- School of Occupational Therapy, Faculty of Medicine, Hebrew University of Jerusalem, Jerusalem, Israel
Purpose: Metacognition, or the ability to monitor the performance of oneself, is known for its fundamental importance for human behavior adjustments. However, studies of metacognition in social behaviors focused on emotion recognition are relatively scarce. In the current study, we aimed to examine the effectiveness of metacognition, measured by self-rated confidence in voice emotion recognition tasks within healthy individuals.
Methods: We collected 180 audio-recorded lexical sentences portraying discrete emotions: anger, happiness, sadness, fear, surprise, and neutrality expressions. Upon listening to voice stimuli, participants (N = 100; 50 females, 50 males) completed the perception task of recognition of emotion. After each trial, a confidence rating (CR) was assigned.
Results: A series of one-tailed t-tests showed that the differences in mean CRs assigned to correct and incorrect performances were significant for all emotions and neutral expression.
Conclusions: Our preliminary results demonstrate efficiency in metacognition of emotion recognition by voice. Theoretically, our results support the difference between accuracy in metacognition, measured by CR, and efficiency in metacognition, as it specified the CR between correct and incorrect performance. To gain better insights into practical issues, further studies are needed to examine whether and what are the differences between accuracy and efficiency in metacognition, as part of social communication.
Introduction
Metacognition refers to the capacity to reflect on, monitor, and control cognitive processes, such as decision-making, memory, and perception (Flavell, 1979). Efficient metacognition commonly represents better self-assessment for one ability. While measuring metacognition ability may be achieved by assigning self-report confidence rating in a given task, efficiency in metacognition is commonly achieved by studying the difference in CR between correct and incorrect performance (Polyanskaya, 2023). Over decades, researchers have been motivated to learn the extensive role metacognition plays in executing adaptive human behaviors, mainly focusing on education and learning aspects (Baer and Kidd, 2022), with relatively less interest in emotional perception (Laukka et al., 2021; Rahnev, 2023). However, studying efficiency in metacognition is still scarce. Given the fundamental role metacognition took in emotion perception as a perceptional process, a better understanding of measuring efficiency in metacognition of emotion recognition is much needed. To fill this gap in the literature, in this preliminary study we aimed to investigate metacognitive efficiency in emotion recognition focusing on voice communication, across different emotions category.
Metacognitive efficiency
Efficient metacognition generally reflects a better introspection of cognitive process. Specifically, effective metacognition is suggested to promote effectiveness in noticing relevant cues and to seek additional information when necessary (Drigas and Mitsea, 2020; Brus et al., 2021). Therefore, metacognition is considered foundational to flexible and adaptive behavior in a range of settings (Baer and Kidd, 2022; Katyal and Fleming, 2024). Furthermore, promoting efficient metacognition by guided intervention had a positive impact on discrimination, working memory, and spatial tasks (Carpenter et al., 2019; McWilliams et al., 2023). A widely used method to study metacognition is to have observers do a challenging task followed by an explicit confidence judgment regarding their task performance (McWilliams et al., 2023). This retrospective CR is further used as a measure of monitoring effectiveness, when a larger difference in CRs assigned to correct and incorrect performances reflects the ability to estimate the likelihood of the observer making an error (Rahnev, 2021; Polyanskaya, 2023). Based on CR measurement, metacognitive efficiency is sometimes considered domain-specific, meaning that one's ability to effectively evaluate their correctness in performance is specific to the object under scrutiny. Hence, individuals can also have high awareness of their performance in one cognitive domain while having limited awareness of their performance in another (Rouault et al., 2018). As such, CRs for correct performance were lower in the perceptual task (i.e., visuospatial task) than in the memory task (Pennington et al., 2021). However, less studies posited a domain-general metacognitive competence based on audio, visual and audio visual modalities (Ais et al., 2016; Carpenter et al., 2019). Together, inconsistency in findings highlights the importance of independent metacognitive efficiency study for a range of domains and skills. Yet, most research remains focused on general knowledge and learning domains, with less interest in social domains (Saoud and Al-Marzouqi, 2020).
Metacognitive efficiency in emotion recognition
As metacognition contributes to accurate performance in other perception tasks, few researchers sought to gain further insights into its potential contribution to accurate perception of others' emotions (Jeckeln et al., 2022; Michel, 2023). Emotion perception competence refers to the ability to perceive and interpret (i.e., decode) relevant social–emotional cues expressed by others (i.e., to encode; Bänziger and Scherer, 2007). Accurate perception of others' feeling and thinking is a key skill associated with achieving successful interpersonal relationships (Mitsea et al., 2022). In the visual channel, for example, authors revealed that higher CR was associated with higher accuracy in the facial emotional recognition task, underscoring the fundamental role of CR in the adjustments of social behavior (Bègue et al., 2019; Garcia-Cordero et al., 2021; Folz et al., 2022; Katyal and Fleming, 2024), whereas metacognitive dysfunction has been implicated as a key source of maladaptive behavior regarding emotional perception (Arslanova et al., 2023).
When it comes to voice communication, studying efficiency in metacognition of emotional perception is relatively small in amount. Voice expressions can be either verbal (e.g., words and sentences) or nonverbal (e.g., screaming, laughing), where all have proven effective in conveying emotions (Eyben et al., 2015). In general, voice comprises unique audible features called prosody. Prosody is an important feature of language, mainly represented by intonation (i.e., how low or high a voice is perceived), loudness (i.e., how loud a sound is perceived), and tempo (i.e., how quickly an utterance is produced; Mitchell et al., 2003). Prosody also conveys important information about a speaker's sex, age, attitude, and mental state (Schwartz and Pell, 2012). Lausen and Hammerschmidt (2020) conducted one of the few studies examining CR's role in vocal emotion perception. They found that participants' confidence judgments increased with increasing correctness in emotion recognition tasks that included verbal and nonverbal stimuli. As a result, they speculated that specific characteristics of voiced stimuli affect CRs. They also found that meaning played a significant role in performance confidence as a higher confidence rating was assigned to stimuli containing lexical-semantic content (e.g., nouns and lexical sentences) rather than stimuli without meaning (e.g., pseudonouns and pseudosentences). More recently, Laukka et al. (2021) extended the concept of CRs to include multimodal analysis. On the basis of visual, audio, and visual–audio channels, the researchers also found a strong correlation between emotional recognition accuracies and CRs, in each channel (Laukka et al., 2021).
While the above studies demonstrate the importance of metacognitive accuracy, based on CR measurement, as far as we know, no study focused on metacognitive efficiency. In the current study, we deal for the first time with efficiency in metacognition concerning voice emotion recognition. Specifically, we asked whether correct performance in emotion recognition test would yield significant higher CR, compared to CR assigned to incorrect performance? As we intend to focus on voice communication, we adhere to previous suggestions that gender roles should be studied within a given societal context, particularly language (Keshtiari and Kuhlmann, 2016; Hall et al., 2021). As a result, we used an emotional target originally designed in Hebrew (Sinvani and Sapir, 2022) in the form of repeated lexical sentences, reflecting discrete emotions (anger, sadness, happiness, fear, and surprise) as well as neutral expression. In this way, we intended to focus on emotional prosodic cues separately from semantic cues. Earlier studies have discussed such methodologies (Paulmann and Kotz, 2008; Sinvani and Sapir, 2022), with particular recommendations in the diagnostic analysis of nonverbal accuracy 2 (DANVA-2), emphasizing the independent role of prosodic cues in emotion recognition (Nowicki and Duke, 2008). To answer our question, we used performance-based tests for emotion recognition by voice, followed by CR reported trial by trial. For the purpose of metacognitive efficiency detection, we set the mean CR scores for correct to incorrect performances. We hypothesis to find significant difference between CR assigned to correct and incorrect performance, where higher CR would be assigned to the correct performance. By hypothesizing that, we actually expect to illustrate here efficient in metacognition across all tested emotions, perceived by voice.
Materials and methods
Recording stage
Participants
A sample of recorders (i.e., encoders) comprised of 30 participants (16 men; 14 women). All encoders were actors (15 professional; 15 non-professional), native Hebrew speakers and were either undergraduate or graduate students at Haifa University, 20–35 years of age (M = 25.07, SD = 2.29). They had normal hearing, which was verified by a standard audiological assessment for pure tones from 0.5 to 8 kHz (Koerner and Zhang, 2018). None had any history of speech, language, or hearing disorders or psychiatric illnesses.
Procedure
Before the experiment commenced, participants were given a description of the experimental procedure and their tasks. Participants then signed a consent form and completed a short demographic questionnaire concerning age and gender. The study procedure included a brief hearing test and a recording stage. All procedures were conducted in the same acoustic room in the Interdisciplinary Clinics Center at Haifa University. Stimuli were repeated lexical sentence in Hebrew, previously reported in Sinvani and Sapir (2022), meaning “Oh really? I can't believe it!” [/Ma/Be/e/met/A/ni/lo//Ma/a/mi/na/]. Here, the same experimenter (first author) instructed the encoders to vocally enact the repeated target sentence in five discrete emotions: anger, happiness, sadness, fear, and surprise. The elicitation of audio samples followed a design of 30 actors × five emotions × 1 sentence, resulting in 150 emotional utterances. In addition, each of the 30 actors uttered the sentence in a neutral, non-emotional fashion, yielding additional 30 neutral stimuli. The speech recordings were obtained in a sound-proof booth using a head-mounted condenser microphone (AKG C410) positioned 10 cm and 45–50° from the left oral angle. The signal was pre-amplified, low pass-filtered at 9.8 kHz and digitized to a computer hard disk at a sampling rate of 22 kHz (Stipancic and Tjaden, 2022), using Cool Edit Pro version 2.0. All audio clips were auditory scanned to ensure adequate amplitude and no missing data (i.e., speakers produced all utterances). The full set of audio clips is available from the authors upon request.
Perceptual tests: emotion recognition task and SRC
Participants
The sample of listeners (i.e., decoders) comprised of 100 participants (50 men; 50 women). All decoders were native Hebrew speakers and were either undergraduate or graduate students at Haifa University, 20–35 years of age (M = 24.37, SD = 2.28). All had normal hearing, which was verified by a standard audiological assessment for pure tones from 0.5 to 8 kHz (Koerner and Zhang, 2018). None had any history of speech, language, or hearing disorders or psychiatric illnesses.
Procedure
Before the experiment commenced, participants were given a description of the experimental procedure and their tasks. Participants then signed a consent form and completed a short demographic questionnaire concerning age and gender. The study procedure included a brief hearing test and an emotion recognition task, followed by retrospective SRC regarding emotion recognition accuracy. All procedures were conducted in the same acoustic room in the Interdisciplinary Clinics Center at Haifa University.
Ten decoders (five men; five women) were invited to each experimental session, which lasted ~10 min. Upon arrival, the experimenter informed the participants about the aim and procedure of the study. Decoders were informed that each stimulus would be presented only once. For each experimental session, 10 decoders judged the same 18 target stimuli, created by three encoders. In each session, targets were randomized in order. In addition, decoders were blinded to the ratings of the remaining decoders, as each decoder sat alone in the acoustic room with headphones for the entire procedure. Stimuli were presented to the participants binaurally via Sennheiser HD 448 headphones plugged in the tower box of a Dell OptiPlex 780 SFF desktop PC computer. In total, the perceptual test generated 1,800 results for ERA and 1,800 results for SRC.
Measures
Voice emotion recognition task
Emotion recognition accuracy was assessed using a performance-based test in a categorical manner. Upon listening to each auditory stimulus, decoders marked one of six categories: happiness, anger, sadness, fear, surprise, or neutrality. As innate and universal emotions, these five categories are frequently referred to as basic emotions (Ekman, 1992; Siedlecka and Denson, 2019). Moreover, we included neutral expressions, as commonly done in the literature on voice emotional recognition (Lausen and Schacht, 2018; Lin et al., 2021). In accordance with the DANVA-2 paradigm, we implemented a repeat lexical sentence designed in Hebrew, meaning “Oh really?” “I can't believe it!” [/Ma/Be/e/met/A/ni/lo//Ma/a/mi/na/]. This target was used in a previous study conducted in our lab (Sinvani and Sapir, 2022). In a preliminary test, we confirmed that identification percentages were significantly greater than chance level, in each of the tested category (Figure 1).
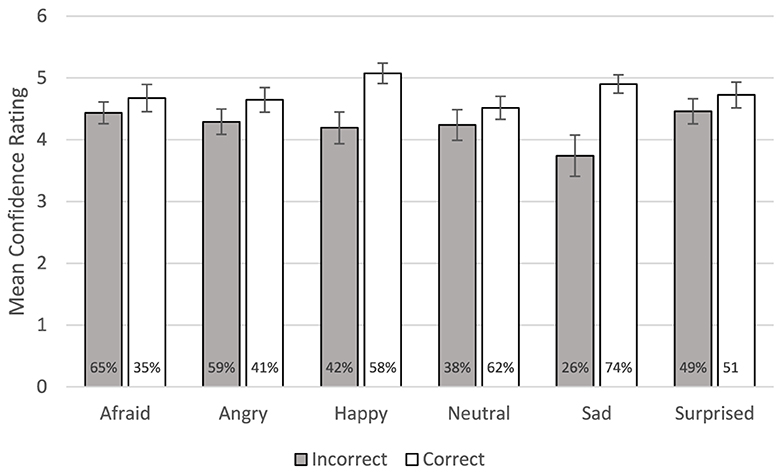
Figure 1. CRs assigned to correct and incorrect performance by the tested emotions and neutrality expression emotions. We provide the accuracy of each tested category (emotions and neutral) in the bottom portion of each bar. For example, 35% of the judgments were correct and 65% were incorrect for expressions of fear.
Retrospective SRC
CR was measured retrospectively by self-reported own confidence in a given response (in the voice emotion recognition task). Based on a Likert-type scale from 0 (not at all confident) to 6 (extremely confident), decoders rated how confident they were in their emotion category judgments. A similar 7-point scale was used in a previous study (Bègue et al., 2019) to report SRC in facial expression recognition.
Ethics
The IRB Ethics Committee of the University of Haifa, Israel approved the study. Participants were recruited using convenience sampling through social media advertisements and word of mouth. Professional and non-professional actors consented to the anonymous use of their shortened recordings in our study. Actors in the recording stage and participants in the perceptual test were paid 20 NIS for their participation.
Data analysis
Statistical analysis was conducted using IBM SPSS (Statistical Package for the Social Sciences) version 28.0. For all analyses, p < 0.05 was considered statistically significant. Independent-samples t-tests were conducted to examine the differences in CRs by the performance (correct/incorrect) across all emotions. The significance is reported controlling for false discovery rate for multiple comparisons (Benjamini and Hochberg, 1995).
Results
We examined metacognitive efficiency in the magnitude of the difference in CRs assigned to correct (i.e., where target emotion fits the response emotion) and incorrect (i.e., where target emotion was different from response emotion) performances for all decoders.
Figure 1 shows that correct performances were assigned higher confidence than incorrect performances for all emotions. A series of one-tailed t-tests showed that the differences in mean CRs assigned to correct and incorrect performances were significant for all emotions and neutral expression (Table 1).
Discussion
In the current preliminary study, we investigated the metacognition efficiency of an emotion recognition task performance, conveyed by voice. To this end, we analyzed differences in CRs between correct and incorrect performance in the emotion recognition task, based on identical vocal lexical sentences portrayed into emotional prosodies of anger, happiness, sadness, surprise and fear, and neutral expression. Following the literature dealing with the global concept of metacognitive efficiency, we expected to demonstrate a greater difference between CRs assigned to correct and incorrect performances, thus illustrating a convenient representation of metacognitive efficacy. As far as we know, this is the first study conducted to examine efficiency in metacognition skills concerning emotional recognition by voice.
As predicted, our results indicate a consistent difference in CRs between correct and incorrect performance, where higher CRs were significantly assigned for correct performances. In accordance with previous studies, our findings support the general established finding for metacognition efficiency in diverse cognitive tasks (Ais et al., 2016; Carpenter et al., 2019). To our knowledge, only two studies have been conducted to examine metacognitive ability based on retrospective confidence rating for vocal emotion recognition (Lausen and Hammerschmidt, 2020; Laukka et al., 2021). Although these studies based their examination for metacognitive skill on the measure of CR in a given response, representing metacognitive accuracy, they ignore the difference in CR between correct to incorrect performance, comprising the metacognitive efficiency.
Following extensive study focusing on brain mechanism underlying correctness in emotion recognition tasks, one of the worth noting question concerns the neural selectivity is if it is determined by the emotion presented by encoder or by the emotion perceived by a decoder (Wang et al., 2014). Based on face stimuli, Wang et al. (2014) concluded that neurons in the human amygdala encode the perceived judgment of emotions rather than the stimulus features themselves. Recently, Zhang et al. (2022) further showed that responses of anterior insula and amygdala were driven by the perceived vocal emotions. However, they revealed that responses of Heschl's gyrus and posterior insula (i.e., both involved in auditory perception) were determined by the presented emotion. Together, it was speculated that extensive connectivity of the amygdala with other brain regions allows it to modulate a few functions essential in adaptive and socio-emotional behaviors (Whitehead, 2021; Atzil et al., 2023). Given the fundamental role metacognition (i.e., confidence) plays in adaptive and socio-emotional behaviors, our results highlight the further need for incorporating neuroscience study into neural mechanism underlying self-confidence to distinguish correct and incorrect performance in emotion recognition task. This study has theoretical and practical significance. From the theoretic point of view, our results confirm earlier studies concerning various cognitive processes by establishing that individuals are capable of efficiently assessing their performance in voice emotion recognition (Cho, 2017; Skewes et al., 2021; Polyanskaya, 2023). Moreover, given the significant association that was indicated across all emotions and neutral expressions, we speculated metacognitive efficiency of being individual “fingerprint”, rather than domain-specific (Wang et al., 2014; Ais et al., 2016; Zhang et al., 2022). Additional studies should incorporate neuroscience methodologies in diverse channels of emotion communication to specify the mechanism of efficiently introspecting vocal emotion recognition to gain support for this claim (Wang et al., 2014; Whitehead, 2021; Zhang et al., 2022; Atzil et al., 2023).
From the practice point of view, in light of previous results showing the increase in performance found among students after they participate in guided intervention for metacognitive awareness (Nunaki et al., 2019), our findings should be taken one step further regarding designing interventions focused on emotion recognition through voice. Unlike vocal channel which deserved much less attention, several studies demonstrated the effectiveness of practicing facial emotion recognition skill as beneficial to social interactions (Chaidi and Drigas, 2020; Dantas and do Nascimento, 2022a,b). Importantly, those works highlight the flexibility of facial emotion recognition skill which in our opinion justify comprehensive research in the voice channel. In addition, our findings may consider valuable by diagnosis means, as extensive research dealt with metacognitive maladaptive in diverse subclinical and clinical populations (Hoven et al., 2019).
Although our study offers a valuable contribution to the current understanding of metacognition in social communication of emotions, some limitations should be noted. First, to investigate emotional recognition, we used a lexical sentence as a repeated item. Therefore, one can argue that prosodic cues are more important than semantics. However, lexical sentences can still affect perception and confidence by contributing meaning (Lausen and Hammerschmidt, 2020). Therefore, researchers should consider various types of stimuli for a better generalization of findings regarding efficiency in metacognition of emotion recognition by voice. Second, it is important to note that our investigation was blinded to acoustical parameters, which have been documented as contributing to the CR discussed elsewhere (Lausen and Hammerschmidt, 2020). Spectral parameters and prosodic contours (Shaqra et al., 2019) would be valuable tools for future research, allowing researchers to detect physiological changes in voice and emotional expressions.
Conclusions
Our study offers a preliminary investigation of metacognitive efficiency in emotion recognition. As far as we know this is the first study to focus on CR for measuring efficacy in emotion recognition task, and not just accuracy in metacognition. Our findings highlight the need for better understand in how individuals utilize metacognitive efficiency in their social interactions. We recommend further studies to investigate efficiency by comparing different groups and different channels of emotion communication. As a result of futural studies we will be able to understand the distinct contribution of efficiency over accuracy in social emotional communication as a fundamental for one's mental health.
Data availability statement
The raw data supporting the conclusions of this article will be made available by the authors, without undue reservation.
Ethics statement
The studies involving humans were approved by the IRB Ethics Committee of the University of Haifa, Israel. The studies were conducted in accordance with the local legislation and institutional requirements. The participants provided their written informed consent to participate in this study.
Author contributions
R-TS: Conceptualization, Data curation, Formal analysis, Methodology, Writing—original draft, Writing—review & editing. HF-G: Conceptualization, Data curation, Formal analysis, Writing – original draft.
Funding
The author(s) declare that no financial support was received for the research, authorship, and/or publication of this article.
In Memoriam
The authors feel honored to dedicate this work in memory of Professor Shimon Sapir and his inspiring leadership and vigorous role in advancing acoustic speech analysis.
Conflict of interest
The authors declare that the research was conducted in the absence of any commercial or financial relationships that could be construed as a potential conflict of interest.
Publisher's note
All claims expressed in this article are solely those of the authors and do not necessarily represent those of their affiliated organizations, or those of the publisher, the editors and the reviewers. Any product that may be evaluated in this article, or claim that may be made by its manufacturer, is not guaranteed or endorsed by the publisher.
References
Ais, J., Zylberberg, A., Barttfeld, P., and Sigman, M. (2016). Individual consistency in the accuracy and distribution of confidence judgments. Cognition. 146, 377–386. doi: 10.1016/j.cognition.2015.10.006
Arslanova, I., Meletaki, V., Calvo-Merino, B., and Forster, B. (2023). Perception of facial expressions involves emotion specific somatosensory cortex activations which are shaped by alexithymia. Cortex. 167, 223–234. doi: 10.1016/j.cortex.2023.06.010
Atzil, S., Satpute, A. B., Zhang, J., Parrish, M. H., Shablack, H., MacCormack, J. K., et al. (2023). The impact of sociality and affective valence on brain activation: a meta-analysis. Neuroimage. 268, 119879. doi: 10.1016/j.neuroimage.2023.119879
Baer, C., and Kidd, C. (2022). Learning with certainty in childhood. Trends Cogn Sci. 26, 887–896. doi: 10.1016/j.tics.2022.07.010
Bänziger, T., and Scherer, K. R. (2007). Using actor portrayals to systematically study multimodal emotion expression: The GEMEP corpus. In: Affective Computing and Intelligent Interaction: Second International Conference, ACII 2007. Lisbon: Springer.
Bègue, I., Vaessen, M., Hofmeister, J., Pereira, M., Schwartz, S., Vuilleumier, P., et al. (2019). Confidence of emotion expression recognition recruits brain regions outside the face perception network. Soc. Cognit. Affect. Neurosci. 14, 81–95. doi: 10.1093/scan/nsy102
Benjamini, Y., and Hochberg, Y. (1995). Controlling the false discovery rate: a practical and powerful approach to multiple testing. J. R. Stat. Soc. B. 57, 289–300. doi: 10.1111/j.2517-6161.1995.tb02031.x
Brus, J., Aebersold, H., Grueschow, M., and Polania, R. (2021). Sources of confidence in value-based choice. Nat Commun. 12, 7337. doi: 10.1038/s41467-021-27618-5
Carpenter, J., Sherman, M. T., Kievit, R. A., Seth, A. K., Lau, H., Fleming, S. M., et al. (2019). Domain-general enhancements of metacognitive ability through adaptive training. J. Exp. Psychol. Gen. 148, 51. doi: 10.1037/xge0000505
Chaidi, I., and Drigas, A. (2020). Autism, Expression, and Understanding of Emotions: Literature Review. International Association of Online Engineering. Retrieved from: https://www.learntechlib.org/p/218023/ (accessed February 25, 2024).
Cho, S-. Y. (2017). Explaining Gender Differences in Confidence and Overconfidence in Math. doi: 10.2139/ssrn.2902717
Dantas, A., and do Nascimento, M. (2022b). Face emotions: improving emotional skills in individuals with autism. Mul. Tools Appl. 81, 25947–25969. doi: 10.1007/s11042-022-12810-6
Dantas, A. C., and do Nascimento, M. Z. (2022a). Recognition of emotions for people with autism: an approach to improve skills. Int J Comp Games Technol. 2022, 1–21. doi: 10.1155/2022/6738068
Drigas, A., and Mitsea, E. (2020). The 8 pillars of metacognition. Int. J. Emerg. Technol. Learn. 15, 162–178. doi: 10.3991/ijet.v15i21.14907
Ekman, P. (1992). An argument for basic emotions. Cogn Emot. 6, 169–200. doi: 10.1080/02699939208411068
Eyben, F., Scherer, K. R., Schuller, B. W., Sundberg, J., Andr,é, E., Busso, C., et al. (2015). The Geneva minimalistic acoustic parameter set (GeMAPS) for voice research and affective computing. IEEE Trans. Affect. Comput. 7, 190–202. doi: 10.1109/TAFFC.2015.2457417
Flavell, J. H. (1979). Metacognition and cognitive monitoring: a new area of cognitive–developmental inquiry. Am. Psychol. 34, 906. doi: 10.1037/0003-066X.34.10.906
Folz, J., Akdağ, R, Nikolić, M, van Steenbergen, H., and Kret, M. E. (2022). Facial mimicry and metacognitive judgments in emotion recognition-modulated by social anxiety and autistic traits? Available online at: https://psyarxivcom/e7w6k/ (accessed 26, 2022).
Garcia-Cordero, I., Migeot, J., Fittipaldi, S., Aquino, A., Campo, C. G., García, A., et al. (2021). Metacognition of emotion recognition across neurodegenerative diseases. Cortex. 137, 93–107. doi: 10.1016/j.cortex.2020.12.023
Hall, K., Borba, R., and Hiramoto, M. (2021). Language and gender. Int. Encycl. Linguis. Anthropol. 2021, 892–912. doi: 10.1002/9781118786093.iela0143
Hoven, M., Lebreton, M., Engelmann, J. B., Denys, D., Luigjes, J., and van Holst, R. J. (2019). Abnormalities of confidence in psychiatry: an overview and future perspectives. Transl. Psychiatry 9:268. doi: 10.1038/s41398-019-0602-7
Jeckeln, G., Mamassian, P., and O'toole, A. J. (2022). Confidence judgments are associated with face identification accuracy: findings from a confidence forced-choice task. Behav. Res. Methods. 2022, 1–10. doi: 10.31234/osf.io/3dxus
Katyal, S., and Fleming, S. M. (2024). The future of metacognition research: balancing construct breadth with measurement rigor. Cortex 171, 223–234. doi: 10.1016/j.cortex.2023.11.002
Keshtiari, N., and Kuhlmann, M. (2016). The effects of culture and gender on the recognition of emotional speech: evidence from Persian speakers living in a collectivist society. Int. J. Soc. Cult. Lang. 4, 71. doi: 10.13140/RG.2.1.1159.0001
Koerner, T. K., and Zhang, Y. (2018). Differential effects of hearing impairment and age on electrophysiological and behavioral measures of speech in noise. Hear. Res. 370, 130–142. doi: 10.1016/j.heares.2018.10.009
Laukka, P., Bänziger, T., Israelsson, A., Cortes, D. S., Tornberg, C., Scherer, K. R., et al. (2021). Investigating individual differences in emotion recognition ability using the ERAM test. Acta. Psychol. 220, 103422. doi: 10.1016/j.actpsy.2021.103422
Lausen, A., and Hammerschmidt, K. (2020). Emotion recognition and confidence ratings predicted by vocal stimulus type and prosodic parameters. Hum. Soc. Sci. Commun. 7, 1–17. doi: 10.1057/s41599-020-0499-z
Lausen, A., and Schacht, A. (2018). Gender differences in the recognition of vocal emotions. Front. Psychol. 9, 882. doi: 10.3389/fpsyg.2018.00882
Lin, Y., Ding, H., and Zhang, Y. (2021). Gender differences in identifying facial, prosodic, and semantic emotions show category-and channel-specific effects mediated by encoder's gender. J. Speech Lang. Hear. Res. 64, 2941–2955. doi: 10.1044/2021_JSLHR-20-00553
McWilliams, A., Bibby, H., Steinbeis, N., David, A. S., and Fleming, S. M. (2023). Age-related decreases in global metacognition are independent of local metacognition and task performance. Cognition 235, 105389. doi: 10.1016/j.cognition.2023.105389
Michel, C. (2023). Towards a new standard model of concepts? Abstract concepts and the embodied mind: Rethinking grounded cognition, by Guy Dove, Oxford University Press, Oxford, 2022, 280. pp., $74.00, ISBN 9780190061975. Abingdon-on-Thames: Taylor & Francis.
Mitchell, R. L., Elliott, R., Barry, M., Cruttenden, A., and Woodruff, P. W. (2003). The neural response to emotional prosody, as revealed by functional magnetic resonance imaging. Neuropsychologia 41, 1410–1421. doi: 10.1016/S0028-3932(03)00017-4
Mitsea, E., Drigas, A., and Skianis, C. (2022). Breathing, attention & consciousness in sync: the role of breathing training, metacognition & virtual reality. Technium Soc Sci J. 29, 79. doi: 10.47577/tssj.v29i1.6145
Nowicki, S., and Duke, M. (2008). Manual for the Receptive Tests of the Diagnostic Analysis of Nonverbal Accuracy 2 (DANVA2). Atlanta, GA: Emory University.
Nunaki, J. H., Damopolii, I., Kandowangko, N. Y., and Nusantari, E. (2019). The effectiveness of inquiry-based learning to train the students' metacognitive skills based on gender differences. Int. J. Instruct. 12, 505–516. doi: 10.29333/iji.2019.12232a
Paulmann, S., and Kotz, S. A. (2008). An ERP investigation on the temporal dynamics of emotional prosody and emotional semantics in pseudo-and lexical-sentence context. Brain Lang. 105, 59–69. doi: 10.1016/j.bandl.2007.11.005
Pennington, C., Ball, H., Swirski, M., Newson, M., and Coulthard, E. (2021). Metacognitive performance on memory and visuospatial tasks in functional cognitive disorder. Brain Sci. 11, 1368. doi: 10.3390/brainsci11101368
Polyanskaya, L. (2023). I. know that I know. But do I know that I do not know? Front. Psychol. 14, 1128200. doi: 10.3389/fpsyg.2023.1128200
Rahnev, D. (2021). Visual metacognition: Measures, models, and neural correlates. Am. Psychol. 76, 1445. doi: 10.1037/amp0000937
Rahnev, D. (2023). Measuring metacognition: a comprehensive assessment of current methods. doi: 10.31234/osf.io/waz9h
Rouault, M., Seow, T., Gillan, C. M., and Fleming, S. M. (2018). Psychiatric symptom dimensions are associated with dissociable shifts in metacognition but not task performance. Biol. Psychiatry. 84, 443–451. doi: 10.1016/j.biopsych.2017.12.017
Saoud, L. S., and Al-Marzouqi, H. (2020). Metacognitive sedenion-valued neural network and its learning algorithm. IEEE Access. 8, 144823–144838. doi: 10.1109/ACCESS.2020.3014690
Schwartz, R., and Pell, M. D. (2012). Emotional speech processing at the intersection of prosody and semantics. PLoS ONE. 7, e47279. doi: 10.1371/journal.pone.0047279
Shaqra, F. A., Duwairi, R., and Al-Ayyoub, M. (2019). Recognizing emotion from speech based on age and gender using hierarchical models. Procedia. Comput. Sci. 151, 37–44. doi: 10.1016/j.procs.2019.04.009
Siedlecka, E., and Denson, T. F. (2019). Experimental methods for inducing basic emotions: a qualitative review. Emot. Rev. 11, 87–97. doi: 10.1177/1754073917749016
Sinvani, R.-T., and Sapir, S. (2022). Sentence vs. word perception by young healthy females: toward a better understanding of emotion in spoken language. Front. Glob. Women's Health. 3, 829114. doi: 10.3389/fgwh.2022.829114
Skewes, J., Frith, C., and Overgaard, M. (2021). Awareness and confidence in perceptual decision-making. Brain Multiphy. 2, 100030. doi: 10.1016/j.brain.2021.100030
Stipancic, K. L., and Tjaden, K. (2022). Minimally detectable change of speech intelligibility in speakers with multiple sclerosis and Parkinson's disease. J. Speech Lang. Hear. Res. 65, 1858–1866. doi: 10.1044/2022_JSLHR-21-00648
Wang, S., Tudusciuc, O., Mamelak, A. N., Ross, I. B., Adolphs, R., Rutishauser, U., et al. (2014). Neurons in the human amygdala selective for perceived emotion. Proc. Nat. Acad. Sci. 111, E3110–E9. doi: 10.1073/pnas.1323342111
Whitehead, J. C. (2021). Investigating the Neural Correlates of Social and Emotional Processing Across Modalities. Montreal, QC: McGill University.
Keywords: social interaction, language, voice communication, speech perception, self-confidence, prosody, metacognitive efficiency, metacognition
Citation: Sinvani R-T and Fogel-Grinvald H (2024) You better listen to yourself: studying metacognitive efficiency in emotion recognition by voice. Front. Commun. 9:1366597. doi: 10.3389/fcomm.2024.1366597
Received: 06 January 2024; Accepted: 19 February 2024;
Published: 12 March 2024.
Edited by:
Martina Micai, National Institute of Health (ISS), ItalyReviewed by:
Adilmar Coelho Dantas, Federal University of Uberlandia, BrazilYang Zhang, Johns Hopkins University, United States
Copyright © 2024 Sinvani and Fogel-Grinvald. This is an open-access article distributed under the terms of the Creative Commons Attribution License (CC BY). The use, distribution or reproduction in other forums is permitted, provided the original author(s) and the copyright owner(s) are credited and that the original publication in this journal is cited, in accordance with accepted academic practice. No use, distribution or reproduction is permitted which does not comply with these terms.
*Correspondence: Rachel-Tzofia Sinvani, rachel-tzofia.sinvani@mail.huji.ac.il