: 10.1109/JBHI.2018.2858789
Fish, R. M., and Geddes, L. A. (2009). Conduction of electrical current to and through the human body: a review. Eplasty 9:e44.
Gepperth, A. R., Hecht, T., and Gogate, M. (2016). A generative learning approach to sensor fusion and change detection. Cogn. Comput. 8, 806–817. doi: 10.1007/s12559-016-9390-z
Gogate, M., Adeel, A., and Hussain, A. (2017a). “Deep learning driven multimodal fusion for automated deception detection,” in 2017 IEEE Symposium Series on Computational Intelligence (SSCI), 1–6. doi: 10.1109/SSCI.2017.8285382
Gogate, M., Adeel, A., and Hussain, A. (2017b). “A novel brain-inspired compression-based optimised multimodal fusion for emotion recognition,” in 2017 IEEE Symposium Series on Computational Intelligence (SSCI), 1–7. doi: 10.1109/SSCI.2017.8285377
Gogate, M., Dashtipour, K., Adeel, A., and Hussain, A. (2020a). Cochleanet: a robust language-independent audio-visual model for real-time speech enhancement. Inform. Fus. 63, 273–285. doi: 10.1016/j.inffus.2020.04.001
Gogate, M., Dashtipour, K., and Hussain, A. (2020b). “Visual speech in real noisy environments (vision): a novel benchmark dataset and deep learning-based baseline system,” in Proc. Interspeech 2020, 4521–4525. doi: 10.21437/Interspeech.2020-2935
Gogate, M., Hussain, A., and Huang, K. (2019). “Random features and random neurons for brain-inspired big data analytics,” in 2019 International Conference on Data Mining Workshops (ICDMW), 522–529. doi: 10.1109/ICDMW.2019.00080
Guellil, I., Adeel, A., Azouaou, F., Benali, F., Hachani, A.-E., Dashtipour, K., et al. (2021). A semi-supervised approach for sentiment analysis of Arab (IC+ IZI) messages: application to the Algerian dialect. SN Comput. Sci. 2, 1–18. doi: 10.1007/s42979-021-00510-1
Howell, T. A., Hadiwidjaja, A., Tong, P. P., and Thomas, C. D. (2014). Method and Apparatus to Sense Hydration Level of a Person. US Patent 8,734,341.
Hussain, A., Tahir, A., Hussain, Z., Sheikh, Z., Gogate, M., Dashtipour, K., et al. (2020). Artificial intelligence-enabled analysis of UK and US public attitudes on facebook and twitter towards covid-19 vaccinations. medRxiv. doi: 10.1101/2020.12.08.20246231
Hussien, I. O., Dashtipour, K., and Hussain, A. (2018). “Comparison of sentiment analysis approaches using modern Arabic and Sudanese dialect,” in International Conference on Brain Inspired Cognitive Systems (Springer), 615–624. doi: 10.1007/978-3-030-00563-4_60
Ieracitano, C., Adeel, A., Gogate, M., Dashtipour, K., Morabito, F. C., Larijani, H., et al. (2018). “Statistical analysis driven optimized deep learning system for intrusion detection,” in International Conference on Brain Inspired Cognitive Systems (Springer), 759–769. doi: 10.1007/978-3-030-00563-4_74
Jan, S. U., and Koo, I. (2018). A novel feature selection scheme and a diversified-input SVM-based classifier for sensor fault classification. J. Sens. 2018:7467418. doi: 10.1155/2018/7467418
Jan, S. U., Lee, Y., Shin, J., and Koo, I. (2017). Sensor fault classification based on support vector machine and statistical time-domain features. IEEE Access 5, 8682–8690. doi: 10.1109/ACCESS.2017.2705644
Jiang, F., Kong, B., Li, J., Dashtipour, K., and Gogate, M. (2021). Robust visual saliency optimization based on bidirectional Markov chains. Cogn. Comput. 13, 69–80. doi: 10.1007/s12559-020-09724-6
Juyal, S., Sharma, S., and Shankar Shukla, A. (2021). Smart skin health monitoring using ai-enabled cloud-based IoT. Mater. Today. doi: 10.1016/j.matpr.2021.01.074
Kannel, W. B., Abbott, R. D., Savage, D. D., and McNamara, P. M. (1982). Epidemiologic features of chronic atrial fibrillation: the Framingham study. N. Engl. J. Med. 306, 1018–1022. doi: 10.1056/NEJM198204293061703
Kulkarni, N., Compton, C., Luna, J., and Alam, M. A. U. (2021). “A non-invasive context-aware dehydration alert system,” in Proceedings of the 22nd International Workshop on Mobile Computing Systems and Applications, 157–159. doi: 10.1145/3446382.3448668
Lapadula, V., Sabatini, A., Zompanti, A., Buscaglione, S., Lanaro, D., and Meron, M. (2020). “A body hydration analysis system to improve running performance,” in 2020 IEEE International Workshop on Metrology for Industry 4.0 & IoT, 507–510. doi: 10.1109/MetroInd4.0IoT48571.2020.9138240
Liaqat, S., Dashtipour, K., Arshad, K., Assaleh, K., and Ramzan, N. (2021). A hybrid posture detection framework: Integrating machine learning and deep neural networks. IEEE Sens. J. 21, 9515–9522.doi: 10.1109/JSEN.2021.3055898
Liaqat, S., Dashtipour, K., Arshad, K., and Ramzan, N. (2020). Non-invasive skin hydration level detection using machine learning. Electronics 9:1086. doi: 10.3390/electronics9071086
Lim, H. W., Hau, Y. W., Lim, C. W., and Othman, M. A. (2016). Artificial intelligence classification methods of atrial fibrillation with implementation technology. Comput. Assist. Surg. 21(Supp. 1), 154–161. doi: 10.1080/24699322.2016.1240303
Liu, L., Shah, S. A., Zhao, G., and Yang, X. (2018). Respiration symptoms monitoring in body area networks. Appl. Sci. 8:568. doi: 10.3390/app8040568
Maisel, W. H., Rawn, J. D., and Stevenson, W. G. (2001). Atrial fibrillation after cardiac surgery. Ann. Intern. Med. 135, 1061–1073. doi: 10.7326/0003-4819-135-12-200112180-00010
Nisar, S., Tariq, M., Adeel, A., Gogate, M., and Hussain, A. (2019). Cognitively inspired feature extraction and speech recognition for automated hearing loss testing. Cogn. Comput. 11, 489–502. doi: 10.1007/s12559-018-9607-4
Ozturk, M., Gogate, M., Onireti, O., Adeel, A., Hussain, A., and Imran, M. A. (2019). A novel deep learning driven, low-cost mobility prediction approach for 5g cellular networks: the case of the control/data separation architecture (CDSA). Neurocomputing 358, 479–489. doi: 10.1016/j.neucom.2019.01.031
Petrenas, A., Marozas, V., Sornmo, L., and Lukosevicius, A. (2012). An echo state neural network for QRST cancellation during atrial fibrillation. IEEE Trans. Biomed. Eng. 59, 2950–2957. doi: 10.1109/TBME.2012.2212895
Pourbabaee, B., and Lucas, C. (2008). Automatic detection and prediction of paroxysmal atrial fibrillation based on analyzing ECG signal feature. IEEE Eng. Med. Biol. 8, 3–6. doi: 10.1109/CIBEC.2008.4786068
Preethi, M., and Sathiyakumari, K. (2016). Analyzing human skin texture using machine learning approaches. Int. J. Comput. Appl. 136, 975–8887. doi: 10.5120/ijca2016908313
Rizwan, A., Ali, N. A., Zoha, A., Ozturk, M., Alomainy, A., Imran, M. A., et al. (2020). Non-invasive hydration level estimation in human body using galvanic skin response. IEEE Sens. J. 20, 4891–4900. doi: 10.1109/JSEN.2020.2965892
Sahoo, S., Lu, W., Teddy, S., Kim, D., and Feng, M. (2011). “Detection of atrial fibrillation from non-episodic ECG data: a review of methods,” in Annual International Conference of the IEEE Engineering in Medicine and Biology Society, 4992–4995.
Sanna, T., Diener, H.-C., Passman, R. S., Di Lazzaro, V., Bernstein, R. A., Morillo, C. A., et al. (2014). Cryptogenic stroke and underlying atrial fibrillation. N. Engl. J. Med. 370, 2478–2486. doi: 10.1056/NEJMoa1313600
Shah, S. A., Abbas, H., Imran, M. A., and Abbasi, Q. H. (2021a). “RF sensing for healthcare applications,” in Backscattering and RF Sensing for Future WirelessCommunication. doi: 10.1002/9781119695721.ch8
Shah, S. A., Ahmad, J., Masood, F., Shah, S. Y., Pervaiz, H., Taylor, W., et al. (2021b). Privacy-preserving wandering behavior sensing in dementia patients using modified logistic and dynamic newton Leipnik maps. IEEE Sens. J. 21, 3669–3679. doi: 10.1109/JSEN.2020.3022564
Shah, S. A., Tahir, A., Ahmad, J., Zahid, A., Pervaiz, H., Shah, S. Y., et al. (2020). Sensor fusion for identification of freezing of gait episodes using wi-fi and radar imaging. IEEE Sens. J. 20, 14410–14422. doi: 10.1109/JSEN.2020.3004767
Singh, A., Thakur, N., and Sharma, A. (2016). “A review of supervised machine learning algorithms,” in 2016 3rd International Conference on Computing for Sustainable Global Development (INDIACom), 1310–1315. Ieee.
Swerdel, J., Janevic, T., Cosgrove, N., and Kostis, J. (2014). Myocardial infarction data acquisition system (midas 24) study group. The effect of Hurricane Sandy on cardiovascular events in New Jersey. J. Am. Heart Assoc. 3:e001354. doi: 10.1161/JAHA.114.001354
Taylor, W., Abbasi, Q. H., Dashtipour, K., Ansari, S., Shah, S. A., Khalid, A., et al. (2020a). A review of the state of the art in non-contact sensing for covid-19. Sensors 20:5665. doi: 10.3390/s20195665
Taylor, W., Shah, S. A., Dashtipour, K., Zahid, A., Abbasi, Q. H., and Imran, M. A. (2020b). An intelligent non-invasive real-time human activity recognition system for next-generation healthcare. Sensors 20:2653. doi: 10.3390/s20092653
Ullah, F., Naeem, M. R., Mostarda, L., and Shah, S. A. (2021). Clone detection in 5g-enabled social IoT system using graph semantics and deep learning model. Int. J. Mach. Learn. Cybern. 1–13. doi: 10.1007/s13042-020-01246-9
Wijffels, M. C., Kirchhof, C. J., Dorland, R., and Allessie, M. A. (1995). Atrial fibrillation begets atrial fibrillation: a study in awake chronically instrumented goats. Circulation 92, 1954–1968. doi: 10.1161/01.CIR.92.7.1954
Williams, N., Zander, S., and Armitage, G. (2006). A preliminary performance comparison of five machine learning algorithms for practical ip traffic flow classification. ACM SIGCOMM Comput. Commun. Rev. 36, 5–16. doi: 10.1145/1163593.1163596
Wu, Z., Feng, X., and Yang, C. (2019). “A deep learning method to detect atrial fibrillation based on continuous wavelet transform,” in 2019 41st Annual International Conference of the IEEE Engineering in Medicine and Biology Society (EMBC), 1908–1912. doi: 10.1109/EMBC.2019.8856834
Xu, X., Wei, S., Ma, C., Luo, K., Zhang, L., and Liu, C. (2018). Atrial fibrillation beat identification using the combination of modified frequency slice wavelet transform and convolutional neural networks. J. Healthc. Eng. 2018:2102918. doi: 10.1155/2018/2102918
Keywords: atrial fibrillation, skin hydration, machine learning and deep learning, healthcare, machine learning
Citation: Liaqat S, Dashtipour K, Zahid A, Arshad K, Ullah Jan S, Assaleh K and Ramzan N (2021) A Review and Comparison of the State-of-the-Art Techniques for Atrial Fibrillation Detection and Skin Hydration. Front. Comms. Net. 2:679502. doi: 10.3389/frcmn.2021.679502
Received: 11 March 2021; Accepted: 22 April 2021;
Published: 15 July 2021.
Edited by:
Uche Kennedy Chude-Okonkwo, University of Johannesburg, South AfricaReviewed by:
Syed Aziz Shah, Coventry University, United KingdomCopyright © 2021 Liaqat, Dashtipour, Zahid, Arshad, Ullah Jan, Assaleh and Ramzan. This is an open-access article distributed under the terms of the Creative Commons Attribution License (CC BY). The use, distribution or reproduction in other forums is permitted, provided the original author(s) and the copyright owner(s) are credited and that the original publication in this journal is cited, in accordance with accepted academic practice. No use, distribution or reproduction is permitted which does not comply with these terms.
*Correspondence: Sidrah Liaqat, c2lkcmExOTNAZ21haWwuY29t
Disclaimer: All claims expressed in this article are solely those of the authors and do not necessarily represent those of their affiliated organizations, or those of the publisher, the editors and the reviewers. Any product that may be evaluated in this article or claim that may be made by its manufacturer is not guaranteed or endorsed by the publisher.
Research integrity at Frontiers
Learn more about the work of our research integrity team to safeguard the quality of each article we publish.