- Phonetics Lab, Department of Linguistics, University of California, Davis, Davis, CA, United States
Laughter is a social behavior that conveys a variety of emotional states and is also intricately intertwined with linguistic communication. As people increasingly engage with voice-activated artificially intelligent (voice-AI) systems, an open question is how laughter patterns during spoken language interactions with technology. In Experiment 1, we collected a corpus of recorded short conversations (~10 min in length) between users (n = 76) and Amazon Alexa socialbots (a voice-AI interface designed to mimic human conversational interactions) and analyzed the interactional and pragmatic contexts in which laughter occurred. Laughter was coded for placement in the interaction relative to various speech acts, as well as for phonetic patterning such as duration and voicing. Our analyses reveal that laughter is most commonly found when the content of Alexa’s speech is considered inappropriate for the discourse context. Laughter in the corpus was also largely short in length and unvoiced– characteristics which are commonly associated with negative social valence. In Experiment 2, we found that a separate group of listeners did not distinguish between positive and negative laughter from our dataset, though we find that laughs rated as more positive are also rated as more excited and authentic. Overall, we discuss our findings for models of human-computer interaction and applications for the use of laughter in socialbot conversations.
1 Introduction
Increasingly, people are using spoken language to interact with technology, such as with voice-activated artificially intelligent (voice-AI) assistants (e.g., Apple’s Siri, Amazon’s Alexa, Google Assistant, Microsoft’s Cortana). Voice-AI assistants are often employed in request- or task-based interactions, such as making calls, setting timers or playing music (Ammari et al., 2019). Starting in 2017, users who wish to have a casual chat with an Amazon Alexa socialbot can do so via the Amazon Alexa Prize competition; anyone with an Alexa-enabled device can talk with one of several “socialbots” designed at various universities (Ram et al., 2018). Rather than taking part in solely task-based interactions (e.g., “Turn on the light,” “Set a timer”), these socialbots (more generically, this type of application is known as a “chatbot”) are voice-AI conversational agents who engage with users in non-task-based and naturalistic chit-chat on various topics (e.g., movies, music, sports). Recently, the development and public availability of OpenAI’s conversational AI ChatGPT (OpenAI, 2022) has also been a huge advancement in chatbot development and its public perception, as AI is now better able to assess user intentions and generate more human-like discourse. As this conversational use of devices continues to grow, it becomes even more important for researchers to understand the social mechanisms that underlie interactions between humans and machines.
1.1 Theoretical frameworks in human-computer interaction
Theories of technology equivalence, such as the Computers Are Social Actors framework (“CASA”) (Nass et al., 1994, 1997), propose that people apply the same social norms and conventions from human-human interactions to human-computer interactions, provided that they perceive the computer as human-like in some way. Voice-AI assistants such as Siri or Alexa have gendered names and voices (Habler et al., 2019) and thus are perceived as more human-like through those characteristics. Previous studies have investigated whether people replicate social dynamics of language use based on their perception of a device’s demographic characteristics; social factors such as gender (Cohn and Zellou, 2019; Zellou et al., 2021) or age (Zellou et al., 2021) mediate the ways in which we communicate with voice-AI assistants. Indeed, there is evidence demonstrating that within human-computer interactions, gendered patterns of phonetic alignment do exist (Cohn and Zellou, 2019; Zellou et al., 2021) and gender stereotypic effects are maintained (Nass et al., 1997). Other behaviors have also been shown to transfer from human-human interaction to interactions with devices; for example, Cowan et al. (2015) found that humans show similar patterns of linguistic alignment toward both device and human voices. Also, Ho et al. (2018) observe that positive effects resulting from emotional disclosure persist in interpersonal relations with chatbots.
Alternatively, it is also possible that alongside increasing familiarity with devices, people have developed specialized routines for human-computer interaction. Routinized accounts (Gambino et al., 2020) expand on equivalence accounts, proposing that in addition to applying social norms from human-human interaction, people also establish separate computer-specific scripts for conversation based on their real experiences interacting with technology. Work by Johnson and Gardner (2007) supports this by demonstrating that people do not treat computers as the same as humans, rating information provided by a computer as lower quality and themselves as being less influenced by the computer interlocutor. There is also some work showing that people show distinct speech learning patterns when they think they are hearing speech from a human vs. a device (Zellou et al., 2023) as well as work showing distinct speech patterns with voice-AI assistants (Cohn and Zellou, 2021; Cohn et al., 2022), also consistent with routinized accounts. It remains to be seen exactly to what extent human patterns of communication are maintained in human-computer interaction, and whether more unique patterns of interaction will emerge as naturalistic interconnection with devices is more common and normalized.
1.2 Laughter during human-human communication
In order to uncover the social and linguistic factors that govern user interactions with socialbots, the current study focuses on the use of laughter in spoken interactions. Laughter appears in highly similar forms across a wide range of cultures and languages (Edmonson, 1987; Sauter et al., 2010; Mazzocconi et al., 2020; Bryant and Bainbridge, 2022). While often associated with humor and joy, laughter can communicate complex emotional states such as romantic attraction (Rathcke and Fuchs, 2022), irony (González-Fuente et al., 2015; Mazzocconi et al., 2021), awkwardness (Osvaldsson, 2004; Mazzocconi et al., 2020; Mazzocconi and Ginzburg, 2023), and embarrassment (Proyer et al., 2013; Mayer et al., 2021), and signaling affiliation or asserting dominance (Wood and Niedenthal, 2018). For example, in an investigation performed by Provine (2001), only 10–20% of 1,200 analyzed instances of laughter were related to humor. Laughter is also observed in many different domains, such as clinical and educational settings (Adelswärd, 1989; Holt and Glenn, 2013; Beach and Prickett, 2017) as well as in casual friendly conversations (e.g., Vettin and Todt, 2004; Fuchs and Rathcke, 2018). Laughter in conversation has frequently been examined using conversation analysis frameworks (Jefferson et al., 1977; Jefferson, 1984; Glenn, 2010; Berge, 2017), which has revealed that laughter is highly ordered (Holt, 2011) and employed strategically and systematically in conjunction with speech (Glenn, 2003). In particular, laughter plays a role in pragmatic discourse, often found as a response to pragmatic and social incongruities in speech (Ginzburg et al., 2020; Mazzocconi et al., 2020; Mazzocconi and Ginzburg, 2023). Laughter is commonly found at prosodic boundaries and is said to “punctuate” speech rather than interrupting it (Provine, 1993; Vettin and Todt, 2004). At the same time, laughter does co-occur with speech and may even overlap speech within utterances (e.g., Kohler, 2008; Tian et al., 2016).
Notably, laughter is often thought to be “contagious” among interlocutors: People generally laugh more when they hear others’ laughter (Palagi et al., 2022; Scott et al., 2022). There is a body of work examining audience reactions to recorded laughter used as a backtrack for comedy media (Chapman, 1973; Lawson et al., 1998; Platow et al., 2005), which finds that the mere acoustic presence of laughter incites a listener to laugh in response. People laugh and smile more if others around them are doing so (Provine, 1993). At the same time, laughter is also shown to be governed by the social connection between two individuals (Scott et al., 2022). This is even more so the case if two interlocutors are friends rather than strangers (Smoski and Bachorowski, 2003a). While hearing pre-recorded laughter may prompt one to laugh, once that laughter is deemed to be artificial, its effects on an audience are weakened (Lawson et al., 1998).
The social characteristics of an interlocutor also influence a laugher’s behavior. Platow et al. (2005) find that people laugh more frequently and for longer durations at in-group laughter than out-group laughter. Reed and Castro (2021) also observe that listeners are sensitive to laughter in making decisions about group affiliation, and do not laugh in response to laughter at the social expense of someone in their in-group. Prior research additionally observes gendered patterns in laughter, although the findings are somewhat inconsistent. Martin and Gray (1996) observe that men and women laugh for equal amounts to a humorous audio tape. Smoski and Bachorowski (2003a,b) also find no differences in laughter in same-gender pairs. Additionally, Vettin and Todt (2004) note no differences between genders in both casual conversations and experimental debriefings. On the other hand, Grammer (1990) finds that women usually produce more laughter than men as speakers, and Provine (1993, 2001) finds that women also produce consistently high amounts of speaker laughter. More recently, McLachlan (2022) suggests that gendered laugh patterns may depend on the social context; men appear as likely to laugh as women in playful discussions, but women are more likely to laugh in more serious discussions often involving differences in status. Additionally, it is not solely the gender of the speaker that determines the amount of laughter they produce, but the interaction between the gender of both interlocutors (Jefferson, 2004; Rieger, 2009) along with social functions such as expressing attraction or the desire to be attractive, among others (Grammer, 1990; Rathcke and Fuchs, 2022).
In order to understand the social nature of laughter in these interactions, we analyze the acoustic characteristics of the laughter in conjunction with its conversational context in the current study. Prior laugh researchers take a holistic and qualitative approach to analyzing laughter, given that individuals exhibit highly variable and unique styles of laughing, while other works construct a typology that describes the function and characteristics of laughter (e.g., Vettin and Todt, 2004; Mazzocconi et al., 2020; Maraev et al., 2021). In the present study, we apply a functional typology of laughter based on its acoustic characteristics, following prior work (e.g., Tanaka and Campbell, 2014). Previous work suggests that social context influences laughter acoustics (Mehu and Dunbar, 2008; Wood, 2020), but also that when social context is removed, laughter is difficult for listeners to interpret (Rychlowska et al., 2022). We extend the investigation of whether laughter produced with varying acoustic features in isolation are perceptually distinct. For instance, it has been proposed that laugh duration may correlate with social closeness; laugh duration has been used as a measure of favorability (Platow et al., 2005), and longer laughs may be perceived as more rewarding (Wood et al., 2017) or authentic (Lavan et al., 2016). Listeners also distinguish between “negative” and “positive” laughs; unvoiced laughs are more likely to be perceived as negative, while voiced laughs are more likely to be perceived as positive (Bachorowski and Owren, 2001; Devillers and Vidrascu, 2007). For example, friends produce more voiced laughter than strangers (Smoski and Bachorowski, 2003a). With this prior work in mind, for the purposes of this study we predict that long and voiced laughter, when presented in isolation, will be evaluated more positively than short and unvoiced laughter. In order to validate this hypothesis, we conduct a perceptual study to determine whether the acoustic characteristics of a laugh have an effect on listeners’ ratings of its valence (following works such as Szameitat et al., 2011; Lavan et al., 2016; Wood, 2020).
In sum, it is suggested that laughter is not a random or automatic behavior. Rather, interlocutors are highly attentive to the social and linguistic dynamics of a conversational interaction while laughing. Therefore, the current study explores the possibility that speakers may also attend to an interlocutor’s perceived “human-likeness,” producing laughter differently in conversations with devices than with humans.
1.3 Laughter in human-computer interaction
The dynamics of laughter during conversational interaction lead us to make predictions about how laughter might be realized during conversational dialogs with devices, in this case with socialbots. Prior work demonstrates that listeners rate machine-like text-to-speech (TTS) voices as less communicatively competent than more human-like ones (Cowan et al., 2015; Cohn et al., 2022), and Siegert and Krüger (2021) find that listeners notice prosodic abnormalities in Alexa’s speech, characterizing it as “robotic” and “monotonous.” Thus, one prediction is that users perceive Alexa as artificial or socially incompetent and then laugh less often, signaling the social distance of the system as an “out-group” member (e.g., Platow et al., 2005). Additionally, the fact that Alexa, at the time of this study, does not laugh may dissuade human interlocutors from laughing, paralleling mirroring of laughter observed in human-human interaction (Palagi et al., 2022; Scott et al., 2022). The average user does not encounter laughs from Alexa in either day-to-day use or in Amazon Alexa Prize conversations, meaning that there is no “contagious” dynamic to laughter present in these interactions. Will users still laugh in conversations with Alexa?
On the other hand, if participants do laugh in conversations with Alexa, what contextual factors might predict such laughter? The existence of laughter in conversations with Alexa is not enough on its own to signify a positive user experience, and identifying the context surrounding each laugh is critical in determining its function, as shown in previous work (e.g., Curran et al., 2018; Ginzburg et al., 2020; Mazzocconi et al., 2020; Rychlowska et al., 2022). For example, the existence of laughter in a conversation can indicate positive emotion and a willingness to cooperate with one’s speech partner (Smoski and Bachorowski, 2003a), which could reveal laughter as an indication of positive user experience.
In parallel with research advancing machine detection of laughter (e.g., Urbain et al., 2010; Cosentino et al., 2013; Petridis et al., 2013a,b; Gillick et al., 2021; Mascaró et al., 2021), there is a growing body of work examining laughter in human-computer interactions (Soury and Devillers, 2014; Tahon and Devillers, 2015; Weber et al., 2018; Maraev et al., 2021; Mills et al., 2021; Mazzocconi, 2022). For example, Soury and Devillers (2014) compared laughter in passive tasks (e.g., watching a video) and in repeating a tongue twister with a Nao robot; they found the most laughs in response to the videos, the majority of which were coded as amused laughs (positive affect). On the other hand, laughs coded as signaling embarrassment were much more frequent in the interactive task with the robot. Additionally, they found that participants with higher extraversion scores tended to produce more amused laughs and additionally produce longer laughs. Maraev et al. (2021) classifies users’ laughter that occurs after an ASR or natural language understanding (NLU) error with a task-based dialogue system as a “rejection signal,” and characterizes this laughter as negative feedback, as well as “awkwardness” following a long pause. Weber et al. (2018) describe a robotic joke teller system that uses reinforcement learning to maximize users’ voiced laughter and smiles.
Tahon and Devillers (2015) examined laughter in a dataset of spoken interactions with a Nao robot, with two tasks (JOKER database): interacting with the robot as it told jokes and an emotion generation game, where the user acted emotions to be recognized by the robot. They found 140 positive laughs (averaging 1.88 s in duration) and 117 negative laughs (averaging 0.99 s in duration). Their classifier, built on this dataset, additionally identified 226 positive laughs (averaging 1.12 s in duration) and 27 negative laughs (averaging 0.76 s in duration) in a separate dataset (ARMEN dataset; Chastagnol and Devillers, 2012), where participants were asked to act out emotions in imagined scenarios; in one task, they completed the emotions with an apparent dialogue system that responded with empathy and understanding (using a Wizard-of-Oz design, where responses were controlled by the experimenters). Mazzocconi (2022) raises “social incongruities” as one potential source of laughter, wherein one possibility is that a laugh “reassures the interlocutor that the situation is not to be taken too seriously” (p. 120). This is one motivation for laughter that could be present in both human-human and human-computer interaction. By examining the distribution of laughter in different interactional contexts with the socialbots, we hope to use these measures of laughter to better understand participants’ experiences with a conversational interface.
1.4 Current study
In order to uncover information about the use of laughter in the present study, we will examine how laughter covaries with its context within the communicative interaction with Alexa socialbots, as well as its acoustic patterning across contexts, and external raters’ perception of the different acoustic laughter types from these conversations. The goal is to provide a descriptive analysis of laughter occurrences in human-Alexa socialbot conversations. We predict that the voicing and length of a laugh will indicate its valence, which in turn will reveal the nature of the user’s experience with the socialbot. Specifically, we predict that long and voiced laughter will be perceived as positive, while short and unvoiced laughter will be perceived as negative.
There are several potential implications for the possible meanings of the presence of positive and negative laughter in relation to the device’s mode of communication. The Alexa socialbots display some conversational oddities unique to computer-generated speech, such as (perceived) robotic text-to-speech (TTS) and automatic speech recognition (ASR) errors not found in human-human interactions and that are salient to users; for example, participants consciously report abnormalities in Alexa’s speech (Siegert and Krüger, 2021) and rate voice-AI systems as less communicatively competent (Cohn et al., 2022). We predict that participants will laugh less frequently in non-human-like contexts than in human-like contexts, as this signals the machine’s out-group status (cf. Platow et al., 2005). Additionally, we predict that these scenarios will also elicit short, negative laughter, given the negative effects of being misunderstood or understanding the system (e.g., Kim et al., 2021).
Furthermore, Alexa may sometimes utter statements that imply she has a human body or performs human actions that she cannot do (such as stating that she attends school). These are inconsistent with the user’s mental models of Alexa as a machine. We predict these “too-human” statements highlight Alexa’s machine nature and the incongruency will lead to laughter. This prediction stems from the frameworks that propose that laughter is driven by incongruency (Bergson, 1900; Howarth, 1999): in observing “something mechanical in something living” (e.g., inelasticity/rigidities that lead a person to fall). In the case of a socialbot, laughter might be reflecting the converse: perception of human-likeness in something mechanical. We predict this laughter will be long and positive, reflecting the humor in these scenarios.
We will also examine the role of gender and individual differences in laughter with socialbots. Whether or not there are actually differences in how often men and women generally laugh is somewhat debatable. Previous studies in human-human interaction find that there may be no gender differences in how much men and women laugh (Martin and Gray, 1996; Smoski and Bachorowski, 2003a,b; Vettin and Todt, 2004), or alternatively, that women laugh more than men (Grammer, 1990; Provine, 1993, 2001), though the gender of interlocutor is also important (Jefferson, 2004; Rieger, 2009). We expect to find similar gendered laugh dynamics on the grounds that gendered patterns persist in human-computer interaction (Habler et al., 2019). Additionally, prior work reports that extroverts tend to laugh more in conversations than introverts (Deckers, 1993; Wood et al., 2022) and tend to produce more amused laughs in interactions with machines (Soury and Devillers, 2014). Therefore, we also predict that a measure of the extraversion personality traits will correlate with laughter occurrences in human-computer interaction, and more extraverted participants will laugh more often—and produce more positive laughs—than less extraverted ones.
To these ends, we performed two experiments. In the first experiment, we investigate the patterning of laughter within a corpus composed of naturalistic, non-task-based 10-min conversations between a human user and Alexa. We examine the conversational contexts in which people laugh and the acoustic features of those laughs. We also examine whether users’ gender and personality traits (here, extraversion) predicts their patterns of laughter in social conversations with Alexa. In the second experiment, we perform a perceptual experiment using laugh tokens from the corpus in the first experiment. We ask participants to listen to laughs separated from their contexts, and make judgments (how positive, excited, authentic; cf. Szameitat et al., 2022) based solely on their acoustic characteristics.
2 Experiment 1: corpus analysis of laugh patterns
The purpose of Experiment 1 was to collect a corpus of speakers’ conversational interactions with Amazon Alexa socialbots in order to analyze laughter patterns within these interactions.
2.1 Methods
2.1.1 Participants
Participants (n = 76) were native English speakers, recruited from the UC Davis psychology subjects pool. All participants were native English speakers ages 18–27, including 42 female, 33 male, and 1 nonbinary participant. Forty-one participants reported that they speak other languages as well (Spanish, Mandarin, Cantonese, Urdu, Punjabi, Malayalam, Sindhi, Romanian, Tamil, Arabic, Korean, Tagalog, Vietnamese, Gujarati, American Sign Language). Of the participants who produced laughter for analysis (see below for details), 22 spoke other languages (Spanish, Mandarin, Cantonese, Urdu, Tamil, Arabic, Korean, Tagalog, American Sign Language). All participants reported no hearing loss or auditory disorders. All participants consented to the study following the UC Davis Institutional Review Board and received course credit for participation in the study.
Participants’ reported prior experience with using voice assistants in their daily lives (provided in Supplementary Appendix A). Most participants reported that they had never used Amazon’s Alexa, Google’s Assistant or Microsoft’s Cortana before, having the most experience with Apple’s Siri. Seven participants reported having zero experience with any voice assistant. Participants reported using voice assistants for simple task-based requests, most commonly using the assistants for setting reminders, playing music, asking about the weather, navigation, searching for definitions and hands-free navigation.
2.1.2 Procedure
At-home user studies occurred across May–July 2021, where speakers participated in an online experiment. The participants completed the study at home using their own devices, activating the socialbot and recording the chat with their computer microphone in a quiet room. Participants were told that they were interacting with the Alexa app and not a human. Participants were instructed to install the Alexa app onto their own phones and initiate one of the Amazon Alexa Prize socialbots by uttering the phrase, “Alexa, let us chat.” Participants engaged one of the socialbots from the Amazon Alexa Prize (Ram et al., 2018) at random and thus we did not control for which socialbot was used. The participants were instructed to “record yourself having one conversation with the socialbot for 10 min,” but were not given specific instruction on what to talk about, or what the researchers would be examining in the study. They were also instructed to restart the conversation if the bot crashed before 10 min had been completed. The chats spanned a wide range of topics (e.g., sports, movies, animals, books) as well as general chit-chat and questions probing the participants’ interests. Recordings were made in Qualtrics with Pipe,1 including a practice recording stage prior to the conversation (cf. Cohn et al., 2021).
After the conversation, participants completed a ratings task to gauge their perception of the socialbot, giving numerical ratings on a sliding scale (0–100) for overall engagement (0 = not engaging; 100 = engaging) as well as how human-like (0 = not human-like, 100 = extremely human-like) and creepy/eerie (0 = not creepy/eerie, 100 = extremely creepy/eerie) they found the socialbot. They were also prompted to provide a short response detailing any errors that arose during the experiment or additional comments, and answered questions regarding the use of voice assistants in their everyday lives. Participants were also asked whether the bot “seemed like a real person” and to explain why or why not in a short answer. Additionally, the participants’ extraversion was assessed via the revised Eysenck personality questionnaire (Colledani et al., 2018): participants answered the 23 items judging extraversion rather than the full survey (provided in Supplementary Appendix B). The participants received scores on a scale ranging from 1 to 23, with higher scores indicating higher levels of extraversion (the distribution of participants’ extraversion scores is provided in Supplementary Appendix B).
2.1.3 Laugh annotation
The conversation audio was transcribed using Sonix.2 All transcriptions were reviewed and corrected for accuracy by a trained graduate student researcher (the first annotator). While reviewing the transcripts for accuracy, the first annotator labeled each instance of laughter in a Praat Textgrid (Boersma and Weenink, 2023), but did not annotate the duration or context of the laughter. The laugh data was later reviewed separately by the first author (the second annotator). Separate from the first annotator, the second annotator (the second author) reviewed the full conversational corpus for any missing instances of laughter, and recorded the conversational context, duration, and other acoustic properties of each laugh. Inter-annotator reliability was analyzed using the psych R package (Revelle and Revelle, 2015); the first and second annotators were moderately in agreement for laugh types: Cohen’s kappa ranging between κ = 0.43 and κ = 0.67 (estimate: κ = 0.55). For observations where the first two annotators disagreed, a third annotator (the third author) coded those laughs and discussed them with the other annotators until agreement was reached.
Laughter events were coded as any vocalization that would reasonably be identified as a laugh by an ordinary listener. Laughter was grouped into three distinct types: primarily voiced laughter that is sometimes vowel-like or melodic, turbulent unvoiced laughter, and speech-laughs produced concurrently with a participant’s utterance. Voicing in voiced laughter typically manifested in vowel-like segments of the laugh. While voiced laughs occasionally included a prolonged voiceless inhale or exhale, we categorized them as voiced if the majority of the laugh’s duration (> 50%) contained voicing. Speech-laughs were characterized by a sharp spike in aspiration at a syllable onset or unvoiced sound, glottal pulses through voiced segments such as vowels, and an overall breathy quality.
The onset of laughter duration was marked at the start of an exhale, aspiration spike or glottal pulse. The offset was marked at the end of the last bout, or rhythmic “ha”-like pulse within a laughter event, at the end of a laugh. Any laughter that began within an utterance was annotated from the onset of the irregular glottal behavior. Prolonged exhalation or inhalation at the edge of a laugh was included in the duration measurement (as seen in Urbain et al., 2010). Long bursts of laughter were not divided into separate laughs unless there was an extended period of silence (> 600 ms) between each laughter event (following Rathcke and Fuchs, 2022).
We defined six types of “laugh contexts” at the outset of coding the laughter seen in each interaction. Table 1 provides the criteria used to categorize each laugh into one of these six categories, as well as any subcategorization criteria, and examples of each. These contexts are events caused by the Alexa socialbot’s behavior which triggered a laugh from the participant. Some are unique to an interaction with a machine, such as errors in the TTS or ASR. All categorizations were approached from the perspective of the human listener. That is, while some behaviors such as abrupt topic changes could also have been generated by a misrecognition of the participant’s speech, if the participant was likely to perceive it as social awkwardness rather than a machine error, it was annotated as such.
In order to capture greater nuance within each category, three of the laugh contexts were given further subcategorizations. TTS and ASR errors are grouped together as one category, but information about both distinct types was also annotated separately. Discourse atypicalities represent speech from Alexa that was inappropriate for the current conversation on the basis of its pragmatic content. Specifically, Alexa may change the topic abruptly (abrupt topic change), say something that implies she has human characteristics (too-human content), or generally say things that may be socially awkward or misplaced and thus trigger a laugh, but could realistically be said by a human (inappropriate content). Discontinuities in timing that triggered laughter were annotated as either interruptions or long pauses, respectively.
2.2 Analysis and results
Laughter events were analyzed in terms of the distribution (section 2.2.1) and duration (section 2.2.2) of laughter, as well as how these patterned according to laugh subcategorization and type (sections 2.2.3 and 2.2.4). We also explore whether speaker gender (section 2.2.5) and ratings of the device (section 2.2.6) affected laughter.
2.2.1 Laugh distribution
Thirty three speakers did not produce any laughter, so their interactions were excluded from the analysis. The subset of the sample for analysis included laughter produced by 43 participants (28 female, 14 male, 1 nonbinary). Within those interactions, the total number of laughs produced was 136. The counts of laughter events produced in conversations with Alexa across the different contexts are illustrated in Figure 1. As seen, laughs produced after a discourse atypicality were most frequent (n = 69), and in fact account for slightly over 50% of all laughter events. In order to test the relation between laughter occurrence and context, a chi-square test of independence was used. The relation was significant [χ2(10, N = 136) = 138.6, p < 0.001] indicating that laughter was more likely to occur in some contexts than in others.
2.2.2 Laugh duration
While laugh duration is variable across individuals, large databases of laughter in human-human interaction report mean duration ranging from 1.65 to 3.5 s (Urbain et al., 2010; Petridis et al., 2013a,b). In contrast, a sizable majority of the laughter found in our corpus was less than 1 s in length (100 out of 136 total), indicating that these laughs are short in comparison to prior findings. Figure 2 displays the differences in laugh duration across all laugh contexts.
Laugh duration was modeled with a linear regression in R. The model included laugh context as a fixed effect with six levels (timing error, TTS/ASR error, post-own speech, conversation end, humorous statement, reference level = discourse atypicality, treatment-coded), Gender as a fixed effect with three levels (male, nonbinary, reference level = female, treatment-coded), and their interaction.3
The model showed that the duration of laughter following discourse atypicalities was not significantly different than those following timing errors (p = 0.3), TTS/ASR errors (p = 0.9), post-own speech laughs (p = 0.2), humorous statements (p = 0.7), or laughter at the conversation end (p = 0.2). There was an effect of Gender (Coef = −0.43, t = −2.24, p = 0.02), indicating that men produced shorter laughs than women. There was no difference in duration between laughs produced by non-binary and women speakers (p = 0.4).
As mentioned above, short laughter (less than 1 s in length) was overall more frequent than long laughter (short laugh count = 100, long laugh count = 36). As discussed in the previous section, laughs produced after a discourse atypicality were significantly more frequent than laughs in other contexts. These laughs also tended to be shorter (57 out of 69 discourse atypicality laughs were less than 1 s). In contrast, laughs following humorous statements and post-own speech were more evenly distributed across short and long durations.
2.2.3 Laugh distribution and duration by subcategorization
The distribution of laughter events produced at various types of discourse atypicalities are illustrated in Figure 3. The three events that constitute discourse atypicalities are inappropriate content, too-human content, and abrupt topic changes. In order to test the relationship between these three types, a chi-square test of independence was used. The relation was significant [χ2(2, N = 69) = 43.8, p < 0.05] indicating that laughter was more likely to occur at some types of discourse atypicalities than others. Forty eight laughs were produced at inappropriate content, 14 laughs were produced at too-human content, and 6 laughs were produced at abrupt topic changes, with laughter at inappropriate content constituting approximately 70% of all laughter at discourse atypicalities. Thus, we can reasonably infer that laughs triggered by inappropriate content were the most frequent within this category.
Laugh duration between the subcategorizations was modeled with a third linear regression model. Laugh subcategorization was used as a fixed effect with three levels (Too-human, abrupt topic change, reference level = inappropriate content, treatment-coded). There was a significant difference in duration between laughs produced at inappropriate content and too-human content (Coef = −0.6, t = −3.6, p < 0.05), but no significant difference between laughs at inappropriate content and abrupt topic changes (p = 0.2).
2.2.4 Laugh distribution and duration by laugh type
The distribution of laughter events according to their type (voiced, unvoiced, or co-occurring with speech) is shown in Figure 4. Unvoiced laughs were most frequent, and accounted for more than 44% of all laughs (unvoiced laugh count = 60; voiced laugh count = 54; speech laugh count = 22). In order to test the relation between laughter occurrence and laugh type, a chi-square test of independence was used. The relation was significant [χ2(2, N = 136) = 18.4, p < 0.01] indicating that some laugh types were more likely to occur than others. We can observe according to the figure that the count of unvoiced laughs is higher than the count of voiced laughs, which is higher than the count of speech-laughs. Therefore, we infer that unvoiced laughter occurs most often.
Laugh duration across all laugh types was again modeled with a linear regression model. Laugh type was added as a fixed effect with three levels (voiced, speech-laugh, reference level = unvoiced, treatment-coded). There was a significant difference in duration between unvoiced laughs and voiced laughs (Coef = 0.42, t = 3.4, p < 0.001) and between unvoiced laughs and speech-laughs (Coef = 0.44, t = 2.7, p < 0.001), but no significant difference between voiced laughs and speech-laughs (p = 0.9).
2.2.5 Laugh distribution by gender
The counts of participants and laughter events by gender, as well as their relative proportions, are given in Table 2. In addition to the data on men and women, one instance of laughter was produced by a nonbinary speaker. While there is twice as much laughter produced by women compared to men, there are also roughly twice as many women in the study. Since the proportion of laughter produced by a given gender and the proportion of participants of that gender are essentially equal, we observe no substantial evidence for gender differences in the amount of laughter in conversations with Alexa.
2.2.6 Laughter and ratings
We examined the relationship between the amount of laughter a participant produced within each conversation with Alexa and their extraversion, as well as how they responded to each of the rating questions. Participants who did not laugh were excluded from this main analysis (but note that we conducted a post hoc analysis including all participants).4
The number of laughs a speaker produced in an interaction was modeled with a linear regression model in R. We included fixed effects for the extraversion score as well as satisfaction, creepy/eerie, and human-like ratings. All predictors were centered around the mean. We checked for collinearity using the check_collinearity package in R (Lüdecke et al., 2021), and found low collinearity between the model predictors. The model showed no effect of extraversion (p = 0.8), satisfaction rating (p = 0.3), human-like rating (p = 0.07) or creepy/eerie-ness rating (p = 0.9) on the number of laughs.
3 Experiment 2: perceptual evaluation of the acoustic characteristics of laughter
Prior work in laughter research shows that speakers produce a range of laugh types in human-human interaction, such as variation in voicing (Bachorowski et al., 2001). Acoustic properties of laughs are also associated with differences in perceived valence, arousal, and authenticity by external listeners (Szameitat et al., 2011; Lavan et al., 2016; Wood et al., 2017). For example, longer laughs are perceived as more rewarding and authentic (Lavan et al., 2016; Wood et al., 2017) and therefore more positive; listeners also perceive voiced laughter as more positive than unvoiced laughter (Devillers and Vidrascu, 2007). Using the laughs from Experiment 1 as stimuli, our goal in this second experiment is to test how an independent group of listeners rate the valence, arousal, and authenticity of each laugh based solely on its acoustic characteristics (i.e., presented in isolation, removed from the pragmatic context of the discourse).
3.1 Methods
3.1.1 Participants
Twenty five participants were recruited from the UC Davis Psychology subjects pool for this experiment. None of the participants participated in Experiment 1. All participants completed the study online via Qualtrics and received course credit for their participation. The participants were college students ages 18–23. Of the 25 total participants, 16 identified as female, eight identified as male, and one as gender non-conforming. All participants were native English speakers (16 also reported that they spoke other languages, including Spanish, Mandarin, Cantonese, Korean, Hindi, and Tamil) and reported no hearing loss or auditory disorders. All participants consented to the study following the UC Davis Institutional Review Board and received course credit for participation in the study.
3.1.2 Stimuli
Stimuli consisted of 56 laughs, which were extracted directly from the corpus data (Experiment 1). Thirty four of these laughs co-occurred with speech and 22 laughs did not. Of the laughter not overlapping speech, 24 were voiced and 10 were unvoiced. The duration of these laughs ranged from 150 to ~3,000 ms. In order to extract speech-laughs from the data, in cases where laughter overlapped with speech, we included the entire last word or words (rather than excising portions of words) that overlapped with the laughter but not the entire utterance preceding it. Laugh tokens were removed if they overlapped with Alexa’s voice, as the participant was laughing while the device was speaking, since this made the laughs difficult to hear. Additionally, we excluded overly noisy and short laughs which could not reasonably be identified as laughter by a listener when extracted and played in isolation from the larger recording. Seventy seven laughs from the corpus data were excluded from the stimuli for these reasons.
3.1.3 Procedure
This experiment included two blocks (order fixed). In the first block, the participants were asked to rate the laughter that did not co-occur with speech; in the second block, they rated the laughter that did co-occur with speech (speech-laughs). Laughter that did not overlap with speech was included in a separate block from speech-laughs because they may co-occur with lexical items that may induce a valence bias (such as laughter that overlapped the words “yes” or “no”).
On a given trial, participants listened to each laugh twice in succession, with a pause of 350 ms between the repetitions. They were then asked to rate what they had heard on three different scales before moving onto the next item. Following the measures used in prior work (Lavan et al., 2016), the participants were asked to rate each laugh on sliding scales ranging from 0 to 100 in terms of how negative or positive (valence; 0 = negative, 100 = positive), calm or excited (arousal; 0 = calm, 100 = excited), and real or fake (authenticity; 0 = real, 100 = fake).
3.2 Analysis and results
The acoustic measures we investigated are the same as in the prior corpus study (Experiment 1); we examined the effect of laugh type (voiced, unvoiced, or speech-laugh) and duration (continuous) on all ratings.
To test the relationship between laugh type, duration and the listeners’ ratings, we used three linear mixed effects models with the lme4 package in R (Bates et al., 2015), with the dependent variables of valence, arousal, and authenticity. Each of the three models had the same structure: laugh type (voiced, speech-laugh, reference level = unvoiced, treatment-coded), laugh duration (continuous), and their interaction. The random effects structure included by-listener random intercepts, as well as by-item random intercepts and by-listener random slopes for laugh type. Due to singularity errors, we simplified the random effects structure in each model.5 We found no evidence for collinearity between the model predictors in any of the three models using the check_collinearity package in R (Lüdecke et al., 2021). p-values for all linear mixed effects models in the study were calculated using the lmerTest package in R (Kuznetsova et al., 2017).
None of the models showed evidence that laugh type significantly affects valence (all p > 0.05), arousal (p > 0.05), or authenticity ratings (p > 0.05), as illustrated below in Figure 5. Duration also did not have a significant effect on any rating.
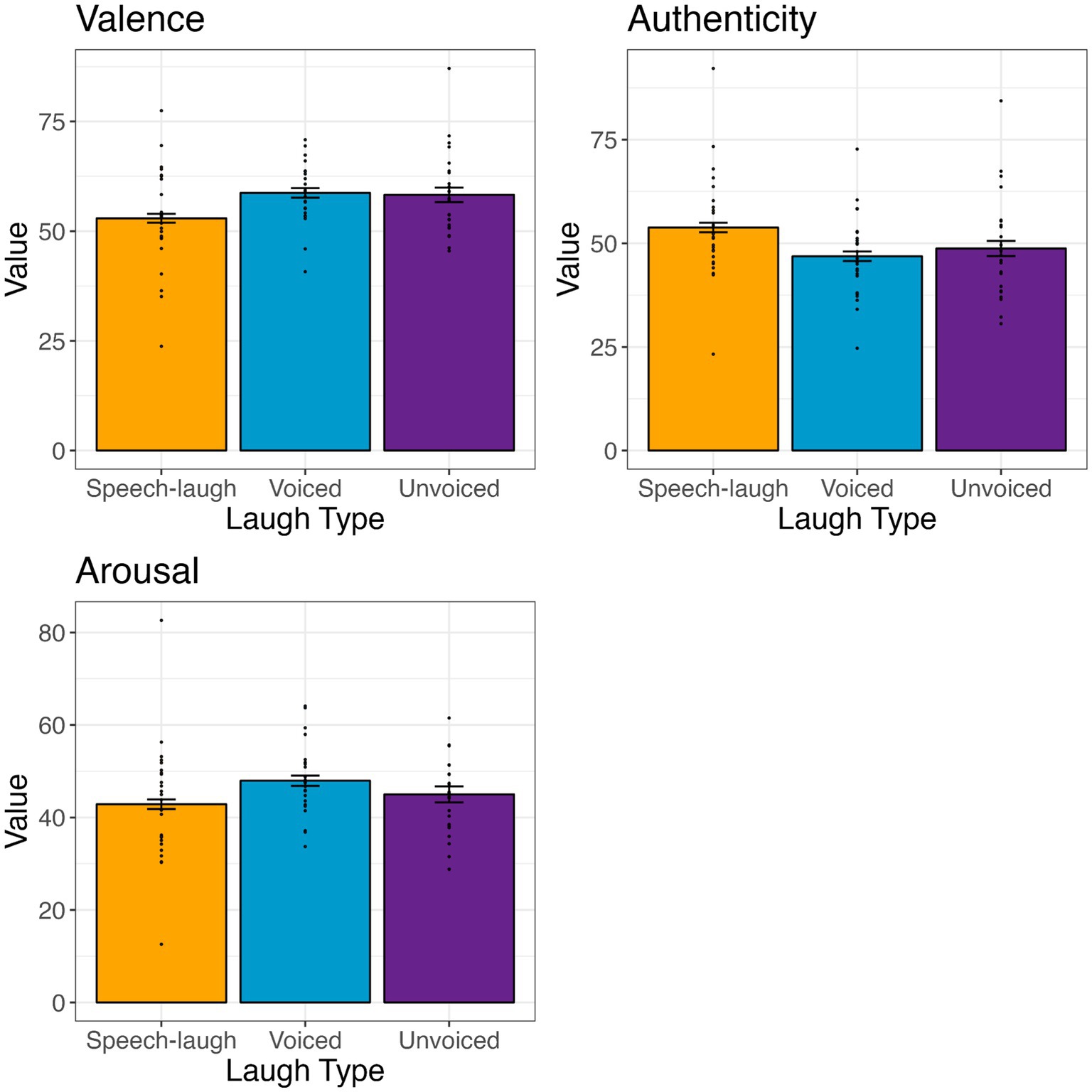
Figure 5. Average valence, arousal, and authenticity ratings by laugh type. Values on the y axis indicate valence (0 = negative, 100 = positive), arousal (0 = calm, 100 = excited), or authenticity (0 = real, 100 = fake).
We ran a separate linear mixed effects model to examine the interactions between the three rating types. The model included valence as a function of authenticity and arousal, which are continuous predictors. The model also included by-item and by-listener random intercepts. All values were centered around the mean.6 We found a relationship between valence and arousal ratings: laughs rated as more positive were also rated as more excited (Coef = 0.29, t = 11.93, p < 0.001) (see Figure 6, left panel). As seen in Figure 6 (right panel), there was a negative relationship between valence and authenticity ratings; laughs rated as more positive were also rated as less fake (Coef = −0.33, t = −15.39, p < 0.001).
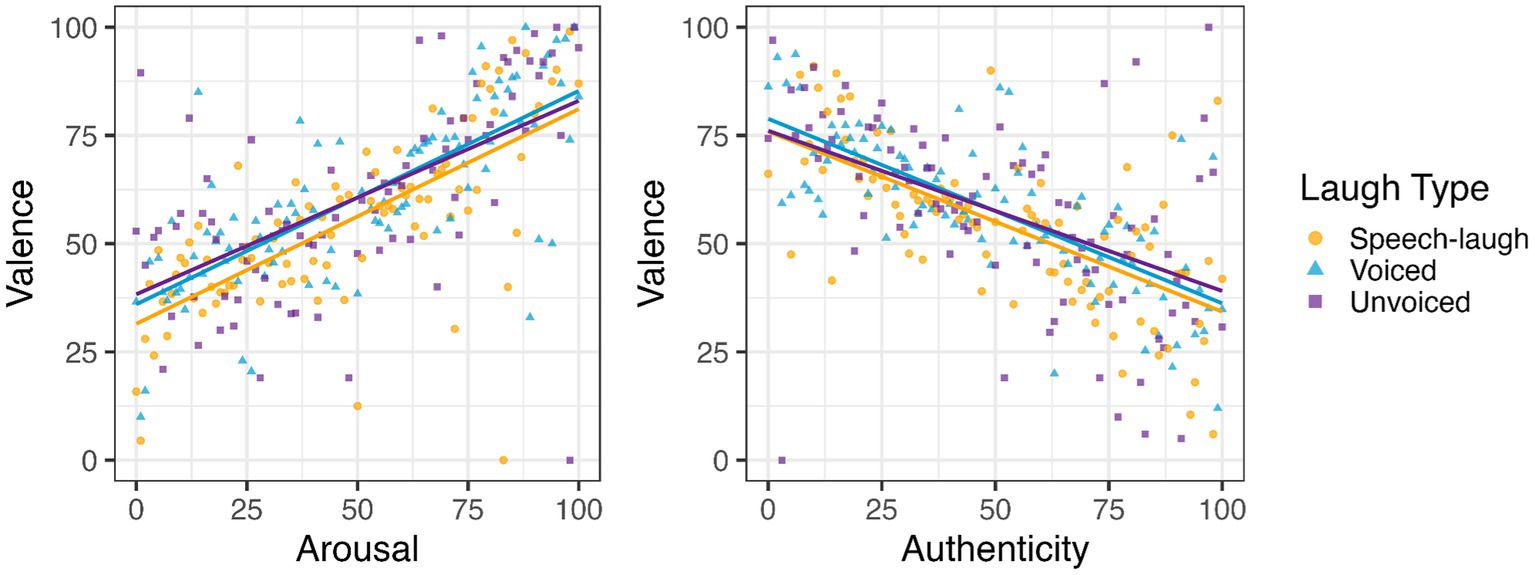
Figure 6. Valence-authenticity and valence-arousal correlations. Values on the x axis indicate valence (0 = negative, 100 = positive), arousal (0 = calm, 100 = excited), or authenticity (0 = real, 100 = fake).
4 General discussion
The current work aims to classify what situations prompt people to laugh in conversations with a socialbot, the acoustic properties of the laughter (Experiment 1), and whether that laughter is realized in ways that can be independently perceived as having positive or negative social meaning (Experiment 2).
4.1 Relatively infrequent laughter in socialbot conversations
One question raised by the present study is whether participants would produce any laughter at all in conversations with Alexa. When talking to the Alexa socialbots, we found that participants tend to laugh relatively infrequently. Of the 76 total participants, only 43 (~57%) produced any laughter at all. Those who did laugh averaged 3 laughs per 10 min. When compared to the frequency of laughter in descriptions of corpora of face-to-face human-human interaction, the amount of laughter produced in the present study is relatively low. For example, work on friendly human-human conversations of similar lengths finds greater amounts of laughter: Vettin and Todt (2004) report an average of 5.8 laughs per 10 min across talkers (SD = 2.5). Mazzocconi et al. (2020) find varying averages across speakers of different languages, but all the reported rates are greater than in our corpus—French dyadic exchanges contain 45 laughs per 10 min, and Mandarin Chinese dyads produce 26 laughs per 10 min. In Fuchs and Rathcke (2018) study on speed-dating, on average daters produced 21 laughs in a span of 5 min (SD = 9.3). Thus, our results suggest that people laugh less in conversations with devices than when talking to other humans (but note we do observe large cross-speaker variation, with the greatest amount of laughter from any one participant 10 laughs/10 min).
4.2 Laughter driven by discourse atypicalities
We predicted that participants would laugh less frequently in non-human-like contexts. However, when considering the scenarios that prompted laughter, we found that participants who did laugh tended to do so most frequently after discourse atypicalities (inappropriate content, too-human content, and abrupt topic changes) than after the other conversational scenarios observed in our corpus. These other discourse contexts included TTS and ASR errors, speech timing errors, humorous statements, and the end of a conversation, as well as laughs following the participant’s own speech. These findings parallel those in human-human interaction, where people laugh at “social incongruities” to save face for an interlocutor, sometimes in order to convey levity to their interlocutor’s odd response (Mazzocconi et al., 2020; Mazzocconi, 2022). Thus, participants laughed often at errors by Alexa that were human-like in nature, rather than reacting to prosodic peculiarities or robotic mispronunciations unique to the TTS generation. This suggests that laughter occurs for individuals and contexts where Alexa is perceived as more human-like.
For example, Alexa began to tell a joke to a participant in one interaction, asking “What is a dog’s favorite food?” The participant asked “Meat?” in response, which prompted Alexa to reply “Meat is a great name for a dog.” This elicited laughter from the participant. Later, when asked if they experienced any technical difficulties in the post-recording survey, the participant wrote:
“I thought that the bot was asking me what my dog's favorite food was and I said ‘meat.’ However, the bot mistakenly thought my dog's name was meat, which made the conversation awkward.”
In another example, in one conversation where the topic was about sports, Alexa switched the topic abruptly in response to the participant’s speech, stating “I was built by a team competing in the Alexa Prize.” In reply, the participant stated “Well [pause] this conversation is a bit hard to follow” and laughed. Again, in the survey that participant wrote:
“Yes. There were a lot of errors in my conversation, it did not feel fluid at all and definitely felt like I was talking to a robot. A lot of times by errors I mean that she did not have an appropriate response to my question or the conversation was just choppy and hard to follow.”
Here, while the participant notes that talking with Alexa feels like talking to a robot, the errors they choose to highlight relate to the appropriateness of a response and the fluidity of the conversation, rather than TTS or ASR errors. These types of atypical responses from Alexa were noted frequently by participants, such as one who wrote that “When I told her I had not ate [sic] breakfast she said that was awesome.” These are utterances that do not contain machine-specific speech errors, and could reflect a human’s misunderstanding as well. However, due to their social oddness, listeners still flag them as awkward or inappropriate. We also predicted that participants would laugh at “too-human” statements, where Alexa implies that she herself is human, but did not find more laughter in these contexts than in other socially awkward contexts where Alexa does not mention her human-ness. Overall, people tended to laugh when Alexa said something not socially acceptable or conversationally misplaced, behaviors which are found in human-human interaction. Laughter in response to this awkward speech from Alexa may be used as a way to alleviate social discomfort (Mazzocconi et al., 2020; Mazzocconi, 2022).
At the same time, participants do not produce nearly as much laughter when the socialbot disrupts the timing of the interaction or makes an error that highlights its machine-ness. However, several participants did make note of timing errors in the ending survey, stating that “Alexa would not let me finish speaking” or “Alexa… does not possess the social skills that are considered normal (i.e., not interrupting or taking long pauses before answering).” The “robotic-ness” of Alexa’s voice was also mentioned multiple times when participants were asked if Alexa “seems like a real person,” with participants giving statements such as “too robotic of a voice” and “Alexa sounds like a robot.” These responses are consistent with prior findings illustrating that users find Alexa robotic and less competent in conversation (Cowan et al., 2015; Siegert et al., 2020; Cohn et al., 2022). This suggests that within human-computer interactions, timing and TTS/ASR errors may be noticeable but not “laughable” to human listeners, compared to human-like social incongruities which prompt more laughter. This finding is consistent with the Bergsonian proposal that laughter is driven by incongruency between human-like and mechanistic elements in a person (Bergson, 1900; Howarth, 1999). Here, we see that the converse is also the source of laughter: a human-like element in a machine. While speculative, it is possible that we observe less laughter in cases where an error is clearly machine-specific (e.g., TTS, ASR) due to less incongruency, as a machine is expected to produce machine-like errors. Taken together, we see the placement and function of laughter in these conversations is nuanced and contextual, reflecting work on laughter in human-human (Holt and Glenn, 2013; Wood and Niedenthal, 2018; Rathcke and Fuchs, 2022; Mazzocconi and Ginzburg, 2023) as well as human-computer interaction (Soury and Devillers, 2014; Tahon and Devillers, 2015; Maraev et al., 2021).
4.3 Acoustic properties of laughter
Our acoustic analyses of laughter revealed that laughs in socialbot conversations were overwhelmingly short in duration. Laughter annotation differs across corpora, leading to varying reports on the average duration of laughter. In the current study, our inclusion of extended breaths when measuring the boundaries of laughter onset and offset likely affected the measured laugh durations. Urbain et al. (2010) use a similar annotation method to that used in our study, including the final inhale/exhale in the duration measure, yet they report overall much longer laugh durations (3.5 s for spontaneous laughter and 7.7 s for elicited laughter). In comparison, the majority of laughs in the current study were less than 1 s long, indicating that most laughs in the data were short relative to those observed in human-human interaction. Our findings are consistent with relatively shorter laughs observed in related work in human-computer interaction, where laughs with robots have mean durations ranging from 0.99 to 1.88 s (e.g., Tahon and Devillers, 2015). Laughs were also classified into three categories depending on their voicing and co-occurrence with speech. We found that unvoiced laughter was the most common, followed by voiced laughter, followed by laughs overlapping with speech.
We predicted that the voicing and length of a laugh would indicate its valence, and that voiced, longer laughter would be perceived as positive by external listeners, paralleling work in human-human interaction where longer and voiced laughter is highly likely to be perceived as positive and shorter unvoiced laughter is highly likely to be perceived as negative (Devillers and Vidrascu, 2007; Lavan et al., 2016; Wood et al., 2017). This might indicate that the majority of laughs in the present study correspond to negative qualities, though our perceptual study does not find that listeners associated voicing or duration with positivity or negativity within the pool of laughs they were exposed to.
Regardless, it would appear that participants tend to produce short, unvoiced laughter when the socialbot says something inappropriate during conversation, which could signal its limitations in human-like communicative fluency. A similar observation was observed in Mazzocconi (2022), who found that mothers sometimes laugh when their children produce similar social incongruities in conversation, using this laughter to provide reassurance and smooth over any discomfort on behalf of the child. Mazzocconi and Ginzburg (2022) find that the overall average duration for mothers’ laughter is 1.65 s (SD = 1.01), which is longer than the average laughter duration in this study (0.79 s, SD = 0.58). While speculative, a possibility is that the short and unvoiced laughter seen in this study indicates a similar, but weaker desire by the user to assuage negative social feelings of the machine, compared to human interaction.
4.4 Perception of laughs
In the perception experiment, we found that external listeners did not rate different types of laughs—voiceless, voiced, and co-speech laughs, as well as laughs of varying durations—as having different valence, arousal, or authenticity scores. This is in contrast to related work in human-human interaction, where acoustic properties of laughs shape their ratings (e.g., Szameitat et al., 2011; Wood et al., 2017). Here, one limitation is that the ratings were performed on laughs presented in isolation. Laughter is highly dependent on context to be understood (Rychlowska et al., 2022), and it is difficult to distinguish between different types of laughter that are presented in isolation, especially without visual cues that aid laughter audibility (Jordan and Abedipour, 2010). At the same time, we found that when listeners rated a laugh as sounding more positive, they also rated it as sounding less fake and more excited, implying that there may be a link between the perceived valence, arousal, and authenticity of laughter, a finding which is consistent with prior studies (Szameitat et al., 2022).
4.5 Implications for theories of human-computer interaction accounts
In the current study, we observe that scenarios that most commonly prompt laughter in human-socialbot interaction parallel those in human-human interaction: after discourse atypicalities, which are also observed in mother–child interactions (Mazzocconi, 2022). This finding supports technology equivalence theories (Nass et al., 1997; Nass and Lee, 2001), wherein people apply social scripts from human-human interactions to technology.
At the same time, we observe very low rates of laughter in the current study than observed in human-human interaction (Vettin and Todt, 2004; Fuchs and Rathcke, 2018; Mazzocconi et al., 2020), as well as relatively short and unvoiced laughter. One interpretation of this difference from human-human interaction is that it reflects a routinized interaction (Gambino et al., 2020): participants have different expectations for humans and machine interlocutors, specifically that the machine will not laugh back. While, at the time of this study, Alexa does not produce laughter, it is an open question whether users are consciously aware of this fact and if they utilize that information during communication. In theory, users who frequently chat with Alexa will know from prior experience that she does not laugh, and they may also believe that Alexa will not respond if they laugh, perhaps producing less salient laughs (short, unvoiced laughter) as a result. Indeed, the only instances in which Alexa responded to a laugh in our dataset took place directly after she told a joke (“Is that laughter I hear?”). Otherwise, Alexa did not explicitly comment on any laugh. This expectation is also consistent with related work showing that people rate voice assistants as sounding emotionless and robotic (Siegert and Krüger, 2021; Cohn et al., 2023).
Yet, an alternative explanation for the lower laugh rate is that participants in the current study mirrored the lack of laughter by the socialbot in their own patterns, transferring a behavior from human-human interaction (Palagi et al., 2022; Scott et al., 2022). As previously mentioned, the presence of laughter often prompts further laughter from a listener in human-human interaction (Platow et al., 2005; Palagi et al., 2022; Scott et al., 2022). This lack of laughter could then be indicative of technology equivalence (Nass et al., 1994, 1997). Future work manipulating presence and degree of laughter in the system itself (e.g., verbal laughter in Inoue et al., 2022; typed laughter in Mills et al., 2021) could tease apart these possibilities.
4.6 Differences across gender or extraversion
We also examined gender differences in the production of laughter with Alexa. We do not find evidence to suggest that women and men produce different rates of laughter in these interactions. This is in line with some prior studies in human-human interaction (Martin and Gray, 1996; Smoski and Bachorowski, 2003a,b; Vettin and Todt, 2004). However, we did find that women tended to produce longer laughs than men, when they did laugh. There is room for further experimentation in future work on gender effects on laughter in human-computer interaction.
We also find no evidence to suggest that more extraverted participants laugh differently, in contrast to prior research on laughter and extraversion in human-human (Deckers, 1993) and human-computer interaction (Soury and Devillers, 2014).
4.7 Future directions
Several new avenues for future research can be proposed based on our current findings. First, on the point of whether people laugh more in interactions with devices versus humans, a future experiment could investigate this more thoroughly by placing each participant in separate human and chatbot interactions and comparing whether one context elicits more laughter. Second, we also observed no evidence to suggest there are gender differences in how much men and women produce laughter within human-chatbot discussions; another possible direction is to manipulate the chatbot’s gender. Previous work finds that people tend to laugh more frequently at men’s speech than women’s (Provine, 2001). Whether the perceived masculinity or femininity of a chatbot’s voice and social characteristics affects how much users laugh in response to them has yet to be determined. Another gap in the laughter literature is examining how laughter is realized across nonbinary individuals. The current study only had one nonbinary participant, which was not enough to do a substantive analysis. But, it is a ripe direction for future work to explore how people with different gender and sexual identities, above and beyond the binary, use laughter during conversation.
We also found no effect of participants’ extraversion score on their use of laughter. This is in contrast to our prediction that extraverted participants would laugh more often, in accordance with previous work (Deckers, 1993). However, the revised Eysenck personality questionnaire (Colledani et al., 2018) that we used is just one method of assessing extraversion, and extraversion is only one personality trait out of many that could influence one’s propensity to laugh. A more thorough examination of how personality interacts with laughter could also examine other facets of personality. Another avenue for further research is to investigate how humans’ use of laughter patterns with their ratings of the socialbot’s extraversion, as previous studies indicate that personality cues in computer speech affect users’ evaluations of said computer (Nass and Lee, 2001; Ahmad et al., 2021; Luttikhuis, 2022).
An additional limitation of the present study is that we examine only audio data, as we did not record video of the chatbot conversations. Prior work shows multimodal laugh annotation is more accurate, as facial movements and body posture enhance the identification of laughter (Jordan and Abedipour, 2010; Petridis et al., 2011; Urbain et al., 2013). Utilizing audiovisual data into the laugh annotation process in future work could increase precision of duration measures, as well as probe multimodal emotional expressiveness.
While participants engaged the system themselves from home using their own device, one limitation in the present study is that we did not control which of the socialbots from the Amazon Alexa Prize competition the participants would talk to in Experiment 1. It is possible that some of the socialbots may have more fluent, human-like speech than others, which could contain less of the conversational oddities outlined in the general discussion. Some socialbots might also produce more machine errors than others. These individual differences could affect the participants’ use of laughter across different socialbots. Future work explicitly manipulating the anthropomorphic features of the system and errors it produces can further tease apart these possible contributions.
Many of the quirks present in the chats from this study arise from Alexa’s unique chatbot behaviors, such as her lack of self-produced laughter or reactions to the user’s laughter. In order to further examine this, one could manipulate Alexa’s behaviors to be more human-like. For example, if Alexa commented on the users’ laughter more often, would their rate of laughing increase, decrease, or remain unchanged? Will we find that people exhibit different patterns of laughter if we allow Alexa to laugh alongside them, paralleling other advancements in laughter detection and generation (e.g., Urbain et al., 2010)? Would participants still laugh at social errors generated by a human speech partner who might be emotionally affected by their laughing behaviors? Dynamics that relate to in- and out-group social categorization become increasingly complex when an out-group member is not human. A controlled and experimental comparison of reactions to human and socialbot laughter is needed to further explore these possibilities.
5 Conclusion
In conclusion, our findings contribute to the scientific understanding of human and socialbot interaction, as well as the linguistic patterning of laughter. We find that speakers do laugh in interactions with an Alexa chatbot, utilizing a behavior seen in human-human interaction– however, laughter is overall observed less frequently in this corpus than in human-human laughter studies. While the strategies of laughter use are similar to that seen in human-human communication, we find that laughter may be used as an appraisal of incongruity between a participant’s expectation of Alexa’s speech and her actual behavior. Overall, this work shows that laughter can serve as a lens for the social dynamics at play with human interactions with technology, which has broad implications for theories of human-computer interaction and advancements across fields (e.g., education, healthcare).
Data availability statement
The raw data supporting the conclusions of this article will be made available by the authors, without undue reservation.
Ethics statement
The studies involving humans were approved by UC Davis Institutional Review Board. The studies were conducted in accordance with the local legislation and institutional requirements. The participants provided their written informed consent to participate in this study.
Author contributions
NP: Conceptualization, Data curation, Formal analysis, Writing – original draft. MC: Conceptualization, Writing – review & editing. GZ: Conceptualization, Funding acquisition, Supervision, Writing – review & editing.
Funding
The author(s) declare that financial support was received for the research, authorship, and/or publication of this article. This material was based upon work supported by the Amazon Faculty Research Award to GZ.
Acknowledgments
We thank Ashley Keaton for transcription correction.
Conflict of interest
The authors declare that the research was conducted in the absence of any commercial or financial relationships that could be construed as a potential conflict of interest.
Publisher’s note
All claims expressed in this article are solely those of the authors and do not necessarily represent those of their affiliated organizations, or those of the publisher, the editors and the reviewers. Any product that may be evaluated in this article, or claim that may be made by its manufacturer, is not guaranteed or endorsed by the publisher.
Supplementary material
The Supplementary material for this article can be found online at: https://www.frontiersin.org/articles/10.3389/fcomm.2024.1346738/full#supplementary-material
Footnotes
3. ^Retained model: Duration ~ Context * Gender.
4. ^We also explored a model that included the data from all participants, including those who did not laugh (coded as “0” for laughter count). The effect structure of this model was identical to the model reported here. This model showed no effect of extraversion (p = 0.83), satisfaction rating (p = 0.34), creepy/eerie-ness ratings (p = 0.89), or human-like ratings (p = 0.06) on the number of laughs a participant produced.
5. ^Retained authenticity and arousal models: value ~ Laugh Type + Duration + (1 | Item) + (1 + Laugh Type | Listener); Retained valence model: value ~ Laugh Type + Duration + (1 | Item) + (1 | Listener).
6. ^Retained model: Valence ~ Authenticity * Arousal + (1 | Item) + (1 | Listener).
References
Adelswärd, V. (1989). Laughter and dialogue: the social significance of laughter in institutional discourse. Nord. J. Linguist. 12, 107–136. doi: 10.1017/S0332586500002018
Ahmad, R., Siemon, D., and Robra-Bissantz, S. (2021). Communicating with machines: conversational agents with personality and the role of extraversion. Proceedings of the 54th Hawaii International Conference on System Sciences. Honolulu, HI: HICSS, 4043–4052.
Ammari, T., Kaye, J., Tsai, J. Y., and Bentley, F. (2019). Music, search, and IoT: how people (really) use voice assistants. ACM Trans. Comp. Hum. Interact. 26, 1–28. doi: 10.1145/3311956
Bachorowski, J. A., and Owren, M. J. (2001). Not all laughs are alike: voiced but not unvoiced laughter readily elicits positive affect. Psychol. Sci. 12, 252–257. doi: 10.1111/1467-9280.00346
Bachorowski, J. A., Smoski, M. J., and Owren, M. J. (2001). The acoustic features of human laughter. J. Acoust. Soc. Am. 110, 1581–1597. doi: 10.1121/1.1391244
Bates, D., Mächler, M., Bolker, B., and Walker, S. (2015). Fitting linear mixed-effects models using lme4. J. Stat. Softw. 67, 1–48. doi: 10.18637/jss.v067.i01
Beach, W. A., and Prickett, E. (2017). Laughter, humor, and cancer: delicate moments and poignant interactional circumstances. Health Commun. 32, 791–802. doi: 10.1080/10410236.2016.1172291
Berge, M. (2017). The role of humor in learning physics: a study of undergraduate students. Res. Sci. Educ. 47, 427–450. doi: 10.1007/s11165-015-9508-4
Bergson, H. (1900). Laughter: an essay on the meaning of the comic (Le rire, essai Sur la signification du Comique). Paris: Presses Universitaires de France.
Boersma, P., and Weenink, D. (2023). Praat: doing phonetics by computer [computer program]. Version 6.4. Available at: http://www.praat.org/ (Accessed November 15, 2023).
Bryant, G. A., and Bainbridge, C. M. (2022). Laughter and culture. Philos. Trans. R. Soc. B 377:20210179. doi: 10.1098/rstb.2021.0179
Chapman, A. J. (1973). Funniness of jokes, canned laughter and recall performance. Sociometry 36, 569–578. doi: 10.2307/2786252
Chastagnol, C., and Devillers, L. (2012). “Collecting spontaneous emotional data for a social assistive robot” in Workshop Programme LREC, vol. 12. (Istanbul, Turkey: ELRA), 62.
Cohn, M., Keaton, A., Beskow, J., and Zellou, G. (2023). Vocal accommodation to technology: the role of physical form. Lang. Sci. 99:101567. doi: 10.1016/j.langsci.2023.101567
Cohn, M., Liang, K. H., Sarian, M., Zellou, G., and Yu, Z. (2021). Speech rate adjustments in conversations with an Amazon Alexa socialbot. Front. Commun. 6:671429. doi: 10.3389/fcomm.2021.671429
Cohn, M., Segedin, B. F., and Zellou, G. (2022). Acoustic-phonetic properties of Siri-and human-directed speech. J. Phon. 90:101123. doi: 10.1016/j.wocn.2021.101123
Cohn, M., and Zellou, G. (2019). “Expressiveness influences human vocal alignment toward voice-AI” in Proceedings of Interspeech. (Graz, Austria: ISCA), 41–45.
Cohn, M., and Zellou, G. (2021). Prosodic differences in human-and Alexa-directed speech, but similar local intelligibility adjustments. Front. Commun. 6:675704. doi: 10.3389/fcomm.2021.675704
Colledani, D., Anselmi, P., and Robusto, E. (2018). Using item response theory for the development of a new short form of the Eysenck personality questionnaire-revised. Front. Psychol. 9:1834. doi: 10.3389/fpsyg.2018.01834
Cosentino, S., Kishi, T., Zecca, M., Sessa, S., Bartolomeo, L., Hashimoto, K., et al. (2013). “Human-humanoid robot social interaction: laughter” in 2013 IEEE International Conference on Robotics and Biomimetics (ROBIO) (Shenzhen, China: IEEE), 1396–1401.
Cowan, B. R., Branigan, H. P., Obregón, M., Bugis, E., and Beale, R. (2015). Voice anthropomorphism, interlocutor modelling and alignment effects on syntactic choices in human− computer dialogue. Int. J. Hum. Comput. Stud. 83, 27–42. doi: 10.1016/j.ijhcs.2015.05.008
Curran, W., McKeown, G. J., Rychlowska, M., André, E., Wagner, J., and Lingenfelser, F. (2018). Social context disambiguates the interpretation of laughter. Front. Psychol. 8:2342. doi: 10.3389/fpsyg.2017.02342
Deckers, L. (1993). Do extraverts “like to laugh”? An analysis of the situational humor response questionnaire (SHRO). Eur. J. Personal. 7, 211–220. doi: 10.1002/per.2410070402
Devillers, L., and Vidrascu, L. (2007). “Positive and negative emotional states behind the laughs in spontaneous spoken dialogs” in Interdisciplinary workshop on the phonetics of laughter. Saarbruken, Germany: Saarland University Press), 37–40.
Fuchs, S., and Rathcke, T. (2018). “Laugh is in the air? Physiological analysis of laughter as a correlate of attraction during speed dating” in Proceedings of laughter workshop 2018. eds. J. Ginzburg and C. Pelachaud, (Paris, France: Sorbonne University Press), 21–24.
Gambino, A., Fox, J., and Ratan, R. A. (2020). Building a stronger CASA: extending the computers are social actors paradigm. Hum. Mach. Commun. 1, 71–86. doi: 10.30658/hmc.1.5
Gillick, J., Deng, W., Ryokai, K., and Bamman, D. (2021). “Robust laughter detection in Noisy environments” in Proceedings of Interspeech, 8, Brno, Czechia: ISCA.
Ginzburg, J., Mazzocconi, C., and Tian, Y. (2020). Laughter as language. Glossa 5, 1–51. doi: 10.5334/gjgl.1152
Glenn, P. (2010). Interviewer laughs: shared laughter and asymmetries in employment interviews. J. Pragmat. 42, 1485–1498. doi: 10.1016/j.pragma.2010.01.009
González-Fuente, S., Escandell-Vidal, V., and Prieto, P. (2015). Gestural codas pave the way to the understanding of verbal irony. J. Pragmat. 90, 26–47. doi: 10.1016/j.pragma.2015.10.002
Grammer, K. (1990). Strangers meet: laughter and nonverbal signs of interest in opposite-sex encounters. J. Nonverbal Behav. 14, 209–236. doi: 10.1007/BF00989317
Habler, F., Schwind, V., and Henze, N. (2019). “Effects of smart virtual assistants' gender and language” in Proceedings of mensch und computer, (New York: ACM), 469–473.
Ho, A., Hancock, J., and Miner, A. S. (2018). Psychological, relational, and emotional effects of self-disclosure after conversations with a chatbot. J. Commun. 68, 712–733. doi: 10.1093/joc/jqy026
Holt, E. (2011). On the nature of “laughables” laughter as a response to overdone figurative phrases. Quart. Publ. Int. Pragm. Assoc. 21, 393–410. doi: 10.1075/prag.21.3.05hol
Howarth, W. D. (1999). “Bergson revisited: Le Rire a hundred years on” in French humour. (Ed.) J. Parkin (Amsterdam, Netherlands: Rodopi), 139–156.
Inoue, K., Lala, D., and Kawahara, T. (2022). Can a robot laugh with you?: shared laughter generation for empathetic spoken dialogue. Front. Robot. AI 9:933261. doi: 10.3389/frobt.2022.933261
Jefferson, G. (1984). “On the organization of laughter in talk about troubles” in Structures of social action: studies in conversation analysis, vol. 346, (Cambridge: Cambridge University Press), 346–369.
Jefferson, G. (2004). A note on laughter in ‘male–female’ interaction. Discourse Stud. 6, 117–133. doi: 10.1177/1461445604039445
Jefferson, G., Harvey, S., and Schegloff, E. (1977). “Preliminary notes on the sequential organization of laughter” in Pragmatics microfiche, vol. 1, (Cambridge: Cambridge University, Department of Linguistics), A2eD9.
Johnson, D., and Gardner, J. (2007). The media equation and team formation: further evidence for experience as a moderator. Int. J. Hum. Comput. Stud. 65, 111–124. doi: 10.1016/j.ijhcs.2006.08.007
Jordan, T. R., and Abedipour, L. (2010). The importance of laughing in your face: influences of visual laughter on auditory laughter perception. Perception 39, 1283–1285. doi: 10.1068/p6752
Kim, S., Garlapati, A., Lubin, J., Tamrakar, A., and Divakaran, A. (2021). Towards understanding confusion and affective states under communication failures in voice-based human-machine interaction. In 2021 9th International Conference on Affective Computing and Intelligent Interaction Workshops and Demos (ACIIW), (Nara, Japan: IEEE), 1–5.
Kohler, K. J. (2008). ‘Speech-smile’, ‘speech-laugh’, ‘laughter’ and their sequencing in dialogic interaction. Phonetica 65, 1–18. doi: 10.1159/000130013
Kuznetsova, A., Brockhoff, P. B., and Christensen, R. H. B. (2017). Lmer test package: tests in linear mixed effects models. J. Stat. Softw. 82, 1–26. doi: 10.18637/jss.v082.i13
Lavan, N., Scott, S. K., and McGettigan, C. (2016). Laugh like you mean it: authenticity modulates acoustic, physiological and perceptual properties of laughter. J. Nonverbal Behav. 40, 133–149. doi: 10.1007/s10919-015-0222-8
Lawson, T. J., Downing, B., and Cetola, H. (1998). An attributional explanation for the effect of audience laughter on perceived funniness. Basic Appl. Soc. Psychol. 20, 243–249. doi: 10.1207/s15324834basp2004_1
Lüdecke, D., Ben-Shachar, M. S., Patil, I., Waggoner, P., and Makowski, D. (2021). Performance: an R package for assessment, comparison and testing of statistical models. Jo. Open Source Softw. 6:3139. doi: 10.21105/joss.03139
Luttikhuis, O. (2022). Investigating the influence of a matching personality between user and chatbot on the user satisfaction of chatbot interactions. Njimegen, Netherlands: Radboud University Radboud Educational Repository.
Maraev, V., Bernardy, J. P., and Howes, C. (2021). Non-humorous use of laughter in spoken dialogue systems. Linguist. Cogn. Appr. Dialog. Agents. 2935, 33–44.
Martin, G. N., and Gray, C. D. (1996). The effects of audience laughter on men's and women's responses to humor. J. Soc. Psychol. 136, 221–231. doi: 10.1080/00224545.1996.9713996
Mascaró, M., Serón, F. J., Perales, F. J., Varona, J., and Mas, R. (2021). Laughter and smiling facial expression modelling for the generation of virtual affective behavior. PLoS One 16:e0251057. doi: 10.1371/journal.pone.0251057
Mayer, A. V., Paulus, F. M., and Krach, S. (2021). A psychological perspective on vicarious embarrassment and shame in the context of cringe humor. Humanities 10, 110.1–110.15. doi: 10.3390/h10040110
Mazzocconi, C. (2022). “Laughter meaning construction and use in development: children and spoken dialogue systems” in International conference on human-computer interaction (Cham: Springer Nature Switzerland), 113–133.
Mazzocconi, C., and Ginzburg, J. (2022). A longitudinal characterization of typical laughter development in Mother–child interaction from 12 to 36 months: Formal features and reciprocal responsiveness. Journal of Nonverbal Behavior, 46, 327–362. doi: 10.1007/s10919-022-00403-8
Mazzocconi, C., and Ginzburg, J. (2023). Growing up laughing: Laughables and pragmatic functions between 12 and 36 months. J. Pragmat. 212, 117–145. doi: 10.1016/j.pragma.2023.04.015
Mazzocconi, C., Maraev, V., Somashekarappa, V., and Howes, C. (2021). “Looking for laughs: gaze interaction with laughter pragmatics and coordination” in Proceedings of the 2021 International Conference on Multimodal Interaction, (New York: ACM), 636–644.
Mazzocconi, C., Tian, Y., and Ginzburg, J. (2020). What’s your laughter doing there? A taxonomy of the pragmatic functions of laughter. IEEE Trans. Affect. Comput. 13, 1302–1321. doi: 10.1109/TAFFC.2020.2994533
McLachlan, A. (2022). The relationship between familiarity, gender, disagreement, and status and bouts of solitary and joint laughter. Curr. Psychol. 42, 25730–25744. doi: 10.1007/s12144-022-03671-1
Mehu, M., and Dunbar, R. (2008). Naturalistic observations of smiling and laughter in human group interactions. Behaviour 145, 1747–1780. doi: 10.1163/156853908786279619
Mills, G., Gregoromichelaki, E., Howes, C., and Maraev, V. (2021). Influencing laughter with AI-mediated communication. Interact. Stud. 22, 416–463. doi: 10.1075/is.00011.mil
Nass, C., and Lee, K. M. (2001). Does computer-synthesized speech manifest personality? Experimental tests of recognition, similarity-attraction, and consistency-attraction. J. Exp. Psychol. Appl. 7, 171–181. doi: 10.1037/1076-898X.7.3.171
Nass, C., Moon, Y., and Green, N. (1997). Are machines gender neutral? Gender-stereotypic responses to computers with voices. J. Appl. Soc. Psychol. 27, 864–876. doi: 10.1111/j.1559-1816.1997.tb00275.x
Nass, C., Steuer, J., and Tauber, E. R. (1994). “Computers are social actors” in Proceedings of the SIGCHI Conference on Human Factors in Computing Systems. (Boston, MA: ACM), 72–78.
OpenAI . (2022). Introducing chat GPT. Open AI. Available at: https://openai.com/blog/chatgpt (Accessed March 10, 2023).
Osvaldsson, K. (2004). On laughter and disagreement in multiparty assessment talk. Text Talk 24, 517–545. doi: 10.1515/text.2004.24.4.517
Palagi, E., Caruana, F., and de Waal, F. B. (2022). The naturalistic approach to laughter in humans and other animals: towards a unified theory. Philos. Trans. R. Soc. B 377:20210175. doi: 10.1098/rstb.2021.0175
Petridis, S., Leveque, M., and Pantic, M. (2013b). Audiovisual detection of laughter in human-machine interaction. In 2013 Humaine Association Conference on Affective Computing and Intelligent Interaction, IEEE, 129–134.
Petridis, S., Martinez, B., and Pantic, M. (2013a). Audiovisual detection of laughter in human-machine interaction. In 2013 Humaine Association Conference on Affective Computing and Intelligent Interaction. (Geneva, Switzerland: IEEE), 129–134.
Petridis, S., Pantic, M., and Cohn, J. F. (2011). Prediction-based classification for audiovisual discrimination between laughter and speech. In 2011 IEEE International Conference on Automatic Face & Gesture Recognition (FG), (Santa Barbara, CA: IEEE), 619–626.
Platow, M. J., Haslam, S. A., Both, A., Chew, I., Cuddon, M., Goharpey, N., et al. (2005). “It’s not funny if they’re laughing”: self-categorization, social influence, and responses to canned laughter. J. Exp. Soc. Psychol. 41, 542–550. doi: 10.1016/j.jesp.2004.09.005
Provine, R. R. (1993). Laughter punctuates speech: linguistic, social and gender contexts of laughter. Ethology 95, 291–298. doi: 10.1111/j.1439-0310.1993.tb00478.x
Proyer, R. T., Meier, L. E., Platt, T., and Ruch, W. (2013). Dealing with laughter and ridicule in adolescence: relations with bullying and emotional responses. Soc. Psychol. Educ. 16, 399–420. doi: 10.1007/s11218-013-9221-y
Ram, A., Prasad, R., Khatri, C., Venkatesh, A., Gabriel, R., Liu, Q., et al. (2018). Conversational AI: the science behind the Alexa prize. ArXiv. doi: 10.48550/arXiv.1801.03604
Rathcke, T., and Fuchs, S. (2022). Laugh is in the air: an exploratory analysis of laughter during speed dating. Front. Commun. 7, 1–16. doi: 10.3389/fcomm.2022.909913
Reed, L. I., and Castro, E. (2021). Are you laughing at them or with them? Laughter as a signal of in-group affiliation. J. Nonverbal Behav. 46, 71–82. doi: 10.1007/s10919-021-00384-0
Rieger, C. L. (2009). Comparing same-gender and opposite-gender conversations: a laughing matter?. In Gender and Laughter, (Leiden, Netherlands: Brill), 355–370.
Rychlowska, M., McKeown, G. J., Sneddon, I., and Curran, W. (2022). The role of contextual information in classifying spontaneous social laughter. J. Nonverbal Behav. 46, 449–466. doi: 10.1007/s10919-022-00412-7
Sauter, D. A., Eisner, F., Ekman, P., and Scott, S. K. (2010). Cross-cultural recognition of basic emotions through nonverbal emotional vocalizations. Proc. Natl. Acad. Sci. U. S. A. 107, 2408–2412. doi: 10.1073/pnas.0908239106
Scott, S. K., Cai, C. Q., and Billing, A. (2022). Robert provine: the critical human importance of laughter, connections and contagion. Philos. Trans. R. Soc. B 377:20210178. doi: 10.1098/rstb.2021.0178
Siegert, I., Busch, M., and Krüger, J. (2020). “Does users’ system evaluation influence speech behavior in HCI?–first insights from the engineering and psychological perspective” in Konferenz Elektronische Sprachsignalverarbeitung (Dresden: TUDpress), 241–248.
Siegert, I., and Krüger, J. (2021). ““Speech melody and speech content Didn’t fit together”—differences in speech behavior for device directed and human directed interactions” in Advances in data science: methodologies and applications (Cham: Springer International Publishing), 65–95.
Smoski, M., and Bachorowski, J. A. (2003a). Antiphonal laughter between friends and strangers. Cognit. Emot. 17, 327–340. doi: 10.1080/02699930302296
Smoski, M., and Bachorowski, J. A. (2003b). Antiphonal laughter in developing friendships. Ann. N. Y. Acad. Sci. 1000, 300–303. doi: 10.1196/annals.1280.030
Soury, M., and Devillers, L. (2014). Smile and Laughter in Human-Machine Interaction: a study of engagement In Proceedings of the Language Resources and Evaluation Conference (Reykjavik, Iceland: LREC), 3633–3637.
Szameitat, D. P., Darwin, C. J., Wildgruber, D., Alter, K., and Szameitat, A. J. (2011). Acoustic correlates of emotional dimensions in laughter: arousal, dominance, and valence. Cognit. Emot. 25, 599–611. doi: 10.1080/02699931.2010.508624
Szameitat, D. P., Szameitat, A. J., and Wildgruber, D. (2022). Vocal expression of affective states in spontaneous laughter reveals the bright and the dark side of laughter. Sci. Rep. 12, 1–12. doi: 10.1038/s41598-022-09416-1
Tahon, M., and Devillers, L. (2015). “Laughter detection for on-line human-robot interaction” in Interdisciplinary Workshop on Laughter and Non-verbal Vocalisations in Speech. Enschede, Netherlands: University of Twente Press), 35–37.
Tanaka, H., and Campbell, N. (2014). Classification of social laughter in natural conversational speech. Comput. Speech Lang. 28, 314–325. doi: 10.1016/j.csl.2013.07.004
Tian, Y., Mazzocconi, C., and Ginzburg, J. (2016). “When do we laugh?” in Proceedings of the 17th Annual Meeting of the Special Interest Group on Discourse and Dialogue. (Los Angeles, CA: ACL), 360–369.
Urbain, J., Niewiadomski, R., Bevacqua, E., Dutoit, T., Moinet, A., Pelachaud, C., et al. (2010). AVLaughterCycle: enabling a virtual agent to join in laughing with a conversational partner using a similarity-driven audiovisual laughter animation. J. Multim. User Interfaces 4, 47–58. doi: 10.1007/s12193-010-0053-1
Urbain, J., Niewiadomski, R., Mancini, M., Griffin, H., Cakmak, H., Ach, L., et al. (2013). “Multimodal analysis of laughter for an interactive system” in Intelligent Technologies for Interactive Entertainment: 5th International ICST Conference, INTETAIN 2013, Mons, Belgium, July 3–5, 2013, revised selected papers (New York, NY: Springer International Publishing), 183–192.
Vettin, J., and Todt, D. (2004). Laughter in conversation: features of occurrence and acoustic structure. J. Nonverbal Behav. 28, 93–115. doi: 10.1023/B:JONB.0000023654.73558.72
Weber, K., Ritschel, H., Lingenfelser, F., and André, E. (2018). “Real-time adaptation of a robotic joke teller based on human social signals” in AAMAS '18 Proceedings of the 17th International Conference on Autonomous Agents and Multi Agent Systems. eds. E. André, S. Koenig, M. Dastani, and G. Sukthankar, (New York, NY: ACM), 2259–2261.
Wood, A. (2020). Social context influences the acoustic properties of laughter. Affect. Sci. 1, 247–256. doi: 10.1007/s42761-020-00022-w
Wood, A., Martin, J., and Niedenthal, P. (2017). Towards a social functional account of laughter: acoustic features convey reward, affiliation, and dominance. PLoS One 12:e0183811. doi: 10.1371/journal.pone.0183811
Wood, A., and Niedenthal, P. (2018). Developing a social functional account of laughter. Soc. Personal. Psychol. Compass 12:e12383. doi: 10.1111/spc3.12383
Wood, A., Templeton, E., Morrel, J., Schubert, F., and Wheatley, T. (2022). Tendency to laugh is a stable trait: findings from a round-robin conversation study. Philos. Trans. R. Soc. B 377:20210187. doi: 10.1098/rstb.2021.0187
Zellou, G., Cohn, M., and Ferenc Segedin, B. (2021). Age-and gender-related differences in speech alignment toward humans and voice-AI. Front. Commun. 5:600361. doi: 10.3389/fcomm.2020.600361
Keywords: laughter, conversation analysis, human-computer interaction, production, perception
Citation: Perkins Booker N, Cohn M and Zellou G (2024) Linguistic patterning of laughter in human-socialbot interactions. Front. Commun. 9:1346738. doi: 10.3389/fcomm.2024.1346738
Edited by:
Antonio Benítez-Burraco, University of Seville, SpainReviewed by:
Tilda Neuberger, Hungarian Academy of Sciences, HungaryJuan Carlos Farah, École Polytechnique Fédérale de Lausanne, Switzerland
Chiara Mazzocconi, Aix-Marseille Université, France
Copyright © 2024 Perkins Booker, Cohn and Zellou. This is an open-access article distributed under the terms of the Creative Commons Attribution License (CC BY). The use, distribution or reproduction in other forums is permitted, provided the original author(s) and the copyright owner(s) are credited and that the original publication in this journal is cited, in accordance with accepted academic practice. No use, distribution or reproduction is permitted which does not comply with these terms.
*Correspondence: Nynaeve Perkins Booker, bmJvb2tAdWNkYXZpcy5lZHU=