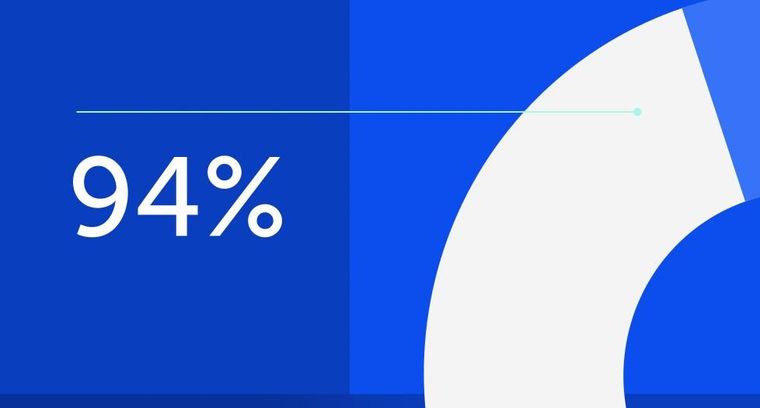
94% of researchers rate our articles as excellent or good
Learn more about the work of our research integrity team to safeguard the quality of each article we publish.
Find out more
ORIGINAL RESEARCH article
Front. Commun., 13 March 2025
Sec. Media Governance and the Public Sphere
Volume 10 - 2025 | https://doi.org/10.3389/fcomm.2025.1484186
This article is part of the Research TopicAI and CommunicationView all articles
Introduction: The current debate regarding artificial intelligence (AI) raises the question of whether AI-generated news articles on controversial topics can reduce news consumers’ hostile media perceptions (HMP). In addition, there is a debate about people’s prior attitudes toward AI and how it influences people’s perceptions of AI-generated news articles.
Methods: Based on the theoretical foundation of the MAIN model and the Hostile Media Phenomenon, we conducted two preregistered experimental studies in the United States (N = 1,197). All subjects were presented with a news article on a divisive and polarizing topic (gun regulation), but we systematically varied the supposed author of the article (human journalist, AI-generated, AI and human journalist working together).
Results and Discussion: In both studies exposure to the AI-generated news article significantly reduced participants’ HMP. However, the effect was only detected for individuals with negative and moderate prior attitudes toward AI. Individuals with positive AI attitudes did not benefit from AI-generated articles. AI-assisted news reporting showed very limited effects (only in Study 2). Furthermore, we examined if HMP predict online engagement. While Study 1 showed no effects on online engagement, Study 2 revealed that exposure to an AI-generated news article indirectly increased online engagement (intention to share a news article with friends and family). Implications for communication and journalism are discussed.
The development of generative artificial intelligence (AI) technology has brought about a significant shift in the way we interact with technology and people (e.g., Böhm et al., 2023) with implications for many fields, including journalism (e.g., Fletcher and Nielsen, 2024; Gil de Zúñiga et al., 2024). This shift is currently evident with regard to the global discussions around large language models such as OpenAI’s Chat GPT. In particular, the use of AI for language production raises the question about the possibility of AI replacing human reporters in journalism. For example, major media outlets such as The Washington Post and Bloomberg are developing AI tools to generate news articles or to assist journalists with writing news (Tandoc et al., 2020; The Washington Post, 2017). Furthermore, the Associated Press, which first began using AI for the creation of news content recently announced a partnership with OpenAI to further explore the use of AI in news (Reuters, 2023). In addition, news channels (e.g., Channel 1) are emerging that rely entirely on AI to create and present news (Robledo, 2023; see also Levy-Landesberg and Cao, 2024).
As scientific interest in AI-generated news increases (see Gil de Zúñiga et al., 2024), previous research has investigated how humans assess the reliability and credibility of news articles that have been automatically generated using algorithms (e.g., Graefe et al., 2018; Jia and Gwizdka, 2020; Wu, 2020). However, little attention has been devoted to assessing if and how AI can reduce people’s hostile media perceptions. In times of heightened political polarization (e.g., within the United States) (Pew Research Center, 2017; see Kubin and von Sikorski, 2021) and low levels of trust in news (Digital News Report, 2024), the hostile media phenomenon (Vallone et al., 1985) may be one theoretical account in understanding why people perceive quality news coverage as biased and unfair (Giner-Sorolla and Chaiken, 1994; Gunther and Liebhart, 2006; Gunther and Schmitt, 2004; Perloff, 2015). The hostile media phenomenon (also referred to as the hostile media effect) refers to the tendency of individuals with strong pre-existing attitudes to perceive media coverage as biased against their side and in favor of their opponents’ point of view. In their seminal study, Vallone et al. (1985) first described the phenomenon and investigated the effect experimentally to comprehend the variables influencing people’s assessments of fairness and perceived bias in media coverage. Their research showed that partisans viewed neutral or even-handed news coverage as biased against their side, whereas non-partisans perceived it as neutral.
Further research revealed that the source of information (e.g., a news brand) plays an important role in this context and can trigger hostile media perceptions (e.g., Arpan and Raney, 2003; Chia et al., 2007; Eveland and Shah, 2003; Giner-Sorolla and Chaiken, 1994) independently of the particular media content (e.g., Ariyanto et al., 2007; Gunther and Liebhart, 2006). For example, Gunther and Liebhart (2006) exposed partisans to the controversial issue of genetically modified food showing that the effect was absent when the article was (allegedly) authored by a student. However, they detected a strong hostile media effect when the author of the identical article was a journalist, illustrating the importance of the alleged source (i.e., author) of news for hostile media perceptions.
In recent decades this effect has been replicated multiple times in various news media contexts, in different cultural settings, and in connection with diverse topics (e.g., abortion, genetic modification of food, election campaigns, global warming; see Arpan and Raney, 2003; Feldman, 2011; Giner-Sorolla and Chaiken, 1994; Gunther and Chia, 2001; Gunther and Liebhart, 2006; Gunther et al., 2017; Gunther and Schmitt, 2004; Hartmann and Tanis, 2013; Kleinnijenhuis et al., 2020; Li et al., 2022; Matthes, 2013; Matthes et al., 2023; Perloff, 1989). In addition, a meta-analytical study showed that the effect is robust and produces small to moderate effects (Hansen and Kim, 2011).
But how can the dissemination of information through AI (rather than human journalists) influence hostile media perceptions (HMP)? Can AI reduce HMP, even for people with different prior attitudes toward AI? And can a news article supposedly generated by AI increase online engagement and people’s willingness to share a news article with others? These questions remain largely unanswered.
Based on the MAIN model (Sundar, 2008), initial research on the hostile media effect (Cloudy et al., 2021) and emerging research on the effects of exposure to AI-generated information (Böhm et al., 2023; Lermann Henestrosa et al., 2023), we theorized that a textual AI-generated news article regarding controversial (and polarizing) issues (e.g., gun regulation in the United States; e.g., see Kubin et al., 2023) may lead to reductions in HMP. The rationale behind this is based on seminal research revealing that people tend to regard news selected by a machine as more credible than news selected by a human journalist (Sundar and Nass, 2001).
Furthermore, we theorized that AI-assisted news coverage—AI and a human journalist working together (Tandoc et al., 2020; Waddell, 2019; Wölker and Powell, 2018)—may also be beneficial in dampening HMP, as AI is at least involved to a certain extent in the news production process.
However, a person’s prior attitude toward AI may be of key importance in this context (see Gambino et al., 2020). That is, people with positive prior attitudes toward AI may perceive AI-generated (or AI-assisted) news article as less hostile compared to people with more negative prior attitudes toward AI—an important aspect not considered in previous research.
To address these persisting research gaps, we conducted two experimental studies in the United States (Study 1: N = 408; Study 2: N = 789). We exposed participants to a factual and neutral news article (i.e., the article had no judgmental statements and a precisely weighed number of pro and con arguments) allegedly published in USA Today that addressed the controversial topic of gun regulation in the United States. Similar to previous research (Gunther et al., 2017), we used USA Today as a relatively moderate and neutral news source. All participants in both studies were given the same news article to read. However, the author of the news article was systematically varied between the experimental groups: (1) human journalist, (2) AI-generated, and (3) AI-assisted (AI and human journalist working together).
The results of both studies showed that exposure to the article purportedly written by AI significantly reduced participants’ HMP. However, the effect was evident only for individuals with negative and moderate prior attitudes toward AI, whereas individuals with positive prior attitudes toward AI did not benefit from AI. In contrast, AI-assisted news had no main effect on people’s HMP in both studies, but reduced HMP for people with positive prior attitudes toward AI in Study 2. Results of Study 2 further revealed that exposure to an AI-generated news article indirectly increased the willingness of people to share the article with friends and family (i.e., online engagement).
The increasing use of automation processes and artificial intelligence (AI) in the field of journalism has led to a great deal of attention and discussion in communication research (e.g., Fletcher and Nielsen, 2024; Suchman, 2023; see also Deuze and Beckett, 2022; Gil de Zúñiga et al., 2024). In line with Gil de Zúñiga et al. (2024), we generally understand AI in communication research “as the tangible real-world capability of non-human machines or artificial entities to perform, task solve, communicate, interact, and act logically as it occurs with biological humans” (p. 317). But how do people react to media content that has been automatically generated by machines and what are the implications for people’s hostile media perceptions?
Sundar (2008) developed the MAIN model to theoretically explain how individuals react to new media technologies and how people use new applications. In general, the MAIN model classifies digital media affordances into modality, agency, interactivity, and navigability, and assesses content credibility based on several heuristics triggered by the cues provided by the affordances (Sundar, 2008). Thus, the model describes how technology interfaces can trigger cognitive heuristics in media users, ultimately affecting the perceived reliability of media content (Sundar, 2008).
Based on this assumption, AI and new forms of automation in journalism can therefore fundamentally lead to people using cognitive heuristics to evaluate content. More specifically, AI-generated content can trigger heuristic processing in readers that leads them to believe that the information is free of bias simply because it was created or selected by a machine (Sundar and Nass, 2001; see Lermann Henestrosa et al., 2023). If the source is a machine, the model suggests that the so-called machine heuristic will be triggered in people, and news content will be perceived as objective and free of ideological bias (Sundar, 2008). This prediction was initially derived from the work of Sundar and Nass (2001), who observed that news purportedly selected by a machine was evaluated as more credible than news purportedly selected by a human journalist.
Therefore, machines are closely related to credibility, which is a key criterion to evaluate whether news properly reflects reality (Newhagen and Nass, 1989). Similar to the assessment of machines more generally, AI-generated news may thus be perceived as neutral and more objective than the reporting of human journalists (for initial findings on the effects of automated computer-generated news see Graefe et al., 2018; Reeves, 2016), who are regularly perceived as biased and partisan actors—by some news consumers (DellaVigna and Kaplan, 2007; Patterson and Donsbach, 1996). This may be particularly the case in times of high levels of political polarization and low levels of trust in news, especially in the United States (Digital News Report, 2024).
To better understand the effects of automated news, emerging research is experimentally investigating how people evaluate the credibility of news depending on the source of information and whether there are differences in perceptions of human journalists and computers. Results reveal that news attributed to a machine are perceived as more credible than news attributed to human journalists (Bellur and Sundar, 2014; Clerwall, 2014; Graefe et al., 2018; Waddell, 2019). In line with the MAIN model, removing human bias in the news production process, people tend to heuristically assume that machines are more objective than humans are (Graefe et al., 2018; Reeves, 2016; Sundar, 2008). However, recent findings also indicate that messages attributed to a human author are perceived as more credible than messages attributed to a machine (Graefe and Bohlken, 2020; Jia and Johnson, 2021). Furthermore, a recent meta-analytical study based on 121 experimental studies showed that AI agents were perceived as similarly persuasive as human agents (Huang and Wang, 2023).
Besides examining credibility assessments of news consumers in regard to automated journalism, initial studies have also explored how automated journalism impacts HMP. Cloudy et al. (2021) showed that HMP were indirectly dampened when people were exposed to a news story preview on Facebook (i.e., preview of a headline), allegedly authored by AI. Their results revealed that exposure to AI-authored previews of news stories positively affected AI attitudes (i.e., machine heuristics) and in turn decreased HMP. In general, these results suggest that AI-generated news can lead to a reduction in HMP under certain circumstances (see also Huang and Wang, 2023).
Cloudy et al. (2021) used a Facebook post on the topic of abortion as a stimulus in their study and exposed participants to a neutral headline (“8 Key Findings from Newly Released Report on Abortion”; for a similar approach using headlines see Jia and Liu, 2021). However, it is important to note that the article preview did not contain any further textual information such as pro or con arguments on the topic of abortion, as the participants only saw the headline of the article.
Previous studies on people’s HMP have typically used news articles with carefully weighed arguments (see Hansen and Kim, 2011), for example, pro-life or pro-choice arguments related to the topic of abortion (e.g., Hartmann and Tanis, 2013). The neutrality of such a journalistic article thus arises at the text level, so that individuals without strong preconceptions about a topic may conclude: after weighing the pro and con arguments, the article is neutral. This means that people without strong preconceptions perceive an article as relatively unbiased due to non-judgmental statements and a balanced number of pro and con arguments (see Hansen and Kim, 2011).
Since Cloudy et al. (2021) used a post consisting of a short neutral headline in their research, it remains unclear how individuals respond when they see a full news story reflecting different perspectives and arguments on an issue—content that is common in real news articles. That is, oftentimes key arguments and facts that are perceived to be in line or against one’s issue position or attitude vividly come to mind when arguments are explicitly stated in a news article—such information may trigger HMP (Giner-Sorolla and Chaiken, 1994; Gunther and Liebhart, 2006; Hansen and Kim, 2011; Perloff, 2015). Thus, as a first step, we explored if HMP can also be dampened when people are exposed to a typical news story with (carefully balanced) pro and con arguments on a controversial topic (i.e., gun regulation in the United States). Based on our theorizing we formulate the following hypothesis:
H1: Participants exposed to an AI-generated news article (i.e., full news story) will show a lower level of HMP compared to individuals exposed to a news article written by a human journalist.
In addition to AI-generated news (i.e., AI that writes an article in its’ entirety), journalists are increasingly turning to AI to write articles collaboratively. For example, major media outlets such as The Washington Post and Bloomberg developed AI tools to assist human journalists in generating news articles (Tandoc et al., 2020; The Washington Post, 2017). Other media outlets, including The Guardian and Reuters, have also experimented with various AI systems to help human journalists create news content (Peiser, 2019; Van Dalen, 2012), and the Associated Press recently began a partnership with OpenAI to use AI for news production (Reuters, 2023). Even though many newsrooms still produce news following traditional practices and local news outlets are still at the beginning of integrating AI into workflows (Beckett and Yaseen, 2023), AI tools are becoming more and more prevalent. The rationale behind this is that AI can improve efficiency by compiling key facts and data quickly—freeing up time for journalists to complete other tasks.
It remains unclear so far what effects AI-assisted news coverage has on people’s HMP. Therefore, this study aims to fill the gap by investigating whether news stories co-authored by an AI and a human journalist can also dampen HMP. Initial research has shown mixed results for AI-assisted authorship. Waddell (2019) found that AI-assisted authorship reduced perceived media bias and indirectly increased perceived article credibility. However, in the same statistical model Waddell (2019) also shows a separate mediating pathway. That is, AI-assisted news reduced source anthropomorphism (i.e., the AI author is perceived as less human-like) compared to a sole human author. Further, AI-assisted authorship negatively affected perceived article credibility. Additionally, Jia and Liu (2021) found that AI-assisted news headlines were perceived as less credible compared to news headlines written solely by human authors.
Initial research sheds some light on the impact of AI-assisted news coverage on HMP, but the results are preliminary (due to the low number of studies) and inconsistent. Therefore, further research is needed to clarify how news consumers respond to articles co-authored by AI and human journalists and how such articles influence HMP. Since the available research does not allow us to make assumptions about the differences between AI-assisted and AI-written articles (without human involvement) with respect to HMP, we formulate the following research question.
RQ1: In what way does exposure to AI-assisted news affect individuals’ HMP?
A possible explanation for some contradictory results regarding the question of whether AI-generated news is perceived as credible could be related to specific preconceptions about AI. That is, individuals are developing more differentiated attitudes toward AI—in times of large language models such as Chat GPT—and that some news consumers do not automatically consider machines programmed by (potentially biased) humans to be more credible than human journalists (see Gambino et al., 2020). Specifically, people with negative prior attitudes toward AI may react more negatively to AI-generated news (vs. human-written articles).
Building on initial previous studies (e.g., Oh and Park, 2022; Wischnewski and Krämer, 2021), we theorized that people’s prior attitudes toward AI will moderate the effect of AI-generated messages on people’s HMP, which is generally consistent with recent theoretical assumptions. In general, AI attitudes have been shown to predict perceptions of specific machine agents (Nomura et al., 2006). Wischnewski and Krämer (2021) recently showed that AI attitudes are key for trust perceptions in regard to news and that persons with more positive AI attitudes judged AI news more trustworthy. Based on these results, we theorized that people with more positive attitudes (vs. negative attitudes) toward AI will show lower levels of HMP when exposed to AI-generated news and AI-assisted news coverage (vs. news by a human journalist). More formally, we formulate our second hypothesis (H2):
H2: Positive prior attitudes toward AI reduce HMP for individuals exposed (a) AI-authored and (b) news authored by AI and a human journalist (AI-assisted) compared with news authored by a human journalist.
Different authors (i.e., a human journalist or AI) could theoretically influence HMP as outlined above, but do HMP subsequently predict online engagement? Previous research has not systematically examined how HMP affect users’ online engagement. Online engagement can take many forms (Ksiazek et al., 2016), but posting information on social media, sharing information with friends and family, and commenting on messages are the most common forms. It is important to better understand online engagement in this context because online engagement determines whether platforms will further spread content to other users. That is, are messages shared and commented on more often when they are written by an AI or created in collaboration with an AI? So far, it remains unknown if HMP increase or decrease online engagement or, if HMP are not a relevant predictor for online engagement. Therefore, the first step is an exploratory investigation into the extent to which HMP predicts online engagement. Since there is no corresponding research available so far, we formulated the following research question:
RQ2: In what way(s) do HMP predict online engagement (posting, sharing and commenting)?
To test hypotheses 1 and 2, we conducted an online experiment (between-subjects design) in United States. Study 1 was preregistered (N = 408) and was conducted in September 2022. Study 2 (N = 789) was also preregistered, conducted in November 2022 and aimed to conceptually replicate Study 1. Thus, there was a time gap between the studies of about 2 months (i.e., in order to first evaluate the data from Study 1 and, for example, to check whether the scales for measuring the central constructs are reliable, which was the case). Data, analytic materials, and preregistrations (for both studies) are available at https://osf.io/qwzak/?view_only=fb1554d0597b43d98dfa9f4491c3c97a. Ethics approval was received from (Department of Psychology, University of Kaiserslautern-Landau (RPTU)).
In total, 507 American participants were recruited via Amazon MTurk. We chose the topic of gun regulation—a highly divisive topic in the United States e.g., (see Pew Research Center, 2021)—to test HMP of individuals with pro-gun regulation and anti-gun regulation attitudes. To recruit participants with pro-gun and anti-gun attitudes, we sampled individuals by political affiliation (i.e., Democrats and Republicans) as it is not possible to recruit people with pro-gun and anti-gun attitudes directly on MTurk. We anticipated there would be less people with pro-gun attitudes in our sample (as MTurk samples tend to be more left-leaning (Levay et al., 2016; see also Pew Research Center, 2021) and thus more likely to be anti-gun). Research revealed that liberals tend to be more supportive of gun regulation laws compared to conservatives in the United States (Oraka et al., 2019). Therefore, we oversampled Republicans (i.e., 60% Republicans and 40% Democrats) to attempt to collect similar numbers of people with pro-gun and anti-gun attitudes. Individuals that did not pass the manipulation check were excluded, a pre-registered decision. This resulted in a total sample of 408 participants (230 female [56.4%], 178 males [43.6%]; Age M = 42.14, SD = 12.88, Range = 19–78 years). Fifty-four percent of the participants reported having a Bachelor’s degree or above.
First, participants completed two items regarding their stance on gun regulation in the United States. Then they responded to our moderator variable (i.e., attitude toward AI) before encountering the experimental stimuli (news articles) to ensure valid measurement of individual’s pre-existing attitudes toward AI. Similar to previous research in the field (Haim and Graefe, 2017; Jia, 2020), we then exposed participants to general information about AI-generated news explaining that news organizations in the United States recently began to use AI-generated news and AI-assisted news besides regular news articles written by human journalists. Also, participants were informed that the news site USA Today uses AI named JAMIE (a gender-neutral name that also includes the letters “A” and “I” spelled as JAmIe in the article, see Appendix A).
Next, participants were randomly assigned to one of three groups. In all conditions, participants were exposed to an identical news article by USA Today “The pros and cons of gun regulation in the United States” (see Appendix A). Importantly, all articles were identical except for the byline where we systematically varied who the author was. Participants in Group 1 were exposed to the news article purportedly written by AI (JAMIE, n = 151). Group 2 participants were exposed to the article purportedly written by AI and a human journalist (JAMIE and Charlie Miller, n = 132; AI-assisted condition). Group 3 participants were exposed to the article purportedly written by a human journalist (Charlie Miller, n = 125). Importantly, all participants were exposed to the identical news article, which was based on factual information and was written in a strictly neutral manner; i.e., the same number of pretested pro and con arguments (3 pro, 3 con) were presented and the article only differed in regard to who the author was—which was mentioned in the byline (see Appendix A).
Individuals then completed other survey items including the dependent variable and a manipulation check. The survey software was programmed so that a participant was exposed to a news article for at least 30 s before being able to continue with the survey. Participants then answered several items including our dependent variable, demographic information, and control variables. The participants were then thanked for their participation and debriefed.
All items can be found in Appendix B. As in previous research on HMP (e.g., Gunther and Liebhart, 2006), we first determined a participant’s stance on gun regulation using two items adapted from previous research (Kubin et al., 2021) (e.g., “Do you think there should be more gun regulations in the United States?”) on a 6-point Likert scale ranging from 1 = “Strongly Disagree” to 6 = “Strongly Agree” (M = 4.19, SD = 1.76; rSpearman-Brown = 0.98) to classify them as gun regulation supporters (n = 271) or gun regulation opponents (n = 137). Second, we measured participants’ prior attitude toward AI using four items (e.g., “Artificial intelligence will benefit humankind”; 1 = Agree, 7 = Disagree; M = 4.34, SD = 1.13; Cronbach’s α = 0.763) based on Sindermann et al. (2022).
Next, we measured participants’ HMP, our dependent variable. Similar to previous research (Arpan and Raney, 2003; Gunther and Schmitt, 2004), we measured perceived author bias using a 7-point scale ranging from 1 (Strongly Disagree) to 7 (Strongly Agree) with two items (e.g., “Do you think this article was written by an author who opposes gun regulations?”) and created a score out of the two items (M = 3.61, SD = 1.40).
Furthermore, we measure audience engagement with the help of three items on a 7-point scale (1 = Strongly Agree, 7 = Strongly Disagree). Participants reported their willingness to leave a comment under the article (M = 3.14, SD = 1.94), share the article with friends and family (M = 3.30, SD = 1.97), and posting the article on their personal social media (M = 2.75, SD = 1.86). Also, we measured political orientation (1 item; 1 = Very Liberal to 7 = Very Conservative; M = 4.19, SD = 2.09), and party identification (2 items; 1 = Not at all to 7 = Extremely; Republican, M = 3.81, SD = 2.32, Democrat, M = 3.44, SD = 2.29).
To test the hypotheses, we first dummy coded the two AI conditions (AI-generated condition and AI-assisted condition) with the human author condition as the reference group. The results revealed that exposure to the AI-generated article had a significant and negative effect on people’s HMP (b = −0.53, SE = 0.17, p = 0.001) compared to the human author condition. This supports H1 indicating that the AI-generated news article (vs. news authored by a human journalist) reduced individuals’ HMP. Answering RQ1, the results did not show a significant effect on participants’ HMP in the AI-assisted condition (b = 0.07, SE = 0.17, p = 0.67) compared to the human author condition.
To test the anticipated moderation effect, we conducted a moderation analysis using the PROCESS macro in R (Hayes, 2013; Hayes and Matthes, 2009; Model 1). The prior AI attitudes variable was mean centered prior to computation. Also, 95% bias-corrected bootstrap confidence intervals based on 5,000 bootstrap samples were used for statistical inference (Hayes, 2013; Hayes and Matthes, 2009). The results revealed a significant interaction effect between the AI-generated news article condition and attitude toward AI on participants’ HMP (b = 0.24, SE = 0.12, p = 0.045). Further, we examined this significant moderation effect at different levels of the moderator using the pick-a-point approach (the 16th, 50th, and 84th percentiles of the moderator) (Hayes and Matthes, 2009).
The findings revealed that the AI-generated news article only affected individuals with a negative prior attitude toward AI (b = −0.80, SE = 0.21, 95% (CIs) [−1.21, −0.38]) and persons with a moderate AI attitude (b = −0.49, SE = 0.17, 95% (CIs) [−0.82, −0.17]). In contrast, individuals with a positive prior attitude toward AI were not affected (β = −0.25, SE = 0.22, 95% (CIs) [−0.68, 0.18]). Taken together, the results reveal a significant moderation effect, as predicted in H2a. However, the effect emerged in the opposite direction of predictions. Thus, H2a is not supported.
Next, we conducted a moderation analysis to examine a potential interaction effect between the AI-assisted condition and attitude toward AI on participants’ HMP. However, the results revealed no significant effect indicating that prior AI attitudes did not affect participants’ HMP (b = −0.17, SE = 0.13, p = 0.20) (see Figure 1). H2b was thus rejected.
Figure 1. Study 1 (N = 408). Moderation model, unstandardized path coefficients. Bold lines indicate significant effects. Significance code: **p < 0.01.
Next, we tested whether an individual’s HMP affected online engagement (RQ2). HMP was modeled as a mediator variable, prior AI attitudes were included as a stage 1 moderator (as in the previous analysis) and online engagement (commenting, sharing, posting) as the dependent variable (we ran a separate model for each variable). Answering RQ2, we conducted a conditional process model (PROCESS macro in R, Model 7), which showed non-significant findings for all three dependent variables both for the AI-generated and the AI-assisted conditions on online engagement through HMP (see Figure 2). Also, no direct effects on any of the engagement variables were detected. Thus, HMP did not predict online engagement.
Figure 2. Study 1 (N = 408). Conditional process model, unstandardized path coefficients. Bold lines indicate significant effects. Significance codes: ***p < 0.001; **p < 0.01.
The results of Study 1 reveal that exposure to an AI-generated news article decreased individuals’ HMP, although preconceptions about AI did have an influence. This finding generally supports and extends previous research (Cloudy et al., 2021; Graefe et al., 2018; Liu and Wei, 2019; Waddell, 2019; Wu, 2020). In contrast, people in the AI-assisted condition did not show lower levels of HMP compared to the human author condition. This result supports and extends previous findings (Jia and Liu, 2021; Waddell, 2019) suggesting that as soon as a journalist is actively involved in the news creation process the dampening effect on HMP disappears. One explanation for this could be that individuals may have the perception that a journalist is selectively using information provided by AI, while neglecting information that is not in line with his or her expectations. However, this is only one possible explanation and it should be determined whether this effect can be replicated. Quantitative and qualitative studies should explore this possibility further.
Furthermore, and as theorized, we detected a significant moderation effect. However, the direction of the moderation was different than expected. That is, people with positive prior attitudes toward AI were not affected, while the dampening effect on HMP was detected for moderates and people with negative attitudes toward AI in the AI-generated news condition. This effect was surprising, but may be explained with the help of expectancy violation theory (Burgoon and Le Poire, 1993). In essence, the theory predicts that violations of expectations in communication situations are sometimes preferred by individuals, compared to confirmations of expectations. Importantly, the theory differentiates between positive and negative violations (Burgoon and Le Poire, 1993). Transferred to the context of the present study, people with negative and moderate AI attitudes did not expect AI to be able to write such a professional news article. Their expectation was thus exceeded. The positive violation of the expectation may then have transferred to the AI author (i.e., JAMIE) and reduced HMP. In contrast, no expectations were violated for individuals with positive attitudes and thus there was no effect on HMP. Future research should examine this possibility in depth.
In summary, Study 1 showed that an allegedly AI-generated news article reduced HMP. This effect was moderated by negative and moderate attitudes toward AI. In contrast, exposure to news (allegedly) authored by a journalist and AI working together (AI-assisted) did not affect people’s HMP. Furthermore, HMP did not predict online engagement (commenting, sharing, posting). Yet, it remains unclear how robust these results are and whether they can be replicated.
Study 2 was an online experiment (between-subjects design) that had a goal of conceptually replicating the findings of Study 1.
For Study 2, a total of 1,018 American participants were recruited from MTurk. Similar to Study 1, we oversampled Republicans with a goal of recruiting similar numbers of pro- and anti-gun participants. This time, however, we slightly adjusted our recruitment strategy compared to Study 1 to further optimize the number of people with a pro- and anti-gun regulation stance during recruitment. Thus, in Study 2, we selected 500 Republicans and 500 participants without any specific ideological criteria, this means that the latter 500 participants were recruited from across the ideological spectrum (e.g., Republicans, Democrats, Independents, etc.).
Similar to Study 1, people who did not pass the manipulation check and who were unable to correctly identify the author of the news article were eliminated from the dataset resulting in a total sample of 789 participants (447 female [56.7%], 337 males [42.7%], 5 others [0.6%]; Age M = 42.76, SD = 13.04, Range = 18–79); 54.4% reported having a Bachelor’s degree or above. A total of 471 participants had anti-gun attitudes and 318 participants had pro-gun attitudes (M = 3.90, SD = 1.77).
In Study 2, we used the same items as in Study 1 (see Appendix B). Again, we first determined a participant’s stance on gun regulation with two items on a 6-point Likert scale ranging from 1 = “Strongly Disagree” to 6 = “Strongly Agree” (M = 3.90, SD = 1.77; rSpearman-Brown = 0.97) to classify participants as gun regulation supporters (n = 471) or gun regulation opponents (n = 318). Second, we again measured participants’ prior attitude toward AI using four items (1 = Agree, 7 = Disagree; M = 4.03, SD = 1.17; Cronbach’s α = 0.783). Participants’ HMP were measured by using a 7-point scale ranging from 1 (Strongly Disagree) to 7 (Strongly Agree) with two items that were again combined to a score (M = 2.64, SD = 1.39). Furthermore, we measure audience engagement with the help of three items on a 7-point scale (1 = Strongly Agree, 7 = Strongly Disagree). Participants reported their willingness to leave a comment under the article (M = 3.13, SD = 1.85), share the article with friends and family (M = 3.32, SD = 1.91), and posting the article on their personal social media (M = 2.74, SD = 1.83). Also, we measured political orientation (1 item; 1 = Very Liberal to 7 = Very Conservative; M = 4.53, SD = 1.85), and party identification (2 items; 1 = Not at all to 7 = Extremely; Republican, M = 4.04, SD = 2.18, Democrat, M = 2.89, SD = 1.99).
First, the results revealed a significant and negative effect of the AI-generated news article condition on participants’ HMP (b = −0.38, SE = 0.12, p = 0.003, 95% (CIs) [−0.61, −0.15]), compared with the human author condition. In line with the findings from Study 1, this supports H1.
Second, we examined effects of the AI-assisted condition (RQ1). In line with Study 1, we did not find a main effect of the AI-assisted condition on HMP (b = −0.14, SE = 0.13, p = 0.528; 95% (CIs) [−0.39, −0.11]) compared with the human author condition.
Next, we examined the moderating role of participants’ prior AI attitudes using the PROCESS macro in R (Hayes, 2013; Model 1). As in Study 1, AI attitude was mean centered prior to computing the product. Again, 95% bias-corrected bootstrap confidence intervals were used based on 5,000 bootstrap samples for statistical inference (Hayes, 2013; Hayes and Matthes, 2009).
The results showed a significant interaction effect between AI attitudes and the AI-generated news article condition (b = 0.32, SE = 0.09, p < 0.001) (human author condition as the reference group). Using the pick-a-point approach (the mean and ± 1 SD from the mean) (Hayes and Matthes, 2009), we found that the AI-generated article affected people with negative AI attitudes (b = −0.71, SE = 0.15, 95% (CIs) [−0.99, −0.42]) and people with moderate AI attitudes (b = −0.39, SE = 0.12, 95% (CIs) [−0.62, −0.16]) (see Figure 3). No effect was detected for individuals with positive attitude toward AI (β = 0.01, SE = 0.16, 95% (CIs) [−0.30, 0.32]). Replicating the findings of Study 1, this indicates that people with negative and moderate AI attitudes showed lower levels of HMP when AI (allegedly) authored the news article. Although the results reveal a significant moderation effect, as predicted in H2a, the effect again emerged in the opposite direction. H2a is therefore not supported.
Figure 3. Study 2 (N = 789). Moderation model, unstandardized path coefficients. Bold lines indicate significant effects. Significance code: **p < 0.01.
In the next step, we examined the moderation effect of prior AI attitudes on HMP for people exposed to the AI-assisted condition. Results revealed a significant interaction effect (b = −0.26, SE = 0.09, p = 0.003, 95% (CIs) [−0.43, −0.09]). Again, we used the pick-a-point approach (the 16th, 50th, and 84th percentiles of the moderator) (Hayes and Matthes, 2009), which showed that the AI-assisted condition did not affect people with negative AI attitudes (b = 0.13, SE = 0.16), 95% (CIs) [−0.18, 0.44]), nor people with moderate AI attitudes (b = −0.13, SE = 0.13), 95% (CIs) [−0.38, 0.11]). However, people with positive attitudes toward AI (b = −0.46, SE = 0.16), 95% (CIs) [−0.78, −0.15]) were affected. These results partially support H2b indicating that positive prior AI attitudes result in lower levels of HMP for people exposed to the AI-assisted condition.
Next, we again tested whether an individual’s HMP affected engagement (RQ2). First, the results revealed that HMP significantly predicted sharing intentions (b = −0.15, SE = 0.05, p = 0.003).
Second, we conducted a conditional process analysis (PROCESS macro in R, model 7), which showed that exposure to AI-authored news (vs. the human author condition) indirectly increased sharing intentions via lower levels of HMP (index of moderated mediation: (b = −0.05, SE = 0.02), 95% (CIs) [−0.10, −0.01]). Thus, the results indicate that the AI-generated news article reduced HMP in individuals with negative and moderate prior AI attitudes and, in turn, significantly predicted sharing intentions for these individuals (see Figure 4). However, similar to the results of Study 1, no effects were detected for commenting and posting intentions. Also, no direct effects of condition on commenting, sharing, nor posting were detected.
Figure 4. Study 2 (N = 789). Conditional process model, unstandardized path coefficients. Bold lines indicate significant effects. Significance code: **p < 0.01.
Finally, we re-ran the conditional process model with the AI-assisted condition (vs. the human author condition; PROCESS macro, model 7), which showed a significant indirect effect for people with positive prior attitudes toward AI (index of moderated mediation: b = 0.04, SE = 0.02, 95%, CIs [0.01, 0.09]) on sharing intentions.
Study 2 was a conceptual replication of Study 1. In line with H1 and the results of Study 1, participants exposed to an AI-generated news article showed a lower level of HMP. Thus, this result was directly replicated. Furthermore, similar results emerged for the moderation effect. That is, prior AI attitudes once again moderated the effect of HMP. While people with positive prior AI attitudes were not affected, people with negative and moderate AI attitudes showed significantly lower levels of HMP when exposed to the news article (allegedly) authored by AI. Thus, the interaction effect that was detected in Study 1 was replicated in Study 2.
One finding differed between Study 1 and Study 2. In Study 2, we detected a significant interaction effect (absent in Study 1) in the AI-assisted condition (vs. the human author condition). That is, people with positive prior AI attitudes showed reduced levels of HMP. Although our hypothesis (H2b) was partially confirmed in Study 2, it could be that the AI-assisted condition generally produces less consistent results (compared to the AI author condition) and that human involvement in the creation of news is judged more or less critically depending on the sample. This was also reflected in the previous results on AI-assisted news in the literature (e.g., Jia and Liu, 2021; Waddell, 2019).
Possibly, specific sample characteristics also had an impact on sharing intentions in Study 2. A comparison of the mean values for participants’ HMP showed clear differences between the two studies (Study 1: M = 3.63, Study 2: M = 2.66). Thus, in Study 2, the overall lower mean value in HMP may have influenced the willingness of individuals both in the AI-generated news article condition and the AI-assisted condition to share the article. That is, lower HMP led to a higher willingness to share the article with friends and family. Further experimental research is needed to explore this possibility.
Our research explores whether an AI-generated news article can mitigate hostile media perceptions. Based on the MAIN model (Sundar, 2008), we conducted two experimental studies. The results of both studies confirm and extend findings of previous research (Cloudy et al., 2021; Graefe et al., 2018; Oh and Park, 2022; Waddell, 2019) and show that journalistic articles purportedly written by AI mitigate HMP. However, this effect is only evident for individuals with negative and moderate prior attitudes toward AI and not for people with positive prior AI attitudes.
This is a novel finding that adds to our understanding regarding how people with different dispositions are affected by AI-generated news articles and shows important boundary conditions that should be considered (see Gambino et al., 2020). This finding is also in line with recent research (Lermann Henestrosa et al., 2023) and meta-analytic results showing that AI agents are perceived overall as being as persuasive as human agents (Huang and Wang, 2023) and that specific prior AI attitudes play a key role in this context (Gambino et al., 2020), making AI effective agents that have the power to reduce HMP.
However, this dampening effect on HMP reverses once a human journalist intervenes in the news creation process. In Study 1, all participants exposed to AI-assisted news showed higher levels of HMP. In Study 2—possibly because of sample characteristics—only people with positive prior attitudes toward AI exhibited lower levels of HMP when exposed to the AI-assisted condition. This finding adds to the (so far, partly inconclusive) literature on effects of AI-assisted news coverage (see Bussone et al., 2015; Jacobs et al., 2021; Jia and Liu, 2021; Waddell, 2019) and shows the need for further research investigating the effects of journalist and AI collaboration.
Furthermore, we examined for the first time if people’s (dampened) HMP (triggered by AI-generated news) predict the likelihood of online engagement in news consumers. We find some evidence that people were indeed indirectly more willing to share an article with family and friends when they believed that the article was AI-generated (Study 2). Although, this finding should be interpreted with caution, as the indirect effect of HMP on sharing intentions is correlational in nature, future research should examine if AI-generated news can increase online engagement in news consumers.
The present study opens several new avenues for future investigation. Future research should further examine how specific prior AI attitudes affect people’s reaction to new AI technology and AI-generated news more specifically.
Knowledge of and attitudes about AI technologies is rapidly changing and expanding since the introduction of OpenAI’s GPT and other large language models (Fletcher and Nielsen, 2024). Specific AI attitudes and knowledge, should therefore be considered in future studies in order to more deeply understand the effects of AI-generated news.
Furthermore, future research should investigate whether individuals who know more about AI are also less likely to follow certain machine heuristics (Sundar and Nass, 2001), as has been hypothesized based on the MAIN model (Sundar, 2008). Possibly, knowledge of AI could lead people to see AI as inherently biased—as it is trained by (biased) humans—and thus may generate biased information (Lloyd, 2018; Gupta et al., 2021)—potentially leading some to believe AI-generated articles are still biased, thus leading to continued HMP. However, the processing of AI-generated information by individuals cannot be fully explained on the basis of quantitative data alone. This underlines the need for qualitative research methods (e.g., free response methodologies). Qualitative research could provide valuable insights into how individuals subjectively interpret and rationalize AI-generated, AI-assisted and human authored news.
Our results contribute to research on the hostile media phenomenon and the question of how to mitigate the hostile media effect (e.g., Cloudy et al., 2021; Hansen and Kim, 2011; Gunther and Liebhart, 2006; Matthes et al., 2023; Waddell, 2019). The present findings suggest that HMP can be reduced by the use of AI-generated news articles, at least for some individuals (i.e., those with negative and moderate biases toward AI).
Our results also have many implications for journalism practice. For example, journalists should be aware that using AI technology to support reporting does not necessarily increase news consumer’s quality assessments of news (e.g., increased credibility, reduced perceptions of bias). Recent data shows that people still prefer news produced by human journalists (vs. AI) (Fletcher and Nielsen, 2024). At the same time, however, younger news audiences are generally more open to the integration of AI into the newsroom. For example, 22% of 18–14-year-olds are comfortable with news generated entirely by AI (vs. 14% in other age groups), and 30% of 18–24-year-olds say they are comfortable with news that is “mostly produced by AI (with some human supervision)” (vs. 21% in other age groups) (Fletcher and Nielsen, 2024). This suggests that attitudes toward the integration of AI into news differ between age groups, and future research should investigate whether the effects of exposure to AI-generated news follow similar patterns.
The present study comes with limitations. First, the two samples we used (total N = 1,197) were not quota-based. Although, our key findings seem to be robust, future studies could use a quota sample and try to replicate these results both within the United States, and in other countries, as recent research revealed that there are cultural differences in people’s AI attitudes and reactions to new technologies (Yam et al., 2023). Further, we focused only on one divisive issue (gun regulation). Although we believe that the effects should replicate in combination with other divisive topics like abortion (see Hartmann and Tanis, 2013), future studies could test this. Future studies should also try to use other panel providers to recruit people directly based on their agreement or disagreement with the topic of gun regulation, which was not possible in our case. Finally, we explored effects of HMP on online engagement in a rather exploratory way. Future research could further examine in depth how perceptions of the media as hostile affect user engagement online.
As AI is increasingly incorporated into today’s media industry, investigating how AI-generated news affects news consumers’ hostile media perceptions is imperative. We conducted two experimental studies in the United States and show that an AI-generated news article mitigates individuals’ hostile media perceptions. However, this effect is only detected in individuals with negative and moderate prior AI attitudes, while people with positive prior AI attitudes were not affected. AI-assisted news (AI and a human journalist working together) had no dampening effect on hostile media perceptions in Study 1 and only people with positive prior AI attitudes showed lower levels of hostile media perceptions in Study 2 (while moderates and those with negative attitudes did not). Results also suggest that lower levels of HMP (triggered by an AI author) may increase people’s online engagement (i.e., sharing of news with friends and family). These findings add to our understanding on how AI-generated news affects news consumers and makes a theoretical contribution to research informed by the hostile media phenomenon (Perloff, 2015; Vallone et al., 1985) and the MAIN model (Sundar, 2008).
The datasets presented in this study can be found in online repositories. The names of the repository/repositories and accession number(s) can be found in the article/Supplementary material.
The studies involving humans were approved by Ethics Committee of the Department of Psychology, University of Kaiserslautern-Landau (RPTU). The studies were conducted in accordance with the local legislation and institutional requirements. The participants provided their written informed consent to participate in this study.
EH: Conceptualization, Formal analysis, Investigation, Methodology, Validation, Writing – original draft, Writing – review & editing, Data curation, Visualization. EK: Conceptualization, Data curation, Formal analysis, Investigation, Methodology, Writing – review & editing, Supervision. CS: Conceptualization, Investigation, Methodology, Supervision, Writing – review & editing, Funding acquisition, Project administration, Resources, Validation, Writing – original draft.
The author(s) declare that financial support was received for the research and/or publication of this article. We are grateful for support from the Schlieper Foundation to CS (No. 4507-LD-28202).
The authors declare that the research was conducted in the absence of any commercial or financial relationships that could be construed as a potential conflict of interest.
All claims expressed in this article are solely those of the authors and do not necessarily represent those of their affiliated organizations, or those of the publisher, the editors and the reviewers. Any product that may be evaluated in this article, or claim that may be made by its manufacturer, is not guaranteed or endorsed by the publisher.
The Supplementary material for this article can be found online at: https://www.frontiersin.org/articles/10.3389/fcomm.2025.1484186/full#supplementary-material
Ariyanto, A. M., Hornsey, J., and Gallois, C. (2007). Group allegiances and perceptions of media bias: taking into account both the perceiver and the source. Group Process. Intergroup Relat. 10, 266–279. doi: 10.1177/1368430207074733
Arpan, L. M., and Raney, A. A. (2003). An experimental investigation of news source and the hostile media effect. Journal. Mass Commun. Quart. 80, 265–281. doi: 10.1177/107769900308000203
Beckett, C., and Yaseen, M. (2023). Generating change: a global survey of what news organisations are doing with artificial intelligence. London: JournalismAI, London School of Economics.
Bellur, S., and Sundar, S. S. (2014). How can we tell when a heuristic has been used? Design and analysis strategies for capturing the operation of heuristics. Commun. Methods Meas. 8, 116–137. doi: 10.1080/19312458.2014.903390
Böhm, R., Jörling, M., Reiter, L., and Fuchs, C. (2023). People devalue generative AI’s competence but not its advice in addressing societal and personal challenges. Commun. Psychol. 1:32. doi: 10.1038/s44271-023-00032-x
Burgoon, J. K., and Le Poire, B. A. (1993). Effects of communication expectancies, actual communication, and expectancy disconfirmation on evaluations of communicators and their communication behavior. Hum. Commun. Res. 20, 67–96. doi: 10.1111/j.1468-2958.1993.tb00316.x
Bussone, A., Stumpf, S., and O’Sullivan, D. (2015). The role of explanations on trust and reliance in clinical decision support systems. 2015 International conference on healthcare informatics.
Chia, S. C., Yong, S. Y. J., Wong, Z. W. D., and Koh, W. L. (2007). Personal bias or government bias? Testing the hostile media effect in a regulated press system. Int. J. Public Opin. Res. 19, 313–330. doi: 10.1093/ijpor/edm011
Clerwall, C. (2014). Enter the robot journalist. Journal. Pract. 8, 519–531. doi: 10.1080/17512786.2014.883116
Cloudy, J., Banks, J., and Bowman, N. D. (2021). The str(AI)ght scoop: artificial intelligence cues reduce perceptions of hostile media bias. Digit. Journal. 11, 1577–1596. doi: 10.1080/21670811.2021.1969974
DellaVigna, S., and Kaplan, E. (2007). The Fox news effect: media bias and voting. Q. J. Econ. 122, 1187–1234. doi: 10.1162/qjec.122.3.1187
Deuze, M., and Beckett, C. (2022). Imagination, algorithms and news: developing AI literacy for journalism. Digit. Journal. 10, 1913–1918. doi: 10.1080/21670811.2022.2119152
Digital News Report (2024). Reuters Institute for the study of journalism. Available at: https://reutersinstitute.politics.ox.ac.uk/sites/default/files/2024-06/RISJ_DNR_2024_Digital_v10%20lr.pdf (Accessed Febraury 18, 2025).
Eveland, W. P., and Shah, D. V. (2003). The impact of individual and interpersonal factors on perceived news media bias. Polit. Psychol. 24, 101–117. doi: 10.1111/0162-895x.00318
Feldman, L. (2011). Partisan differences in opinionated news perceptions: a test of the hostile media effect. Polit. Behav. 33, 407–432. doi: 10.1007/s11109-010-9139-4
Fletcher, R., and Nielsen, R. K. (2024). What does the public in six countries think of generative AI in news? Available at: https://reutersinstitute.politics.ox.ac.uk/sites/default/files/2024-05/Fletcher_and_Nielsen_Generative_AI_and_News_Audiences.pdf (Accessed Febraury 18, 2025).
Gambino, A., Fox, J., and Ratan, R. (2020). Building a stronger CASA: extending the computers are social actors paradigm. Hum. Mach. Commun. 1, 71–86. doi: 10.30658/hmc.1.5
Gil De Zúñiga, H., Goyanes, M., and Durotoye, T. (2024). A scholarly definition of artificial intelligence (AI): advancing AI as a conceptual framework in communication research. Polit. Commun. 41, 317–334. doi: 10.1080/10584609.2023.2290497
Giner-Sorolla, R., and Chaiken, S. (1994). The causes of hostile media judgments. J. Exp. Soc. Psychol. 30, 165–180. doi: 10.1006/jesp.1994.1008
Graefe, A., and Bohlken, N. (2020). Automated journalism: a meta-analysis of readers’ perceptions of human-written in comparison to automated news. Media Commun. 8, 50–59. doi: 10.17645/mac.v8i3.3019
Graefe, A., Haim, M., Haarmann, B., and Brosius, H. (2018). Readers’ perception of computer-generated news: credibility, expertise, and readability. Journal. Theory Pract. Crit. 19, 595–610. doi: 10.1177/1464884916641269
Gunther, A. C., and Chia, S. C. Y. (2001). Predicting pluralistic ignorance: the hostile media perception and its consequences. Journal. Mass Commun. Quart. 78, 688–701. doi: 10.1177/107769900107800405
Gunther, A. C., and Liebhart, J. L. (2006). Broad reach or biased source? Decomposing the hostile media effect. J. Commun. 56, 449–466. doi: 10.1111/j.1460-2466.2006.00295.x
Gunther, A. C., McLaughlin, B., Gotlieb, M. R., and Wise, D. A. (2017). Who says what to whom: content versus source in the hostile media effect. Int. J. Public Opin. Res. 29, edw009–edw383. doi: 10.1093/ijpor/edw009
Gunther, A. C., and Schmitt, K. (2004). Mapping boundaries of the hostile media effect. J. Commun. 54, 55–70. doi: 10.1111/j.1460-2466.2004.tb02613.x
Gupta, M., Parra, C. M., and Dennehy, D. (2021). Questioning racial and gender bias in AI-based recommendations: do espoused national cultural values matter? Inf. Syst. Front. 24, 1465–1481. doi: 10.1007/s10796-021-10156-2
Haim, M., and Graefe, A. (2017). Automated news: better than expected? Digit. Journal. 5, 1044–1059. doi: 10.1080/21670811.2017.1345643
Hansen, G. J., and Kim, H. (2011). Is the media biased against me? A meta-analysis of the hostile media effect research. Commun. Res. Rep. 28, 169–179. doi: 10.1080/08824096.2011.565280
Hartmann, T., and Tanis, M. (2013). Examining the hostile media effect as an intergroup phenomenon: the role of ingroup identification and status. J. Commun. 63, 535–555. doi: 10.1111/jcom.12031
Hayes, A. F. (2013). Methodology in the social sciences. Introduction to mediation, moderation, and conditional process analysis: a regression-based approach. New York, NY: The Guilford Press.
Hayes, A. F., and Matthes, J. (2009). Computational procedures for probing interactions in OLS and logistic regression: SPSS and SAS implementations. Behav. Res. Methods 41, 924–936. doi: 10.3758/BRM.41.3.924
Huang, G., and Wang, S. (2023). Is artificial intelligence more persuasive than humans? A meta-analysis. J. Commun. 73, 552–562. doi: 10.1093/joc/jqad024
Jacobs, M., Pradier, M. F., McCoy, T. P., Perlis, R. H., Doshi-Velez, F., and Gajos, K. Z. (2021). How machine-learning recommendations influence clinician treatment selections: the example of antidepressant selection. Transl. Psychiatry 11, 108–109. doi: 10.1038/s41398-021-01224-x
Jia, C. (2020). Chinese automated journalism: a comparison between expectations and perceived quality. Int. J. Commun. 14, 1–21.
Jia, C., and Gwizdka, J. (2020). “An eye-tracking study of differences in reading between automated and human-written news” in Information systems and neuroscience. eds. F. D. Davis, R. Riedl, J. V. Brocke, P.-M. Léger, A. B. Randolph, and T. Fischer (Switzerland: Springer, Cham NeuroIS Retreat), 100–110.
Jia, C., and Johnson, T. (2021). Source credibility matters: does automated journalism inspire selective exposure? Int. J. Commun. 15, 3760–3781.
Jia, C., and Liu, R. (2021). Algorithmic or human source? Examining relative hostile media effect with a transformer-based framework. Media Commun. 9, 170–181. doi: 10.17645/mac.v9i4.4164
Kleinnijenhuis, J., Hartmann, T., Tanis, M., and van Hoof, A. M. F. (2020). Hostile media perceptions of friendly media do reinforce partisanship. Commun. Res. 47, 276–298. doi: 10.1177/0093650219836059
Ksiazek, T. G., Peer, L., and Lessard, K. (2016). User engagement with online news: conceptualizing interactivity and exploring the relationship between online news videos and user comments. New Media Soc. 18, 502–520. doi: 10.1177/1461444814545073
Kubin, E., Gray, K. J., and von Sikorski, C. (2023). Reducing political dehumanization by pairing facts with personal experiences. Polit. Psychol. 44, 1119–1140. doi: 10.1111/pops.12875
Kubin, E., Puryear, C., Schein, C., and Gray, K. (2021). Personal experiences bridge more and political divides better than facts. Proc. Natl. Acad. Sci. 118, 1–9. doi: 10.1073/pnas.2008389118
Kubin, E., and von Sikorski, C. (2021). The role of (social) media in political polarization: a systematic review. Ann. Int. Commun. Assoc. 45, 188–206. doi: 10.1080/23808985.2021.1976070
Lermann Henestrosa, A., Greving, H., and Kimmerle, J. (2023). Automated journalism: the effects of AI authorship and evaluative information on the perception of a science journalism article. Comput. Hum. Behav. 138:107445. doi: 10.1016/j.chb.2022.107445
Levay, K. E., Freese, J., and Druckman, J. N. (2016). The demographic and political composition of mechanical Turk samples. SAGE Open 6, 1–17. doi: 10.1177/2158244016636433
Levy-Landesberg, H., and Cao, X. (2024). Anchoring voices: the news anchor’s voice in China from television to AI. Media Cult. Soc. 47, 229–251. doi: 10.1177/01634437241270937
Li, J., Foley, J. M., Dumdum, O., and Wagner, M. W. (2022). The power of a genre: political news presented as fact-checking increases accurate belief updating and hostile media perceptions. Mass Commun. Soc. 25, 282–307. doi: 10.1080/15205436.2021.1924382
Liu, B., and Wei, L. (2019). Machine authorship in situ: effect of news organization and news genre on news credibility. Digit. Journal. 7, 635–657. doi: 10.1080/21670811.2018.1510740
Lloyd, K. (2018). Bias amplification in artificial intelligence systems. arXiv (Cornell University). Available at: http://arxiv.org/pdf/1809.07842.pdf (Accessed February 18, 2025).
Matthes, J. (2013). The affective underpinnings of hostile media perceptions: exploring the distinct effects of affective and cognitive involvement. Commun. Res. 40, 360–387. doi: 10.1177/0093650211420255
Matthes, J., Schmuck, D., and von Sikorski, C. (2023). In the eye of the beholder: a case for the visual hostile media phenomenon. Commun. Res. 50, 879–903. doi: 10.1177/00936502211018596
Newhagen, J., and Nass, C. (1989). Differential criteria for evaluating credibility of newspapers and TV news. Journal. Q. 66, 277–284. doi: 10.1177/107769908906600202
Nomura, T., Kanda, T., and Suzuki, T. (2006). Experimental investigation into influence of negative attitudes toward robots on human–robot interaction. AI & Soc. 20, 138–150. doi: 10.1007/s00146-005-0012-7
Oh, S., and Park, E. (2022). Are you aware of what you are watching? Role of machine heuristic in online content recommendations. ArXiv. doi: 10.48550/arXiv.2203.08373
Oraka, E., Thummalapally, S., Anderson, L., Burgess, T., Seibert, F., and Strasser, S. (2019). A cross-sectional examination of US gun ownership and support for gun control measures: sociodemographic, geographic, and political associations explored. Prev. Med. 123, 179–184. doi: 10.1016/j.ypmed.2019.03.021
Patterson, T. L., and Donsbach, W. (1996). News decisions: journalists as partisan actors. Polit. Commun. 13, 455–468. doi: 10.1080/10584609.1996.9963131
Peiser, J. (2019). The rise of the robot reporter. The New York Times. Available at: https://www.nytimes.com/2019/02/05/business/media/artificial-intelligence-journalism-robots.html (Accessed February 18, 2025).
Perloff, R. M. (1989). Ego-involvement and the third person effect of televised news coverage. Commun. Res. 16, 236–262. doi: 10.1177/009365089016002004
Perloff, R. M. (2015). A three-decade retrospective on the hostile media effect. Mass Commun. Soc. 18, 701–729. doi: 10.1080/15205436.2015.1051234
Pew Research Center (2017). The partisan divide on political values grows even wider. Available at: https://www.pewresearch.org/politics/2017/10/05/the-partisan-divide-on-political-values-grows-even-wider/ (Accessed November 4, 2023).
Pew Research Center (2021). Key facts about Americans and guns. Available at: https://www.pewresearch.org/short-reads/2021/09/13/key-facts-about-americans-and-guns/ (Accessed November 4, 2023).
Reeves, J. (2016). Automatic for the people: the automation of communicative labor. Commun. Crit. Cult. Stud. 13, 150–165. doi: 10.1080/14791420.2015.1108450
Reuters (2023). Associated Press, OpenAI partner to explore generative AI use in news. Available at: https://www.reuters.com/business/media-telecom/associated-press-openai-partner-explore-generative-ai-use-news-2023-07-13/ (Accessed July 22, 2023).
Robledo, A. (2023). None of these anchors are real: Channel 1 plans for AI to generate news, broadcasters. USA Today. Available at: https://eu.usatoday.com/story/tech/news/2023/12/14/channel-1-ai-news/71925360007/ (Accessed November 25, 2024).
Sindermann, C., Sha, P., Zhou, M., Wernicke, J., Schmitt, H. S., Li, M., et al. (2022). Assessing the attitude towards artificial intelligence: introduction of a short measure in German, Chinese, and English language. Künstl. Intell. 35, 109–118. doi: 10.1007/s13218-020-00689-0
Suchman, L. (2023). The uncontroversial ‘thingness’ of AI. Big Data Soc. 10:20539517231206794. doi: 10.1177/20539517231206794
Sundar, S. (2008). “The MAIN model: a heuristic approach to understanding technology effects on credibility” in Digital media, youth, and credibility. eds. M. J. Metzger and J. Flanagin (Cambridge: The MIT Press), 72–100.
Sundar, S. S., and Nass, C. (2001). Conceptualizing sources in online news. J. Commun. 51, 52–72. doi: 10.1111/j.1460-2466.2001.tb02872.x
Tandoc, E. C. Jr., Yao, L. J., and Wu, S. (2020). Man vs. machine? The impact of algorithm authorship on news credibility. Digit. Journal. 8, 548–562. doi: 10.1080/21670811.2020.1762102
The Washington Post. (2017). The Washington post leverages Heliograf to cover high school football [press release]. The Washington Post. Available at: https://www.washingtonpost.com/pr/wp/2017/09/01/the-washington-post-leverages-heliograf-to-cover-high-school-football/ (Accessed February 18, 2025).
Vallone, R. P., Ross, L., and Lepper, M. R. (1985). The hostile media phenomenon: biased perception and perceptions of media bias in coverage of the Beirut massacre. J. Pers. Soc. Psychol. 49, 577–585. doi: 10.1037/0022-3514.49.3.577
Van Dalen, A. (2012). The algorithms behind the headlines. Journal. Pract. 6, 648–658. doi: 10.1080/17512786.2012.667268
Waddell, T. F. (2019). Can an algorithm reduce the perceived bias of news? Testing the effect of machine attribution on news readers’ evaluations of bias, anthropomorphism, and credibility. Journal. Mass Commun. Quart. 96, 82–100. doi: 10.1177/1077699018815891
Wischnewski, M., and Krämer, N. (2021). The role of emotions and identity-protection cognition when processing (mis)information. Technol. Mind Behav. 2. doi: 10.1037/tmb0000029
Wölker, A., and Powell, T. D. (2018). Algorithms in the newsroom? News readers’ perceived credibility and selection of automated journalism. Journal. Theory Pract. Crit. 22, 86–103. doi: 10.1177/1464884918757072
Wu, Y. (2020). Is automated journalistic writing less biased? An experimental test of auto-written and human-written news stories. Journal. Pract. 14, 1008–1028. doi: 10.1080/17512786.2019.1682940
Keywords: AI-generated news, AI attitudes, HMP, online engagement, experiment
Citation: Huh E, Kubin E and von Sikorski C (2025) Can AI-generated news reduce hostile media perceptions? Findings from two experiments. Front. Commun. 10:1484186. doi: 10.3389/fcomm.2025.1484186
Received: 21 August 2024; Accepted: 14 February 2025;
Published: 13 March 2025.
Edited by:
Anke van Kempen, Munich University of Applied Sciences, GermanyReviewed by:
Maximilian Eder, Ludwig Maximilian University of Munich, GermanyCopyright © 2025 Huh, Kubin and von Sikorski. This is an open-access article distributed under the terms of the Creative Commons Attribution License (CC BY). The use, distribution or reproduction in other forums is permitted, provided the original author(s) and the copyright owner(s) are credited and that the original publication in this journal is cited, in accordance with accepted academic practice. No use, distribution or reproduction is permitted which does not comply with these terms.
*Correspondence: Christian von Sikorski, Y2hyaXN0aWFuLnZvbnNpa29yc2tpQHJwdHUuZGU=
Disclaimer: All claims expressed in this article are solely those of the authors and do not necessarily represent those of their affiliated organizations, or those of the publisher, the editors and the reviewers. Any product that may be evaluated in this article or claim that may be made by its manufacturer is not guaranteed or endorsed by the publisher.
Research integrity at Frontiers
Learn more about the work of our research integrity team to safeguard the quality of each article we publish.