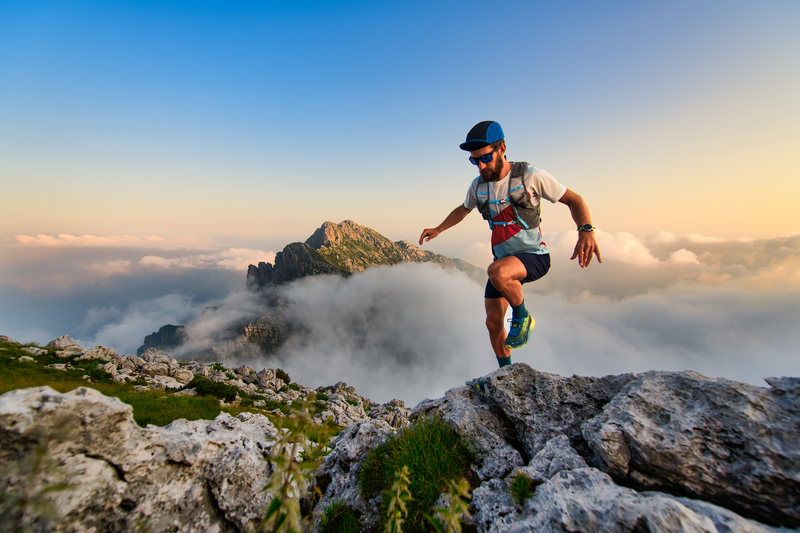
95% of researchers rate our articles as excellent or good
Learn more about the work of our research integrity team to safeguard the quality of each article we publish.
Find out more
REVIEW article
Front. Commun. Netw. , 17 May 2021
Sec. IoT and Sensor Networks
Volume 2 - 2021 | https://doi.org/10.3389/frcmn.2021.648181
This article is part of the Research Topic COVID-19: Role of Wireless Communication, Networking & Sensing Technologies for Rapid Pandemic Response View all 10 articles
COVID-19, caused by SARS-CoV-2, is now a global pandemic disease. This outbreak has affected every aspect of life including work, leisure, and interaction with technology. Governments around the world have issued orders for travel bans, social distancing, and lockdown to control the spread of the virus and prevent strain on hospitals. This paper explores potential applications for radar-based non-contact remote respiration sensing technology that may help to combat the COVID-19 pandemic, and outlines potential advantages that may also help to reduce the spread of the virus. Applications arising from recent developments in the state of the art for transceiver and signal processing technologies will be discussed along associated technical implications. These applications include remote breathing rate monitoring, continuous identity authentication, occupancy detection, and hand gesture recognition. This paper also highlights future research directions that must be explored further to bring this innovative non-contact sensor technology into real-world implementation.
Severe acute respiratory syndrome coronavirus 2 (SARS-CoV-2) has spread globally, after pneumonia of unknown cause was detected in Wuhan, China and first reported to the world health organization (WHO) on December 31, 2019 (World Health Organization, 2020). Since then, the associated coronavirus disease, COVID-19, has infected more than 54 million people all over the world and almost 10.9 million people in the United States (John Hopkins Medicine, 2020). The world is facing unprecedented challenges due to COVID-19 that jeopardize the priority of ensuring public health and safety. It has been found in various clinical investigations that most people infected by COVID-19 experience mild to moderate respiratory illness, and in some cases deadly breathing-related problems (Singhal, 2020). Governments in different countries are imposing travel bans, social distancing, and personal hygiene awareness. However, the spread of this deadly virus is increasing at a rapid rate which also imposes severe restrictions on day-to-day life. As more and more countries are on lockdown due to COVID-19 and an increasing number of people are living in isolation, the installation of in-home Doppler radar-based respiratory sensing systems can be beneficial. Doppler radar can potentially provide remote tracking of breathing rate and heart rate for isolated subjects (Lin, 1975), and recognize variations in breathing pattern to detect potential symptoms of COVID-19 (Grado et al., 2020; Taylor et al., 2020). Effective screening and timely isolation of patients can help reduce the number of infections. In addition, early recognition of infection through vital signs self-monitoring can allow early intervention and associated improvement in outcomes (Guidance for Industry and Food and Drug Administration Staff, 2020). Moreover, this unobtrusive respiration sensing technology can also be beneficial to track the status of breathing patterns after patients have left the hospital or ICU. Existing technology and solutions need to be developed quickly for diagnosis, monitoring, and molecular assessment to combat the current pandemic. Technologies need to provide unobtrusive monitoring to identify outbreak hotspots, count of occupants in closed indoor environments, and accurate recognition of symptoms without the need for physical proximity or contact.
Thus, remote vital sign sensing using microwave Doppler radar can have the potential to provide critical breathing-related information unobtrusively during this pandemic. A radar modified for biomedical purposes can track breathing rate through clothing (Droitcour et al., 2001; Islam et al., 2019), heart rate variability (Massagram et al., 2009a), tidal volume (Massagram et al., 2009b), and pulse pressure (Singh et al., 2011). Moreover, radar sensor technology has proved its initial efficacy for recognizing subject identity from breathing patterns (Islam et al., 2020e) and can also be used to count the number of occupants in a room (Yavari et al., 2014), which can potentially be used for security and surveillance applications to implement lockdown restrictions properly. This non-contact sensing solution is also intrinsically hygienic as it can also be used for gesture recognition unobtrusively (Zhang et al., 2016) or to replace push-button screens with contactless interactions.
This article provides an in-depth discussion of how radar-based non-contact sensing technology and its application in different areas can be used to help fight COVID-19 and reduce the spread of the disease. Prior research has mostly focused on discussing the state of the art of non-contact sensing techniques, without elaborating on the specific potential scope to fight COVD-19 (Taylor et al., 2020). The core contribution of this work is to illustrate different potential areas of non-contact radio-based sensing technology that may help in this fight. In this context, non-contact radio-based sensing technologies have the below capabilities which will be described in detail in the rest of the paper:
• Radar can track breathing rate and heart rate remotely without attaching any sensor to body surfaces. So, this non-contact sensing methodology can help to reduce the spread of the disease from patients to healthcare workers.
• An emerging area for radar sensing technology is the recognition of individuals from body motion information (breathing rate and heart rate). Authenticating people remotely can also help to maintain travel-related lockdown restrictions.
• Radar non-contact sensing technology can count the number of occupants in indoor settings, which may help to maintain lockdown restrictions and provide information for contact-tracing activities.
• Radar sensing technology can recognize hand gesture motion patterns. Unobtrusive hand gesture recognition is intrinsically hygienic and can replace contact interactions such as pushing buttons and/or touching screens, which can potentially reduce the spread of COVID-19.
The rest of the manuscript is organized as follows. How Does COVID-19 Affect the Heart and Respiratory System? section presents the effect of COVID-19 on the heart and respiratory system, whereas Basic Principle of Radar-Based Physiological Sensing section is focused on the basic principles of radar-based non-contact physiological sensing technology. Application Areas of Radar-Based Physiological Sensing Technology to Combat COVID-19 section provides a discussion on specific applications of radar-based non-contact respiration sensing technology (i.e., identity authentication systems, occupancy detection, and hand gesture recognition) to combat COVID-19. Discussion section concludes this paper.
The effect of COVID-19 on the lungs and heart is becoming well understood and, as the pandemic continues, more information about the role of the virus is damaging the human respiratory system and other organs will become much clearer (Singhal, 2020). It has been observed in various clinical investigations that during severe SARS-COV-2 infection, heart function decreases because of direct viral infection in the heart (John Hopkins Medicine, 2020). From prior studies and recent pandemic experiences, it is quite clear that COVID-19 is a respiratory-related disorder severely affecting the lungs (Singhal, 2020). Respiratory patterns associated with COVID-19 are considered to be generally distinct from those associated with flu or the common cold, with many infected persons exhibiting Tachypnea (Wang et al., 2020). While normal breathing involves rates of about 12 breaths/min and chest displacement on the order of 1 cm, COVID-19 related rates can be 20–30 breaths/min, with chest displacements of 0.5 cm or less. In the case of older patients, heart failure is occurring due to coronary artery disease or hypertension, as the body has decreased cardiac reserve capacity (John Hopkins Medicine, 2020). On the other hand, for younger patient’s heart failure occurs due to inflammation of the heart muscles and heart electrical system (John Hopkins Medicine, 2020). Shortness of breath is another common symptom of COVID-19 which can indicate when patients should seek medical attention. In addition, during exams, medical professionals need to interact directly with the patients to monitor vital signs which increases the risk of further infection. Thus, remote respiration sensing using microwave Doppler radar may help not only reveal the presence and severity of the disease but also minimize the risk of infection transmission between medical professionals and patients due to close contact (Lin, 1975).
Doppler radar first emerged in 1930 and it was employed for physiological sensing research since 1971 (Lin, 1975). Radar stands for “radio detection and ranging” (Lin, 1975). Radar is in its essence a transceiver that contains a transmitter and receiver of electromagnetic waves. Different radar topologies are used for acquiring the desired information (e.g., range, velocity, and angle) (Lin, 1975). Radars are usually named after the modulation technique which is used for the transmit signal. A simple configuration can be thought of as a pure continuous-wave (CW) system that can sense target motion based on the Doppler shift of the received reflected signal. A CW radar constantly sends and receives a narrow bandwidth signal. Due to its narrow bandwidth, the design of filters and amplifiers are simpler, as are the demodulation and signal processing algorithms (Droitcour, 2006). Although CW radar can measure velocity without any ambiguity, the main limitation is that pure CW radars cannot measure absolute range (Lin, 1975). To detect the target range with CW radar, the signal should have a type of timing marker to allow measurement of the round-trip delay time. One solution to the range issue is using frequency-modulated continuous-wave (FMCW) radar. This can detect both range and velocity of the target (Droitcour, 2006). The frequency modulation is usually triangular for FMCW systems, and the frequency varies gradually. Altimeters and Doppler navigation devices use this type of radar (Fioranelli et al., 2019). A Doppler radar motion sensing system typically transmits a continuous wave (CW) electromagnetic signal (sometimes frequency modulated) that is reflected off a target and then demodulated in the receiver (Droitcour, 2006). According to Doppler theory, a target with a time-varying position but no net velocity will modulate a reflected signal with a phase shift that varies in proportion to the time-varying position of the target. The phase difference is due to the variations in the round-trip time travel of the signal reflected off the target (Droitcour, 2006). Figure 1 illustrates the human subject’s chest motion and phase modulation caused by this movement. The transmitted signal can be expressed by the following equations:
where,
The transmitted microwave signal travels to the target and the backscattered signal which carries body motion information is captured by the receiving antenna. The received portion of the signal goes through a phase change due to path length variations and amplitude change. It can be expressed by the following equations:
where R(t) is the time-delayed version of the transmitted signal,
Thus, demodulating the phase will then provide a signal directly proportional to the small movement of the chest surface due to cardio-respiratory activities (Droitcour, 2006). The peak-to-peak chest motion due to respiration ranges in adults from 4 to 12 mm (Droitcour, 2006), while the peak-to-peak motion due to the heartbeat is in the range of 0.5 mm, with smaller variations from subject to subject (Benchetrit, 2000). When the wavelength is less than twice the peak-to-peak motion, the signal can be demodulated by simply multiplying the signal with an unmodulated signal from the same source (Droitcour, 2006). A Doppler radar with a frequency band around 2.4–24 GHz is mostly used for this type of biomedical application (Droitcour, 2006). There are no known health hazards attached to the system, as the maximum power consumption limit is almost 1,000 times less than the peak power of the ordinary global system for mobile communication (GSM) cellphone that is commonly encountered in day-to-day life (Benchetrit, 2000). The signal is then down-converted to baseband by mixing this with the transmitted signal and then sent to a low noise amplifier for amplification. When the signal is down-converted, it is multiplied with the same phase signal and a quadrature version of the transmitted signal. We receive two different types of channel signals; one is called the in-phase signal (I channel) and the other one is the quadrature-phase signal (Q channel). The output can be described by:
where,
FIGURE 1. Basic principle of non-contcat physiological sensing using microwave Doppler radar. The phase change of the reflected signal represented as
FIGURE 2. Doppler transceiver functionality. A typical single channel Doppler radar trasnceiver, (A), is used to produce time varying signals and spectra, (B) resulting from a human subject 1.5 m away from the radar. From Li et al. (2013).
In literature, many technologies have been utilized for daily activities and vital signs monitoring such as camera-based sensors and wearable devices (accelerometer, gyroscope, and magnetic sensor) (Shah and Fioranelli, 2019). Vision-based sensing is a mature technology, however it is light-dependent and often creates privacy concerns when deployed in private home environments. Wearable devices need to be worn on the body or otherwise carried around, which is often uncomfortable and not suitable for long-term monitoring applications (sleep, daily activities) (Shah and Fioranelli, 2019). Additionally, both vision-based and wearable sensors can come at a high cost due to their deployment complexity, maintenance overhead, and computationally expensive processing (Shah and Fioranelli, 2019). On the other hand, radar has non-contact-monitoring capabilities and an increasingly lower cost due to growth in inexpensive consumer electronics operating at microwave/millimeter-wave frequencies driven by the demand in automotive collision avoidance as well as 5G and Wi-Fi communications. As an example of the economical integration of radar systems with everyday objects, Google has integrated a 60-GHz radar with a mobile phone for automatic contactless control utilizing hand gestures, and also more recently within smart thermostats for human presence detection (Bohn, 2019; CNBC, 2020).
Remote life sensing of humans with Doppler radar has been widely used and reported in several research articles, with proof of concepts demonstrated for various applications (Li et al., 2013). This non-contact and non-invasive form of measurement has several potential advantages especially for neonates or infants at risk of sudden infant death syndrome (Hafner et al., 2007), adults with sleep disorders (Baboli et al., 2020), and burn victims because of its non-contact and unobtrusive measurement (Li et al., 2013). This form of respiration monitoring reduces patient discomfort and distress as electrodes need not be attached to the body. The inherent advantage of this unobtrusive non-contact measurement technique has increased its area of potential applications in occupancy detection, energy management in smart homes, baby monitoring, and has also opened the door to many other indoor applications (Li et al., 2013). In the last decade, with the growing interest in health and life sciences in the engineering community, many researchers have been contributing to the development of new front-end architectures, baseband signal processing methods, and system-level integration in biomedical radar to improve detection accuracy and robustness (Li et al., 2013). Moreover, Doppler radar has also been implemented to monitor the health and behavior of land and sea animals, including lizards and fish (Singh et al., 2012). Sleep monitoring is another emerging application that alleviates the use of obtrusive devices such as spirometers that can change the sleeping pattern (Baboli et al., 2015). A clinical study was performed to monitor patients with sleep apnea problems using radar sensors in conjunction with traditional sensors (Baboli et al., 2020). Sleep respiration monitoring devices have recently received FDA approval for commercial use in the United States (FDA, 2009). As radar can track sleep apnea events and sleep stages, any kind of breathing disorder can be easily tracked by such a biomedical sensor (Baboli et al., 2020). All these achievements can be used to present a clear picture of how this sensor technology can help to fight against the COVID-19 pandemic.
At the time of writing this article, most countries are on lockdown to minimize the spread of COVID-19. Moreover, those patients who have tested positive for COVID-19 without severe symptoms are in self-isolation at home. Even the robust healthcare system in developed countries is facing shortages of healthcare professionals, personal protective equipment, and mechanical ventilators in intensive-care-units (ICU) (Kissler et al., 2020). Thus, remote vital sign sensing of patients using radiofrequency (Radar) is gaining attention to support the monitoring of patient vital signs in their home environment during this public health emergency (Alwashmi, 2020). Additionally, new policies have been introduced to the development of remote vital signs monitoring devices to improve existing vital signs monitoring facilities (FDA, 2009). Considering the inherited advantages of this sensor technology, the system has the potential to fight COVID-19, as illustrated in the areas of applications in Figure 3. Specifically, we will focus on describing four different potential technological aspects of biomedical Doppler radar to combat COVID-19: remote breathing monitoring, unique identification, occupancy detection, and hand gesture recognition. The subsections will describe in detail the potential application areas.
FIGURE 3. Potential application areas of Radar-based physiological sensing technology for fighting the COVID-19 pandemic.
Triage decisions, diagnosis, prognosis, and the early detection of COVID-19 patient deterioration also depend on changes in respiratory rate (Massaroni et al., 2020). The World Health Organization (WHO) suggests that in a sedentary condition, if breathing rate crosses greater than 30 breaths/min then there is a higher chance of severe pneumonia in adults, while the threshold value for infants varies according to their age (World Health Organization, 2020). The decision on the use of supplemental oxygen in the ICU is also decided based on the breathing rate of the patient (Ayebare et al., 2020). Additionally, continuous monitoring of breathing rate helps with timely recognition of COVID-19 patient deterioration, thus contributing to early intervention strategies (Sun Q. et al., 2020). Thus, microwave Doppler radar which exploits the modulation of the radio waves reflected by respiratory-related thoracic movements is a promising technology for continuous respiratory monitoring (Lin, 1975; Droitcour, 2006; Li et al., 2013). Prior research has demonstrated the feasibility of utilizing Doppler radar for tracking breathing rate and heart rate accurately (Lin, 1975, Islam et al., 2019a; Droitcour et al., 2001; Droitcour, 2006; Li et al., 2013). Moreover, the feasibility of extracting different abnormal respiratory patterns using microwave Doppler radar has been investigated (Islam et al., 2019b; Lee et al., 2014). Figure 4 illustrates the spectral density (FFT) and continuous wavelet transform of radar captured normal (Figure 4A) and Cheyne-Stokes (Figure 4B) breathing patterns. For normal breathing, the breathing rate can be seen in the FFT and continuous wavelet transform, which is around 0.24 Hz (Lee et al., 2014). For Cheyne-Stokes breathing the dominant FFT peak is not as visible but it is much more visible in the continuous wavelet transform which is around 0.977 Hz. Cheyne-Stokes breathing is a respiratory disorder often encountered by patients with stroke, brain tumors, and traumatic brain injury (Lee et al., 2014). The overall validation of the radar measurement in comparison to the gold standard respiration belt signal for eight different abnormal respiratory patterns was also investigated and the accuracy of the radar measurement was around 94.25% (Lee et al., 2014). Patients that need continuous vital sign monitoring, even during everyday life activities, can be equipped with the radar system in their home environment, which can provide valuable information about their breathing status. During pandemic isolation, if a patient has a breathing problem occurring, an alarm can go off to warn caregivers that he/she may need emergency contact with the medical team. Similarly, radar can also track any breathing rate related discrepancies, such as if respiratory rate exceeds 30 breaths/min, and could trigger an alarm to warn the monitored subject. In this way, early medical intervention can be ensured at the earliest onset of breathing problems to help reduce fatality rates. Also, proximity to an infected person during the measurement of vital signs greatly increases the likelihood of contagion transfer. Non-contact radar-based vital signs monitoring may thus allow greater control of viral spread due to its remote form of measurement. However, there are some technological challenges in deploying such a sensor system in a multi-subject environment, which is likely to occur in a hospital or in-home environment. When there are multiple subjects in front of the radar, a combined mixture of breathing patterns results which may appear as interference countering the determination of the true respiration patterns (Islam et al., 2018a; Islam et al., 2018b; Islam et al., 2020d). Thus, the next section details some technical achievements addressing the challenge of multiple subject monitoring.
FIGURE 4. Spectral density (FFT) and continuous wavelet transform of normal breathing, (A), and Cheyne Stokes breathing, (B). Taken from Lee et al. (2014).
Separating individual respiratory signatures from a combined mixture is a critical challenge that must be met in order to implement this sensor technology in real-world scenarios (Islam et al., 2018a; Islam et al., 2018b; Islam et al., 2020d). The technology works well for isolated single subjects, especially when subjects are following social isolation and keeping physically separated from each other. In a typical home or hospital environment, there is a significant probability of the presence of multiple subjects in the radar field of view, which results in radar measurement of a combined mixture of breathing patterns that is difficult to separate (Islam et al., 2018a; Islam et al., 2018b). Figure 5 illustrates the challenges of respiration sensing in multi-subject environment. Prior research has focused on utilizing separate antennas for each different occupant, which is not usefully scalable in realistic scenarios (Islam et al., 2018b). Still, having more than one antenna element helps to estimate the angular location or spatial position of the target (Islam et al., 2018b). If the antenna array elements are separated by distance, d, with a wavefront incident at an angle,
FIGURE 5. Illustration of challenges of respiration sensing in multi-subject environments. While it is possible to isolate one well-spaced subject’s respiratory signature from a combined mixture using direction of arrival (DOA), subjects closer together impose antenna array resolution limitations for DOA. Alternatively, the ICA/JADE algorithm can employ spectral analysis to isolate respiratory signaturesfor subjects in close proximity.
In summary, ICA attempts to recover pure source signals by estimating a linear transformation using a criterion that measures statistical independence among the sources. The objective of ICA is to find a separating matrix or demixing matrix, W which is equivalent to
One of the fundamental challenges in radio-frequency (RF) based respiration sensing technology is the motion noise produced by random torso or limb movement. Prior research successfully demonstrated radar remote respiration sensing during random body movement (Li and Lin, 2008; Gu et al., 2013a; Gu et al., 2013b). In their proposed method random body movement can be minimized in three different ways. The frist method involves utilization of phase compensation at the RF front end (Gu et al., 2013a), the second involves adding phase-compensation at the baseband signal (Gu et al., 2013a), and the third involves cancellation of random body movement during demodulation (Li and Lin, 2008). Multipath is another challenging problem in this research area, where multiple reflections of the transmitted signal return via different special paths. However, recent advancements in directional antennas and beamforming can help to mitigate this effect by making direct path refections distinct from and multipath copies. Also, multipath signals mostly act as static clutter that can be removed in the RF front end, and also during postprocessing by bandpass filtering and removing the mean of the signal (Yang et al., 2020).
Artificial intelligence (AI) has been used by countries to support the fight against the viral pandemic affecting the entire world since the beginning of 2020 (John Hopkins Medicine, 2020; World Health Organization, 2020). China, the first epicenter of this disease, used authentication technology such as facial recognition to restrict the movement of the population which helped stop the spread of the virus (Reuters, 2020). However, video camera image-based approaches suffer from several irreconcilable dilemmas, including a lack of privacy and degraded performance under low light ambient conditions (Islam et al., 2020e). Besides, cybercrimes are increasing rapidly and thousands of coronavirus-related websites are subjects to attacks and abuse (Reuters, 2020). Phishing attacks through these websites can potentially be used to steal people’s passwords, ID, and social security card information. Thus, multi-factor authentication can also help to fight against this type of unprecedented situation during the COVID-19 pandemic (Block et al., 2020). Social distancing and good implementation of lockdown can help prevent the spread of the virus (Block et al., 2020). Thus, a Radar-based identity authentication system has certain potential advantages to overcome the challenges faced by users and the government of the country to implement lockdown properly.
Identity authentication using microwave Doppler radar is gaining interest and is another emerging potential field that researchers are exploring as an alternative to the camera and fingerprint-based biometric systems which have several privacy issues (Lin et al., 2017, Rahman et al., 2018). The existing biometric-based authentication system is far from satisfactory and not completely trustworthy, as it is a one-pass validation system (Lin et al., 2017). People must intentionally engage themselves, such as scanning a fingerprint or repeating their password after a certain interval of time (Lin et al., 2017). To replace or supplement traditional biometric systems, researchers have tried using different physiological traits, such as iris (Jiu et al., 2016), vein (Wang and Wang, 2017), eye movement (Lyamin and Cherepovskaya, 2017), keystroke dynamics (Pinto et al., 2014), and gaze pattern (Mock et al., 2012) to create multi-factor continuous authentication. All these proposed attempts require either the user’s intentional engagement with the authentication system or contact with the data acquisition sensors (Lin et al., 2017, Rahman et al., 2018). Considering all these limitations, unobtrusive forms of measurement for cardiac motion-based security systems are gaining more research interest in the search for new biometric authentication systems.
The basic idea of cardiopulmonary motion-based identity authentication exploits the fact that different people have differences in physical organ, size, shape, and muscle strength (Islam et al., 2020e). In addition, different people breathe differently in terms of their tidal volume, airflow profile, breathing depth, and energy (Benchetrit, 2000). The complete cardiac motion cycle consists of five different stages including 1) ventricular filling (VF) 2) atrial systole (AS), 3) isovolumetric ventricular contraction (IC), 4) ventricular ejection (VE), and 5) isovolumetric ventricular relaxation (IR) (Benchetrit, 2000). These cycles are significantly unique because of different volumes, surface shape, and moving dynamics (speed, acceleration, etc.), and deformation of the heart (Benchetrit, 2000; Islam et al., 2020e). It has also been demonstrated in various clinical investigations that no two persons have the same cardiac blood circulation heart (Benchetrit, 2000; Islam et al., 2020e).
Dynamic segmentation is a method that essentially evaluates the displacement and identifying points in the range of 30–70% amplitude of both inhale and exhale episodes, which defines four boundary points of a trapezium of the radar-captured respiration patterns (Rahman et al., 2018). The ratio of these two areas provides a useful feature that indicates how quickly the next cycle of inhalation starts (Rahman et al., 2018). Figure 6 illustrates the inhale/exhale area ratio features for two different subjects, which differ significantly. Based on these unique features, a k-nearest neighbor (KNN) algorithm was integrated to identify each person which showed a classification accuracy of almost 90% (Rahman et al., 2018). To increase the accuracy of the proposed method, the minor component analysis was performed on subject data sets which showed overlapping inhale and exhale area ratios. For extracting minor components, a linear demodulation technique is employed. The variations in minor components show the radar-cross section (RCS) and a high-frequency component of respiration and heart signal modulation. Higuchi fractal dimension analysis was performed on minor components of the radar captured signals to identify overlapped inhale/exhale area ratios of subjects, which increased the classification accuracy to 95% (Rahman et al., 2018). The proposed dynamic segmentation method clearly shows efficacy.
FIGURE 6. Respiratory pattern classifiers used for subject recognition. Dynamically segmented inhale/exhale area ratios of two subjects significantly differ, (A), as do signal patterns relating to the dynamics of breathing near the points where the inhale and exhale transition occurs, (B). From Rahman et al. (2018).
A study was conducted by a research group at the University of Buffalo on seventy-eight different participants for identity authentication (Lin et al., 2017). From the radar captured signal they extracted five different points which were hypothesized to fully represent cardiac motion. Based on this hypothesis, the experiment illustrated that heart-based geometry measures differ from person to person due to differences in size, position, and anatomy of the heart, chest configuration, and various other factors. From their experimental findings, it was also clear that no two subjects had the same heart, tissue, and blood circulation system, as there were significant differences in their cardiac cycle points measured in the radar data set (Lin et al., 2017). Figure 7 illustrates the cardiac motion marker for one segment captured from the radar respiration measurement. Within that segment from the five different points velocity and displacements were calculated and stored in the system for different participants. A support vector machine (SVM) with a radial basis function kernel classifier was employed to uniquely identify different participants. A study of 78 subjects was reported, and the proposed system achieved an accuracy of 98.61% and a 4.42% equal error rate. One of the limitations of the proposed system is that the complete study was performed with healthy sedentary persons. For further investigation, in another study, the feasibility of recognizing people after performing post-physiological activities was tested (Islam et al., 2020b). It was found that subject recognition still worked with an accuracy of 92% (Islam et al., 2019a; Islam et al., 2020b). Experimental results demonstrated that, after short exertion, dynamically segmented exhale area and breathing depth increased by more than 1.4 times for all participants, which made evident the uniqueness of the residual heart volume after expiration for recognizing each subject, even after short exertion (Islam et al., 2019a; Islam et al., 2020b). Furthermore, there was another investigation of identity authentication of patients with obstructive sleep apnea (OSA) symptoms based on extracting respiratory features (peak power spectral density, packing density, and linear envelop error) for radar captured paradoxical breathing patterns, in a small-scale clinical sleep study integrating three different machine learning classifiers (SVM, KNN, and random forest) (Islam et al.,2020). Their proposed OSA-based authentication method was tested and validated for five OSA patients with 93.75% accuracy, using a KNN classifier that outperformed other classifiers (Islam et al.,2020). A research group at MIT also published papers on emotion recognition from radar captured respiration patterns (Zhao et al., 2016).
FIGURE 7. Cardiac motion marker. The cardiac motion cycle defined by five different markers (red dots) at five different points of displacement and timing was calculated as a unique feature for recognizing people. From Lin et al. (2017).
The radar-based identity authentication approach is not a mature technology yet. However, all the reported research demonstrated the efficacy of the proposed radar captured cardiopulmonary-based identity authentication technique, which encourages further investigations. Thus, the emerging radar-based identity authentication approach can help to recognize people unobtrusively for implementing lockdown or tracking. The multi-factor authentication system described can thus be beneficial for reducing vulnerability to malicious activities (theft of personal information, ID, and social security) during this pandemic.
Radar is an attractive approach for estimating the number of occupants in a building and it is gaining research attention (Yavari et al., 2014). The detection of human cardiopulmonary motion using microwave Doppler radar can be a potential way of recognizing the number of occupants in a room (Yavari et al., 2014). Conventional occupancy sensors are typically motion sensors such as passive infrared (PIR) sensors (Abedi et al., 2019). A significant drawback of PIR sensors is the high rate of false detection rate as it only recognizes motion (Abedi et al., 2019). When subjects are stationary in a home environment, traditional occupancy sensors cannot determine the true presence of humans (Yavari et al., 2014). An alternative to the PIR sensor is video camera-based sensing technology (Abedi et al., 2019). However, video-image-based occupancy detection is accompanied by considerable privacy concerns (Abedi et al., 2019). The detection of human cardiopulmonary motion with Doppler radar could provide a promising approach to overcome the conventional sensor problems of false triggering and dead spots when humans are sedentary (Yavari et al., 2018).
Prior research efforts have demonstrated the efficacy of this technology to count the number of occupants in a home environment based on received signal strength (RSS) (Yavari et al., 2018). Radar reflected power at the receiving antenna can be described by the radar equation:
FIGURE 8. Radar-based subject presence detection and count. Experimental setup for radar measurement in a classroom, (A), filled with subjects for testing, (B), and raw radar data used for assessing presence, (C). From Yavari et al. (2018).
FIGURE 9. Experimental results with ten occupants in an indoor environment. The RSS of the received signal is significantly different for different numbers of occupants. From Yavari et al. (2018).
In another attempt, a 24-GHz K-LC3 wide-angle CW Doppler radar transceiver has been employed for detecting the occupancy state (vacancy/occupied) (Abedi et al., 2019). In their attempt, they investigated two different approaches, the first approach is a threshold-based decision algorithm and another one is a deep neural network-based approach. By utilizing the deep learning model, the need for specialized feature extraction is removed, because the task for feature extraction in a DNN model is performed automatically by the initial layers (Zhao et al., 2016). For the threshold-based approach, a threshold was predefined based on the amplitude of the radar-captured signal within the range of recorded signal amplitude (−1 V to +1 V). If the average signal amplitude for a certain window size of 10 s crosses the amplitude level, then this method can determine whether the room is occupied or vacant (Abedi et al., 2019). Figure 10 illustrates the output of the radar sensor signal where the blue line represents the occupied state, and the red line illustrates the unoccupied cases. For the case of the deep learning model, 10-s of sensor data is fed into the model wherein 500 of these cases, the room was unoccupied and in the other 500 cases room was occupied. The evaluation of the model was performed through 3-fold cross-validation. The threshold-based method has achieved an average accuracy of 84.3% (Abedi et al., 2019). On the other hand, the deep neural network model-based approach outperformed with an average accuracy of 98.9%. The experimental study clearly illustrates the efficacy of reliable and accurate occupancy detection and counting the number of people in a home environment.
FIGURE 10. Experimental results of radar-based occupancy state determination where blue line represents occupied and red line denotes unoccupied. From Abedi et al. (2019).
During this COVID-19 pandemic governments in different countries are struggling for implementing strict lockdowns. Occupancy detection using radar systems can help to track the number of occupants in a room and at the same time, and if some new people enter a room it can produce some alarm. Strict social isolation and quarantine can be better implemented by installing this intelligent radar-based physiological sensing system.
During this pandemic, a lot of people have been infected with COVID-19 by touching the contaminant surface (Kampf et al., 2020). In general, viruses and bacteria surviving on any surface for a long time could contaminate the interface and cause people’s health problems (Sun Y. et al., 2020). Radar-based hand gesture recognition may help people to interact with the system in a contactless way to reduce the risk of being infected with the virus in a public environment (Sun Y. et al., 2020). For example, recently tech giant Google has integrated a 60 GHz radar into the smartphone Pixel 4 which essentially allows users to control different buttons without touching the screen (Bohn, 2019). This non-contact hand gesture recognition is intrinsically hygienic because users need to touch much fewer surfaces or buttons. Additionally, radar sensors are preferred over cameras due to privacy-invasive reasons and are insensitive to ambient light (Sun Y. et al., 2020).
In one study, a 2.4 GHz CW radar system with a transmission power of 10.0 dBm was employed for hand gesture recognition (Sakamoto et al., 2018). The same antenna is used for transmitting and receiving, where the transmitting/receiving signals are isolated with a hybrid coupler. The received signal is mixed with the in-phase and quadrature-phase of the transmitted signal and then low-pass filtered with a cut of frequency 10 Hz. After analog to digital conversion in-phase (I) and quadrature (Q) signal is obtained with the sampling frequency of 1 kHz. The A/D converter is connected to the signal cable through dc coupling, and dc subtraction is also performed in the post-processing of the signal. The dc subtraction helps avoid distortion in I-Q plots, while still capturing even slow-moving respiration and heartbeat. This study was performed on ten different participants for recognizing six different hand gestures. The subjects were seated in front of the radar system with their arm approximately 120 cm from the radar antennas. For gesture recognition, they used the trajectory image of the I-Q plot of received signals. This I-Q plot signal not only changes in terms of the phase but also varies with the amplitude. The trajectory images are converted to a low-resolution JPEG image with a size of 30 pixels and then feed into a convolutional neural network (CNN) to recognize different hand gestures (Sakamoto et al., 2018). Figure 11 illustrates the experimental setup, radar I/Q plot associated JPEG images, and block diagram of the CNN architecture. The basic idea of having different orientations in the I-Q plot is due to the change in effective radar cross-section (ERCS) with the orientation of the hand gestures. ERCS changes with the shape, size of the target/subject (Snigdha et al., 2020). The input images with a size of
FIGURE 11. Radar-based hand gesture recognition. Experimental setup with subjects in seated position in front of the radar system in an anechoic chamber, (A), and example images of radar I/Q plots associated with gestures, (B), and block diagram of CNN used of pattern recognition, (C). From Sakamoto et al. (2018).
FIGURE 12. Range Doppler Image (RDI) and Range Angle Image (RAI) gesture recognition. Experimental results are shown for horizontal RDI, with palm swept from right to left (A), vertical RDI (B), horizontal RAI (C), and vertical RAI (D). Gesture recognition system network model is also shown, (E). From Yu et al. (2020).
Israel’s military radar system is being adapted to remotely monitor the vital signs of patients suffering from COVID-19 (TechRadar, 2019). The system uses an array of radar and optical sensors with which vital signs were measured and displayed on monitors for medical professionals (doctors and nurses) in a sterile environment to avoid direct contact for the risk of infection (No Camels Team Israel, 2020). The Israel defense company Elbit Systems and Vayyar, a tech company, are working together to advance this technology and have already demonstrated the efficacy of this non-contact remote respiration monitoring technology to fight against the COVID-19 pandemic (No Camels Team Israel, 2020). Additionally, they have also conducted an extensive clinical study with Rabin Medical Center to test the efficacy of radar-based non-contact breathing monitoring of COVID-19 patients at the point of diagnosis and during treatment (No Camels Team Israel, 2020). The goal of their project is to use non-contact sensing of respiration to help reduce the number of infections by helping medical personnel avoid close contact with patients.
In another attempt, MIT’s Computer Science and Artificial Intelligence Laboratory (CSAIL) in collaboration with the Boston clinical team also reported some promising results of remote sensing of respiration of COVID-19 patients (Adam, 2020). MIT developed a Wi-Fi-like box called Emerald which also uses reflected wireless signals in the environment and artificial intelligence to infer people’s vital signs, sleep, and movement (Adam, 2020). This signal emits roughly 1,000 times less radiation than a standard cell phone so there are no known additional health hazards for this technique (Adam, 2020). Additionally, with prior consent, they installed their device into a COVID-19 patient’s room to monitor respiration, demonstrating efficacy for monitoring breathing rate and heart rate remotely, confirmed with checks by medical professionals (Adam, 2020). Figure 13 illustrates the results of the recorded breathing rate of COVID-19 patients in their home environment. Remote monitoring products like Emerald developed by MIT could also help to expand healthcare facilities as the demand on hospital spikes with an increasing number of COVID-19 patients. Additionally, safe, remote, non-contact, and continuous breathing monitoring could also allow hospitals and physicians to triage less severe patients and monitor them in their own home, while still allowing them to respond quickly in case of aggravated illness. Many other research groups and industries are working hard to bring this potential sensor technology into the real world to combat COVID-19.
FIGURE 13. Radar measurement of COVID-19 affected breathing. A dataset of COVID-19 patient breathing recorded by MIT’s product clearly illustrates the feasibility of remote respiration sensing using radar to fight against COVID-19. From Adam (2020).
This COVID-19 pandemic has disrupted and impacted the lives of hundreds of thousands of people and continues to bring new challenges every day. During these times of uncertainty, high stress, and anxiousness, it is important to make supportive technology available to common people to at least minimize the spread of the virus and associated distress. Researchers and industry should work together to bring this remote sensing technology into real-world implementation, addressing functionality and production issues, to ease current life difficulties associated with precautions and isolation.
Radar is an attractive approach for sensing breathing rate and heart rate remotely, as installing this sensor technology in a home or hospital environment can help to reduce the risk of potential exposure from close contact with infected persons for measurement of their vital signs. Furthermore, tracking breathing rate and heart rate ubiquitously can provide screening for signs of respiratory distress and associated infection. For COVID-19 infected patients with less severe symptoms, a non-contact continuous breathing monitoring system can help them to self-monitor and respond quickly in case of aggravated illness or shortness of breath. At the same time, the radar system can be integrated with a telemedicine system to send respiration-related information to physicians so that the patients need not be worried about unnecessary emergency visits to the hospital.
For implementing lock-down and social isolation restrictions strictly, radar-based occupancy sensing can also provide potential help. Radar can determine the number of occupants in a room or a house. So, if there is an intruder or any inappropriate social gathering, it can objectively raise an alarm to make sure that people are following social isolation and quarantine rules strictly. Maintaining strict social isolation and quarantine can help to reduce the spread of the virus which also helps to reduce the fatality rate. For proper implementation of lockdown and travel bans, a radar-based ID system can potentially track a person if this type of sensor system is integrated with a cell phone. Many countries are struggling when visitors are traveling from an infected area. Many governments have imposed a 14-day quarantine rule for visitors. However, in reality, it is difficult to track each visitor, many of who are visiting for recreation. Thus, a radar-based unobtrusive recognition system can help to implement this type of quarantine rule more strictly so that the spread of the virus can be reduced. Radar-based automatic hand gesture recognition can also help to reduce the spread of the virus.
Over the last four decades, there has been significant improvement in Doppler radar-based physiological sensing technology. Many researchers continue to significantly improve the necessary hardware and signal processing techniques. This is high time for industry and researchers to work together to bring this sensor technology to the application for the benefit of humanity during this unprecedented COVID-19 pandemic.
In a nutshell, this paper summarizes recent advancements in different emerging applications of non-contact radar sensing technologies having relevance in the fight against COVID-19, including remote breathing monitoring, continuous identity authentication, occupancy sensing, and hand gesture recognition. It also discusses recently demonstrated techniques and their associated advantages in application areas relevant to COVID-19. Table 1 provides a summary analysis, comparing recently integrated algorithms and their accuracy to the established state of the art.
TABLE 1. Advancement in the latest technical methods with a comparative accuracy analysis for different radar-based applications.
SI contributed to the conception and design of the work. SI, FF contributed to the manuscript drafting. SI, FF, and VL contributed to the critical revision of the article, and approval for the final version of the article.
Research by SI and FF for writing this paper was not funded by any organization. During the writing of this paper VL was supported in part by the United States National Science Foundation under Grant No. IIS1915738.
The authors declare that the research was conducted in the absence of any commercial or financial relationships that could be construed as a potential conflict of interest.
Abedi, M., Jazizadeh, F., and Banff, A. B. (2019). “Deep-learning for occupancy detection using Doppler radar and infrared thermal array sensors,” in 36th international symposium on automation and robotics in construction, Banff, Canada, May 21, 2019. doi:10.22260/ISARC2019/0146
Adam, C.-S. (2020). CSAIL device lets doctors monitor COVID-19 patients from a distance. Available at: https://www.csail.mit.edu/news/csail-device-lets-doctors-monitor-covid-19-patients-distance (Accessed August, 2020).
Alwashmi, M. F. (2020). The use of digital health in the detection and management of COVID-19. Int. J. Environ. Res. Public Health 17, E2906. doi:10.3390/ijeprh17082906
Ayebare, R. R., Flick, R., Okware, S., Bodo, B., and Lamorde, M. (2020). Adoption of COVID-19 triage strategies for low-income settings. Lancet Respir. Med. 8, e22. doi:10.1016/s2213-2600(20)30114-4
Baboli, M., Singh, A., Soll, B., Boric-Lubecke, O., and Lubecke, V. M. (2015). Good night: sleep monitoring using a physiological radar monitoring system integrated with a polysomnography system. IEEE Microwave 16 (6), 34–41. doi:10.1109/MMM.2015.2419771
Baboli, M., Singh, A., Soll, B., Boric-Lubecke, O., and Lubecke, V. M. (2020). Wireless sleep apnea detection using continuous wave quadrature Doppler radar. IEEE Sensors J. 20 (1), 538–545. doi:10.1109/JSEN.2019.2941198
Benchetrit, G. (2000). Breathing pattern in humans: diversity and individuality. Respir. Physiol. 122 (2–3), 123–129. doi:10.1016/S0034-5687(00)00154-7
Block, P., Hoffman, M., Raabe, I. J., Dowd, J. B., Rahal, C., Kashyap, R., et al. (2020). Social network-based distancing strategies to flatten the COVID-19 curve in a post-lockdown world. Nat. Hum. Behav. 4 (6), 588–596. doi:10.1038/s41562-020-0898-6
Bohn, D. (2019). Google Pixel 4 and 4 XL handson: this time, it’s not about the camera. Available at: https://www.theverge.com/2019/10/15/20908071/google-pixel-4-xl-photos-video-hands-on-camera-screen-specs-price(Accessed November 4, 2020).
CNBC (2020). Google announces new Nest Thermostat that uses radar to detect when you’re nearby. Available at: https://www.cnbc.com/2020/10/12/google-announces-new-nest-thermostat-with-soli-radar.html (Accessed October 12, 2000).
Droitcour, A. D. (2006). Non-contact measurement of heart and respiration rates with a single chip microwave Doppler radar. Ph.D. dissertation. Stanford (CA): Stanford University.
Droitcour, A., Lubecke, V., Lin, J., and Boric-Lubecke, O. (2001). “A microwave radio for Doppler radar sensing of vital signs,” in 2001 IEEE MTT-S international microwave sympsoium digest (Cat. No. 01CH37157), Phoenix, AZ, May 20–24, 2001 (New York, NY: IEEE), 175–178. doi:10.1109/MWSYM.2001.966866
Fioranelli, F., Le Kernec, J., and Shah, S. A. (2019). Radar for health care: recognizing human activities and monitoring vital signs. IEEE Potentials 38 (4), 16–23. doi:10.1109/MPOT.2019.2906977
Grado, C., Islam, S. M. M., Lubecke, L. C., and Lubecke, V. (2020). “UAV radar sensing of respiratory variations for COVID-type disorders,” in IEEE Asia-pacific microwave conference (APMC’20). Hong Kong, China, 8–11 December 2020 (New York, NY: IEEE).
Gu, C., Wang, G., Inoue, T., and Li, C. (2013a). “Doppler radar vital sign detection with random body movement cancellation based on adaptive phase compensation,” in Proceedings of the 2013 IEEE MTT-S international microwave symposium digest (MTT), Seattle, WA, June 2–7, 2013 (New York, NY: IEEE), 1–3. doi:10.1109/MWSYM.2013.6697618
Gu, C., Wang, G., Li, Y., Inoue, T., and Li, C. (2013b). A hybrid radar-camera sensing system with phase compensation for random body movement cancellation in Doppler vital sign detection. IEEE Trans. Microwave Theory Tech. 61, 4678–4688. doi:10.1109/TMTT.2013.2288226
Guidance for Industry and Food and Drug Administration Staff (2020). Enforcement policy for non-invasive remote monitoring devices used to support patient monitoring during the coronavirus disease 2019 (COVID-19) public health emergency (revised). Available at: https://www.fda.gov/regulatory-information/search-fda-guidance-documents/enforcement-policy-non-invasive-remote-monitoring-devices-used-support-patient-monitoring-during (Accessed October, 2020).
Hafner, N., Mostafanezhad, I., Lubecke, V. M., Boric-Lubecke, O., and Host-Madsen, A. (2007). “Non-contact cardiopulmonary sensing with a baby monitor,” in 29th annual international conference of the IEEE engineering in medicine and biology society, Lyon, France, August 22–26, 2007 (New York, NY: IEEE), 2300–2302. doi:10.1109/IEMBS.2007.4352785
Islam, S. M. M., Yavari, E., Rahman, A., Lubecke, V. M., and Boric-Lubecke, O. (2018a). “Separation of respiratory signature for multiple subjects using independent component analysis with the JADE algorithm,” in 2018 40th annual international conference of the IEEE engineering in medicine and biology society, Honolulu, HI, July 17–21, 2018 (New York, NY: IEEE), 1234–1237. doi:10.1109/EMBC.2018.8512583
Islam, S. M. M., Yavari, E., Rahman, A., Lubecke, V. M., and Boric-Lubecke, O. (2018b). “Direction of arrival estimation of physiological signals of multiple subjects using phase comparison monopulse radar,” in 2018 Asia-Pacific microwave conference (APMC), Kyoto, Japan, November 6–9, 2018 (New York, NY: IEEE), 411–413. doi:10.23919/APMC.2018.8617539
Islam, S. M. M., Rahman, A., Prasad, N., Boric-Lubecke, O., and Lubecke, V. M. (2019a). “Identity authentication system using a support vector machine (SVM) on radar respiration measurements,” in 2019 93rd ARFTG microwave measurement conference (ARFTG), Boston, MA, June 7, 2019 (New York, NY: IEEE), 1–5. doi:10.1109/ARFTG.2019.8739240
Islam, S. M. M., Yavari, E., Rahman, A., Lubecke, V. M., and Boric-Lubecke, O. (2019b). “Multiple subject respiratory pattern recognition and estimation of direction of arrival using phase-comparison monopulse radar,” in 2019 IEEE radio and wireless symposium (RWS), Orlando, FL, January 20–23, 2019 (New York, NY: IEEE), 1–4. doi:10.1109/RWS.2019.8714272
Islam, S. M. M., Rahman, A., Yavari, E., Baboli, M., Boric-Lubecke, O., and Lubecke, V. M. (2020a). “Identity authentication of OSA patients using microwave Doppler radar and machine learning classifiers,” in 2020 IEEE radio and wireless symposium (RWS), San Antonio, TX, January 26–29, 2020 (New York, NY: IEEE), 251–254. doi:10.1109/RWS45077.2020.9049983
Islam, S. M. M., Sylvester, A., Orpilla, G., and Lubecke, V. M. (2020b). “Respiratory feature extraction for radar-based continuous identity authentication,” in 2020 IEEE radio and wireless symposium (RWS), San Antonio, TX, January 26–29, 2020 (New York, NY: IEEE), 119–122. doi:10.1109/RWS45077.2020.9050013
Islam, S. M. M., Motoyama, N., Pacheco, S., and Lubecke, V. M. (2020c). “Non-contact vital signs monitoring for multiple subjects using a millimeter wave FMCW automotive radar,” in 2020 IEEE/MTT-S international microwave symposium (IMS), Los Angeles, CA, August 4–6, 2020 (New York, NY: IEEE), 783–786. doi:10.1109/IMS30576.2020.9223838
Islam, S. M. M., Boric-Lubecke, O., and Lubekce, V. M. (2020d). Concurrent respiration monitoring of multiple subjects by phase-comparison monopulse radar using independent component analysis (ICA) with JADE algorithm and direction of arrival (DOA). IEEE Access 8, 73558–73569. doi:10.1109/ACCESS.2020.2988038
Islam, S. M. M., Borić-Lubecke, O., Zheng, Y., and Lubecke, V. M. (2020). Radar-based non-contact continuous identity authentication. Remote Sens. 12 (14), 2279. doi:10.3390/rs12142279
Jiu, F., Noronha, K., and Jayaswal, D. (2016). “Biometric identification through detection of retinal vasculature,” in 2016 IEEE first international conference on power electronics, intelligent control and energy systems (ICPEICES), Delhi, India, July 4–6, 2016, 1–5. doi:10.1109/ICPEICES.2016.7853731
John Hopkins Medicine (2020). Can coronavirus cause heart damage? Available at: https://www.hopkinsmedicine.org/health/conditions-and-diseases/coronavirus/can-coronavirus-cause-heart-damage (Accessed October 30, 2020).
Johns Hopkins University and Medicine (2020a). Coronavirus disease (COVID-19) Pandemic. Available at: https://www.who.int/emergencies/diseases/novel-coronavirus-2019 (Accessed October 30, 2020).
Johns Hopkins University and Medicine (2020b). Coronavirus disease. Available at: https://coronavirus.jhu.edu/map.html (Accessed October 30, 2020).
FDA (2009). Kai sensors receives FDA clearance for its wireless, non-contact respiratory device. Available at: https://www.meddeviceonline.com/doc/kai-sensors-receives-fda-clearance-for-its-0001 (Accessed June 17, 2009).
Kampf, G., Todt, D., Pfaender, S., and Steinmann, E. (2020). Persistence of coronaviruses on inanimate surfaces and its inactivation with biocidal agents. J. Hosp. Infect. 104, 246–251. doi:10.1016/j.jhin.2020.01.022
Kissler, S. M., Tedijanto, C., Goldstein, E., Grad, Y. H., and Lipsitch, M. (2020). Projecting the transmission dynamics of SARS-CoV-2 through the post-pandemic period. Science 368, 860–868. doi:10.1126/science.abb5793
Lee, Y. S., Pathirana, P. N., Steinfort, C. L., and Caelli, T. (2014). Monitoring and analysis of respiratory patterns using microwave Doppler radar. IEEE J. Transl. Eng. Health Med. 2, 1–12. doi:10.1109/JTEHM.2014.2365776
Li, C., and Lin, J. (2008). Random body movement cancellation in Doppler radar vital sign detection. IEEE Trans. Microwave Theory Tech. 56, 3143–3152. doi:10.1109/TMTT.2008.2007139
Li, C., Lubecke, V. M., Boric-Lubecke, O., and Lin, J. (2013). A review on recent advances in Doppler radar sensors for noncontact healthcare monitoring. IEEE Trans. Microwave Theory Tech. 61 (5), 2046–2060. doi:10.1109/TMTT.2013.2256924
Lin, F., Song, C., Zhuang, Y., Xu, W., Li, C., and Ren, K. (2017). “Cardiac scan: a non-contact and continuous heart-based user authentication system,” in Proceedings of the 23rd annual conference on mobile computing and communication, Snowbird, UT, October 16–20, 2017, 315–328. doi:10.1145/3117811.3117839
Lin, J. C. (1975). Noninvasive microwave measurement of respiration. Proc. IEEE 63 (10), 1530. doi:10.1109/proc.1975.9992
Lyamin, A. V., and Cherepovskaya, E. N. (2017). An approach to biometric identification by using low-frequency eye tracker. IEEE Trans. Inform. Forensics Secur. 12 (4), 881–891. doi:10.1109/TIFS.2016.2639342
Massagram, W., Lubecke, V. M., and Boric-Lubecke, O. (2009a). “Microwave non-invasive sensing of respiratory tidal volume,” in Annual international conference of the IEEE engineering in medicine and biology society, Minneapolis, MN, September 3–6, 2009, 4832–4835. doi:10.1109/IEMBS.2009.5332667
Massagram, W., Lubecke, V. M., Host-Madsen, A., and Boric-Lubecke, O. (2009b). Assessment of heart rate variability and respiratory sinus arrhythmia via Doppler radar. IEEE Trans. Microwave Theor. Techn. 57 (10), 2542–2549. doi:10.1109/TMTT.2009.2029716
Massaroni, C., Nicolò, A., Schena, E., and Sacchetti, M. (2020). Remote respiratory monitoring in the time of COVID-19. Front. Physiol. 11, 635. doi:10.3389/fphys.2020.00635
Mock, K., Hoanca, B., Weaver, J., and Milton, M. (2012). “Real-time continuous iris recognition for authentication using an eye tracker,” in Proceedings of the 2012 ACM conference on Computer and communications security–CCS’12, Raleigh, NC, October 1, 2012, 1007–1009. doi:10.1145/2382196.2382307
No Camels Team Israel (2020). Adapts military radar systems for remote COVID-19 patient monitoring. Available at: https://nocamels.com/2020/04/israel-military-radar-systems-remote-monitoring/ (Accessed November 1, 2020).
Pinto, P., Patrão, B., and Santos, H. (2014). “Free typed text using keystroke dynamics for continuous authentication,” in Advanced information systems engineering. Editors C. Salinesi, M. C. Norrie, and Ó. Pastor (Berlin, Heidelberg: Springer Berlin Heidelberg), Vol. 7908, 33–45.
Rahman, A., Lubecke, V. M., Boric-Lubecke, O., Prins, J. H., and Sakamoto, T. (2018). Doppler radar techniques for accurate respiration characterization and subject identification. IEEE J. Emerg. Sel. Top. Circuits Syst. 8 (2), 350–359. doi:10.1109/JETCAS.2018.2818181
Reuters (2020). China’s coronavirus campaign offers glimpse into surveillance system. Available at: https://www.reuters.com/article/us-health-coronavirus-china-surveillance/chinas-coronavirus-campaign-offers-glimpse-into-surveillance-system-idUSKBN2320LZ (Accessed September, 2020).
Ritchie, M., Jones, A., Brown, J., and Griffiths, H. D. (2017). “Hand getsure classification using 24 GHz FMCW dual polarised radar,” in International conference on radar systems (Radar 2017), Belfast, NIR, October 23–26, 2017, 1–6. doi:10.1049/cp.2017.0482
Sakamoto, T., Gao, X., Yavari, E., Rahman, A., Boric-Lubecke, O., and Lubecke, V. M. (2018). Hand gesture recognition using a radar echo I-Q plot and a convolutional neural network. IEEE Sens. Lett. 2 (3), 1–4. doi:10.1109/LSENS.2018.2866371
Shah, S. A., and Fioranelli, F. (2019). RF sensing technologies for assisted daily living in healthcare: a comprehensive review. IEEE Aerosp. Electron. Syst. Mag. 34 (11), 26–44. doi:10.1109/MAES.2019.2933971
Singh, A., Lee, S., Butler, M., and Lubecke, V. (2012). “Activity monitoring and motion classification of the lizard Chamaeleo jacksonii using multiple Doppler radars,” in Annual IEEE international engineering in medicine and biology society conference, San Diego, CA, August 28–September 1, 2012 (New York, NY: IEEE), 4525–4528. doi:10.1109/EMBC.2012.6346973
Singh, A., Lubecke, V., and Boric-Lubecke, O. (2011). “Pulse pressure monitoring through non-contact cardiac motion detection using 2.45 GHz microwave Doppler radar,” in Annual international conference of the IEEE engineering in medicine and biology society, Boston, MA, August 30–September 3, 2011 (New York, NY: IEEE), 4336–4339. doi:10.1109/IEMBS.2011.6091076
Singhal, T. (2020). A review of coronavirus disease-2019 (COVID-19). Indian J. Pediatr. 87 (4), 281–286. doi:10.1007/s12098-020-03263-6
Snigdha, F., Islam, S. M. M., and Boric-Lubecke, O. (2020). “Obstructive sleep apnea events classification by effective radar cross-section (ERCS) method by using microwave Doppler Radar and machine learning classifiers,” in IEEE MTT-S international microwave biomedical conference (IMBIOC’20). Toulouse, France, December 14–17, 2020 (New York, NY: IEEE).
Sun, Q., Qiu, H., Huang, M., and Yang, Y. (2020). Lower mortality of COVID-19 by early recognition and intervention: experience from Jiangsu Province. Ann. Intensive Care 10, 33. doi:10.1186/s13613-020-00650-2
Sun, Y., Fei, T., Li, X., Warnecke, A., Warsitz, E., and Pohl, N. (2020). Real-time radar-based gesture detection and recognition built in an edge-computing platform. IEEE Sensors J. 20 (18), 10706–10716. doi:10.1109/JSEN.2020.2994292
Taylor, W., Abbasi, Q. H., Dashtipour, K., Ansari, S., Shah, S. A., Khalid, A., et al. (2020). A review of the state of the art in non-contact sensing for COVID-19. Sensors 20 (19), 5665. doi:10.3390/s20195665
Techradar (2019). Google Pixel 5 needs the Pixel 4's most maligned feature, because of Covid-19. Available at: https://www.techradar.com/news/google-pixel-5-needs-the-pixel-4s-most-maligned-feature-because-of-covid (Accessed November 1, 2020).
Wang, J., and Wang, G. (2017). Quality-specific hand vein recognition system. IEEE Trans. Inform. Forensics Secur. 12 (11), 2599–2610. doi:10.1109/TIFS.2017.2713340
Wang, S., Song, J., Lien, J., Poupyrev, I., and Hilliges, O. (2016). “Interaction with Soli: exploring fine-grained dynamic gesture recognition in the radio-frequency spectrum”, in Proccedings of the 29th annual Symposium on user interface Software and technology. New York, NY, December 21, 2020, 851–860.
Wang, Y., Hu, M -H., Li, Q., Zhang, X. -P., Zhai, G., and Yao, N. (2020). Abnormal respiratory patterns classifier may contribute to large-scale screening of people infected with COVID-19 in an accurate and unobtrisve manner. ArXiv abs/2002.05534.
World Health Organization (2020). WHO clinical management of severe acute respiratory infection (SARI) when COVID-19 disease is suspected. Available at: https://www.who.int/publications/i/item/10665-332299 (Accessed October 30, 2020).
Yang, F., He, Z., Guo, S., Fu, Y., Li, L., Lu, J., et al. (2020). Non-contact driver respiration rate detection technology based on suppression of multipath interference with directional antenna. Information 11 (4), 192. doi:10.3390/info11040192
Yavari, E., Gao, X., and Boric-Lubecke, O. (2018). “Subject count estimation by using Doppler radar occupancy sensor,” in 40th annual international conference of the IEEE engineering in medicine and biology society, Honolulu, HI, July 18–21, 2018 (New York, NY: IEEE), 4428–4431. doi:10.1109/EMBC.2018.8513388
Yavari, E., Song, C., Lubecke, V., and Boric-Lubecke, O. (2014). Is there anybody in there?: intelligent radar occupancy sensors. IEEE Microwave Mag. 15 (2), 57–64. doi:10.1109/MMM.2013.2296210
Yu, J.-T., Yen, L., and Tseng, P.-H. (2020). “mmWave radar-based hand gesture recognition using range-angle image,” in IEEE 91st vehicular technology conference (VTC2020-Spring), Antwerp, Belgium, May 25–28, 2020 (New York, NY: IEEE), 1–5. doi:10.1109/VTC2020-Spring48590.2020.9128573
Zhang, S., Li, G., Ritchie, M., Fioranelli, F., and Griffiths, H. (2016). “Dynamic hand gesture classification based on radar micro-Doppler signatures,” in CIE international conference on radar (RADAR), Guangzhou, China, October 10–13, 2016 (New York, NY: IEEE), 1–4. doi:10.1109/RADAR.2016.8059518
Zhang, Z., Tian, Z., and Zhou, M. (2018). Latern: dynamic continuous hand gesture recognition using FMCW radar sensor. IEEE Sensors J. 18 (8), 3278–3289. doi:10.1109/jsen.2018.2808688
Keywords: radar remote sensing, identity authentication, gesture recognition, occupancy sensing, breathing
Citation: Islam SMM, Fioranelli F and Lubecke VM (2021) Can Radar Remote Life Sensing Technology Help Combat COVID-19?. Front. Comms. Net 2:648181. doi: 10.3389/frcmn.2021.648181
Received: 31 December 2020; Accepted: 08 February 2021;
Published: 17 May 2021.
Edited by:
Ahmad Alsharoa, Missouri University of Science and Technology, United StatesReviewed by:
Daniyal Haider, De Montfort University, United KingdomCopyright © 2021 Islam, Fioranelli and Lubecke. This is an open-access article distributed under the terms of the Creative Commons Attribution License (CC BY). The use, distribution or reproduction in other forums is permitted, provided the original author(s) and the copyright owner(s) are credited and that the original publication in this journal is cited, in accordance with accepted academic practice. No use, distribution or reproduction is permitted which does not comply with these terms.
*Correspondence: Shekh M. M. Islam, c2hla2hAaGF3YWlpLmVkdQ==
Disclaimer: All claims expressed in this article are solely those of the authors and do not necessarily represent those of their affiliated organizations, or those of the publisher, the editors and the reviewers. Any product that may be evaluated in this article or claim that may be made by its manufacturer is not guaranteed or endorsed by the publisher.
Research integrity at Frontiers
Learn more about the work of our research integrity team to safeguard the quality of each article we publish.