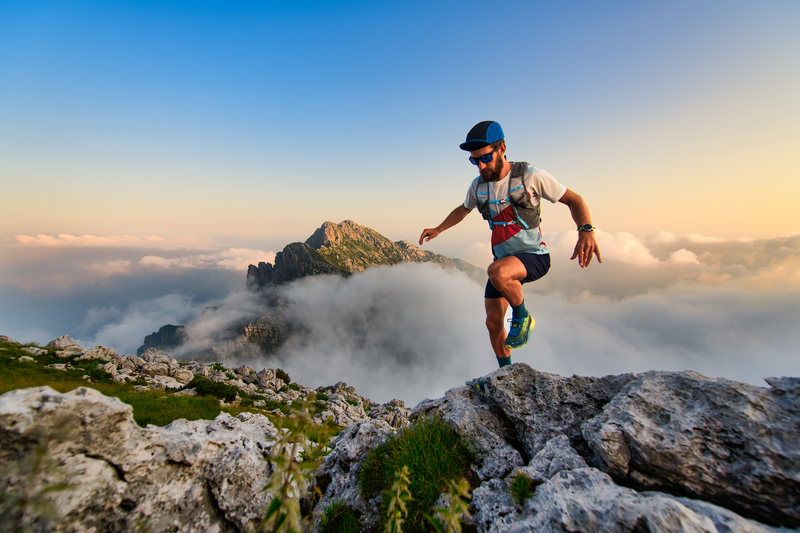
94% of researchers rate our articles as excellent or good
Learn more about the work of our research integrity team to safeguard the quality of each article we publish.
Find out more
ORIGINAL RESEARCH article
Front. Commun. , 04 April 2024
Sec. Disaster Communications
Volume 9 - 2024 | https://doi.org/10.3389/fcomm.2024.1366995
Introduction: We conducted mental model interviews in Aotearoa NZ to understand perspectives of uncertainty associated with natural hazards science. Such science contains many layers of interacting uncertainties, and varied understandings about what these are and where they come from creates communication challenges, impacting the trust in, and use of, science. To improve effective communication, it is thus crucial to understand the many diverse perspectives of scientific uncertainty.
Methods: Participants included hazard scientists (n = 11, e.g., geophysical, social, and other sciences), professionals with some scientific training (n = 10, e.g., planners, policy analysts, emergency managers), and lay public participants with no advanced training in science (n = 10, e.g., journalism, history, administration, art, or other domains). We present a comparative analysis of the mental model maps produced by participants, considering individuals’ levels of training and expertise in, and experience of, science.
Results: A qualitative comparison identified increasing map organization with science literacy, suggesting greater science training in, experience with, or expertise in, science results in a more organized and structured mental model of uncertainty. There were also language differences, with lay public participants focused more on perceptions of control and safety, while scientists focused on formal models of risk and likelihood.
Discussion: These findings are presented to enhance hazard, risk, and science communication. It is important to also identify ways to understand the tacit knowledge individuals already hold which may influence their interpretation of a message. The interview methodology we present here could also be adapted to understand different perspectives in participatory and co-development research.
Managing natural hazard events (NHEs) requires communicating the science of those hazards effectively to a diverse range of individuals, from planners to policymakers, or emergency responders to community members involved in hazard preparedness, mitigation, response, and recovery (Doyle and Becker, 2022). Recent events in Aotearoa New Zealand (AoNZ), such as Cyclone Gabrielle in 2023 and the Mw7.8 Kaikōura earthquake in 2016, demonstrated how communicating this science effectively, and in a manner that meets decision-makers’ needs and perspectives, is vital to encourage appropriate individual responses to hazards, warnings, and advice (e.g., Vinnell et al., 2022). However, the effective communication of the uncertainty associated with this information is an ongoing challenge impacting how individuals trust, evaluate, and respond (or not) to a scientific message (Johnson and Slovic, 1995; Smithson, 1999; Miles and Frewer, 2003; Freudenburg et al., 2008; Wiedemann et al., 2008; Oreskes, 2015; Kovaka, 2019). Therefore, it is crucial to explore the varied ways in which individuals perceive this uncertainty. Communication can thus be improved by adapting it to different audiences’ perspectives and views (Doyle and Becker, 2022).
Models of both science and risk communication have evolved from one-way dissemination approaches that assume a deficit of knowledge or science literacy that expert knowledge can inform via knowledge transfer, through to a more recent two-way democratic approach focused on empowerment, knowledge sharing, and engagement, where both the science/risk communicators and the public are learning from each other and incorporating multiple sources of knowledge and expertise (Fisher, 1991; Fischhoff, 1995; Leiss, 1996; Covello and Sandman, 2001; Miller, 2001; Gurabardhi et al., 2005; Bauer et al., 2007; Kappel and Holmen, 2019; Doyle and Becker, 2022; Tayeebwa et al., 2022). In the latter approach communication is driven by a goal to build a ‘model of mutual constructed understanding’ (Leiss, 1996; Renn, 2014).
As discussed in Doyle and Becker (2022) both the dissemination and engagement approaches to communication are valuable for natural hazards risk, as the suitability of their use depends upon the context and time pressures: one-way dissemination approaches are appropriate in crisis and warning situations where short time frames require rapid response; meanwhile democratic, two-way, deliberative approaches are more appropriate in the communication of longer term risk (and associated science) such as during the readiness, response, and recovery phases of natural hazards management. Ideally, the former rapid dissemination approach is enhanced with participatory work prior to a crisis to inform communication design (e.g., Bostrom et al., 2008; Kostelnick et al., 2013; Morrow et al., 2015). Thus, it is vital that a communicator understands peoples’ prior perceptions of the science or natural hazards system (including both the physical, social, and cultural context), whether they are communicating via one-way dissemination or through two-way engaged discussions, as such perceptions will influence how people understand science/risk information in whichever manner it is received.
Several communication models and frameworks for disaster risk and science have thus been developed to incorporate people’s perceptions as a core aspect of their approach (Doyle and Becker, 2022). These include the Community Engagement Theory (CET) which incorporates risk communication with broader community development concepts (Paton, 2019), and considers factors such as participation, collective efficacy, social capital, empowerment, and trust (Paton, 2019; Paton and Buergelt, 2019). Alternatively, the Risk Information Seeking and Processing Model (RISP) (Griffin et al., 1999; Yang et al., 2014) aims to understand how people’s perceptions of risk influence the way they seek and interact with information, to “disentangle the social, psychological, and communicative factors that drive risk information seeking” (Yang et al., 2014, p. 20). Drawing on practice-based contexts, the Crisis and Emergency Risk Communication model (CERC) (Veil et al., 2008) brings together risk and crisis communication principles through five core stages: pre-crisis communication and education; in-event rapid communication; maintenance communication including correction of misunderstandings; resolution considering updates, new understandings of risk, discussion about the cause; and evaluation of response and lessons learnt. Similarly, the IDEA model (Sellnow et al., 2017; Sellnow and Sellnow, 2019) considers four components: internalization (whether people see the risk relevant to themselves); distribution (whether people can access the information via various channels); explanation (whether people understand and think a risk is credible); and action (whether people take actions to protect themselves). This framework identifies key components of a process for instructional risk communication to lay audiences and has been successfully applied in varied contexts from earthquake early warning (Sellnow et al., 2019) through to the recent COVID-19 pandemic (Cook et al., 2021).
Individuals interpret such scientific information and advice in diverse ways due to influences such as how the information is framed (Teigen and Brun, 1999; Morton et al., 2011; Doyle et al., 2014), cognitive, cultural and social factors (Lindell and Perry, 2000; Olofsson and Rashid, 2011; Medin and Bang, 2014; Huang et al., 2016), expertise and experience (Becker et al., 2022; Vinnell et al., 2023), expectations of message content (Rabinovich and Morton, 2012; Maxim and Mansier, 2014), and the uncertain context within which perils are situated (Stirling, 2010; Fischhoff and Davis, 2014; Doyle et al., 2019). Scientific uncertainty is considered herein to exist in complex social-environmental contexts, and thus both directly arises from the science (such as due to the data availability, or model validity) and indirectly arises from judgments associated with the science (such as model choice and how governance decisions influence science direction), see Doyle et al. (2022). It can arise from the natural stochastic uncertainty (variability of the system), the epistemic uncertainty (lack of knowledge), disagreements among scientists due to incomplete information, inadequate understanding, and undifferentiated alternatives, as well as a lack of understanding not only of what to say but who to communicate it to.
Communicating this uncertainty is particularly challenging (Doyle et al., 2019), as while acknowledgment of its presence can promote trust, it can also be used to discount a message (Johnson and Slovic, 1995; Smithson, 1999; Miles and Frewer, 2003; Wiedemann et al., 2008), and deny science or discount scientific findings (Freudenburg et al., 2008; Oreskes, 2015; Kovaka, 2019). Prior perceptions of the environment of uncertainty, including of the communicator and the communication network, the perceptions of the physical system being assessed, and the perceptions of the sources of uncertainty in the data and models of that system, all influence how individuals interpret and act upon associated information (Doyle et al., 2022). Such perceptions act as a lens through which science information is interpreted and can override communicated information, particularly when that uncertainty is high.
Thus, as communication models and research indicate, effective science communication prior to, during, and after NHEs requires a more comprehensive understanding of the range of existing perceptions about the uncertainty present (Bostrom et al., 1992; Greca and Moreira, 2000; Doyle et al., 2014, 2019), and how they might vary between individuals with different disciplinary and organizational backgrounds. By advancing our theoretical understanding of these perceptions we can enhance communication practices for the effective provision of scientific advice. This is vital to increase public and professional confidence in scientific information for situations when the message content might contradict existing perceptions of the science (Eiser et al., 2012; Sword-Daniels et al., 2018; Becker et al., 2019; Doyle et al., 2019).
As reviewed in Doyle et al. (2022, 2023) such perceptions are based upon people’s mental models of how they think the world works (Bostrom et al., 1992; Morgan et al., 2001; Johnson-Laird, 2010; Jones et al., 2011), including their model of scientific processes, motivations, beliefs and values; leading to epistemic differences across disciplines and organizations (Bostrom, 2017; Doyle et al., 2019). Previous research has qualitatively explored the mental models people hold about hazardous processes and other phenomena, to enhance science education (e.g., Greca and Moreira, 2000; Tripto et al., 2013; Lajium and Coll, 2014), to understand hypothesis formation (e.g., Brewer, 1999; Hogan and Maglienti, 2001; Rapp, 2005), and to design risk communication products, e.g., hurricane warnings (Bostrom et al., 2016).
Most recently, Doyle et al. (2023) conducted a series of qualitative mental model interviews to elicit people’s perceptions of the sources of uncertainty. Through a qualitative thematic analysis of the interviews, they identified several sources of uncertainty discussed across the group of participants (from policymakers, to scientists, to emergency managers and the public). These included The Data (not having enough, its uniqueness, interpretation of), The Actors (including the scientists, the media, communicators, key stakeholders), and The Known Unknowns (including the range of possible outcomes, unexpected human responses, and the presence of unknown unknowns). Underlying and influencing these sources were a range of additional factors, including governance and funding, societal and economic factors, the role of emotions, how perceived outcomes helped scaffold meaning making of uncertainty, the communication landscape, and influences from time and trust.
Previous work has also shown that experts in a field can have more coherent, structured, and consistent mental models of issues within that field than non-experts or novices, who may use a patchwork of mental models to understand the issues instead (Chi et al., 1981; Gentner and Gentner, 1983; Collins and Gentner, 1987; Schumacher and Czerwinski, 1992). However, it is unclear how such domain expertise (e.g., in a specific scientific discipline) relates to understanding and perspectives of scientific uncertainty associated with NHE advice. This is important to understand, as lessons can inform effective communication of uncertainty between the wide range of science advisors and decision-makers usually involved in managing natural hazards risk (and who come from across diverse disciplines, e.g., from health to geology, or policy to emergency responders). Thus, in the study herein we build on the work of Doyle et al. (2023) by conducting a detailed comparative analysis of participants’ mental model maps of scientific uncertainty, driven by the research question: “How do perceptions of uncertainty differ between individuals depending upon their experience of, and training in, science?.” Findings will deepen our understanding of what different audiences anticipate the uncertainty influences on science advice to be, to inform communication guidelines that address those diverse audience perceptions.
A multi-phase interview procedure was developed to understand individuals’ perceptions of uncertainty associated with natural hazard science, based on the Conceptual Cognitive Concept Mapping (3CM) approach (Kearney and Kaplan, 1997; Romolini et al., 2012). Here we present results from Phase 2, an exploratory mental model mapping activity to understand where participants perceive uncertainty to come from. Phase 1, which involved initial direct elicitation questions to explore how participants define uncertainty, is presented in Doyle et al. (2023). The analysis of Phase 3, exploring participants’ wider understanding of the production of science, is currently in progress.
Interviews were conducted by two of the authors either in person, or virtually via Zoom, from late 2020 to mid-2022. Participants used post-it notes to brainstorm their thoughts onto a large sheet of paper in response to the question “Thinking about natural hazards science, where do you think uncertainty comes from?.” Those interviewed via Zoom used virtual post-it notes via the virtual online whiteboard (Mural).1 Participants were then asked to cluster these post-it notes to form groups of similar or related sources and to explain why, to give that cluster a ‘name’ if they wanted to, and to draw their perceived connections between these clusters, such as through dependencies or relational links, if they perceived any connections or relational links to exist. Some participants chose not to make connections/links. This method was designed to enable participants to access implicit knowledge (Levine et al., 2015) and to promote higher-level thinking (Grima et al., 2010) due to the creative, tactile nature of the task (Cassidy and Maule, 2012; Doyle et al., 2022). If participants struggled to brainstorm sources of uncertainty, they were prompted with the question “What might increase or decrease uncertainty associated with science advice about hazards?” to help them identify sources.
We used a broad initial definition of uncertainty as “unknowns associated with, or within, science and science advice” from which our participants’ conversations then developed. Explicit definitions were not provided such that participants were not biased or influenced by them and were free to define uncertainty in ways and terms that met their own understanding. The total interview took between 60 and 90 minute, and was audio recorded and transcribed verbatim for later analysis. The Massey University Ethical Code of Conduct was followed, and this research received a Low-Risk Ethics Notification Number of 4000023593. Participants were given a $40 supermarket voucher as a thank you for their time. See Doyle et al. (2023) for further details about interview design and sampling.
The study was conducted in AoNZ, where most people experience natural hazards often, either directly or indirectly, including earthquakes, volcanoes, severe weather, and its consequences, and thus have high exposure to natural hazard information. Individuals were recruited via advertising and snowballing and needed to be 18 years or older to participate. The initial recruitment phase (n = 25) included the science and research community (including universities, consultancies, and crown research institutes), emergency management and civil defense authorities, local and regional councils, the policy and planning communities, and broader community members who may act upon associated information during a NHE. The initial sample presented in Doyle et al. (2022) under-represented participants with little to no science training and also represented a highly educated cohort. Thus, to provide a larger Lay public sample and enable an exploratory study of how perspectives vary between Scientist and Lay participants, we recruited an additional 6 members of the public via more targeted advertising for individuals who had less science experience and expertise. These participants were recruited through the social networks of two of the authors; all were non-scientists, their highest qualifications were either high school (n = 3) or undergraduate (n = 3), with 5 of the 6 not studying science since high school while the sixth studied science alongside their education degree (completed several decades prior to this study). In total, 31 individuals thus participated in the study. All were residing in AoNZ at the time of the interview. Ages ranged from 25 to 75 years old, with 11 participants identifying as male and 20 participants identifying as female. Ethnicities included NZ European/ Pākehā2 (n = 21), European (including Italian, English, and British; 5), Māori / NZ European (2), Latino (1), and Iranian/Middle Eastern (1). One participant chose not to disclose demographic details. Professions included 4 physical scientists, 2 boundary or other knowledge transfer scientists, 2 social scientists, 6 policy writers/analysts and planners, 2 engineers, 4 emergency management practitioners, 2 with a legal background, and 9 others including a journalism student, teachers, administrator/secretaries, a city council worker, an artist, an independent historian, and an anthropologist.
The cohort of participants was analyzed in three groups: Scientists, Science Literate, and Lay Public. The Scientists (n = 11) were considered to have high levels of expertise and experience. They were those with a tertiary education in a science field and also working in a science field, and included natural hazards scientists, engineers, environmental and geoscientists, social scientists, public health, risk and data scientists. The Science Literate (n = 10) were those who had high levels of experience, but lower levels of expertise, and who either had a tertiary education in science or were working in a science field, but not both. Meanwhile, the Lay Public (n = 10) were those with no tertiary education in science and not working in a science field. The designation of participants to each group was based on how participants self-described their education and employment. All participants were assigned a pseudonym prior to interviews.
Each participant’s mental map was analyzed using a ‘descriptive’ content analysis approach, considering their post-its and their clusters (groups of post-its) (Cassidy and Maule, 2012; Romolini et al., 2012). The number of post-its, the number of clusters produced, the number of links between clusters, the total time it took to produce the map and the number of pauses for each participant was calculated and totaled for each group, as shown in Table 1. Pauses were indicated by an absence of verbal of physical response (i.e., not moving post-its or drawing on the map) that lasted for more than a few seconds, and usually required a prompt from the interviewer to continue the mapping. Maps were categorized into ‘complex’ (containing multiple clusters with many interconnecting links) or ‘not complex’ categories (containing very few clusters with minimal links) and a general description of how the clusters appeared on the page was developed, such as clusters looking like ‘columns’ or ‘groups’. The links were described by how the clusters were connected to each other, via five categories (like Levy et al., 2018), see Figure 1 for examples:
1. None – no links were drawn between clusters and no relationships were described during the interview.
2. Simple links – participants linked one cluster to one other cluster, or only linked some of their clusters, but not all of them.
3. All related – participants drew no relational lines but stated their clusters were ‘all related’ during the interview.
4. Sequential links – participants drew a chain of direct relations from one cluster to the next in a sequence. This may have been 1–2 clusters, or more, but may not have included all clusters.
5. Multi layered links – clusters were connected to many other clusters through single or bidirectional relationship lines.
Table 1. Construction descriptions of participants maps: considering all participants in each group of scientists (n = 11), science literate (n = 10), and the lay public (n = 10).
Figure 1. Example participant maps generated during the interviews, included here to compare map organization. (A) Fearn’s map, Lay Public group, Simple Links; (B) Luis’ map, Lay Public group, Complex Links; (C) Zeta’s Map, Scientist group, Complex Links; (D) Iota’s Map, Science Literate group, Simple Links (on Mural, virtual whiteboard).
After considering the structure and complexity of each map individually, a reflective process of comparison and collation of maps occurred, and a descriptive content analysis was conducted. For each participant’s map within a group, a cluster and its key components (the post-its) were identified by the ‘name’ of a cluster identified by a participant, and then a comparison was made between how that participant had represented that cluster compared to other participants in their group in order to produce an overall collective representation of that cluster for that group, through the process illustrated in Figure 2, producing master cards such as that shown in Figure 3 (see Tripto et al. (2016) for a similar approach using category trees). Through this analysis, the focus was on the dominant components and concepts across each group, noting that not all participants used the same term (or named their clusters) and not all participants discussed the concept represented by that cluster. The motivation was to consider the dominant cluster concepts (sources of uncertainty) mapped by each group, to facilitate assessing differences and similarities between groups, while acknowledging individual differences within a group that were not captured in the analysis.
Figure 2. The process for developing thematic master cards for each group from the individual mental maps and, from those master cards, the process for developing the collective ‘master’ map for each group. The example shown is for the data cluster in the Lay Public group, producing the master card shown in Figure 3, and contributing to the final master map for this group in Figure 4.
Figure 3. A ‘master’ cluster card for ‘data’ from the Lay Public group. Note, the number in the top right-hand corner denotes how many participants in the group had a cluster for ‘data’ on their maps. In the Lay Public group, 4 participants out of 10 discussed data.
As in Cassidy and Maule (2012), the map analysis included the collective descriptive content analysis of the maps produced across each group, described above, followed by a supportive qualitative interpretation of the interview transcripts corresponding to the mapping tasks. This helped contextualize the descriptive content analysis of the maps and enabled a richer understanding of the meanings of the post-its, clusters, and links (see also Doyle et al., 2023). Because the participants did not produce maps in a focus group situation, no direct conflicts arose when deciding which clusters were linked, as our focus of analysis was on identifying the most prevalent or prominent connections shared across each group. If a participant did not draw a link between clusters, we cannot infer that this means a link does not exist, as consideration of the associated audio transcript indicated they did not explicitly say there was no link. Rather they either omitted a discussion of that link due to a focus on other clusters on their map, or they talked about their map more generally.
This process was repeated for each group, generating three collective ‘master’ maps, presented in Section 3. We note, the master maps produced are not illustrating the participants’ individual maps, rather they are a collective conceptual description of the maps from across all participants within a group. This collective description seeks to draw out the dominant concepts and themes mapped by the group, and to organize the concepts the group considered key sources of uncertainty. The master maps thus do not represent a directly elicited group mental model (which would require the group to work together to produce a map). Rather they are an analysis tool to facilitate comparison between the concepts and themes that each group mapped most prominently, and could be considered an indirectly elicited mental model of the group (see Doyle et al., 2022), similar to that of Abel et al. (1998) and Grima et al. (2010).
The results of the analyses of each participant group are presented next. This interpretative analysis was conducted by the two lead authors and thus reflects their experiences and expertise in critical health psychology, and through transdisciplinary training in physical and social sciences of natural hazards and disasters. The analysis thus incorporated regular reflexive discussions between the two lead researchers throughout to understand how those experiences were shaping interpretation (Braun et al., 2022).
The master concept maps for the Lay Public, the Science Literate, and the Scientists are each presented in turn below. First, we outline how each group developed individual maps and how this fed into their own ‘master’ map, followed by a comparison between the content of the master maps for the different groups. In these maps, the nodes represent a collective interpretation of the clusters of post-it’s on the participants’ maps for that group (as outlined in section 2.2, Figures 2, 3). The bullet points associated with each node (see Figures 4–6) represent the post-it’s on the individual maps and describe the node.
Figure 4. The master map for the lay public group. The nodes represent a collated representation of clusters from the participants’ maps and the bullet points beneath describe the node. For example, the node titled ‘government’ represents post-it notes identifying government as a source of uncertainty, and the bullet points describe some specificity of government being a source of uncertainty, such as indecisive leadership.
Figure 5. The master map for the science literate group. The nodes represent a collated representation of clusters from the participants’ maps and the bullet points beneath describe the node. For example, the node titled ‘media’ represents post-it notes identifying the media as a source of uncertainty, and the bullet points describe some of the specificity of the media being a source of uncertainty, such as a perception that the media try to be part of the story.
Figure 6. The master maps for the scientists group. The nodes on the master map represent a collated representation of clusters from the participants’ maps and the bullet points beneath describe the node. For example, the node titled ‘funding’ represents post-it notes identifying funding as a source of uncertainty, and the bullet points describe some of the specificity of funding being a source of uncertainty, such as there being more uncertainty when an area of research is underfunded.
The master map for this group is presented in Figure 4 and shows a complex system of nodes with many overlapping relational links. The solid lines represent links the participants made on their own maps, while a broken line represents links that were interpreted from the audio of the mapping process. That is, the broken links were not drawn on the maps by participants but represent statements such as ‘they are all related’.
On average, the Lay Public group produced 22.3 post-it’s during the brainstorming task, and an average of four clusters when asked if they would consider grouping their post-its (see Table 1). When asked if they considered there to be any relationships between the clusters on their map, this group produced an average of 2.2 links between their clusters. Two participants did not produce any links on their maps, two participants produced simple links, one participant produced a Venn diagram, one participant linked all their clusters to a ‘fear’ cluster, and the remaining four participants said their clusters were ‘all related’ – including one displaying this through a bi-directional circle. Analysis of the associated audio revealed that none of the participants described what the relationship lines represented. As mentioned above, some Lay Public participants simply noted that ‘these ones go together’ or ‘these ones are linked,’ resulting in a lower number of explicitly drawn links than the other groups. Thus, many Lay Public participants did not provide specific descriptions of how their clusters linked, creating some ambiguity as to how to represent the links between concepts on the collective master map (described in Section 2.2). To address this, we undertook a deeper interpretation of their associated discussions to provide insight into links they described but did not directly draw. This interpretative analysis process thus enabled the formation of indirectly elicited mental models of the group (section 2.2; Doyle et al., 2022) by overlaying links described by participants in their interviews to produce the final ‘master’ map for this group, shown in Figure 4. Thus, the relationships shown in these maps represent an interpretation of how the participants believed one concept linked to another.
While some drew directional arrows, causal links were not discussed by participants, thus we cannot infer causality. Rather, the lines represent forms of connection between concepts, for example, how they believed data is linked to the event, is also linked to the government, and is also linked through to other concepts. Double headed arrows demonstrate reciprocal relationships; and as can be seen in Figure 4 this group indicate a vast array of connectivity between nodes (described as “they are all related”) in a less organized fashion than the other groups, presented next.
The master map for this group is presented in Figure 5 and shows a complex system with many, but much more organized, relational, links compared to the Lay Public master map. On average, the Science Literate group produced 21.5 post-it’s during the brainstorming task, and an average of 4.6 clusters (Table 1). They produced an average of 3.9 links between their clusters, when asked if they considered there to be any relationships between the clusters on their map. There were fewer post-it’s than the Lay Public group but more clusters and explicit relationship lines than the Lay Public. Similarities between the relationship links across individual maps could be determined more easily than for the Lay Public group. Five of the participants in this group drew simple links between their clusters to denote relationships, two participants drew sequential links, one participant had no links, one participant stated their clusters were ‘all related’ and one participant had multi-layered links. No relationships between clusters needed to be inferred from the transcripts for this group.
Figure 5 illustrates how the Science Literate group drew or discussed many more links than the Lay Public group and, on some maps, explicitly used arrows to indicate directional or influential relationships, though they did not elaborate on those relationships to describe how they were influential. The maps produced were also more organized than the Lay Public. In the audio recordings of this group, participants seemed more confident than the Lay Public group to draw relationship links between their clusters, as indicated by fewer pauses by this group when asked to consider any relationship links (Table 1). This suggests greater confidence or easier access to their mental model of uncertainty.
The master map for the Scientists group is presented in Figure 6 and also shows a complex system of organized relational links. On average, they produced 18.8 post-it’s during the brainstorming task, an average of 5.45 clusters, and an average of 6.9 explicit links between clusters (Table 1). There are fewer post-it’s for this group than the other two groups, but the individual maps produced were more complex, with more clusters and significantly more relationship lines present than the other two groups. The map also presents a more organized structure than the Lay Public, and slightly more organized than the Science Literate groups (see also Figure 1). This may indicate that these participants had much clearer ideas of how the clusters were related to or interacted with each other, and that one post-it conveyed several or more complex ideas or concepts.
Five participants in this group drew multi-layered links between their clusters to denote relationships, four participants drew linear links, and two participants drew simple links. No participant in this group had an absence of links on their maps. In the audio recordings of this group, participants seemed more confident to draw relationship links between their clusters, like the Science Literate group. They also had fewer pauses when asked if they would cluster their post-its or if they would like to add any relational links to their map (Table 1).
In addition to the clusters and links, several participants described the randomness of things, or human factors, as elements running across all parts of the map, like an overarching influence rather than a distinct node or cluster. Similarly, although time was represented on some maps as a cluster, it was more often discussed as an element of several clusters on a participant’s map, or as running across sections of their map. Thus, time was also interpreted as an overarching element on the collective master map, as shown at the bottom of Figure 6.
In comparing the three collective master maps, it is clear that some nodes are represented in all three master maps, although sometimes under different node names. Table 2 lists the different nodes for each map and how they are represented through different terminology. For example, the Lay Public have a node for ‘environment’, the Science Literate group called this ‘environmental’, and the Scientists group discussed the same or similar elements under the nodes ‘hazards’, ‘local context’, and ‘hazard impacts’. The exception to this is the node ‘trust’ which only appears explicitly on the Lay Public map (see Figure 4 for a fuller description of this node). The Lay Public talked about being able to trust the source of research or the agency that conducted the research, the reputation of the scientist or research institute, whether government was trustworthy compared to independent research institutes, how political influences can lower their trust in science, how there is lower trust in the media and friends who communicate about NHEs, and how a scientist’s trustworthiness depends upon their ability to communicate.
Table 2. A summary of the nodes and clusters from each group’s map regarding sources of uncertainty. Nodes representing similar concepts are aligned.
While some of the elements around trust described above by the Lay Public group were also discussed by the other two groups on various post-its, those other groups did not draw out trust as a stand-alone cluster/node. Additionally, trust also appeared on the nodes for ‘media’, ‘research institute’, and ‘government’ on the Lay Public map. This may demonstrate the importance of trust for the Lay Public when it comes to their understanding of uncertainty in science advice. Similarly, while the Lay Public and Scientists groups also discussed ‘public’ and ‘fear’, the Science Literate group did not. Although the Science Literate group did acknowledge emotions were involved regarding uncertainty in natural hazards science advice, this was discussed in the ‘response’ node and was more centered around how emotional responses affected reactions to NHEs.
Additionally, while some of the nodes had the same titles or discussed similar elements of uncertainty, these elements were often slightly different or nuanced, in a way that may reflect the lens through which they were seen, understood, or articulated by the participants. For example, as shown in Figure 4 and Table 3, the Lay Public node representing ‘data’ and ‘information’ as a source of uncertainty in natural hazards advice centered on the amount of data available, limitations in the ability to collect data, contradictory data, and what is or is not being researched. Meanwhile the Science Literate group, as shown in Figure 5, discussed similar concepts, but under the nodes ‘knowledge status’, ‘lack of understanding’, and ‘existing knowledge’, drawing out additional concepts such as comparisons to overseas data, not knowing how to prioritize, and modeling assumptions. The Scientists group, as shown in Figure 6, discussed similar concepts to the Lay Public group, but under the nodes ‘research/information’ and ‘model/output’. They also drew out further nuance through discussions of reliability of the data, knowledge development, inherent uncertainty, and complexity of model limitations.
Table 3. Example comparison of each group’s nodes and summarized post-its representing sub-concepts for those nodes, considering those that reference and discuss ‘data’ or ‘information’. Nodes representing similar concepts are aligned.
Considering the ‘data/information’ related nodes described in Table 2, and how they appear on Figures 4–6, enables us to understand how the participants articulated key concepts differently. Considering a direct comparison (Table 3) for that node type, we see that all three groups discussed the amount of data available, or a lack of data as a source of uncertainty. However, the remaining elements were either only discussed by one group or by two. For example, ‘which questions are asked’ was mentioned by the Lay Public group, while the Science Literate group noted a source of uncertainty as ‘not knowing what to ask’, and the Scientists group did not discuss research questions as impacting uncertainty at all. The Scientists group also focused more specifically on models, and the nuances of models (such as limitations based on computing power, measurement errors, missing information in models), while the Science Literate group acknowledged assumptions are made about models, and the Lay Public group did not discuss models at all. The Science Literate articulations of sources of uncertainty were more nuanced than those of the Lay Public group but did have some overlap with them. Meanwhile, the Scientist group had the most nuanced description. This may reflect the lay public having only a higher-level, surface, understanding of science and uncertainty, while the Scientists and Science Literate have a deeper more nuanced understanding. This may also be reflected in how both the Scientists and Science Literate group noted uncertainty/inherent uncertainties exist in data, while the Lay Public did not.
Results indicate that the level of science experience and expertise, represented by our participant categorization from Lay Public, to Science Literate, to Scientists, influences the structure of participants’ mental models of scientific uncertainty. There are also corresponding differences in how participants articulate key concepts, and the level of priority they ascribe to those concepts. We next consider how the participant groups ‘know differently’ through an exploration of the literature on the role of expertise, tacit knowledge, and language. This is followed by a discussion of limitations and future research.
Previous work has shown that experts in a field have more coherent, structured, and consistent mental models than non-experts or novices who may use a patchwork or pastiche of models (Chi et al., 1981; Gentner and Gentner, 1983; Collins and Gentner, 1987; Schumacher and Czerwinski, 1992). This difference in understanding comes not just from knowing more, but also from knowing differently. The wider expertise literature shows that, compared to novices, experts (Chi, 2006; Gobet, 2016):
1. generate good, accurate solutions even under time pressure (Klein et al., 2008);
2. readily chunk features of the task environment into larger, domain-relevant patterns;
3. detect deep, task-relevant structural similarities in superficially dissimilar situations;
4. engage in intuitive pattern matching, and “sees what needs to be done, in response to salient aspects of the situation” (Epstein, 1994; Dreyfus, 2002; Endsley, 2006; see also Barsalou, 2008; Baber, 2019, p. 245)
5. spend a relatively long time analyzing and developing representations of problems;
6. are good at self-monitoring and detecting errors in performance;
7. and have large stores of experiential tacit (automatized, proceduralized, chunked) domain-specific knowledge that is often difficult to articulate, but that can be accessed with minimal effort (Polanyi, 1958, 1967; Klein and Hoffman, 1993; Klein et al., 2008; Gobet, 2016).
Our findings are in line with this literature, showing that in general, there is an increase in map organization and the ordering of participants’ mental models of scientific uncertainty as their level of science experience and expertise increased, demonstrated by an increase in structure and complexity of their maps. Interestingly, while the literature points to greater expertise in a science discipline resulting in more structured mental models for that scientific discipline, this study also shows that expertise in science results in more structured mental models of NHE scientific uncertainty even if the former is in a different (non-NHE) scientific discipline.
The Lay Public participants predominantly produced maps with a simpler internal structure, less clarity about the nature of the links between clusters, often referring to the concepts and clusters as being “all related.” By comparison, the Science Literate participants’ maps had more clusters and more links, but used fewer post-it notes than the lay participants. They rarely described what the links meant in much detail, although some did discuss them being “influential.” Finally, the Scientists’ maps had the greatest number of clusters and links, but used the fewest post-it notes; each ‘post-it’ note descriptor conveyed a collection of ideas or represented more complex concepts. Scientists also had clearer ideas about how the different clusters were related to each other or interacted with each other. In general, the Scientist group were also much more confident in their description and narration of their mental map.
The greater organization of clusters in our more scientifically experienced participants’ mental maps thus echoes the findings in the expertise literature described above about pattern detection and recognition, and in particular ‘feature chunking’. However, Klein (2015a) notes that experience does not automatically translate to expertise, and we saw this too in the variety of maps produced within each group. It is likely these differences may represent the domain within which individuals developed their experience of science and scientific uncertainty. For example, some scientists may have greater expertise in natural hazards science in general, rather than having expertise in scientific uncertainty and risk specifically, and, thus, might produce relatively few post-it notes, and simpler maps. Similarly, some of the lay public participants were highly articulate, produced many post-it notes, and required very little prompting to elicit more ideas and produced complex maps. This may represent a greater experience and expertise in understanding risk and uncertainty even if they have less expertise in NHE science. The latter is an example of how knowledge from other domains (including self-taught knowledge or problem-solving processes) can also produce complex and sophisticated, but still relevant mental models, even in the absence of subject matter expertise. Dunbar (2000) observed similar, finding that scientists use analogy to fill gaps in current knowledge, such as mentally considering a similar problem that they may have solved elsewhere and importing that knowledge into a current problem.
As well as the explicit training-based knowledge, tacit knowledge3 also influences the differences between Scientists, Science-Literate, and the Lay-Public (as described for experts and novices in the summary above). Following Polanyi (1967), Spiekermann et al. (2015) describe tacit knowledge as “a different kind of knowledge, a hidden, implicit, or silent knowledge” (p. 98). An example of tacit knowledge would be our knowledge of how to balance when riding a bike: a skill that was learned through experience, but once learnt does not require explicit thinking to achieve and would be hard to explain or articulate to another. In the context of natural hazard events, an example of tacit knowledge could be the intuitive feel that an individual may have as to how an event may evolve or the impacts that may occur, such as “how the weather should normally be in their locales at certain times of the year” (Meisch et al., 2022, p. 1). This experience- and context-based knowledge is hard to articulate or share with individuals who may be “at a loss for words” when describing it (ibid, p. 4). It compares to explicit or defined knowledge which is learnt through study and training and is more easily articulated and shared, and is often captured in research reports or organizational policies. Spiekermann et al. (2015) propose that more effective integration of tacit and practical knowledge based on local experience may help address some of the key challenges in disaster risk reduction, encouraging us to move beyond focusing on addressing a (research-based) knowledge gap, and focus instead on a recognition of existing knowledge, supported by an understanding of priorities and needs such that target-oriented methods of communication can be developed (see also Doyle and Becker, 2022).
Recognizing these other concerns also helps explain why concepts have different priorities across our groups and individuals (Sec. 3.4), with, for example, some listing trust and data as ‘sub’ nodes while others position those as master nodes. The prioritization of the nodes relates to the participants perceptions of other influences, such as societal factors, “power structures, personal attitudes, values, world views” (Spiekermann et al., 2015, p. 107) Thus, while the level of experience of science, expertise-based knowledge, and/or tacit knowledge, help inform the content, structure, and complexity of the model, the prioritization of concepts within the model will be informed by a participant’s perception of other societal factors alongside their personal concerns and needs (see, e.g., Doyle et al., 2023). To enhance science communication, it is thus important not just to identify the expert, training, or experiential knowledge an audience may have, but also to identify ways to understand their tacit knowledge which may also influence their interpretation of a message.
Differences also emerged in the language used by our participants in their mental model maps and discussions, with Lay Public maps being more focused on safety and what is out of their control when it comes to preparing for the hazard that the science is advising on. Meanwhile the Scientists maps focused on formal modeling of risk and the likelihood of events and impacts. Similarly, participants described similar issues in their nodes, but used different names for those issues (Section 3.4, Tables 2, 3). In addition, some nodes had similar titles, but were discussed differently with different elements within, reflecting how these concepts were viewed through different lenses by participants, being understood and focused on differently. This is consistent with prior research which has shown that the public often perceives, and responds to, risk differently from those who assess, manage, and communicate it (see, e.g., Fischhoff, 2009). Powell and Leiss (1997) interpreted such differences in terms of a ‘public’ language (grounded in social and intuitive knowledge) and an ‘expert’ language (grounded in scientific, statistical knowledge). The different levels of expertise and training, or type of tacit knowledge, will inform the words people use to define concepts and the language they use to define the nuance around how they relate to other concepts.
Language, and the terms used to describe concepts or clusters of concepts representing sources of uncertainty, thus can become both an important tool for effective communication as well as a barrier to that communication (Bullock et al., 2019). For example, linguistic terms can be used to help organize and chunk the concepts into a pattern or structure (see Sections 4.1), where someone’s expertise enables them to make a mental shortcut and rapidly group items under a known concept, while those without such expertise may struggle to articulate the concepts they perceive. However, such specialist terms readily become technical jargon, unfamiliar, and inaccessible to others. As discussed by Renn (2008, p. 212), such specialized language is often intended to transmit precise messages to peers and is not intended to convey information to public.
While it is well known that jargon differences can create barriers for public communication (Bullock et al., 2019), these differences can also cause communication errors to occur between (non-public) peer science groups and is often overlooked in communication planning. This occurs due to different usages of the same jargon term, or if the term represents complex cluster of concepts for some, compared to more surface-level concepts for others (such as the difference between the language we observed used in post-it concepts versus in the higher-level master nodes, Table 3). This difference in understanding represents a linguistic uncertainty (Walker et al., 2003; Grubler et al., 2015; Doyle et al., 2019). Due to the challenges associated with this, there have been numerous calls for a simplification of jargon and a common understanding of terminology when communicating across disciplines, such as AoNZ’s Plain Language Act.4 Given the wide range of individuals involved in natural hazards risk management, such an approach is particularly important. To achieve this effectively requires understanding an audience’s or collaborator’s perspectives on risk and uncertainty, their use of terminology, and their information needs, prior to communication (see also Doyle and Becker, 2022). The mental model techniques used herein thus also offer a tool to develop shared understanding of each other’s perspectives, alongside other participatory and relationship building tools.
As well as the lessons learnt regarding the differences in language and the structure of the mental models with varying levels of science expertise (Sections 4.1 and 4.2), the differences in the content of the maps provide important lessons for disaster risk communication specifically (see Section 3.4). The Lay Public participants prioritized several communication factors more explicitly than did other participants, such as the role of trust. They discussed the importance of trusting the source research or agency, the reputation of the scientist, and the agency (government or independent), as well as having lower trust in media and friends, and that a scientist’s ability to communicate also influences the Lay Public’s perceived trustworthiness of that scientist. Research has demonstrated that we rely on trust in, experience of, and knowledge about, a communicator to judge communications when we do not fully understand the message (Renn and Levine, 1991; Hocevar et al., 2017; Johnson et al., 2023). Research has also shown that individuals use various indicators of trust as a heuristic to evaluate scientific information, such as recognizing an organization’s logo or stamp on a message or image (Bica et al., 2019). Any distrust in this agency or in the media carrying their message, due to political views or distrust of authority, can thus also impact this evaluation. This can be compounded in cases where there is alignment with the views of friends and families in a form of ‘identity motivated reasoning’ (Kahan, 2012). Our findings indicate trust in the communicator or agency is also important for how our Lay Participants characterize the uncertainty associated with the science advice, as well as the overall message. In comparison, the scientists focused their reasoning more on the inherent NHE phenomena, methods, and knowledge.
Given most of our Lay Public appear to base their understanding on their personal experiences and public releases of information, announcements, summaries in the media and social media, the NHE information they construct their mental models from is inherently simplified along scientific dimensions, compared to the information used by and familiar to active or previously-active/trained scientists. Thus, to make meaning from this information and help reconcile any gaps in information or understanding, they thus draw on these other ‘observable’ features such as who the scientist is, and their prior experience of that communicator (Johnson et al., 2023). The Lay Public’s use of these other factors to understand (and respond to) information has also been recognized in various risk communication models (see section 1), including the Risk Information Seeking and Processing Model that recognizes people draw on a range of social, psychological, and communicative factors in seeking and interpreting information (Yang et al., 2014), the Community Engagement Theory model that highlights the importance of trust for effective risk communication (Paton, 2019), and the IDEA model’s internalization and explanation factors (whether people see the risk as relevant to them, understand it, and think it is trustworthy and credible; Sellnow et al., 2017).
We thus suggest that to improve effective communication of scientific uncertainty to broad audiences, further research should explore the heuristics that individuals may use to infer the presence or not of scientific uncertainties, and identify what features they use to judge or infer those uncertainties, including exploring how their personal political views, their judgment of an agency, or their judgment of a communicator’s political stance or personality may influence their characterization and understanding of the uncertainty present.
This use of these other nuanced ‘observable’ features may also be an additional explanation for why the Lay Public’s mental models appear less organized, shallower, and less structured (Sections 3.4 and 4.1); while the scientists’ models have a deeper more nuanced and complex structure. This reliance on judging the communicator to resolve uncertainty may be particularly influential when scientific advice rapidly evolves and people have less time to evaluate associated information. However, this can create an ongoing communication challenge, as such rapid evolution of advice can also be perceived by the Lay Public, and represented in the media, as due to the scientists repeatedly changing their mind (e.g., Capurro et al., 2021) rather than a rapidly changing situation. In comparison, an expert scientist would ideally have more theoretical knowledge alongside practical experience-based knowledge (Spiekermann et al., 2015), enabling them to understand the underlying principles and evolving evidence resulting in this changing message, and use that knowledge to resolve overall uncertainty rather than relying on their trust and judgment of the science communicator.
Thus, for effective communication, this research suggests there is a need to consider including more information about the process behind the science output to help non-scientists make sense of science and uncertainty (Donovan and Oppenheimer, 2016); and develop a form of accessible theoretical knowledge to aid understanding and increase trust in the science (De Groeve, 2020), perhaps through messaging that helps individuals develop more coherent and structured mental models. This is particularly important when the science being communicated has changed due to a change in risk, new information, or updated analysis; and when a dialog around the reasons behind those changes can help develop trust, as referenced by the Lay Public in their mental model maps. When the information needs to be disseminated in a one-way approach (see section 1) including such process information could help facilitate comprehension, important if there is no opportunity to develop such understanding of process through two-way deliberate discussions (Doyle and Becker, 2022). However, there is a delicate balance between providing enough information to help people understand and update their mental model, and providing too much information that it can result in information overload and confusion (Eppler and Mengis, 2004). This is particularly important when decisions need to be made under high pressure or tight time constraints (Endsley, 1997). Thus, it is more reasonable to provide this information about the science process during non-crisis times, such as for longer term risk and forecast decisions, or during periods of preparedness and resilience building (Doyle and Becker, 2022). During an acute phase, consideration should also be given to people’s proximity to the affected region, as those more distal may have more capacity to understand this additional information than those directly affected. This is true for both less experienced and experienced individuals and some of the Scientist group also discussed the issue of too much information, and managing large volumes of information, more so than the other two groups, including ‘more information increases uncertainty’ on their maps.
Klein (2015b) similarly discuss how experts’ decision performance goes down when too much information is gathered, or when information is received in a way that is not useful or is difficult to interpret (Doyle and Becker, 2022). Thus, addressing, or resolving, decision uncertainty due to absence of data cannot always be solved by gathering more information. The need to provide the right volume and type of information reiterates our earlier recommendation (Section 4.2) to first understand an audience’s perspectives and needs before communicating; and to understand that decision makers will reconcile information based on other factors alongside their mental models and theoretical understanding, such as using contextual and practical knowledge to discern what information is relevant (Spiekermann et al., 2015), as well as judging and interpreting information based on personal, social, cultural, and organizational factors (Doyle and Becker, 2022).
There are several limitations that must be considered in this exploratory study. The relational links in the Lay Public collated map were interpreted based on the maps produced by this group as well as the interview discussion, and not restricted to the links explicitly drawn by the participants themselves; the comments by half the group that their clusters were ‘all related’ guided the inference of connections where none were explicitly drawn. Although this method of creating a collated map is not unusual, as it is how implicit mental models (compared to explicit mental models) are drawn (see review in Doyle et al., 2022), it differentiates the Lay Public master map from the other two while also demonstrating the differences in map and mental model organization between groups and the difficulty this group had articulating and drawing their mental models.
In future research, assessing absent relationship lines by participants could be investigated in several ways. A partial map could be produced and presented to participants as a starting point for their own map (e.g., Cassidy and Maule, 2012), or similarly to produce the components of the maps (the ‘nodes’) and ask participants to brainstorm the links, as the M-Tool does (van den Broek et al., 2021). However, while such prompting may help participants identify, articulate, and draw the relationships between clusters, having such prior explicit prompts can introduce researcher influence into the final output (Romolini et al., 2012; Doyle et al., 2022). In addition, the difficulty the Lay Participants had drawing these relationships represents one of our findings, showing that less experienced individuals have less organization of their map and mental model, such that it appears ‘fuzzier’ or less refined. Thus, rather than force someone to make an unstructured model ‘clearer’ with additional links, it might be more instructive to ask participants to explicitly describe the relationship links they do produce via direct questions about those relationships, such as asking them to describe why two concepts are similar or linked to each other, but are different and unlinked from a third as a form of card sorting exercise similar to Ben-Zvi Assaraf et al. (2012), see also Morgan et al. (2001).
Other limitations that indicate future research include: (a) the restrictions on participant recruitment and method due to Covid-19 limiting use of broader public interviews and the use of in-person group elicitation mental model techniques; (b) the need to consider the participants’ prior experience of scientific agencies or communicators, and how that influences their models; (c) the need in future to explore how these maps might vary between different natural hazard phenomena (e.g., flooding vs. earthquakes) or scientific domains (e.g., climate change vs. public health), or dependent and cascading hazards (e.g., changes to flood risk post-earthquake); (d) the need to consider temporal changes in the location and type of uncertainty (see also Doyle et al., 2023); and (e) the need to recruit more Lay participants whose highest qualification was High School or less, such that we can more broadly explore how formal education level (beyond science experience and expertise) impacts perspectives. There is also a need, (f), to identify how the participants prioritize the different drivers of uncertainty and risk, to identify what they see as the primary and secondary sources, and to understand what that means for communication. Finally, we propose that research should explore how the provision of information to support pattern recognition may help support effective science communication, by enabling those with less organized mental models to structure or organize the communicated science and update their mental models to enhance their understanding.
Managing natural hazards risk necessitates scientific advice and information from a broad range of disciplines. This is then incorporated into decision-making processes by individuals with a diverse range of knowledge, experience, training, and background, including the public, planners, policy makers, and government and response agencies, and the wider public. Individuals draw on a range of factors to interpret this information, including their mental models of the issue, their disciplinary training and expertise, their experience of and trust in the communicator, and other personal, social, and cultural factors.
Our study, exploring a comparative analysis of individuals’ mental model maps of scientific uncertainty produced by individuals with varying levels of training and experience in science, indicates increasing organization of maps and mental models with increasing scientific literacy. Greater training or experience with science results in a more structured mental model of natural hazard related scientific uncertainty, even if the former is in a discipline distinct from natural hazards. This process is akin to the ‘chunking’ process identified in other domains, where individuals progressively collect features into chunks and recognize and prioritize patterns as their level of experience increases. Those with more theoretical knowledge, due to experience and training, use this to assess scientific information. Meanwhile, those with less experience focus on features of the situation to facilitate interpretation, relying less on knowledge of patterns or sequences. The less experienced thus drew on other features, or knowledge, to support interpretation of scientific uncertainty and characterize their mental models. This was also reflected in substantive differences observed between the different groups, such as Lay Public participants focusing more on perceptions of control, safety, and trust as sources of uncertainty, while the Scientists focused more on formal models of risk and likelihood. However, those with less science experience in this domain may use tacit or theoretical knowledge from other domains to rationalize and understand scientific information. Our findings thus indicate that for effective communication, communicators should not only develop an understanding of audiences’ priorities and needs relevant to the problem domain, but should also recognize that an audience may draw their knowledge and language from across domains to help interpret uncertain information, which may influence their interpretation of a message.
The datasets presented in this article are not readily available because the interview data is confidential and viewable only by the research team due to ethical requirements. Requests to access the datasets should be directed to Emma Hudson-Doyle, ZS5lLmh1ZHNvbi1kb3lsZUBtYXNzZXkuYWMubno=.
The data collection method for this study received peer-reviewed approval under Massey University’s code of ethical conduct for low risk research, teaching, and evaluations involving human participants (Application ID 4000023593), consistent with our University’s processes for low risk research. The studies were conducted in accordance with the local legislation and institutional requirements. The participants provided their written informed consent to participate in this study. No potentially identifiable images or data are presented in this study.
ED: Conceptualization, Data curation, Formal analysis, Funding acquisition, Investigation, Methodology, Project administration, Resources, Supervision, Visualization, Writing – original draft, Writing – review & editing. JT: Data curation, Formal analysis, Investigation, Methodology, Project administration, Visualization, Writing – original draft, Writing – review & editing. SRH: Conceptualization, Formal analysis, Methodology, Writing – original draft, Writing – review & editing. MW: Conceptualization, Formal analysis, Methodology, Writing – original draft, Writing – review & editing. DP: Conceptualization, Methodology, Writing – original draft. SEH: Conceptualization, Methodology, Writing – original draft, Writing – review & editing. AB: Conceptualization, Formal analysis, Methodology, Writing – original draft, Writing – review & editing. JB: Conceptualization, Formal analysis, Methodology, Writing – original draft, Writing – review & editing.
Sadly, co-author Douglas Paton passed away during this research, on 24 April 2023. He will be missed by many, and we are forever grateful for the invaluable insights and laughter Douglas shared with us as a friend and colleague. Tributes can be shared at https://www.forevermissed.com/profdouglas-paton/about.
The author(s) declare that financial support was received for the research, authorship, and/or publication of this article. This research was supported by Massey University Research funding 2020, 2021, and 2022, and public research funding from the Government of Aotearoa New Zealand. EEHD is supported by the National Science Challenges: Resilience to Nature’s Challenges Kia manawaroa – Ngā Ākina o Te Ao Tūroa 2019–2024; and (partially) supported by QuakeCoRE Te Hiranga Rū – Aotearoa NZ Centre for Earthquake Resilience 2021, an Aotearoa New Zealand Tertiary Education Commission-funded Centre.
The authors would like to thank our interview participants for their vital contributions to this research. We thank the three reviewers for their very helpful and constructive feedback that have improved this manuscript.
The authors declare that the research was conducted in the absence of any commercial or financial relationships that could be construed as a potential conflict of interest.
The reviewer DA declared a shared affiliation with the authors ED, JT, SH, MW, and JB to the handling editor at the time of review.
All claims expressed in this article are solely those of the authors and do not necessarily represent those of their affiliated organizations, or those of the publisher, the editors and the reviewers. Any product that may be evaluated in this article, or claim that may be made by its manufacturer, is not guaranteed or endorsed by the publisher.
1. ^https://www.mural.co/ (last accessed 2nd Dec 2023).
2. ^Pākehā means “New Zealander of European descent” in the Māori language, the indigenous language of Aotearoa New Zealand (Te Aka Māori dictionary, https://maoridictionary.co.nz/, last accessed 2nd Dec 2023). Individuals may define Pākehā as representing people with European ancestry and who are of multiple generations in Aotearoa New Zealand, and thus being distinct from more recent European immigrants.
3. ^See also “tacit knowledge: The informal understandings of individuals (especially their social knowledge) which they have not verbalized and of which they may not even be aware, but which they may be inferred to know (notably from their behavior). This includes what they need to know or assume in order to produce and make sense of messages (social and textual knowledge)”. Oxford Reference. Retrieved 10 Dec. 2023, from https://www.oxfordreference.com/view/10.1093/oi/authority.20110803101844995.
4. ^In AoNZ the Plain Language Act came into effect for Public Service agencies and Crown agents in 2022, to improve accessibility for the public to documents and communications (see https://www.legislation.govt.nz/act/public/2022/0054/latest/whole.html last accessed 4th Dec 2023).
Abel, N., Ross, H., and Walker, P. (1998). Mental models in rangeland research, communication and management. Rangel. J. 20, 77–91. doi: 10.1071/RJ9980077
Baber, C. (2019). “Is expertise all in the mind?How embodied, embedded, enacted, extended, situated, and distributed theories of cognition account for expert performance” in The Oxford handbook of expertise. eds. P. Ward, J. M. Schraagen, and E. M. Roth (Oxford, UK: Oxford University Press), 243–261.
Barsalou, L. W. (2008). Grounded cognition. Annu. Rev. Psychol. 59, 617–645. doi: 10.1146/annurev.psych.59.103006.093639
Bauer, M. W., Allum, N., and Miller, S. (2007). What can we learn from 25 years of PUS survey research? Liberating and expanding the agenda. Public Underst. Sci. 16, 79–95. doi: 10.1177/0963662506071287
Becker, J. S., Potter, S. H., McBride, S. K., Wein, A., Doyle, E. E. H., and Paton, D. (2019). When the earth doesn’t stop shaking: how experiences over time influenced information needs, communication, and interpretation of aftershock information during the Canterbury earthquake sequence, N.Z. Int. J. Disaster Risk Reduction 34, 397–411. doi: 10.1016/j.ijdrr.2018.12.009
Becker, J. S., Vinnell, L. J., McBride, S. K., Nakayachi, K., Doyle, E. E. H., Potter, S. H., et al. (2022). The effects of earthquake experience on intentions to respond to earthquake early warnings. Front. Commun. (Lausanne) 7:7004. doi: 10.3389/fcomm.2022.857004
Ben-Zvi Assaraf, O., Eshach, H., Orion, N., and Alamour, Y. (2012). Cultural differences and students’ spontaneous models of the water cycle: a case study of Jewish and Bedouin children in Israel. Cult. Stud. Sci. Educ. 7, 451–477. doi: 10.1007/s11422-012-9391-5
Bica, M., Demuth, J. L., Dykes, J. E., and Palen, L. (2019). Communicating hurricane risks: multi-method examination of risk imagery diffusion. In: Conference on Human Factors in Computing Systems–Proceedings.
Bostrom, A. (2017). Mental models and risk perceptions related to climate change. Clim. Sci. 2017:303. doi: 10.1093/acrefore/9780190228620.013.303
Bostrom, A., Anselin, L., and Farris, J. (2008). Visualizing seismic risk and uncertainty. Ann. N. Y. Acad. Sci. 1128, 29–40. doi: 10.1196/annals.1399.005
Bostrom, A., Fischhoff, B., and Morgan, M. G. (1992). Characterizing mental models of hazardous processes: a methodology and an application to radon. J. Soc. Issues 48, 85–100. doi: 10.1111/j.1540-4560.1992.tb01946.x
Bostrom, A., Morss, R. E., Lazo, J. K., Demuth, J. L., Lazrus, H., and Hudson, R. (2016). A mental models study of hurricane forecast and warning production, communication, and decision-making. Weather Clim. Soc. 8, 111–129. doi: 10.1175/WCAS-D-15-0033.1
Braun, V., Clarke, V., Hayfield, N., Davey, L., and Jenkinson, E. (2022). “Doing reflexive thematic analysis” in Supporting research in counselling and psychotherapy (Cham: Springer International Publishing), 19–38.
Brewer, W. F. (1999). Scientific theories and naive theories as forms of mental representation: psychologism revived. Sci. Educ. (Dordr) 8, 489–505. doi: 10.1023/A:1008636108200
Bullock, O. M., Colón Amill, D., Shulman, H. C., and Dixon, G. N. (2019). Jargon as a barrier to effective science communication: evidence from metacognition. Public Underst. Sci. 28, 845–853. doi: 10.1177/0963662519865687
Capurro, G., Jardine, C. G., Tustin, J., and Driedger, M. (2021). Communicating scientific uncertainty in a rapidly evolving situation: a framing analysis of Canadian coverage in early days of COVID-19. BMC Public Health 21:2181. doi: 10.1186/s12889-021-12246-x
Cassidy, A., and Maule, J. (2012). “Risk communication and participatory research: ‘fuzzy felt’, visual games and group discussion of complex issues” in Visual Methods in Psychology: Using and Interpreting Images in Qualitative Research. ed. P. Reavey (London: Routledge), 205–222.
Chi, M. T. H. (2006). Two approaches to the study of experts’ characteristics. In: K. A. Ericsson, R. R. Hoffman, A. Kozbelt, and A. M. Williams The Cambridge Handbook of Expertise and Expert Performance. Cambridge, UK: Cambridge University Press, pp. 21–30.
Chi, M. T. H., Feltovich, P. J., and Glaser, R. (1981). Categorization and representation of physics problems by experts and novices*. Cogn. Sci. 5, 121–152. doi: 10.1207/s15516709cog0502_2
Collins, A., and Gentner, D. (1987). How people construct mental models. In: A. Holland and N. Quinn Cultural Models in Language and Thought. Cambridge, UK: Cambridge University Press, pp. 243–266.
Cook, J., Sellnow, T. L., Sellnow, D. D., Parrish, A. J., and Soares, R. (2021). “Communicating the science of COVID-19 to children: meet the helpers” in Communicating Science in Times of Crisis: The COVID-19 Pandemic. eds. H. Dan O’Hair and M. J. O’Hair (Hoboken, NJ: John Wiley and Sons)
Covello, V. T., and Sandman, P. M. (2001). “Risk communication: evolution and revolution” in Solutions to an environment in peril. ed. A. Wolbarst (Baltimore: John Hopkins University Press), 164–178.
De Groeve, T. (2020). “Knowledge-based crisis and emergency management” in Science for policy handbook. ed. M. Sienkiewicz (Amsterdam, Netherlands: Elsevier), 182–194.
Donovan, A., and Oppenheimer, C. (2016). Resilient science: the civic epistemology of disaster risk reduction. Sci. Public Policy 43, 363–374. doi: 10.1093/scipol/scv039
Doyle, E. E. H., and Becker, J. S. (2022). “Understanding the risk communication puzzle for natural hazards and disasters” in Oxford research encyclopedia of natural Hazard science. ed. E. E. E. Doyle (Oxford, United Kingdom: Oxford University Press)
Doyle, E. E. H., Harrison, S. E., Hill, S. R., Williams, M., Paton, D., and Bostrom, A. (2022). Eliciting mental models of science and risk for disaster communication: a scoping review of methodologies. Int. J. Disaster Risk Reduction 77:103084. doi: 10.1016/J.IJDRR.2022.103084
Doyle, E. E. H., Johnston, D. M., Smith, R., and Paton, D. (2019). Communicating model uncertainty for natural hazards: a qualitative systematic thematic review. Int. J. Disaster Risk Reduction 33, 449–476. doi: 10.1016/j.ijdrr.2018.10.023
Doyle, E. E. H., McClure, J., Paton, D., and Johnston, D. M. (2014). Uncertainty and decision making: volcanic crisis scenarios. Int. J. Disaster Risk Reduction 10, 75–101. doi: 10.1016/j.ijdrr.2014.07.006
Doyle, E. E. H., Thompson, J., Hill, S., Williams, M., Paton, D., Harrison, S., et al. (2023). Where does scientific uncertainty come from, and from whom? Mapping perspectives of natural hazards science advice. Int. J. Disaster Risk Reduction 96:103948. doi: 10.1016/j.ijdrr.2023.103948
Dreyfus, H. L. (2002). Intelligence without representation-Merleau-Ponty’s critique of mental representation the relevance of phenomenology to scientific explanation. Phenomenol. Cogn. Sci. 1, 367–383. doi: 10.1023/A:1021351606209
Dunbar, K. (2000). How scientists think in the real world: implications for science education. J. Appl. Dev. Psychol. 21, 49–58. doi: 10.1016/S0193-3973(99)00050-7
Eiser, J. R., Bostrom, A., Burton, I., Johnston, D. M., McClure, J., Paton, D., et al. (2012). Risk interpretation and action: a conceptual framework for responses to natural hazards. Int. J. Disaster Risk Reduction 1, 5–16. doi: 10.1016/j.ijdrr.2012.05.002
Endsley, M. R. (1997). “The role of situation awareness in naturalistic decision making” in Naturalistic decision making. eds. C. E. Zsambok and G. Klein (Mahwah, NJ: Lawrence Erlbaum Associates), 269–284.
Endsley, M. R. (2006). “Expertise and situation awareness” in The Cambridge handbook of expertise and expert performance (Cambridge, UK: Cambridge University Press), 633–652.
Eppler, M. J., and Mengis, J. (2004). The concept of information overload: a review of literature from organization science, accounting, marketing, MIS, and related disciplines. Inf. Soc. 20, 325–344. doi: 10.1080/01972240490507974
Epstein, S. (1994). Integration of the cognitive and the psychodynamic unconscious. Am. Psychol. 49, 709–724. doi: 10.1037/0003-066X.49.8.709
Fischhoff, B. (1995). Risk perception and communication unplugged: twenty years of process. Risk Anal. 15, 137–145. doi: 10.1111/j.1539-6924.1995.tb00308.x
Fischhoff, B. (2009). “Risk perception and communication” in Oxford textbook of public health. eds. R. Detels, R. Beaglehole, M. A. Langsang, and M. Gulliford. 5th ed (Oxford: Oxford University Press), 940–952.
Fischhoff, B., and Davis, A. L. (2014). Communicating scientific uncertainty. Proc. Natl. Acad. Sci. 111, 13664–13671. doi: 10.1073/pnas.1317504111
Fisher, A. (1991). Risk communication challenges. Risk Anal. 11, 173–179. doi: 10.1111/j.1539-6924.1991.tb00590.x
Freudenburg, W. R., Gramling, R., and Davidson, D. J. (2008). Scientific certainty argumentation methods (SCAMs): science and the politics of doubt*. Sociol. Inq. 78, 2–38. doi: 10.1111/J.1475-682X.2008.00219.X
Gentner, D., and Gentner, D. R. (1983). “Flowing waters or teeming crowds: mental models of electricity” in Mental models. eds. D. Gentner and A. L. Stevens (Hillsdale, NJ: Lawrence Erlbaum Associates), 99–129.
Greca, I. M., and Moreira, M. A. (2000). Mental models, conceptual models, and modelling. Int. J. Sci. Educ. 22, 1–11. doi: 10.1080/095006900289976
Griffin, R. J., Dunwoody, S., and Neuwirth, K. (1999). Proposed model of the relationship of risk information seeking and processing to the development of preventive behaviors. Environ. Res. 80, S230–S245. doi: 10.1006/enrs.1998.3940
Grima, J., Leal Filho, W., and Pace, P. (2010). Perceived frameworks of young people on global warming and ozone depletion. J. Balt. Sci. Educ. 9, 35–49.
Grubler, A., Ermoliev, Y., and Kryazhimskiy, A. (2015). Coping with uncertainties-examples of modeling approaches at IIASA. Technol. Forecast Soc. Change 98, 213–222. doi: 10.1016/j.techfore.2015.06.004
Gurabardhi, Z., Gutteling, J. M., and Kuttschreuter, M. (2005). An empirical analysis of communication flow, strategy and stakeholders’ participation in the risk communication literature 1988-2000. J. Risk Res. 8, 499–511. doi: 10.1080/13669870500064192
Hocevar, K. P., Metzger, M., and Flanagin, A. J. (2017). “Source credibility, expertise, and Trust in Health and Risk Messaging” in Oxford research encyclopedia of communication. ed. K. P. Hocevar (Oxford, UK: Oxford University Press)
Hogan, K., and Maglienti, M. (2001). Comparing the epistemological underpinnings of students’ and scientists’ reasoning about conclusions. J. Res. Sci. Teach. 38, 663–687. doi: 10.1002/tea.1025
Huang, S. K., Lindell, M. K., and Prater, C. S. (2016). Who leaves and who stays? A review and statistical meta-analysis of hurricane evacuation studies. Environ. Behav. 48, 991–1029. doi: 10.1177/0013916515578485
Johnson, B. B., Mayorga, M., and Dieckmann, N. F. (2023). How people decide who is correct when groups of scientists disagree. Risk Anal. doi: 10.1111/RISA.14204
Johnson, B. B., and Slovic, P. (1995). Presenting uncertainty in health risk assessment: initial studies of its effects on risk perception and trust. Risk Anal. 15, 485–494. doi: 10.1111/j.1539-6924.1995.tb00341.x
Johnson-Laird, P. N. (2010). Mental models and human reasoning. Proc. Natl. Acad. Sci. U. S. A. 107, 18243–18250. doi: 10.1073/pnas.1012933107
Jones, N. A., Ross, H., Lynam, T., Perez, P., and Leitch, A. (2011). Mental models: an interdisciplinary synthesis of theory and methods. Ecol. Soc. 16:art46. doi: 10.5751/ES-03802-160146
Kahan, D. M. (2012). Ideology, motivated reasoning, and cognitive reflection: an experimental study. SSRN Electron. J. 424, 407–424. doi: 10.2139/ssrn.2182588
Kappel, K., and Holmen, S. J. (2019). Why science communication, and does it work? A taxonomy of science communication aims and a survey of the empirical evidence. Front. Commun. (Lausanne) 4:55. doi: 10.3389/fcomm.2019.00055
Kearney, A., and Kaplan, S. (1997). Toward a methodology for the measurement of knowledge structures of ordinary people: the conceptual content cognitive map. Environ. Behav. 29, 579–617. doi: 10.1177/0013916597295001
Klein, G. A. (2015a). A naturalistic decision making perspective on studying intuitive decision making. J. Appl. Res. Mem. Cogn. 4, 164–168. doi: 10.1016/j.jarmac.2015.07.001
Klein, G. A. (2015b). Reflections on applications of naturalistic decision making. J. Occup. Organ. Psychol. 88, 382–386. doi: 10.1111/joop.12121
Klein, G. A., Associates, K., and Ara, D. (2008). Naturalistic decision making. Hum. Fact. 50, 456–460. doi: 10.1518/001872008X288385
Klein, G. A., and Hoffman, R. R. (1993). “Seeing the invisible: perceptual-cognitive aspects of expertise” in Cognitive science foundations of instruction. ed. M. Rabinowitz (Springdale, NJ: Lawrence Erlbaum Associates, Inc.), 203–226.
Kostelnick, J. C., McDermott, D., Rowley, R. J., and Bunnyfield, N. (2013). A cartographic framework for visualizing risk. Cartographica 48, 200–224. doi: 10.3138/carto.48.3.1531
Kovaka, K. (2019). Climate change denial and beliefs about science. Synthese 2019:2210. doi: 10.1007/s11229-019-02210-z
Lajium, D. A. D., and Coll, R. K. (2014). Mental models in science education. International Conference on Education.
Leiss, W. (1996). Three phases in the evolution of risk communication practice. Ann. Am. Acad. Pol. Soc. Sci. 545, 85–94. doi: 10.1177/0002716296545001009
Levine, J., Muthukrishna, M., Chan, K. M. A., and Satterfield, T. (2015). Theories of the deep: combining salience and network analyses to produce mental model visualizations of a coastal British columbia food web. Ecol. Soc. 20. doi: 10.5751/ES-08094-200442
Levy, M. A., Lubell, M. N., and McRoberts, N. (2018). The structure of mental models of sustainable agriculture. Nat. Sustain. 1, 413–420. doi: 10.1038/s41893-018-0116-y
Lindell, M. K., and Perry, R. W. (2000). Household adjustment to earthquake Hazard: a review of research. Environ. Behav. 32, 461–501. doi: 10.1177/00139160021972621
Maxim, L., and Mansier, P. (2014). How is scientific credibility affected by communicating uncertainty? The case of endocrine disrupter effects on male fertility. Hum. Ecol. Risk Assess. Int. J. 20, 201–223. doi: 10.1080/10807039.2012.719387
Medin, D. L., and Bang, M. (2014). The cultural side of science communication. Proc. Natl. Acad. Sci. 111, 13621–13626. doi: 10.1073/pnas.1317510111
Meisch, S. P., Bremer, S., Young, M. T., and Funtowicz, S. O. (2022). Extended peer communities: appraising the contributions of tacit knowledges in climate change decision-making. Futures 135:102868. doi: 10.1016/j.futures.2021.102868
Miles, S., and Frewer, L. J. (2003). Public perception of scientific uncertainty in relation to food hazards. J. Risk Res. 6, 267–283. doi: 10.1080/1366987032000088883
Miller, S. (2001). Public understanding of science at the crossroads. Public Underst. Sci. 10, 115–120. doi: 10.1088/0963-6625/10/1/308
Morgan, M. G., Fischhoff, B., Bostrom, A., and Altman, C. J. (2001). Risk communication: A mental models approach. London: Cambridge University Press.
Morrow, B. H., Lazo, J. K., Rhome, J., and Feyen, J. (2015). Improving storm surge risk communication: stakeholder perspectives. Bull. Am. Meteorol. Soc. 96, 35–48. doi: 10.1175/BAMS-D-13-00197.1
Morton, T. A., Rabinovich, A., Marshall, D., and Bretschneider, P. (2011). The future that may (or may not) come: how framing changes responses to uncertainty in climate change communications. Glob. Environ. Chang. 21, 103–109. doi: 10.1016/j.gloenvcha.2010.09.013
Olofsson, A., and Rashid, S. (2011). The white (male) effect and risk perception: can equality make a difference? Risk Anal. 31, 1016–1032. doi: 10.1111/j.1539-6924.2010.01566.x
Oreskes, N. (2015). The fact of uncertainty, the uncertainty of facts and the cultural resonance of doubt. Philos. Trans. R. Soc. A Math. Phys. Eng. Sci. 373:20140455. doi: 10.1098/rsta.2014.0455
Paton, D. (2019). Disaster risk reduction: psychological perspectives on preparedness. Aust. J. Psychol. 71, 327–341. doi: 10.1111/AJPY.12237
Paton, D., and Buergelt, P. (2019). Risk, transformation and adaptation: ideas for reframing approaches to disaster risk reduction. Int. J. Environ. Res. Public Health 16:2594. doi: 10.3390/ijerph16142594
Polanyi, M. (1958). Personal knowledge: Towards a post-critical philosophy. Chicago: University of Chicago Press.
Powell, D., and Leiss, W. (1997). Mad cows and mother’s milk. Montreal: McGill-Queen’s University Press.
Rabinovich, A., and Morton, T. A. (2012). Unquestioned answers or unanswered questions: beliefs about science guide responses to uncertainty in climate change risk communication. Risk Anal. 32, 992–1002. doi: 10.1111/j.1539-6924.2012.01771.x
Rapp, D. N. (2005). “Mental models: theoretical issues for visualizations in science education” in Visualization in science education. ed. J. K. Gilbert (Dordrecht: Springer Netherlands), 43–60.
Renn, O. (2014). Four questions for risk communication: a response to Roger Kasperson. J. Risk Res. 17, 1277–1281. doi: 10.1080/13669877.2014.940601
Renn, O., and Levine, D. (1991). “Credibility and trust in risk communication” in Communicating risks to the public (Dordrecht: Springer Netherlands), 175–217.
Romolini, M., Brinkley, W., and Wolf, K. L. (2012). What is urban environmental stewardship? Constructing a practitioner-derived framework. Washington, DC: USDA Forest Service - Research Note PNW-RN, pp. 1–40.
Schumacher, R. M., and Czerwinski, M. P. (1992). Mental models and the Acquisition of Expert Knowledge. Psychol. Exp. 1, 61–79. doi: 10.1007/978-1-4613-9733-5_4
Sellnow, D. D., Jones, L. M., Sellnow, T. L., Spence, P., Lane, D. R., and Haarstad, N. (2019). “The IDEA model as a conceptual framework for designing earthquake early warning (EEW) messages distributed via Mobile phone apps” in Earthquakes - impact, community vulnerability and resilience. ed. J. Santos-Reyes (London, UK: IntechOpen)
Sellnow, D. D., Lane, D. R., Sellnow, T. L., and Littlefield, R. S. (2017). The IDEA model as a best practice for effective instructional risk and crisis communication. Commun. Stud. 68, 552–567. doi: 10.1080/10510974.2017.1375535
Sellnow, D. D., and Sellnow, T. L. (2019). The IDEA model for effective instructional risk and crisis communication by emergency managers and other key spokespersons. J. Emerg. Manag. 17, 67–78. doi: 10.5055/jem.2019.0399
Smithson, M. (1999). Conflict aversion: preference for ambiguity vs conflict in sources and evidence. Organ. Behav. Hum. Decis. Process. 79, 179–198. doi: 10.1006/obhd.1999.2844
Spiekermann, R., Kienberger, S., Norton, J., Briones, F., and Weichselgartner, J. (2015). The disaster-knowledge matrix – reframing and evaluating the knowledge challenges in disaster risk reduction. Int. J. Disaster Risk Reduction 13, 96–108. doi: 10.1016/j.ijdrr.2015.05.002
Sword-Daniels, V., Eriksen, C., Hudson-Doyle, E. E., Alaniz, R., Adler, C., Schenk, T., et al. (2018). Embodied uncertainty: living with complexity and natural hazards. J. Risk Res. 21, 290–307. doi: 10.1080/13669877.2016.1200659
Tayeebwa, D. W., Wendo, D. C., and Nakiwala, D. A. S. (2022). “Theories and models of science communication” in Science Communication Skills for Journalists, vol. 10 (Wallingford, UK: CABI), 0002.
Teigen, K. H., and Brun, W. (1999). The directionality of verbal probability expressions: effects on decisions, predictions, and probabilistic reasoning. Organ. Behav. Hum. Decis. Process. 80, 155–190. doi: 10.1006/obhd.1999.2857
Tripto, J., Assaraf, O. B.-Z., and Amit, M. (2013). Mapping what they know: concept maps as an effective tool for assessing students’ systems thinking. Am. J. Oper. Res. 3, 245–258. doi: 10.4236/ajor.2013.31a022
Tripto, J., Ben-Zvi Assaraf, O., Snapir, Z., and Amit, M. (2016). The ‘what is a system’ reflection interview as a knowledge integration activity for high school students’ understanding of complex systems in human biology. Int. J. Sci. Educ. 38, 564–595. doi: 10.1080/09500693.2016.1150620
Van den Broek, K. L., Klein, S. A., Luomba, J., and Fischer, H. (2021). Introducing M-tool: a standardised and inclusive mental model mapping tool. Syst. Dyn. Rev. 37, 353–362. doi: 10.1002/SDR.1698
Veil, S., Reynolds, B., Sellnow, T. L., and Seeger, M. W. (2008). CERC as a theoretical framework for research and practice. Health Promot. Pract. 9, 26S–34S. doi: 10.1177/1524839908322113
Vinnell, L. J., Hudson-Doyle, E. E., Inch, P., Tan, M. L., Becker, J. S., and Johnston, D. M. (2022). Evacuation behavior and information needs of Wellington, Aotearoa New Zealand residents following the 5 march 2021 mw 7.3 east cape earthquake. Seismol. Res. Lett. 93, 1452–1463. doi: 10.1785/0220210286
Vinnell, L. J., Tan, M. L., Prasanna, R., and Becker, J. S. (2023). Knowledge, perceptions, and behavioral responses to earthquake early warning in Aotearoa New Zealand. Front. Commun. (Lausanne) 8:247. doi: 10.3389/fcomm.2023.1229247
Walker, W., Harremoes, P., Rotmans, J., Van der Sluijs, J., Van Asselt, M., Janssen, P., et al. (2003). Defining uncertainty: a conceptual basis for ucnertainty management in model-based decision support. Integr. Assess. 4, 5–17. doi: 10.1076/iaij.4.1.5.16466
Wiedemann, P., Schtz, H., and Thalmann, A. (2008). “Perception of uncertainty and communication about unclear risks” in The role of evidence in risk characterization. eds. P. Wiedemann and H. Schutz (Weinheim, Germany: Wiley-VCH Verlag GmbH and Co. KGaA), 161–183.
Keywords: uncertainty, science communication, mental models, natural hazards, expertise, tacit knowledge
Citation: Doyle EEH, Thompson J, Hill SR, Williams M, Paton D, Harrison SE, Bostrom A and Becker JS (2024) Differences in perceived sources of uncertainty in natural hazards science advice: lessons for cross-disciplinary communication. Front. Commun. 9:1366995. doi: 10.3389/fcomm.2024.1366995
Received: 08 January 2024; Accepted: 08 March 2024;
Published: 04 April 2024.
Edited by:
Serena Tagliacozzo, National Research Council (CNR), ItalyReviewed by:
Deanna Dee Sellnow, University of Central Florida, United StatesCopyright © 2024 Doyle, Thompson, Hill, Williams, Paton, Harrison, Bostrom and Becker. This is an open-access article distributed under the terms of the Creative Commons Attribution License (CC BY). The use, distribution or reproduction in other forums is permitted, provided the original author(s) and the copyright owner(s) are credited and that the original publication in this journal is cited, in accordance with accepted academic practice. No use, distribution or reproduction is permitted which does not comply with these terms.
*Correspondence: Emma E. H. Doyle, ZS5lLmh1ZHNvbi1kb3lsZUBtYXNzZXkuYWMubno=
†Deceased
Disclaimer: All claims expressed in this article are solely those of the authors and do not necessarily represent those of their affiliated organizations, or those of the publisher, the editors and the reviewers. Any product that may be evaluated in this article or claim that may be made by its manufacturer is not guaranteed or endorsed by the publisher.
Research integrity at Frontiers
Learn more about the work of our research integrity team to safeguard the quality of each article we publish.