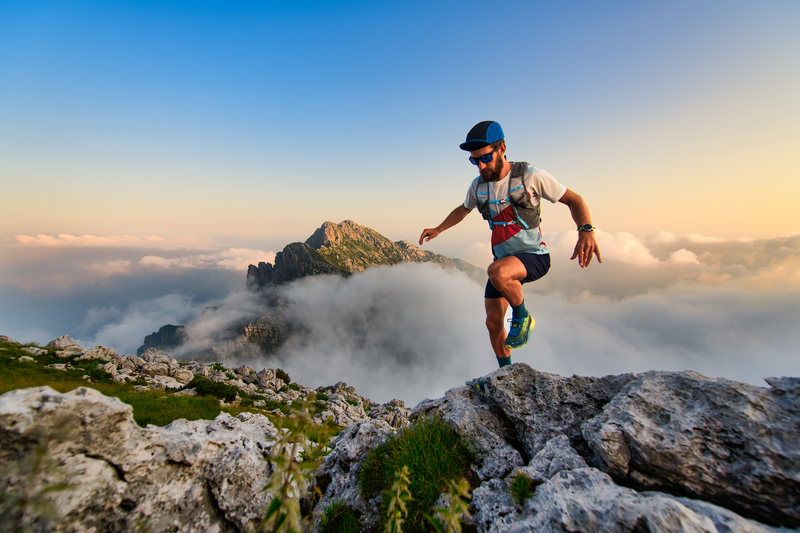
95% of researchers rate our articles as excellent or good
Learn more about the work of our research integrity team to safeguard the quality of each article we publish.
Find out more
ORIGINAL RESEARCH article
Front. Commun.
Sec. Health Communication
Volume 10 - 2025 | doi: 10.3389/fcomm.2025.1525899
This article is part of the Research Topic Health Misinformation: Examining Its Presence and Impact across Communication Contexts View all 7 articles
The final, formatted version of the article will be published soon.
You have multiple emails registered with Frontiers:
Please enter your email address:
If you already have an account, please login
You don't have a Frontiers account ? You can register here
Profiling channels for misinformation can assist platforms and researchers in understanding where and how misinformation spreads. Given the unique features of Telegram, such as enhanced encryption and privacy, the study intends to fill the gap in the existing literature by providing tailored methods and frameworks for analysing misinformation. This study aimed to explore the methodological challenges of collecting high-quality misinformation data on Telegram and developing and testing a conceptual model for identifying malicious content and channels. The research is structured into three main phases: a structured literature review to understand existing work on misinformation in Telegram, the development of a conceptual framework focusing on the features of creators/spreaders, message content, target victims and social context and testing the framework within the antivaccination community on Telegram using latent profile analysis (LPA). Data were collected from 151 anti-vaccination Telegram channels, resulting in 7,550 messages which were manually annotated and analysed. The latent profile analysis (LPA) and T-test results indicate distinct content-based patterns that differentiate malicious and non-malicious channels. These differences are primarily observed in crisis-related engagement, discourse manipulation techniques, and thematic focus.
Keywords: malicious channels, misinformation, antivaccination movement, Telegram platform, Latent Profile Analysis (LAT)
Received: 10 Nov 2024; Accepted: 26 Mar 2025.
Copyright: © 2025 Skarzauskiene, Maciuliene, Dirzyte and Guleviciute. This is an open-access article distributed under the terms of the Creative Commons Attribution License (CC BY). The use, distribution or reproduction in other forums is permitted, provided the original author(s) or licensor are credited and that the original publication in this journal is cited, in accordance with accepted academic practice. No use, distribution or reproduction is permitted which does not comply with these terms.
* Correspondence:
Aelita Skarzauskiene, Mykolas Romeris University, Vilnius, Lithuania
Disclaimer: All claims expressed in this article are solely those of the authors and do not necessarily represent those of their affiliated organizations, or those of the publisher, the editors and the reviewers. Any product that may be evaluated in this article or claim that may be made by its manufacturer is not guaranteed or endorsed by the publisher.
Research integrity at Frontiers
Learn more about the work of our research integrity team to safeguard the quality of each article we publish.