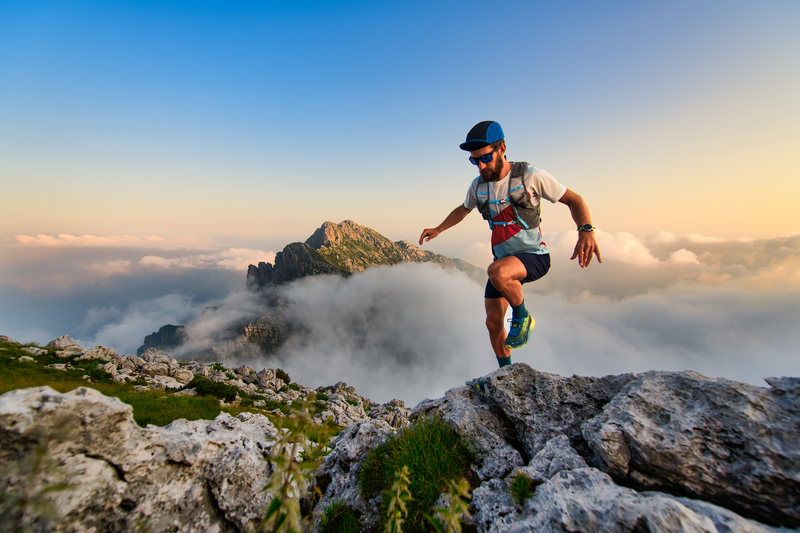
94% of researchers rate our articles as excellent or good
Learn more about the work of our research integrity team to safeguard the quality of each article we publish.
Find out more
ORIGINAL RESEARCH article
Front. Commun. , 24 April 2024
Sec. Multimodality of Communication
Volume 9 - 2024 | https://doi.org/10.3389/fcomm.2024.1356702
This article is part of the Research Topic Drawing Multimodality's Bigger Picture: Metalanguages and Corpora for Multimodal Analyses View all 15 articles
There is a need to develop new interdisciplinary approaches suitable for a more complete analysis of multimodal data. Such approaches need to go beyond case studies and leverage technology to allow for statistically valid analysis of the data. Our study addresses this need by engaging with the research question of how humans communicate about the future for persuasive and manipulative purposes, and how they do this multimodally. It introduces a new methodology for computer-assisted multimodal analysis of video data. The study also introduces the resulting dataset, featuring annotations for speech (textual and acoustic modalities) and gesticulation and corporal behaviour (visual modality). To analyse and annotate the data and develop the methodology, the study engages with 23 26-min episodes of the show ‘SophieCo Visionaries’, broadcast by RT (formerly ‘Russia Today’).
This article presents a new methodology for computer-assisted multimodal annotation and analysis of video data and introduces the resulting dataset. The development of this methodology constitutes a stepping stone in our attempt to answer an overarching research question about how humans communicate multimodally about different conceptions of the future for persuasive and manipulative purposes. Manipulation and persuasion constitute propaganda whenever the true intent of the message is not known to the audience (Jowett and O’Donnell, 2006). They are more effective when communicated multimodally (for review, see Wilson et al., 2023).
To analyse and annotate our data and develop our methodology, we engage with 23 26-min episodes of the RT ‘interview’ show ‘SophieCo Visionaries’. We focus on speech (textual and acoustic modalities) and gesticulation and corporal behaviour (visual modality).
We demonstrate our exploratory engagement with the data through a case study of how multimodal cues trigger the construction of meaning, stance, and viewpoint in a hypothetical future depiction by the RT show host (Section 2). The case study does not offer an exhaustive analysis but works to indicate where cues from different modalities are coordinated. It is one of many conducted to shape our approach and enable the development of our methodology and annotated dataset (Section 3). Although our study presents the case study, our methodology, and our dataset in a linear manner, the processes of conducting case studies, the creation of our annotated dataset, and the development of tools for automated annotation are interdependent and complementary.
Our empirical and data-driven approach is based on the fusion of knowledge and methods from cognitive linguistics, phonetics and phonology, gesture studies, and computer and engineering sciences. We work to find ‘ways of ‘combining’ insights from the variously imported theoretical and methodological backgrounds brought along by previous non-multimodal stages of any contributing discipline’ (Bateman, 2022a, p. 48). We go where the data take us, and do not disregard data that do not fit our hypotheses at the outset of our studies. We consider larger spoken discourse units with their prosodic features and gesticulation as they contribute to viewpoint construction at the semantic-syntactic and pragmatic levels. We rely on technology to speed up and scale up our analysis.
Our multimodal analysis is situated within the framework of conceptual integration/blending theory (Fauconnier and Turner, 2002), which it extends to investigate how multimodal cues—textual, acoustic, and gestural and corporal—trigger the construction of meaning, stance, and viewpoint in RT’s depictions of the future.
Notions of viewpoint and stance are often used interchangeably (Vandelanotte, 2017; Andries et al., 2023). We differentiate between the two, defining viewpoint as a key parameter of a multimodal setup or evoked mental space that represents a point of view of the Speaker or her Interlocutor at this given point in discourse. Viewpoint is ‘marked by just about anything that builds a particular individual’s mental space construal in ways specific to that individual’s cognitive and perceptual access’ (Sweetser, 2012, p. 7). We define stance as epistemic or evaluative constructs in relation to subjects, objects, or states of affairs and as a lower-level phenomenon than viewpoint, while simultaneously influencing configurations of viewpointed mental spaces. We see the viewpoints of the RT host and her guest as voices in Bakhtin’s sense (Bakhtin, 2013). We see their stances as blocks in the building of these voices. We use the term ‘stance construction’ rather than ‘stance-taking’ to reflect its key role in the construction of meaning and viewpoint (cf. Dancygier et al., 2019).
We incorporate in our research insights and methods from prosody and gesture studies, as well as from studies on the interaction of the two (for a literature review, see Loehr, 2014; for recent scholarship, see Pouw et al., 2023).
We use a theoretical approach for prosodic analysis and annotation grounded in the Autosegmental-Metrical approach to intonation (Pierrehumbert, 1980). It sits within a hierarchical theory of prosodic organisation, as expounded by, among others, Nespor and Vogel (1986), Hayes (1989), and Selkirk (2003). We approach our analysis of both prosody and prosody–gesture relations without any prescribed limits to our eventual interpretation, working with all the features together to account for multimodality.
Our interest in the conceptualisations of futures in speech and gesture motivates our interest in temporal gesture (for reviews, see Núñez and Cooperrider, 2013; Cooperrider et al., 2014). We see temporal gesture as belonging to the class of representational gestures, which are defined by Chu et al. as depicting ‘a concrete or abstract concept with the shape or motion of the hands [iconic gestures and metaphoric gestures in McNeill (1992), or point to a referent in the physical or imaginary space (concrete or abstract deictic gestures in McNeill (1992)’ (Chu et al., 2014, p. 2).
In our analysis of the speech–gesture relation, we draw upon the Information Packaging Hypothesis, which ‘states that gesturing helps the speaker organise information in a way suitable for linguistic expression’ (Kita, 2000, p. 180), with the organisation of information relying on collaboration between the speaker’s analytic and spatio-motoric thinking. We see gestures as communicating information (Hostetter, 2011). We define interactive gestures as referring ‘to the interlocutor rather than to the topic of conversation, and they help maintain the conversation as a social system’ (Bavelas et al., 1992, p. 469).
We treat the questions of what gesture is and what gestural boundaries are as open. We do not have preconceived notions of the direction or form of temporal gestures. We analyse gesture–speech relation in RT shows empirically to offer more complete evidence-based answers to these questions (see Uhrig et al., 2023). Therefore, we adopt the notion of a gestural unit or gestural movement rather than the notion of gesture. We view every gestural movement as potentially carrying more than one function (cf. Kok et al., 2016) and discard preconceived notions of gesture annotation such as phases.
For speech, prosodic, and gestural annotation, we use formal, directly observable categories, following Bateman’s call for the use of external languages of description to avoid the ‘danger of becoming ‘stuck’ within [our] pre-existing conceptualisations’ (Bateman, 2022a, p. 53).
There is a wealth of information in human communication that needs to be annotated to allow for a statistically valid analysis. Beyond the addition of huge amounts of (hu)manpower, the only feasible way to ensure that ‘work at scales larger than individual case studies is to be possible’ (Bateman, 2022a,b, p. 42) is to scale up annotation leveraging technology. Therefore, any annotation scheme must be designed to reflect the needs for analysis as informed by case studies and the affordances and constraints of current computer science and engineering methods.
In leveraging technology to scale up and speed up our research, we work to preserve the fine-grained nature of our analysis wherever possible, thus minimising the associated risk that the detail required will ‘restrict the objects of investigation that multimodality can address’ (Bateman, 2022a, p. 42).
Our computational study is driven by our conceptual thinking. Our conceptual thinking is affected by computational parameters. Both are affected by practical considerations. We determine an optimal interdisciplinary approach and implement it at every stage of our research, which makes our approach novel and our resulting annotated dataset different from other multimodal annotated datasets, in that:
i. the majority of datasets annotated for speech and gesture—with some also annotated for prosody (e.g., Kibrik, 2018)—rely on data collected in experimental (lab) conditions, e.g., SAGA (Lücking et al., 2010), CABB (Eijk et al., 2022), FreMIC (Rühlemann and Ptak, 2023), and Mittelberg (2018). These are not ‘naturally occurring’ data in the sense of Sinclair (1991, 171). In contrast, our annotated dataset is generated using media data, which are regarded by linguists as ecologically valid;
ii. those annotated datasets that have used media data either exercised a fully automatic approach to annotation for gesture generation, e.g., the TED Gesture Dataset (Yoon et al., 2019), or a different manual approach, e.g., Valenzuela et al., 2020, used the NewsScape corpus (Steen et al., 2018) to categorise temporal expressions co-occurring with gesture, but in contrast to our approach, they did not do a data-driven study, annotate their data in ELAN,1 or include prosody; and
iii. we have developed computational tools for the automatic annotation of media data and written those annotations into our ELAN files. Our task here was more complex compared to those research teams engaging with lab recordings because of our engagement with media data (e.g., the problem of changes in camera perspective; see Section 3).
The case study presents the results of manual analysis of an episode of RT’s show ‘SophieCo Visionaries’, to illustrate the level and nature of detail needed to address our research question and to inform decisions about what kinds of multimodal cues to annotate for in an automatic or semi-automatic annotation scheme. It shows how modalities—textual, acoustic, gestural, and corporal—may work together to construct subtly manipulative messages.
In the video clip A,2 the host, Sophie Shevardnadze, is in conversation via video conferencing with Tim Kendall, the ex-Facebook Monetisation Director, the ex-President of Pinterest, and the CEO of Moment (United States), about how people have lost control of their smartphones and have become addicted to using social media via them and to scrolling all the time, despite being aware of the associated harmful effects on their health.
Looking at her guest, Sophie produces three multimodal utterances engaging with a hypothetical future depiction. She constructs meaning, stance, and her viewpoint as part of the interaction with her guest to cast doubt upon the validity of her guest’s viewpoint. This forms part of a bigger manipulative strategy of discrediting anything that comes from the West and propagating the idea that the West is inferior to Russia in all respects. Making such ideas ‘infectious’ relies on more than just the multimodal signal produced and received; it relies on various contexts—e.g., situational, linguistic, cultural, and historical—in which the producer and the receiver find themselves. Our case study focuses on determining key cues from three modalities that trigger the construction of meaning, viewpoint, and stance and exploring ways in which the cues are coordinated in the video clip to prompt the audience to share Sophie’s viewpoint.
The guest is not visible in the clip under examination, but he appears on screen either by himself or simultaneously with Sophie elsewhere in the show. As is normal for TV broadcasting, Sophie’s audience is both her interlocutor (guest) and the TV audience (the implied viewer). The audience is prompted to construct a scene of blended joint attention, in which Sophie and them are attending jointly to the topic about smartphones and social media (Turner, 2014, p. 97–105).
As part of this scene (see Table 1 below for visual representation), Sophie says:
Wait so you are the CEO of Moment now—an app which according to the description of the website helps people build healthier relationships with their phones. So if I delete all social networks from my phone, how will my relationship with it become healthier exactly? I mean because, you know, I can really just check Twitter on desktop.
To analyse this example, we utilise conceptual integration/blending theory (Fauconnier and Turner, 2002), also making use of several tools and insights from mental spaces theory (Fauconnier, 1994). These are two related cognitive theories of meaning construction that are often drawn upon for the analysis of persuasive and manipulative discourse (see Pleshakova, 2018 for review). We also rely on the ‘mental spaces’ analysis of causal and conditional conjunctions by Dancygier and Sweetser (2000, 2005). Their studies demonstrate that conditionals like if and because can set up various mental spaces while fulfilling various communicative functions. If ‘can introduce patterns of reasoning at different levels (e.g., predictive, epistemic, or metalinguistic); it can build epistemically distanced or non-distanced or neutral spaces; and those spaces can then be referred to deictically’. Dancygier and Sweetser (2005, p. 58) differentiate between non-conditional, positive-stance future predictions, which ‘are about an expected future (unrealized) development of reality’, and conditional, negative-stance future predictions, which are about future ‘not yet realised and not certain to be realised’. They argue that:
[…] if […] expresses the speaker’s lack of full positive stance with respect to the content. The non-positive stance of if need not commit the speaker to a negative or sceptical stance, but does indicate that she thereby distances herself from full commitment to the contents of the if-clause. Other aspects of a conditional construction may go further, and explicitly mark the speaker’s leaning towards non-belief in the reality of the described situation (Dancygier and Sweetser, 2000, p. 125).
Space-building functions of because-clauses are different, as ‘causal conjunctions are semantically more appropriate to elaboration of spaces’ (Dancygier and Sweetser, 2005, p. 172, 181). The authors showcase the complexity of the mappings between information structure, clause order, and expressions of conditional and causal relationships (Dancygier and Sweetser, 2005, Ch. 7). The human mind is embodied, and we extend the framework of conceptual integration/blending to investigate not only how language and gesture work together in meaning and viewpoint construction (e.g., Parrill and Sweetser, 2004; Narayan, 2012; Parrill, 2012; Tobin, 2017; Turner et al., 2019; Valenzuela et al., 2020), but also how cues of speech (including prosody), gesticulation, and corporal behaviour trigger the construction of meaning, stance, and viewpoint in manipulative media communication.
The RT host Sophie engages with what she herself presents as the viewpoint of her guest: Wait so you are the CEO of Moment now—an app which according to the description of the website helps people build healthier relationships with their phones. She cites the description on the company’s website and states that her guest is the CEO of the company, thereby implying that the website ultimately conveys her guest’s viewpoint. Sophie’s verbal statement and her prosodic, gestural, and corporal behaviour (A1–A18) work to set up the viewpointed mental space (M), which incorporates:
i. Base: Sophie and her guest in interaction, the context of the RT show;
ii. Content: Sophie’s guest is the CEO of Moment—an app described on the company’s website as helping people build healthier relations with their phones;
iii. Focus: the description on the website as the guest’s viewpoint;
iv. Sophie’s Epistemic Stance of certainty towards the Content of the website’s description and her guest’s occupation and the link between them;
v. Sophie’s potentially negative Evaluative Stance towards the guest’s contribution made immediately before.3
Sophie’s prosodic behaviour on wait so and facial gestures—smiling and eyebrows moving up (a ‘peak’)4 (A1–A3)—signal her surprise at the content of her guest’s contribution immediately before. The accompanying prosody and facial gestures help to manage interaction at this turn-taking point. Both make wait so more prominent.
She produces each word—wait and so—as individual phrases. There is strong marking of the final boundary of each of those phrases, with strong glottalisation at the end of both (Figure 1). These two short phrases, which we have interpreted as intermediate phrases (ip), have their own nuclear pitch accents, and together they form a somewhat rhythmic pattern, perceptually. Sophie also starts to say something else, beginning with [w], which could be interpreted as the start of a wh-question before you are. She then reconfigures what she wants to say. The effect is a strong signalling of ‘hold on a second…’, and therefore manifests questioning and sceptical stance.
Figure 1. Waveform and spectrogram for wait…, so… with segmental and prosodic annotation (intonational phrases, pitch accents).
Sophie’s smile signals that she has spotted incongruity in her guest’s contribution and that she may doubt credibility behind his viewpoint.
Sophie’s right-hand gestures co-occurring with you are the CEO of Moment now—an app which according to the description of the website helps people build healthier relationships with their phones are performed in the central gestural zone.
On you are the CEO of Moment now—an app which according to the description of the website, Sophie engages the vertical, lateral, and sagittal axes (A4–A7) to conceptually map the description on the website to her guest. The fingers of her right hand go forward in a quick throwing move to represent the mapping. In addition to the representational function, this movement carries an interactive function in helping to maintain the dialogue between Sophie and her guest.
On helps people build healthier relationships, Sophie performs a complex sequence of right-hand gestural movements of various amplitudes and performed at a changing pace. This complex gestural configuration engages vertical, lateral, and sagittal axes to depict the non-straightforward process of the building of the healthier relationship (A8–A15). On with their phones, Sophie’s right hand goes down to pick up her phone and show it to her guest before putting it back on the desk (A16–A18). Following a quick smile at the beginning of the utterance, Sophie’s facial expression remains neutral throughout, her gaze is focused on the screen. Sophie closes her eyes at the end of her first utterance (A18). There is a simultaneous head nod against the background of her guest’s backchannelling yeah serving as further confirmation of the accuracy of Sophie’s representation of her guest’s viewpoint.
The setup of the mental space M1 relies on the mental space M as the input. The mapping between the viewpointed spaces M and M1 enables the construction of the conceptual and viewpoint blending network and the emergence of the new mental space M2, representing the viewpoint blend.
The network construction is triggered by Sophie’s next multimodal utterance—So if I delete all social networks from my phone, how will my relationship with it become healthier exactly?
Space M1 incorporates:
i. Base: Sophie and her guest in interaction, and the context of the RT show.
ii. Content: the hypothetical future scenario.
iii. Focus: the hypothetical future scenario—if-clause—presented by Sophie and the how-question about Sophie’s future relationship with her phone becoming healthier.
iv. Viewpoint and Epistemic Stance of uncertainty as pertinent to hypothetical future scenarios (the Speaker distances herself from full commitment to the content of the if-clause).
Before Sophie utters the if- and how-clauses, she says uhhh and her gaze goes left signalling her collecting her thoughts (Brône et al., 2017). That ‘leftwards—not in focus’ gaze behaviour co-occurring with uhhh triggers the process of setting up a new input mental space, M1. Sophie’s multimodal if- and how-clauses work to configure this new mental space, representing her own viewpoint on the content. M1 is mapped onto space M, which represents the guest’s viewpoint on the content. The mapping starts the blending process for the two viewpoints—Sophie’s and the guest’s—thereby supporting the interpretation of Sophie’s if- and how-clauses not as independent units but as part of unfolding discourse—a continuum. The blending process generates the viewpointed blend space of M2, in which M1 is interpreted in relation to M, and the viewpoint of M1 is conceptually presented as more authoritative. M1 as blended with M in M2 is also interpreted in relation to a number of other viewpointed mental spaces set up by the preceding discourse. For example, earlier in the discourse, the guest talks about people being digitally addicted. He talks about people going on their phones to check the weather and realising 45 min later that they have been scrolling through their Facebook news feed or Twitter.
The construction of the blend M2 is already triggered by Sophie’s uttering so in So if I delete all social networks from my phone. This works to map the content and viewpoint of space M1 to the content and viewpoint of space M. The outer-space mappings are selectively projected into M2 to become the blend’s inner-space conceptual relations. The question how will my relationship with it become healthier exactly? relies on the presupposition of the predicted result that deleting will lead to a healthier relationship with the host’s phone. The latter in turn relies on the input mental space M. This presupposition enables the construction in M1 of the causal relation in the content of the utterance between the deletion and the relationship becoming healthier. Simultaneously, it enables the construction in M1 of the causal relation between the hypothetical event of the deletion and the how-question as part of the speech interaction scenario.
Blend space M2 incorporates:
i. Base: Sophie and her guest in interaction; the context of the RT show.
ii. Content: Sophie’s guest is the CEO of Moment—an app described on the company’s website as helping people build healthier relations with their phones.
iii. Content and Focus: the hypothetical future scenario—if-clause (deletion, phone)—presented by Sophie and the how-question about the future (relationship becoming healthier, exactly) that she asks.
iv. Epistemic Stance of uncertainty (the Speaker distances themselves from the content).
v. Evaluative Stance of scepticism.
vi. Alternative ‘hypothetical’ Content and Focus: the predicted result of the relationship between the Speaker and the phone not becoming healthier following the deletion of social networks from Sophie’s phone.
vii. Sophie’s Viewpoint that deleting social networks from one’s phone will not make their relationship with their phone any healthier.
The if-utterance comprising if- and how-clauses is loaded, and by the time Sophie has uttered the if-utterance, it is clear that she does not believe that the deletion of the social networks from her phone will make her relationship with her phone any healthier. The whole if-utterance is therefore ultimately interpreted through the lenses of the evaluative and epistemic stances in M2, to where the predicted result of the relationship between the Speaker and the phone not becoming healthier is projected. This predicted result is dependent on the content of the social networks being deleted and constitutes the alternative to the presupposition that the relationship will become healthier.
Next, Sophie produces the utterance incorporating the because-clause: I mean because, you know, I can really just check Twitter on desktop.
This utterance triggers the setup of mental space M3 to offer Sophie’s reasoning in support of her stance and viewpoint already constructed in M2. Although her argument shifts the focus from her relationship with her phone to the use of social networks more generally, the way she presents this because-utterance multimodally creates the impression that she reasons about her relationship with her phone.
Space M3 incorporates:
i. Base: Sophie and her guest in interaction; the context of the RT show.
ii. The Contents of Sophie’s interaction with the guest—‘I mean’, ‘you know’—as well as of Sophie’s ability to check Twitter on her desktop.
iii. Focus on the reasoning—because-clause and making it ‘shared’ reasoning.
iv. Sophie’s Viewpoint—deleting social networks from one’s phone does not prevent them from checking social networks on one’s desktop.
v. Stance of epistemic certainty.
Mental space M3 is mapped into M and M1 and works to further reconfigure and elaborate the blend space M2. The reconfigured M2–M2(1) presents the how-question as expository and as argumentative strategy (see, e.g., Pascual, 2014; Xiang and Pascual, 2016). It features Sophie’s epistemic stance of certainty in support of her reasoning (because-clause in focus). Her evaluative stance is more openly sceptical. Sophie’s reasoning works to further construct her viewpoint that ‘deleting social networks from one’s phone will not make their relationship with their phone any healthier’. Her viewpoint is constructed as more authoritative and believable, despite the lack of logic in her argument (checking Twitter on her desktop might still make her relationship with her phone heathier).
The reconfigured M2(1) blend space incorporates:
i. Base: Sophie and her guest in interaction; the context of the RT show;
ii. The Contents of (a) the situation in which Sophie’s guest is the CEO of Moment—an app described on the company’s website as helping people build healthier relations with their phones; (b) the hypothetical future scenario—if-clause (deletion, phone)—presented by Sophie and the how-question about the future (relationship becoming healthier, exactly) that she asks; (c) Sophie’s interaction with the guest—I mean, you know—as well as of Sophie’s ability to check Twitter on her desktop, offered in the form of the because-clause.
iii. Focus on the hypothetical future scenario—if-clause—presented by Sophie and the how-question about the future that she asks.
iv. Focus on the reasoning—because-clause and making it ‘shared’ reasoning.
v. Epistemic Stance of uncertainty (the Speaker distances themselves from the content).
vi. Epistemic Stance of certainty in the ‘reasoning’ part—the because-clause.
vii. The Evaluative Stance of scepticism.
viii. Alternative ‘hypothetical’ Content and Focus: the predicted result of the relationship between the Speaker and the phone not becoming healthier following the deletion of social networks from Sophie’s phone.
ix. Sophie’s reasoning works to enhance her Viewpoint that ‘deleting social networks from one’s phone will not make people’s relationship with their phone any healthier’. It is constructed to be more authoritative and believable.
On so, Sophie makes a gesture with her right hand to activate the concept of the phone in M1. Her eyes are closed, which may signal the start of the next construction of meaning and viewpoint (A20). The if-clause which follows launches the configuration of M1 as a hypothetical future scenario in which Sophie deletes all social network applications from her phone and checks Twitter on her desktop.
The future deletion is conceptualised in gesture through the ‘slicing’ right-hand rightward and downward movement. The gestural conceptualisation is already there on if I (A21) before Sophie utters the verb delete. It continues on delete (A22).
The if-clause comprises seven prosodic words—So|if I|delete|all|social|networks|from my phone (Figures 2, 3). There are five pitch accents on if I, delete, all, networks, from my phone as well as two phrase accents on delete and phone. The if-clause’s boundaries co-occur with the boundaries of the intonational phrase (IP), which in turn incorporates two intermediate prosodic phrases—so if I delete and all social networks from my phone separated by a pause. The nuclear pitch accents within the respective intermediate phrases (ip) fall on delete and from my phone. The latter two are the only concepts which are also depicted in hand gesture. Sophie further foregrounds delete prosodically via a clear and audible release of the final [t].
Figure 2. Waveform and spectrogram for so if I delete with prosodic annotation (intonational phrases, pitch accents, and pauses).
Figure 3. Waveform and spectrogram for all social networks from my phone with prosodic annotation (intonational phrases, pitch accents, and pauses).
At the same time, she puts some prominence on phone in speech. It comes at the end of the first IP—and is accompanied by low fall with creaky voice signalling a complete conceptual unit in itself, though it is not the final thing Sophie has to say.
While speech and gestural representations for ‘delete’ co-occur, the hand depiction for ‘phone’ and the speech unit phone do not. The gestural phone co-occurs with so at the very beginning of the if-clause and the IP (A20). The speech representation for ‘phone’ is at the very end of the multimodal if-clause and the IP (A25). Thus, speech- and hand-gesture triggers for activation of the same concept phone are located at the boundaries of the multimodal if-clause. Between ‘phone’ in gesture and phone in speech, they bookend the whole IP. This multimodal configuration ensures that the concept of ‘phone’ is in focus throughout the clause.
Both prosody and gesture work in a complementary manner to support the configuration of the blend space M2, which, among other things, seems to include an internal hierarchical structure signalling which concepts are more in focus than others. By using the gestural ‘phone’ and phone in speech at the edges of the IP, Sophie is constructing the background story as being about the phone. She then has the freedom and flexibility to highlight something else within the sub-structure of the IP. This she does by using the longer-lasting gestural ‘delete’ over the intermediate phrase and having strong emphasis on the word delete. Such a distribution of speech–gesture representations for ‘phone’ and ‘delete’ signals the multimodal conceptualisations for the ‘phone’ as fulfilling the ground function and for ‘delete’ as fulfilling the figure against the ground function (On the gestalt psychological principle of figure and ground and the use of the relation in cognitive linguistics, see, e.g., Ungerer and Schmid, 2013, p. 163–191) We also note a parallel in prosodic analysis with the relationship between prosodic ‘domain’—i.e. the prosodic constituent within which a prosodic feature applies—and prosodic ‘prominence’—i.e. the focal element of the domain in question. Prosodic cues may signal both the boundaries of a domain/constituent (‘edges’) and the focal points within domains (‘heads’).
Due to restricted visibility, we cannot see whether there is any hand gesture performed on the rest of the if-clause (A23–26). However, we can see the ‘eyebrow up’ movement co-occurring with all social networks from my phone. This relatively long gestural movement—‘plateau’5—introduces the stance of wondering, further supporting (i) the conceptualisation in which the RT host distances herself from the depicted future scenario; (ii) the construction of epistemic stance of uncertainty and evaluative stance of scepticism; and (iii) the construction of Sophie’s viewpoint where her deletion of social networks from her phone does not lead to having a healthier relationship with it.
As the how-clause is uttered by Sophie, her right hand is formed as a brush with the fingers pointing down (A27–A34). It goes upwards, reaching its highest position on relationship with it become (just above the waist level, see A29–A31). On my relationship, the RH makes shaking movements. The hand then moves rightwards very slightly on with it while still making shaking movements. There is also a slight corporal movement and head to the right (A30). On healthier, the right hand goes slightly downwards (A32) and holds the position on exactly (A33 and A34). All gestural movements have very small amplitudes. The hand is relaxed, and the fingers are spread. The hand moves upwards and rightwards slightly to mark the future on will my relationship with it but remains in the central gestural zone.
There is a contrast between the hand gesture representations of the healthier relationship with one’s phone in the first multimodal utterance (A10–A17) and in the second utterance (A27–A34). Not only do gestural configurations differ in the amplitudes and levels of confidence, but their positioning is also much higher in the first utterance. The direction, including the orientation of fingers, in the first utterance is predominantly upwards–rightwards, whereas in the second utterance it is predominantly downwards–rightwards. Even when the right hand in the second utterance goes upwards, it does not go as high as in the first utterance, and the wrist leads on this ascending in the second utterance with fingers pointing down. This contrast between two gestural configurations co-occurring with the two speech utterances translates into a difference in epistemic and evaluative stance between the two as presented multimodally.
The epistemic and evaluative stances of the because-utterance are positive, and the gestural configuration works to communicate that (A36–A45). The overall characteristics of the gestural movements of the because-utterance resemble those of the first utterance in that they are of a bigger amplitude, more confident, and the palm orientation is up. The overall direction of the gestural sequence forming part of the because-utterance is upwards and rightwards, the same as we observe for the first utterance (cf. A5–A17).
On the prosodic side of the how-clause, there is phrase-initial strengthening on the [h] of how. This could signal the uncertainty embedded in the question. The nuclear accents in the how-clause fall on my relationship, with it, and exactly. In the hand gesture co-occurring with these speech units, we see marking of prominence, too—the right hand is in an elevated position and shaking on my relationship. It is at its highest position and shaking and goes slightly rightwards on with it. It is at its lowest position and holding on exactly. The three nuclear pitch accents constitute the cores of three intermediate phrases, which in turn form one intonational phrase (IP). The nuclear accents on the speech units my relationship and exactly create boundaries of the multimodal ground, which in gesture manifests itself through shaking throughout, consistent small amplitude of hand gesture, slight head tilt right and forward, shoulders slightly lifted. At the same time, this ground constitutes the figure of the IP of the how-clause as a whole. Sophie creates this multimodal ground/figure to signal the content in focus, which should be evaluated through the prism of the epistemic stance of uncertainty and of the evaluative stance of scepticism in M2. She further foregrounds with it as a figure by making a significant pause, thereby placing it in its own intermediate phrase (ip). Furthermore, she uses several phonetic devices to audibly strengthen the ip onset, namely the re-articulation and lengthening [w], as well as articulatory strengthening in the form of ‘stopping’ (the release of which is evident in the spectrogram). Sophie is effectively placing prosodic ‘scare quotes’ around with it, thereby distancing herself from the phone and placing it in some kind of isolated relief. She conveys a lack of trust, signalling that she does not really believe one can have a relationship with a phone, or at least not a natural, healthy one (Figure 4).
Figure 4. Waveform and spectrogram showing the prosodic boundaries around with it (in yellow) and re-articulation and strengthening of phrase onset [w] (in pink).
On with it become healthier exactly—the last two intermediate phrases of the how-clause—we observe another ‘plateau’ eyebrow gesture and a corporal movement forward (A30–A34). Perceived together, they simultaneously fulfil the functions of ground and of figure in their own right. These gestural and corporal movements, on the one hand, create the ground for figures with it and exactly, and on the other hand put the unit with it become healthier exactly in focus as a figure against the ground of the how-clause, working to further configure the hierarchical structure of the M2 blend space.
The eyebrow gestural movement conveys the stance of wondering, which is primarily applied to the content of with it, become healthier exactly. The simultaneous corporal movement forward adds to the prominence of this content, and signals Sophie’s intention to really convey this to her guest.
Sophie’s communicative goal is further evident in her phrasing of what follows, separating I mean, and ‘cause you know’ into intermediate phrases. By isolating first herself and then her interlocutor, she cultivates a knowing and equal ‘pact’ with her interlocutor (i.e., communicating ‘we both know this…’) (Figure 5).
Figure 5. Waveform and spectrogram illustrating the intermediate phrasing of I mean and ‘cause you know.
Simultaneously, we observe the dominance of a conduit gestural movement—right hand palm-up going forward—in the because-utterance (A35–A41). The movement serves the interactional and representational function of offering content to the interlocutor. It has a special configuration going rightwards in addition to going forward. This rightward movement conveys the temporal function of future depiction. This hand gesture is accompanied by the shoulder shrug and head tilt to the right, which also contribute to the construction of the epistemic and evaluative stance of ‘I am confident that I am right, and I am wondering what objections you can possibly have’. The small-scale move rightwards by the right hand on can is in line with its epistemic stance of less certainty (A38). The latter transforms into certainty immediately after, when Sophie’s right hand goes briefly to the centre on just (A40) and then goes much further rightwards and upwards on check Twitter on desktop. We observe a nuclear accent on desktop. At this point, Sophie’s right hand is already returning to the centre from its rightmost and highest position on Twitter (A42).
There is a quick, repeated eye-blinking and a quick head nod on desktop too (A43–A45). The more confident gestural movement—with a bigger amplitude—rightwards and upwards towards the end of the because-utterance signals Sophie’s belief in this possible future scenario as juxtaposed to the previous future scenario depicted multimodally in the if-utterance. Nuclear accents falling on the desktop in the because-utterance and on the phone in the if-utterance seem to also serve a special function here linking and juxtaposing phone and desktop at the same time.
Eye closing plays its own role throughout the three multimodal utterances under consideration (A1–A18; A20–A26; A34–A43). It further marks the boundaries of bigger units (usually IPs), which trigger the construction of meaning in the underlying conceptual blending network.
Core to the network is the emergence of the blend space M2 > M2(1), which features an epistemic and evaluative stance of scepticism and disbelief towards the matter of building a healthier relationship with one’s own phone.
Using all three modalities in concert, Sophie conveys scepticism about both the phone itself, the possibility of having a relationship with the phone, and also the ability to delete social media from it. She conveys her scepticism multimodally to the interlocutor and the TV audience. We observe a hierarchical interplay of cues—features and phrase boundaries—across the textual, visual, and acoustic modalities; conceptually, the cues play distinct but complementary roles.
One aspect of prosodic structure may be cued by many different acoustic-prosodic cues (and combinations thereof), and at the same time, any given acoustic-prosodic feature can cue different aspects of prosodic structure. This means that there is a non-simplistic association between structure and phonetic implementation in both directions. We observe the same complex relationship between gestural movements and the underlying gestural structure. We hypothesise that this complex relationship might also be found between gestural and prosodic modalities.
Not only does Sophie use the interplay of multimodal cues to structure communication through a configuration of mental spaces underlying it, she also segments communication to package information, foreground and background pieces of information, and construct her stance towards them. This process ultimately enables the construction of her own viewpoint, which is communicated as more authoritative and believable than her guest’s, manipulating the viewer to accept it despite some inherent failures of logic within it.
Our blending analysis demonstrates the importance of engaging with units of various lengths and forms belonging to all three modalities—textual, acoustic, and visual—and their interaction for the study of human communication, including manipulation.
To generalise and hence further develop our approach to manual analysis of our video data, we need to be able to analyse more than one example. To achieve that, we need to leverage technology, and to do that in a well-informed and optimal way, we need to do more case studies, with each contributing to our understanding of the conceptual, computational, and practical aspects of ongoing research. This summarises the iterative process we have gone through to make decisions on annotation and the creation of tools, to design our new ELAN annotation scheme with its meta-language, and to construct the expertly annotated dataset described in Section 3. Several case studies, like the one offered in this section, played an integral part in the development of our new methodology for multimodal analysis presented next.
In this section, we describe all the levels of annotation in our dataset, thereby presenting our annotation scheme as a whole. We discuss our motivations—conceptual, computational, and practical—for choosing specific annotation levels and values throughout. We describe the way in which we have interwoven manual and computational approaches to annotation. We present our methodological approach to exploratory analysis and simultaneous annotation of ecologically valid multimodal data, which allow to do both on a larger scale and relatively faster. At the initial stage of our study, we explored RT talk shows in English, namely: SophieCo Visionaries, hosted by a woman, Sophie Shevarnadze; and News with Rick Sanchez, hosted by a man, Rick Sanchez. We also examined the four-episode Russian-language documentary on post-Covid futures, Мир после [The World After], hosted by Tina Kandelaki. Having done some preliminary ‘speech–gesture’ analysis and annotation in ELAN, we opted for first studying SophieCo Visionaries in more depth. This show was of immediate interest to us because it was broadcast by RT in English and its thematic focus was exclusively on world futures. The show constituted data most suitable for answering our research questions, which are centred around the construction of future depictions multimodally for persuasive and manipulative purposes and targeting international audiences. At the outset of our study, we identified ‘will’ as one of the most frequent speech markers for future depictions. We created a corpus of 20-s video clips centred around ‘will’ using searches in CQPweb and subsequently analysed 84 clips using the Rapid Annotator6 to get a preliminary understanding of gestural behaviour of the Speaker co-occurring with future depictions in speech. We subsequently focused on 47 clips in which Sophie was the Speaker and moved to annotating in ELAN to allow for capturing rich multimodal data for more features and in a more precise manner. As we were designing our annotation scheme in ELAN, we had to make several decisions to allow for the annotation to be focused on the ‘future’ aspect of multimodal depictions, be optimal in terms of labour and time required, and be well balanced in terms of conceptual and computational motivations.
We identified discourse units of various lengths centred around speech markers that trigger the construction of viewpointed future depictions. Those included syntactic clauses, sentences, or even sequence of sentences. We then annotated for gestural sequences co-occurring with those discourse units. We prioritised annotating for sequences of gestural movements that were impressionistically perceived by coders as conceptualising time as a line and motion along the line (e.g., Núñez and Cooperrider, 2013 and Cooperrider et al., 2014). We regarded as open the question of direction and axis for future vs. past vs. present gesture, or, in other words, we refrained from assuming that in English, the future is conceptualised via forward and rightward hand gestures only, and the past is conceptualised via backward and leftward gestures only (cf. Valenzuela et al., 2020). To maintain our focus on the future aspect and to keep annotation manageable and machine-learning friendly, at stage 1, we did not include annotation for iconic gestures such as the ‘phone’ hand movement discussed in Section 2. This is because it lacks an obvious temporal function. However, we included iconic gestures such as ‘delete’ as it clearly carries the temporal function of the future in addition to the iconic function of deleting.
The length of intervals chosen for annotation at speech and gestural tiers was determined by our focus on the temporal aspect of meaning and viewpoint construction, as well as practical considerations. Although we had to limit the intervals we could annotate for manually at the first stage, now that we have developed computational approaches for automatic annotation based on that, we are expanding our multimodal annotation—for the tiers described in this section—to include the whole length of shows (23 26-min shows).
Our annotation scheme is the result of multiple iterations, careful considerations, and discussions between the members of our multidisciplinary research team (for more details of our work at earlier stages, see Uhrig et al., 2023).
The textual modality as presented here is an artefact that we include for convenience, fully aware that it is in fact part of the speech signal, which we record on the acoustic channel. From the acoustic channel, Automatic Speech Recognition (ASR) attempts to recognise words for the full files. For the smaller, manually annotated sections, a manual transcription was created by the annotators themselves. Note that any segmentations, e.g., the introduction of punctuation marks in the transcripts, are already interpretations. These can be done by a machine in the case of the automatic transcription, where we used automatic punctuation restoration in the preparation of the files for CQPweb (see Uhrig et al., 2023 and Dykes et al., 2023 for details), i.e., the punctuation marks are purely based on the derived textual modality. For the manual transcription, any punctuation marks would also be inspired by prosodic features such as pauses and intonation.
YouTube provided automatic transcriptions for the videos in our dataset (see Dykes et al., 2023), which are roughly time-aligned on the word level. We import these into ELAN automatically. The manually annotated sections contain a manual transcript, which is, however, not time-aligned on the word level.
There are limitations to this approach in that the word recognition is not always accurate (and the show host’s foreign accent slightly reduces the accuracy), so we manually correct the annotations as we proceed with our annotation for individual intervals, although not systematically for entire files.
Furthermore, we have tried a more recent development, Whisper,7 which on average offers better speech recognition but at the cost of over-standardising (e.g., it removes false starts and hesitation phenomena). Whisper only provides timestamps on the level of an entire subtitle line and not per word, at least not out of the box. For now, we have not pursued this avenue of automatic transcription any further.
Once we had determined video intervals for viewpointed future depictions, we analysed them for further markers of the future in speech. The analysis allowed us to identify seven classes of future markers in English speech:
1. will-future
2. Conditional clauses and counterfactuals (e.g., if-, when-clauses)
3. Modal verbs (e.g., should, must)
4. Time adverbials (e.g., in the future, next year)
5. going to-future and present-tense simple and progressive used with future reference
6. Words with a semantic component of future (e.g., possibility, futurist)
7. Words that acquired future semantics within the specific context (e.g., architect is defined by the speaker both as an engineer and a futurist, and thus acquires the ‘futurist’ semantics for the subsequent discourse)
We then proceeded to include in our annotation scheme the tiers for (i) automatic transcription, (ii) viewpointed future depiction; (iii) future marker in speech; and (iv) future marker class.
As illustrated in Section 2, integration of all three modalities is important for the analysis of persuasive and manipulative communication. Thus, it was necessary to identify the boundaries of the principal constituents of the prosodic hierarchy and prominences within these, which can be thought of as prosodic landmarks. By annotating these, we can then proceed to identify whether and how gestural and corporal landmarks align with them.
Prosodic annotation was done by one or two expert coders manually in Praat8 and then verified by one to two senior experts before being transferred to ELAN. As showcased in Section 2, the relationship between prosodic structure (e.g., edges of prosodic constituents such as prosodic phrases, prominences within a prosodic constituent) and the acoustic cues to prosody (e.g., pauses, variation in f0, duration, and voice quality) is a complex one, with a many-to-one and one-to-many mapping between acoustic cues and prosodic structure. This means that selecting just one acoustic parameter would give not just a partial picture but also one that is also inconsistent in what it depicts. We start with the manual analysis and annotation for prosody—all relevant cues—with the aim of exploring phonetic complexities and laying the groundwork for our future study on the automation of annotation for prosody.
The manual annotation scheme provided below is sufficient to identify two levels of prosodic phrasing (IP and intermediate phrases), prosodic word boundaries, pauses between and within phrases, and two degrees of accentual prominences (phrase accents and the nuclear phrase accent). The annotation was done following the IViE conventions (Grabe et al., 1998). The full process of the manual annotation is described by us in Uhrig et al. (2023: Section 2.7).
Phrase
IP (Intonational Phrase).
ip (intermediate phrase).
ProsWord (Prosodic Word).
Accent
On this tier, all tonal events are labelled:
1. pitch accents, which are associated with specific syllables (with specific words), and lend perceptual prominence (the principal one of which in any prosodic phrase is known as the nuclear pitch accent, and marks the prosodic ‘head’ of that constituent, and the focus of that phrase);
2. phrase accents that appear between the last pitch accent and the boundary tone of a phrase;
3. boundary tones, which are associated not with words but with the phrase, and appear at the phrase edge, carrying information about the type of phrase (e.g., question vs. statement).
Glossary: L*, H*, H* + L, L* + H, H-, L-, H%, L%.
Nuclear stressed syllable
The nuclear stressed syllable was marked. This aligns with the final pitch accent (i.e., the nuclear pitch accent) on the accent tier.
Glossary: N (Nuclear stress).
Comments
On this tier, we noted the following particular prosodic features: mispronunciations, interruptions, speech rate discontinuities, strong focal emphasis, or voice quality effects (Uhrig et al., 2023, Section 2.7).
See the video capturing the annotation for textual and acoustic modalities here.9
As far as visual modality is concerned, the case studies on multimodal future depictions by RT that we have done so far have motivated us to annotate gestural movements by hand, face, and head, as well as corporal movements on individual tiers. There is a hierarchical ‘annotation’ arrangement here since the core focus of our current study is on gestural movements by hand and eyebrows. As explained in sub-section 3.3.2.3, we did not annotate for eye behaviour, gaze movement, head movement, and corporal movement to a full extent since our engagement with those features came secondary out of our primary engagement with the hand and eyebrows.
The complex analysis for meaning, stance, and viewpoint construction that we perform as showcased in Section 2 calls for a fine-grained annotation at a high level of precision.
We therefore started with manual annotation by expert coders for direction and orientation of hand movements. We subsequently worked to automate annotations for hand direction and orientation, guided by both conceptual considerations and constraints posed by the development of the computational tools. We then applied our experience and observations to create a tool for automatic annotation of the direction of hand movements.
We approached our annotation for gestural zones for hands differently, first developing an automatic tool for gestural zone identification and then verifying annotations manually with the help of non-expert coders.
The annotation of gestural zones was more straightforward than the annotation of hand gesture. Our study on algorithms for automatic hand movement detection described in Section 3.3.1.4 allowed for the identification of gestural zones without the need for extensive preliminary manual annotation.
As demonstrated in Section 2, gestural sequences or individual gestural movements that co-occur with future depictions in speech are complex. To be able to capture the complexity of those on the formal level, we opted for annotating for direction on three axes—sagittal, lateral, and vertical. Having a separate tier for gestural trajectory presented a problem due to the lack of a consistent approach for labelling, which tends to use metaphorical labels and, in doing so, already deviates from the purely formal recording of gestural characteristics. If the gestural sequence or a gestural movement had a complex trajectory, e.g., the ‘delete’ gestural sequence in our analysis in Section 2, when the hand goes slightly leftwards but also upwards and then rightwards but also downwards and then just downwards, we captured the complexity by annotating for the same ‘gesture’ on three tiers—sagittal, lateral, and vertical—for the same interval in speech. Gestural movements recorded as performed along different axes may start and/or end either simultaneously or at different times. Thus, the timings of the sub-intervals created on separate tiers for the same gestural sequence may overlap but do not have to coincide.
That resulted in a situation where we did not have a separate tier for gestural trajectory but still captured the trajectory implicitly across a number of tiers for hand movements. Given that we often encountered gestural movements where the hand, the fingers, and the thumb may be moving, pointing in different directions, or even moving along different axes, we opted for annotating for hands and fingers on separate tiers. For the segmentation of longer gestural sequences into individual units, we relied on two criteria: either a change of direction or a change of axis will delineate individual gestures as we understand them.
This type of annotation took into account the constraints of the potential computer vision tools, for which a small set of categories, e.g., the axes and directions, are easier to distinguish than a complex set of labels.
We annotated for hand and finger movements in a certain direction on six tiers—two for sagittal axis, two for lateral axis, and two for vertical axis—in ELAN with handedness captured through labelling the tiers for axes, e.g., right hand going rightwards would be labelled on the tier for lateral axis as ‘RH R’. We had a separate tier for capturing a handshape.
As our approach to analysis and annotation is data-driven, we did not limit ourselves to thinking that future can be conceptualised in gesture for English through forward or rightward movements only. Rather, we used conceptual blending to analyse meaning and viewpoint construction and, through such analysis, to determine whether a certain gestural movement may carry a representational function of future (Section 2). We have analysed examples where we observed various outward-directed hand movements and body-directed hand movements arguably carrying a future function. Therefore, body-directed hand movements were captured as BDG labels on tiers for axes.
The annotation scheme described below was developed to be universally applicable. Although it may not be exhaustive, it has allowed us to capture key parameters of gestural movements with the impressionistically perceived temporal function and to do so through the formal approach to gesture recording.
Sagittal axis hand
Glossary: RH (Right Hand), LH (Left Hand), BH (Both Hands) + F (Forwards), B (Backwards), BDG B (Body-Directed Gesture Backwards).
Lateral axis hand
Glossary: RH (Right Hand), LH (Left Hand), BH (Both Hands) + R (Rightwards), L (Leftwards), S (Spread), C (Centre).
Vertical axis hand
Glossary: RH (Right Hand), LH (Left Hand), BH (Both Hands) + U (Upwards), D (Downwards).
Sagittal axis fingers
Glossary: RH (Right Hand), LH (Left Hand), BH (Both Hands) + Fingers (all fingers), Thumb, IF (Index Finger), MF (Middle Finger), RF (Ring Finger), LF (Little Finger) + F (Forwards), B (Backwards), and BDG B (Body-Directed Gesture Backwards).
Lateral axis fingers
Glossary: RH (Right Hand), LH (Left Hand), BH (Both Hands) + Fingers (all fingers), Thumb, IF (Index Finger), MF (Middle Finger), RF (Ring Finger), LF (Little Finger) + R (Rightwards), L (Leftwards).
Vertical axis fingers
Glossary: RH (Right Hand), LH (Left Hand), BH (Both Hands) + Fingers (all fingers), Thumb, IF (Index Finger), MF (Middle Finger), RF (Ring Finger), LF (Little Finger) + U (Upwards), D (Downwards).
Handshape
The handshape and palm orientation, where applicable, for each moving hand are recorded on a separate tier. The annotation also reflects the changes of handshape during the direction intervals (sagittal/lateral/vertical axis hand).
Glossary: RH (Right Hand), LH (Left Hand), BH (Both Hands) + OP (Open Palm), CP (Closed Palm), Fist, FB (Finger Bunch), FP (Finger Pinch), Prayer, Cup, Hand brush + A (Away—for OP), B (Back—for OP), U (Up—for OP, Cup), D (Down—for OP, Cup), V (Vertical—for OP).
See the video capturing the manual annotation for hand gesture here.10
The detection of hand gesture is usually done in computer vision by detecting hand movement. Accordingly, most computer vision systems do not distinguish gestures from other types of hand movements, which is in line with our data-driven approach, which rejects the practise of discarding data a priori.
Most of our automatic annotation of hand movements is based on body pose estimation, for which we use OpenPose (Cao et al., 2019). With this system, every single frame of the video is annotated with the body keypoints of every person identified.
Figure 6 shows the keypoints for an example from the video analysed in Section 2. Since we rely on media data, the videos do not contain depth information, which means that we only obtain keypoints in 2D space, the x and y values of which correspond to the vertical and lateral axes as long as the speaker is facing the camera. Since keypoints are detected separately for each frame, we often witness so-called jitter, i.e., small changes of keypoint coordinates between frames without any discernible movement. We use statistical methods to smooth these keypoint positions to eliminate those artefacts introduced by the software, which would otherwise lead to false gesture detections. Furthermore, keypoints may not be detected in some frames, often owing to motion blur. In these cases, we interpolate the coordinates linearly between the last detected and the next detected keypoint, i.e., we draw a straight line between them. If missed detections happen at the beginning or at the end of the scene, we extrapolate the first known or the last known value, respectively, to the beginning and end.
Another problem that the analysis of media broadcasts faces is the frequent changes in camera perspectives, either to give a different perspective of the same person or to switch to showing a different person. Often, both the host and the guest appear next to each other in a split screen. As described in Uhrig et al. (2023: Section 2.3), we automatically cut a video into scenes, deploying active speaker detection and biometric clustering, to obtain annotations for the host of the show only when she is visible and speaking. To account for the differences in speaker size on the screen across scenes, we normalised the speaker’s size by expressing all hand positions in relation to the average position of the speaker’s nose in the scene and normalised to the distance between the average position of the nose and the average position of the neck keypoint. We call this distance our normalisation unit.
In a first step of automation, we added time series of the wrist keypoints of both hands for the vertical and lateral axes to ELAN (see short description in Uhrig et al., 2023, Section 2.3). The videos11 (also taken from Uhrig et al., 2023) show the time series for wrists in the second and third time series panels at the top of ELAN’s annotation window. Despite the normalisation procedure outlined above, we can still observe shifts in the time series plot when there is a scene change.
During the manual annotation phases, we established that the wrist keypoints were generally reliable when detected. In order to further speed up and support the manual annotation process, we added a rule-based direction detection on the vertical and lateral axes. Our system detects any movement of the smoothed wrist keypoint (separately for the left and right wrist) that goes in the same direction (i.e., leftwards or rightwards for the lateral axis and upwards or downwards for the vertical axis) for at least six frames (i.e., 0.24 s).
The system is highly sensitive to even very small movements that are hardly visible to the naked eye and may well be just artefacts of the computer vision system’s calculations. We introduced a threshold below which we do not detect, corresponding to roughly 1 mm difference per frame, in order to reduce the number of these wrongly detected ‘gestures’. The exact value of this threshold is currently being evaluated in close conjunction with the manual annotation experts. Therefore, both the unfiltered and the filtered versions of the tier exist in parallel in our ELAN files.
While the system is reliable for most of the data, there are limitations with respect to certain camera perspectives. For instance, at the end of the video snippet above, the speaker is filmed diagonally from behind, sitting in front of a large screen. Here, the direction information is lacking, also because often the right hand is occluded by the body of the speaker. Another problematic case is illustrated by the video snippet analysed in Section 2 above, where in the close-up shots, only the hands are visible from time to time but never the elbow of the speaker. In such cases, OpenPose cannot detect the wrist as part of the speaker’s body because the connection via the elbow keypoint is missing. If this happens for the entire scene, even the interpolation method outlined above cannot help because there are not enough data points available. We are currently evaluating the use of other pose estimation systems that detect hands separately, even if the elbow is not detected.
As demonstrated in Section 2, the Speaker’s hand position in relation to her body is important in the analysis of time conceptualisation in gesture—e.g. if a hand movement with a future function is made within the central gestural zone, that may signal that the Speaker does not believe that the future event depicted will materialise. Because our data are 2D, we have so far automatically annotated for the vertical and lateral axes only. From a conceptual perspective, we have adapted to the needs of our analysis of McNeill’s gesture space diagram (1992: 89). In our adapted diagram (see Figure 7), we distinguish 17 zones. These zones are a combination of boundaries along the vertical and lateral axes.
To automatically identify those 17 gestural zones, we follow the approach described in Section 3.3.1.3, i.e., we make use of normalised, smoothed, and interpolated keypoint coordinates with reference points and normalisation units. We start out by working with both axes separately and identifying five different zones for each. Different reference points and normalisation units are defined for each axis. For the vertical axis, the reference point is a nose y-coordinate, and the normalisation unit (NU) is the distance between nose and neck, as mentioned in Section 3.3.1.3. We match the vertical position of the wrists to the zones defined in Figure 7, e.g., if the wrist’s y-coordinate is below the reference point by more than three times the length of our normalisation unit, we assign the “Down” label to it. The full list of criteria is given in Table 2.
For the lateral axis, the reference point is the neck x-coordinate. We use different normalisation units for the right and left wrists. The normalisation unit for the right wrist (RNU) is the horizontal distance between the neck x-coordinate and the x-coordinate of the right shoulder, and the normalisation unit for the left wrist (LNU) is the horizontal distance between the neck x-coordinate and the x-coordinate of the left shoulder, respectively. Although we generally observe similar values for RNU and LNU, having two independent reference units minimises the effect of jitter discussed in Section 3.3.1.3 and thus leads to more consistent results.
For the horizontal position of the wrists defined in Figure 7, we use the criteria given in Table 3.
As a result, for each frame, we obtain a pair of labels for a wrist position (vertical position label and horizontal position label). We then merge and rename these to produce final labels in accordance with the predefined gestural zones in Figure 7.
Time series panels:
• Right Wrist Lateral Position, Left Wrist Lateral Position
• Right Wrist Vertical Position, Left Wrist Vertical Position
Tiers:
• Right Wrist Lateral Direction Auto
Glossary: Right, Left
• Right Wrist Lateral Direction Auto (Threshold)
Glossary: Right, Left
• Right Wrist Vertical Direction Auto
Glossary: Up, Down
• Right Wrist Vertical Direction Auto (Threshold)
Glossary: Up, Down
• Left Wrist Lateral Direction Auto
Glossary: Right, Left
• Left Wrist Lateral Direction Auto (Threshold)
Glossary: Right, Left
• Left Wrist Vertical Direction Auto
Glossary: Up, Down
• Left Wrist Vertical Direction Auto (Threshold)
Glossary: Up, Down
• Right Wrist Zone Auto
Glossary: Right Up, Up, Left Up, Centre Right Up, Centre Up, Centre Left Up, Right, Centre Right, Centre, Centre Left, Left, Right Down, Centre Right Down, Centre Down, Down, Centre Left Down, Left Down
• Left Wrist Zone Auto
Glossary: Right Up, Up, Left Up, Centre Right Up, Centre Up, Centre Left Up, Right, Centre Right, Centre, Centre Left, Left, Right Down, Centre Right Down, Centre Down, Down, Centre Left Down, Left Down.
See the video capturing the automatic annotation for hand movement and gestural zones here.12
We manually annotated several video clips for facial gesticulation at the initial exploratory stage. Since facial gesticulation cannot be directly linked to temporal representation, we opted to annotate for facial gesticulation throughout videos and did not restrict it to specific temporal speech-led intervals. This approach proved to be too time-consuming and labour-intensive and could not be sustained. At the same time, the exploratory annotation for facial gesticulation informed by our studies allowed us to make a better-informed choice as to what facial gestural feature to annotate first for the purposes of our study on future depictions. We chose to annotate for eyebrow movement along the vertical axis. As showcased in Section 2, eyebrow movements are coordinated with other types of gestural movements (e.g., hand) and with prosodic organisation in meaning and stance construction. For example, eyebrow movements, like prosody, mark prominences and phrase boundaries, contributing to the construction of sceptical stance.
Furthermore, it proved possible to develop an automatic annotation tool for eyebrow movements quickly. This enabled automatic processing of our data for eyebrow movement visualisation first. We then proceeded to analyse automatic eyebrow visualisation time series in an exploratory fashion. We developed an approach for subsequent manual verification of automatic annotation for eyebrows and the addition of further manual annotation. Not only did such an approach allow us to considerably speed up annotation, but it also enabled us to gather further insights into coordination between eyebrow movements, eye behaviour, and head gesticulation that we would not have spotted otherwise. The computer vision algorithm highlighted for us small movements, which we would have ignored during the fully manual annotation due to the richness of the data and the limitations of human attention.
The automatic eyebrow visualisation time series indicates the vertical position of each eyebrow. We cannot, however, equate this to the eyebrow’s vertical position in the video frame because head movements (and particularly head tilts) adversely affect the calculation of the position in relation to a facial landmark. In our software, we use OpenPose’s face keypoints with the same kind of smoothing and interpolation as described above for the hand gestures. The normalisation unit is the distance between the top and the tip of the nose. We calculate the mean position of the eyebrow keypoints and compare this to the mean position of the lower eyelid keypoints. We inherit certain issues from the limitations of OpenPose. Thus, during blinks or longer periods of closed eyes, the keypoints of the lower lid are detected further down, which leads to a relatively higher position of the eyebrows. At first, we regarded this as a flaw in the automatic visualisation, but upon further inspection, we decided that even these blinks and closed eyes may be meaningful units for our analysis of facial gesticulation and its role in the overall meaning, viewpoint, and stance construction, as illustrated in Section 2. We do not know how and in relation to precisely which facial movements humans perceive raised eyebrows, so these cases might function perceptually in a manner similar to raised eyebrows. As explained in further detail in the next sub-section, we opted to do manual annotation for eye, head, and corporal behaviour only as prompted by eyebrow movement, or what the machine, in contrast to human coders, saw as eyebrow movement. Our annotation for facial and head gesticulation or eye or corporal behaviour is by no means exhaustive. It serves the purpose of our ongoing analysis of multimodal depictions of futures as showcased in Section 2 and is, at this stage, exploratory.
Time series panel:
• Right Eyebrow Vertical Position, Left Eyebrow Vertical Position
The automatic tracking of eyebrow movement was reliable in most cases but still had some limitations due to such factors as scene change, head movement, and poor video quality.
Two coders went through all eyebrows time series to establish whether the OpenPose-based movement detection was correct. They created corresponding intervals to note the direction of the eyebrow movement and establish the boundaries of the eyebrow units. Disagreements regarding the boundaries were resolved through discussion.
Coders were observed and annotated for two kinds of errors in automatic annotation. The first was when the machine produced an error that could not be explained by what human coders saw in the video, e.g., the Speaker’s gesticulation, corporal behaviour, or hair masking the eyebrows. The second kind of error could be explained by factors such as scene change, head movement, and poor video quality that the human coders encountered.
Eyebrow movements
Glossary: BU (Both eyebrows Up), BD (Both eyebrows Down), LU (Left eyebrow Up), LD (Left eyebrow Down), RU (Right eyebrow Up), RD (Right eyebrow Down).
Peak or plateau
We differentiated between two types of eyebrow movement: Peak and Plateau. These are working terms emerging from our exploratory analysis that are not grounded in any theoretical framework offered elsewhere. As we proceed with our analysis, we may opt to change the terms and/or offer a new theoretical framework emerging from our observations and analysis.
In our engagement with Peak and Plateau as working terms and concepts, we relied on the length of the domain, which coincides with eyebrow movement, as the criterion. We defined Peak as a short accent-like eyebrow movement (its domain can be a word, prosodic word, or a syllable) and Plateau as a prolonged movement where eyebrows would stay in the same position for a longer time (its domain can be an ip, IP, grammatical clause, or a sentence/phrase).
Glossary: Pk (Peak), Pl (Plateau).
Head movement
Glossary: TL (Tilt Left), TR (Tilt Right), TD (Tilt Down), TU (Tilt Up), TF (Tilt Forward), TB (Tile Backward), Tr L (Turn Left), Tr R (Turn Right), Nod.
When coders impressionistically perceived a head tilt forward as a nod, they recorded it as such, but if in any doubt whatsoever, they annotated it as ‘head tilt forward’. We included the term tilt deliberately to avoid using a more loaded—linked to a function—label and annotated smooth (impressionistically) head movements as tilts in an attempt to capture only the formal side of head gestural movements.
Corporal movement
Glossary: F (Forward), B (Backward), U (Upward), D (Downward), Shrug (Shoulder shrug).
Eyes
Glossary: O (Open wide), B (Blinking), S (Squinting), Cl (Closed eyes), W (Winking).
Gaze
Glossary: U (Up), D (Down), L (Left), R (Right), UL (Up Left), UR (Up Right), DL (Down Left), DR (Down Right).
Gaze focus
Glossary: F (Focused), D (Distanced).
See the video capturing the automatic annotation for eyebrow movement and manual annotation for gesticulation and corporal behaviour here.13
We have presented our annotated multimodal dataset and the methodology underpinning its creation. Our case study showcased the necessity of including in our annotation scheme various tiers and features from three modalities: textual, acoustic, and visual.
The implementation of our approach has relied on ongoing dialogue between our team’s linguists (experts in cognitive linguistics, discourse analysis, phonetics and prosody, gesture study, computational analysis, and area studies), engineers, and computer scientists (cf. Bateman, 2022a, p. 59). Through this interdisciplinary work, we have been able to produce methodologically sound analyses and computationally tractable annotations. We have extended the framework of conceptual integrating/blending as a cognitive theory to explore how cues of speech (including prosody), gesticulation, and corporal behaviour work together to construct meaning, stance, and viewpoint in RT communication and translate our insights into decisions on annotation strategies. Our automatic annotations used theoretically informed categories, and our manual annotations were adjusted for optimal use in machine learning.
Our study continues, and, among other things, we are producing automatic annotation for amplitude and velocity of gestural movements, which our case studies have shown to be important to include in our dataset.
We envisage using our annotated dataset not just for the purposes of generalising our ongoing multimodal analysis of RT’s depictions of the future but also for fine-tuning a multimodal model pre-trained on big data from RT using unsupervised machine learning. To this end, we have already begun to leverage more advanced AI methods to the benefit of all disciplines involved in our multimodal research.
On the conceptual side, our ability to identify the relevant variables within each modality at scale and speed and to see patterns now opens a pathway for building a new theoretical model for speech–gesture interaction.
RT show ‘SophieCo Visionaries’, episode ‘We’ve lost control of our phones’, downloaded from YouTube, last accessed on 3 February 2022 (http://go.redhenlab.org/pgu/0138).
The original contributions presented in the study are included in the article/supplementary material; further inquiries can be directed to the corresponding author.
Ethical approval was not required for the study involving human data in accordance with the local legislation and institutional requirements. Written informed consent was not required for participation in study or for the publication of identifying images or data in accordance with the local legislation and institutional requirements.
AW: Conceptualization, Data curation, Formal analysis, Funding acquisition, Investigation, Methodology, Project administration, Resources, Supervision, Validation, Visualisation, Writing – original draft, Writing – review & editing. IP: Writing – original draft, Writing – review & editing, Data curation, Investigation, Validation, Visualization. EP: Conceptualization, Formal analysis, Methodology, Supervision, Writing – review & editing. IB: Methodology, Software, Writing – original draft. PU: Data curation, Funding acquisition, Methodology, Project administration, Resources, Supervision, Validation, Visualization, Writing – original draft, Writing – review & editing.
The author(s) declare financial support was received for the research, authorship, and/or publication of this article. The research presented in this article was made possible by generous funding provided by the Arts and Humanities Research Council (grant reference AH/W010720/1), the Deutsche Forschungsgemeinschaft (project number 468466485), and The John Fell Oxford University Press Research Fund (grant reference number 0011177). The authors gratefully acknowledge the scientific support and HPC resources provided by the Erlangen National High Performance Computing Centre (NHR@FAU) of the Friedrich-Alexander-Universität Erlangen-Nürnberg (FAU) under the NHR project b105dc. NHR funding is provided by federal and Bavarian state authorities. NHR@FAU hardware is partially funded by the German Research Foundation (DFG)—440719683.
The authors would like to thank: Georgy Krasovitsky for help with gesture annotation; Anna Sapuntsova for help with annotation for prosody; Evie Burrows for annotation for prosody and eyebrow movements; Mary Baltazani for expert engagement with prosody annotation; Scott Hale for help with collecting and storing data; Nathan Dykes for corpus building and NLP; Philip Torr and N. Siddharth for advising on computer vision-related work; and Andrew Wilson for proofreading the manuscript.
The authors declare that the research was conducted in the absence of any commercial or financial relationships that could be construed as a potential conflict of interest.
All claims expressed in this article are solely those of the authors and do not necessarily represent those of their affiliated organizations, or those of the publisher, the editors and the reviewers. Any product that may be evaluated in this article, or claim that may be made by its manufacturer, is not guaranteed or endorsed by the publisher.
1. ^https://archive.mpi.nl/tla/elan
2. ^See http://go.redhenlab.org/pgu/0132/ or scan the QR code.
3. ^See http://go.redhenlab.org/pgu/0137 or scan the QR code.
4. ^See Section 3.3.2.3 for explanation.
5. ^See Section 3.3.2.3 for further explanation.
6. ^https://beta.rapidannotator.org
7. ^https://openai.com/research/whisper
8. ^https://www.fon.hum.uva.nl/praat/
9. ^See http://go.redhenlab.org/pgu/0133/ or scan the QR code.
10. ^See http://go.redhenlab.org/pgu/0134/ or scan the QR code.
11. ^http://go.redhenlab.org/pgu/0130 and http://go.redhenlab.org/pgu/0131
12. ^See http://go.redhenlab.org/pgu/0135 or scan the QR code.
13. ^See http://go.redhenlab.org/pgu/0136 or scan the QR code.
Andries, F., Meissl, K., de Vries, C., Feyaerts, K., Oben, B., Sambre, P., et al. (2023). Multimodal stance-taking in interaction—A systematic literature review. Front. Commun. 8:1187977. doi: 10.3389/fcomm.2023.1187977
Bateman, J. A. (2022a). Multimodality, where next? – some meta-methodological considerations. Multimod. Soc. 2, 41–63. doi: 10.1177/26349795211073043
Bateman, J. A. (2022b). Growing theory for practice: empirical multimodality beyond the case study. Multimod. Commun. 11, 63–74. doi: 10.1515/mc-2021-0006
Bavelas, J. B., Chovil, N., and Lawrie, D. A. (1992). Interactive gestures. Discourse Processes. 15, 469–489. doi: 10.1080/01638539209544823
Brône, G., Oben, B., Jehoul, A., Vranjes, J., and Feyaerts, K. (2017). Eye gaze and viewpoint in multimodal interaction management. Cogn. Linguist. 28, 449–483. doi: 10.1515/cog-2016-0119
Cao, Z., Gines, H., Tomas, S., Shih-En, W., and Yaser, S. (2019). OpenPose: Realtime multi-person 2D pose estimation using part affinity fields. IEEE Trans. Pattern Anal. Mach. Intell. 43, 172–186. doi: 10.48550/arXiv.1611.08050
Chu, M., Meyer, A., Foulkes, L., and Kita, S. (2014). Individual differences in frequency and saliency of speech-accompanying gestures: the role of cognitive abilities and empathy. J. Exp. Psychol. Gen. 143, 694–709. doi: 10.1037/a0036311
Cooperrider, K., Núñez, R., and Sweetser, E. (2014). “The conceptualization of time in gesture. Body-language-communication” in Body–Language–Communication: An International Handbook on Multimodality in Human Interaction. eds. C. Müller, A. Cienki, E. Fricke, S. Ladewig, A. McNeill, and J. Bressem, vol. 2 (Berlin, München, Boston: De Gruyter Mouton), 1781–1788.
Dancygier, B., Hinnell, J., and Lou, A. (2019). “Stance construction in multimodal, multi-media contexts” in Paper presented at the 15th International Cognitive Linguistics Conference. Nishinomya, Japan
Dancygier, B., and Sweetser, E. (2000). Constructions with if, since, and because: causality, epistemic stance, and clause order. Top. English Linguist. 33, 111–142
Dancygier, B., and Sweetser, E. (2005). Mental Spaces in Grammar: Conditional Constructions. New York: Cambridge University Press.
Dykes, N., Wilson, A., and Uhrig, P. (2023). “A pipeline for the creation of multimodal corpora from YouTube videos” in Proceedings of Linguistic Insights from and for Multimodal Language Processing (LIMO 2023) at KONVENS, Ingolstadt.
Eijk, L., Rasenberg, M., Arnese, F., Blokpoel, M., Dingemanse, M., Doeller, C. F., et al. (2022). The CABB dataset: A multimodal corpus of communicative interactions for behavioural and neural analyses. NeuroImage 264:119734. doi: 10.1016/j.neuroimage.2022.119734
Fauconnier, G. (1994). Mental Spaces: Aspects of Meaning Construction in Natural Language. Cambridge: Cambridge University Press.
Fauconnier, G., and Turner, M. (2002). The Way We Think: Conceptual Blending and the Mind’s Hidden Complexities. New York: Basic Books.
Grabe, E., Nolan, F., and Farrar, K. (1998). “IViE—A comparative transcription system for intonational variation in English” in Proceedings of ICSLP 98, Sydney, Australia.
Hayes, B. (1989). “The prosodic hierarchy in meter” in Phonetics and Phonology. eds. P. Kiparsky and G. Youmans, vol. 1 (San Diego: Academic Press), 201–260.
Hostetter, A. B. (2011). When do gestures communicate? A meta-analysis. Psychol. Bull. 137, 297–315. doi: 10.1037/a0022128
Jowett, G., and O’Donnell, V. (2006). Propaganda and Persuasion. Thousand Oaks/London/New Delhi: Sage Publications.
Kibrik, A. (2018). Russian multichannel discourse. Part II. Corpus development and avenues of research [Russkiy mul’tikanal’nyy diskurs. Chast’ II. Razrabotka korpusa i napravleniya issledovaniy]. Psikhol. Zhurnal 39, 78–89. doi: 10.7868/80205959218020083
Kita, S. (2000). “How representational gestures help speaking” in Language and Gesture. ed. D. McNeill (Cambridge: Cambridge University Press), 162–185.
Kok, K., Bergmann, K., Cienki, A., and Kopp, S. (2016). Mapping out the multifunctionality of speakers’ gestures. Gesture 15, 37–59. doi: 10.1075/gest.15.1.02kok
Loehr, D. (2014). “Gesture and prosody” in Body–Language–Communication: An International Handbook on Multimodality in Human Interaction. eds. C. Müller, A. Cienki, E. Fricke, S. Ladewig, A. McNeill, and J. Bressem, vol. 2 (Berlin, München, Boston: De Gruyter Mouton), 1381–1391.
Lücking, A., Bergmann, K., Hahn, F., Kopp, S., and Rieser, H. (2010). “The Bielefeld speech and gesture alignment Corpus (SaGA)” in LREC 2010 workshop: Multimodal corpora–advances in capturing, coding and analyzing multimodality. (eds.) M. Kipp, M. J. -P. Martin, P. Paggio, and D. Heylen, 92–98
McNeill, D. (1992). Hand and Mind: What Gestures Reveal About Thought. Chicago: University of Chicago Press.
Mittelberg, I. (2018). Gestures as image schemas and force gestalts: A dynamic systems approach augmented with motion-capture data analyses. Cogn. Semiot. 11:1. doi: 10.1515/cogsem-2018-0002
Narayan, S. (2012). “Maybe what it means is he actually got the spot: physical and cognitive viewpoint in a gesture study” in Viewpoint in Language: A Multimodal Perspective. eds. B. Dancygier and E. Sweetser (Cambridge: Cambridge University Press), 97–112.
Núñez, R., and Cooperrider, K. (2013). The tangle of space and time in human cognition. Trends Cogn. Sci. 17, 220–229. doi: 10.1016/j.tics.2013.03.008
Parrill, F. (2012). “Interactions between discourse status and viewpoint in co-speech gesture” in Viewpoint in Language: A Multimodal Perspective. eds. B. Dancygier and E. Sweetser (Cambridge: Cambridge University Press), 97–112. doi: 10.1017/CBO9781139084727.008
Parrill, F., and Sweetser, E. (2004). What we mean by meaning: conceptual integration in gesture analysis and transcription. Gesture 4, 197–219. doi: 10.1075/gest.4.2.05par
Pascual, E. (2014). Fictive Interaction: The Conversation Frame in Thought, Language, and Discourse. Amsterdam-Philadelphia: John Benjamins Publishing Company.
Pierrehumbert, J. B. (1980). The phonology and phonetics of English intonation. PhD Dissertation. MIT.
Pleshakova, A. (2018). “Cognitive approaches: media, mind, and culture” in The Routledge Handbook on Language and Media. eds. C. Cotter and D. Perrin (London and New York: Routledge), 77–93.
Pouw, W., Trujillo, J., Bosker, H. R., Drijvers, L., Hoetjes, M., Holler, J., et al. (2023). Gesture and Speech in Interaction (GeSpIn) Conference. doi: 10.17617/2.3527196
Rühlemann, C., and Ptak, A. (2023). Reaching beneath the tip of the iceberg: A guide to the Freiburg multimodal interaction Corpus. Open Linguist. 9:1. doi: 10.1515/opli-2022-0245
Selkirk, E. (2003). “Sentence phonology” in The Oxford International Encyclopedia of Linguistics. eds. W. Frawley and W. Bright. 2nd ed (New York and Oxford: Oxford University Press).
Steen, F., Hougaard, A., Joo, J., Olza, I., Cánovas, C. P., Pleshakova, A., et al. (2018). Toward an infrastructure for data-driven multimodal communication research. Linguist. Vanguard. 4:1. doi: 10.1515/lingvan-2017-0041
Sweetser, E. (2012). “Introduction: viewpoint and perspective in language and gesture, from the ground down” in Viewpoint in Language: A Multimodal Perspective. eds. B. Dancygier and E. Sweetser (Cambridge: Cambridge University Press), 1–22.
Tobin, V. (2017). Viewpoint, misdirection, and sound design in film: the conversation. J. Pragmat. 122, 24–34. doi: 10.1016/j.pragma.2017.06.003
Turner, M. (2014). The Origin of Ideas. Blending, Creativity, and the Human Spark. New York: Oxford University Press.
Turner, M., Avelar, M., and Mendes de Oliveira, M. (2019). Atenção Compartilhada Clássica Mesclada e Dêixis Multimodal. Signo 44, 3–9. doi: 10.17058/signo.v44i79.12710
Uhrig, P., Payne, E., Pavlova, I., Burenko, I., Dykes, N., Baltazani, M., et al. (2023). “Studying time conceptualisation via speech, prosody, and hand gesture: interweaving manual and computational methods of analysis” in Gesture and Speech in Interaction (GeSpIn) Conference. (eds.) W. Pouw, J. Trujillo, H. R. Bosker, L. Drijvers, M. Hoetjes, J. Holler, L. Van Maastricht, E. Mamus, and A. Ozyurek
Ungerer, F., and Schmid, H.-J. (2013). An Introduction to Cognitive Linguistics : London, New York: Routledge.
Valenzuela, J., Pagán Cánovas, C., Inés, O., and Carrión, D. A. (2020). Gesturing in the wild: evidence for a flexible mental timeline. Rev. Cogn. Linguist. 18, 289–315. doi: 10.1075/rcl.00061.val
Vandelanotte, L. (2017). “Viewpoint” in The Cambridge Handbook of Cognitive Linguistics. Ed. B. Dancygier (Cambridge: Cambridge University Press), 2, 157–171.
Wilson, A., Wilkes, S., Teramoto, Y., and Hale, S. (2023). Multimodal analysis of disinformation and misinformation. R. Soc. Open Sci. 10:230964. doi: 10.1098/rsos.230964
Xiang, M., and Pascual, E. (2016). Debate with Zhuangzi: expository questions as fictive interaction blends in ancient Chinese philosophy. Pragmatics 26, 137–162. doi: 10.1075/prag.26.1.07xia
Keywords: multimodality, dataset, methodology, speech, gesture, future, conceptual blending, computer vision
Citation: Wilson A, Pavlova I, Payne E, Burenko I and Uhrig P (2024) World futures through RT’s eyes: multimodal dataset and interdisciplinary methodology. Front. Commun. 9:1356702. doi: 10.3389/fcomm.2024.1356702
Received: 16 December 2023; Accepted: 13 February 2024;
Published: 24 April 2024.
Edited by:
Claudia Lehmann, University of Potsdam, GermanyReviewed by:
Manon Lelandais, Université Paris Cité, FranceCopyright © 2024 Wilson, Pavlova, Payne, Burenko and Uhrig. This is an open-access article distributed under the terms of the Creative Commons Attribution License (CC BY). The use, distribution or reproduction in other forums is permitted, provided the original author(s) and the copyright owner(s) are credited and that the original publication in this journal is cited, in accordance with accepted academic practice. No use, distribution or reproduction is permitted which does not comply with these terms.
*Correspondence: Anna Wilson, YW5uYS53aWxzb25AYXJlYS5veC5hYy51aw==
Disclaimer: All claims expressed in this article are solely those of the authors and do not necessarily represent those of their affiliated organizations, or those of the publisher, the editors and the reviewers. Any product that may be evaluated in this article or claim that may be made by its manufacturer is not guaranteed or endorsed by the publisher.
Research integrity at Frontiers
Learn more about the work of our research integrity team to safeguard the quality of each article we publish.