- 1Department of Marketing and Interdisciplinary Business, School of Business, The College of New Jersey, Ewing, NJ, United States
- 2MediaScience, Austin, TX, United States
- 3Ehrenberg-Bass Institute, Business School, University of South Australia, Adelaide, SA, Australia
Introduction: Synced ads differ from other forms of targeted advertising on mobile devices because they target concurrent media usage rather than location or predicted interest in the brand. For example, a TV-viewer’s smartphone could listen to the ads playing on the TV set and show matching social media ads. These social media ads could be timed to appear simultaneously with the TV ad, or shortly before or after.
Methods: This research reports a meta-analysis (N = 980) of four lab studies that used representative samples of consumers and realistic manipulations of synced ads. These studies contrasted with most previous studies of synced ads, which have used student samples and unrealistic manipulations or imagined scenarios, which means little is known about whether or why synced ads are effective in real life. These four studies manipulated the effects of synced-ad timing (simultaneous vs. sequential before or after) and the size of the mobile ad, to see if these moderate the effects of synced ads.
Results: The results showed that synced ads were more effective, measured by unaided brand recall, when they were shown after the TV commercial, rather than simultaneously. Ad size had no moderating effect, which suggests that normal ads can be used, rather than the full-screen or pop-up ads used in previous studies. A final study, in which ad timing was user-controlled, rather than advertiser-controlled, showed that precise timing is not important for synced-ad effectiveness.
Discussion: These results suggest the effects of synced ads are best explained by repetition rather than synergy between the two exposures. There were no significant effects on brand attitude, ad liking, or purchase intention. These results have implications for theoretical models of synced-ad effectiveness, and for advertisers planning to use synced ads.
1 Introduction
Mobile advertising attracts 40% of advertising expenditure worldwide (Statista, 2023a). In the United States, over two-thirds of people use a mobile phone while watching television (Statista, 2023b). Media multitasking divides attention (Brasel and Gips, 2017), reducing TV advertising effectiveness (Segijn and Eisend, 2019). Synchronized (“synced”) ads, which are matching ads seen on the TV and the mobile phone, were proposed as a remedy for the negative effects of multitasking. Synced ads are also interesting because they are targeted differently compared to other forms of mobile advertising (Boerman et al., 2017). Instead of being targeted by location or likelihood of responding (Bleier and Eisenbeiss, 2015), synced ads are targeted based on concurrent media usage (Segijn, 2019). For example, a social media app on a mobile phone could display synced ads by listening for digital watermarks in TV commercials (Segijn et al., 2021). This requires Automated Content Recognition (ACR) technology, pioneered by Shazam and Gracenote, and exploiting how mobile phones often have location tracking (Collins and Gordon, 2022) and their microphones turned on and collecting data by default (Strycharz and Segijn, 2024). Advertisers are told this rise in dataveillance (Clarke, 1988) will help to deliver more interactive, targeted, and personalized advertising (Main, 2017), but it also threatens to have a chilling effect on people’s behavior, reducing their autonomy to watch whatever they like (Büchi et al., 2022; Strycharz and Segijn, 2024). For these reasons, consumers have strong privacy concerns about synced ads (Boerman and Segijn, 2022), so it is important to understand whether there is a business case for using them.
Currently, the evidence for the effectiveness of synced ads is mixed. An early case study reported a 96% lift in brand awareness when TV commercials were paired with synced ads (Duckler, 2016). Since that time, academic studies have tried to reproduce these commercial results under more controlled conditions (Garaus et al., 2017; Hoeck and Spann, 2020; Segijn et al., 2021; Segijn and Voorveld, 2021; Lee et al., 2023). When these studies have found positive results (e.g., a lift in brand recall versus multitasking), alternative explanations might explain these results. For example, the experiment may have used an unrealistic manipulation of synced ads (e.g., the participants read scenarios rather than experiencing synced ads [Abdollahi et al., 2023]). Or the synced ads may have been unrealistically large (e.g., occupying the entire mobile phone screen), so their effects could be due to salience, rather than the simultaneous timing unique to synced ads (Hoeck and Spann, 2020). Other findings suggest it may not be necessary to time synced ads so they appear simultaneously with the TV ad they are matched with (Segijn et al., 2021). Our research identifies and tests two potential moderating factors, ad size, and timing, that may influence the effectiveness of synced ads.
This research reports a meta-analysis of four studies (from nearly 1,000 participants) that manipulated these moderators, synced ad size and timing, using realistic manipulations of multitasking and TV clutter. In each study, participants in the synced ad condition watched an entire TV program, with ads, on a large computer screen mimicking a flat-screen TV, while also multitasking with program-related interactive content on a smaller tablet screen. These long programs created 15 min of forgetting time to ensure we measured effects on long-term memory rather than just short-term working memory (Eysenck, 1976; Trifilio et al., 2020). Studies 1 to 3 varied the size and salience (i.e., the intrusiveness) of synced ads, and varied their timing, showing them either simultaneously with their matching TV ad, or shortly before or after the TV ad. Study 4 tested whether privacy infringing dataveillance is necessary for the positive effects of synced ads, by showing imprecisely timed synced ads on the tablet screen. The studies all used representative samples of United States consumers, so that our results would apply generally, and not just to students (Segijn and Eisend, 2019). Furthermore, to ensure that our results applied to a wide range of advertised products and brands, we tested synced ads for 10 real brands from a range of categories with low, medium, and high product involvement.
The results show that not all synced ads are the same, and it is important to account for study realism, ad size, and ad timing when comparing the results of synced ad studies. First, these realistic studies clarify the mixed results in the previous literature by showing how differences in ad size and ad timing contribute to synced-ad effectiveness. Second, these results have theoretical and practical implications for researchers and advertisers. If ad size explains differences in synced-ad effectiveness, that would mean the few synced-ad studies reporting positive results, relative to multitasking, have used unrealistic and highly intrusive full screen synced ads. The effects of synced ads would then be explained by theories of attention-getting (ad salience) in addition to ad timing. It would also mean that advertisers would need to buy expensive intrusive ad formats to see positive effects from synced ads. The effects of ad timing have even greater ramifications for synced-ad theory and practice. If synced-ad effectiveness requires simultaneous timing, this would be explained by new theories of simultaneous ad processing, rather than traditional theories of sequential ad processing. On the other hand, synced ads would be easier and cheaper to implement if they did not have to be precisely timed. Furthermore, if the gap between a synced ad and its matching ad can be longer than a minute, there may be little difference between synced ads and cross-media exposure in a typical multimedia campaign. Typical ads would of course be much cheaper than synced ads and raise none of the privacy concerns associated with the dataveillance required for simultaneous timing. If there is no business case for synced ads versus normal ads, there would be no need for businesses to support the unethical practice of wiretapping consumers without their consent.
2 Literature review
2.1 Previous studies
We begin our survey of the synced-ad effectiveness literature by first defining what we mean by “effectiveness.” Effectiveness measures can be biassed by high base-rates (e.g., false-recall) for familiar brands (Singh and Cole, 1985; Chandon et al., 2022). Advertisers can control for this by using fictitious brands (Geuens and De Pelsmacker, 2017), or by using brand “lift” measures for real brands (Google, 2023). These compare measures of ad-effectiveness (e.g., brand awareness) from two matched groups, one which saw the ad, and an unexposed control sample, which did not see the ad. For synced ads, however, the appropriate comparison is with a multitasking control group, who see the TV ad without a matching synced ad (Voorveld and Viswanathan, 2015). Three prior synced-ad studies report significant lifts in brand awareness compared with a media multitasking (2-screen) control condition (Garaus et al., 2017; and 2 studies by Hoeck and Spann, 2020). In the two studies by Hoeck and Spann (2020), a synced ad generated brand awareness (unaided brand recall and brand recognition) equivalent to a normal (1-screen) TV ad exposure (or better). Questions remain, however, about why these synced ads were effective. If participants paid no attention to the TV ad, then the synced ad would have been the only exposure to the brand’s advertising. The explanation for synced-ad effectiveness would then be they merely act as “make-good” replacements for missed TV exposures, similar to other cross-media “roadblock” ads (Huang and Huh, 2018). Hoeck and Spann (2020) measured self-reported attention to the TV ad, and while it was significantly lower when a synced ad was present, it was still high (over 50% on a 0 to 100% attention scale), and not zero. If participants paid attention to both the TV ad and the synced ad, the effect of the combination might be due not only to repeat exposure (1 + 1 = 2), but potentially to simultaneous-viewing synergy between the two exposures (1 + 1 = 3) (Romaniuk et al., 2013; Schmidt and Eisend, 2015; Segijn, 2019).
Although a meta-analysis found no general effect of multitasking on affective outcomes, such as attitudes and intentions (Segijn and Eisend, 2019), synced ad repetition may also improve ad and brand attitudes, and purchase intention (Segijn, 2019). These attitude-change effects might be explained by theories such as the mere exposure effect (Zajonc, 1980; Eijlers et al., 2020), or the multiple-source effect (Harkins and Petty, 1981; Lee-Won et al., 2020). In one previous study, Segijn and Voorveld (2021) reported a lift in the favorability of brand attitude for synced ads compared to an unexposed control sample. This effect occurred whether the synced ad was seen simultaneously with the TV ad, or sequentially (before or after). But a subsequent study found no effect on brand attitude from showing synced ads for the same brand versus a competing brand (Lee et al., 2023). The case where a synced ad shows a competing brand replicates normal multitasking, where viewers see different content across two screens. The results of this recent study (Lee et al., 2023) suggest synced ads may not improve brand attitude relative to normal multitasking, as opposed to relative to an unexposed control cell. As we argued above, a multitasking control group is the more relevant control group for synced ads.
Surveys of multitasking studies have highlighted the moderating effects of the methods used (Segijn et al., 2018; Segijn and Eisend, 2019). One of these moderating factors is the realism of the task. Multitasking has a more negative effect on cognitive measures when an unrealistic computer task is used to simulate multitasking (e.g., both ads on 1 screen), as opposed to a more ecologically valid task in which participants multitask across two screens (Segijn and Eisend, 2019). Multitasking realism affects the internal and external validity of synced ad studies.
Table 1 shows prior synced ad studies separated by whether the realism of the manipulation was high (2 screens) or low (1 screen). Note that this table excludes scenario studies in which participants were asked to imagine what experiencing a synced ad would be like (e.g., Boerman and Segijn, 2022; Segijn and van Ooijen, 2022; Abdollahi et al., 2023; Sifaoui et al., 2023; Segijn et al., 2023a). It is encouraging that two of the significant positive effects of synced ads were associated with high-realism (2-screen) multitasking manipulations. The positive effect of synced ads in the two low-realism studies may have been due to the exaggerated negative effect of simulated multitasking. However, the evidence from the realistic studies is mixed, because one of them found no significant positive effects of synced ads, and others found no improvement versus multitasking. This suggests more studies of synced ads are needed, using realistic multitasking.
2.2 Ad size
The other two columns in Table 1 identify two further potential moderators of the effects of synced ads. The first of these is ad size, which again is related to the realism of the experiment. The most common ad-types seen on a mobile screen are online banner ads and social media ads. For example, a Facebook feed ad1 can be a horizontal rectangle (16:9), or a square (1:1), occupying 100% of width of the screen, but not 100% of its depth. Meta has recently introduced the Facebook story format, which is a highly intrusive full-screen (i.e., 100% of width and depth) interstitial ad2. The story format is expensive because it grabs more attention than the average Facebook feed ad. Feed ads attract only 1.6 s of dwell time (Ebiquity, 2021). In Table 1, it is concerning that the positive effects of synced ads, compared with realistic multitasking, were associated with large, story-like ads that occupied 100% of the screen (Hoeck and Spann, 2020). Consumers and regulators likely would not appreciate advertisers using highly intrusive full-screen ads, if these are the only effective form of synced ads (Segijn et al., 2023a).
2.3 Ad timing
The third moderating variable highlighted in Table 1 is the timing of the synced ad. Timing is what distinguishes synced ads from normal cross-media advertising, which is also targeted, but does not rely on precisely timed concurrent media exposure (Segijn, 2019). If synced ad effectiveness requires simultaneous timing, then some explanations for synced-ad effects are more likely than others (Segijn et al., 2021). For example, the cross-media effects of forward encoding and image transfer require a separation in time between exposures (Voorveld et al., 2011). However, when 2 ads are viewed simultaneously, this may enhance the cross-media effects of multiple-source perception (Segijn, 2019), and cognitive threading of related content (Salvucci and Taatgen, 2008; Hwang and Jeong, 2021). It is interesting, therefore, that simultaneous versus sequential timing has not affected the positive results for synced ads. Synced ads have had the same effects whether they were shown simultaneously with the primary ad (the classic synced ad) or 15 s before or after (Segijn et al., 2021). This short gap in time may have been enough for participants to consider the two ads as separate and not creepily related, and so sequential timing may have improved attitude toward the synced ad (Segijn, 2019). At even longer intervals (e.g., 30 s either side), the effects of synced ads are more likely to be explained by traditional cross-media effects, rather than unique simultaneous effects. If synced ads are just as effective when not precisely timed, they could be delivered without relying on privacy intruding surveillance of current media usage (Boerman and Segijn, 2022).
The present research contributes to the growing literature on synced ads by investigating whether ad size and timing moderate the effects of synced ads. It meta-analyzes data from four studies that all used representative samples and realistic manipulations of multitasking and TV ad clutter but varied two potential moderators of synced ad effectiveness: ad size and timing. These studies tested multiple brands with varying product category involvement and creative execution (early vs. late branding) to avoid the problem of significant results potentially being associated with the unique effects of a single brand or its TV or synced ad execution. The next sections briefly describe these studies and their results. This is followed by a discussion of the implications of these results for theory and practice.
3 Materials and methods
3.1 Overview of the four studies
All four studies used realistic manipulations of media multitasking. Participants watched a TV program on a large flat screen (replicating watching a large flat-screen TV), while also viewing content on a smaller tablet computer (see Figure 1). In Studies 1 to 3, the same computer program delivered content to both screens, allowing precise timing of the synced ads. Participants were randomly assigned to either (1) a synced ads group that watched the TV program with program-related content and synced ads on the smaller screen, or (2) a single-screen (TV-only) group that saw only the TV program on the large screen, because while the TV program was showing the smaller tablet screen was black (see Figure 2). Within the synced ads group, participants were randomly assigned to one of two ad timing groups: (a) a simultaneous-timing group, in which the synced ads appeared at the same time as their matching TV ad, or (b) a sequential-timing group, in which the synced ads appeared before or after their matching TV ad. The test ads without a matching synced ad on the smaller screen tested the distracting effect of multiscreening (i.e., these studies used a powerful within-subject multitasking comparison control condition). Study 4 tested highly intrusive Twitter (now X) ads (i.e., branded posts), which offer a less-precisely timed and more privacy-protecting alternative to using synced ads (see Figure 2). Although the studies were carried out over 10 years ago, their methods are still similar to the most recent tests of synced ads. For example, in Segijn et al. (2023b), participants watched a 7-min TV show segment on a large flatscreen TV while multitasking with a magazine app on a smaller (portrait-orientation) tablet screen, and at a hard-coded time, while matching content played on the TV, a synced banner ad was superimposed as a second layer over the magazine content (i.e., dataveillance triggering of synced ads was not replicated). The methods used in that 2023 study are very similar to those used in the older studies reported here, suggesting these older studies are still relevant today.
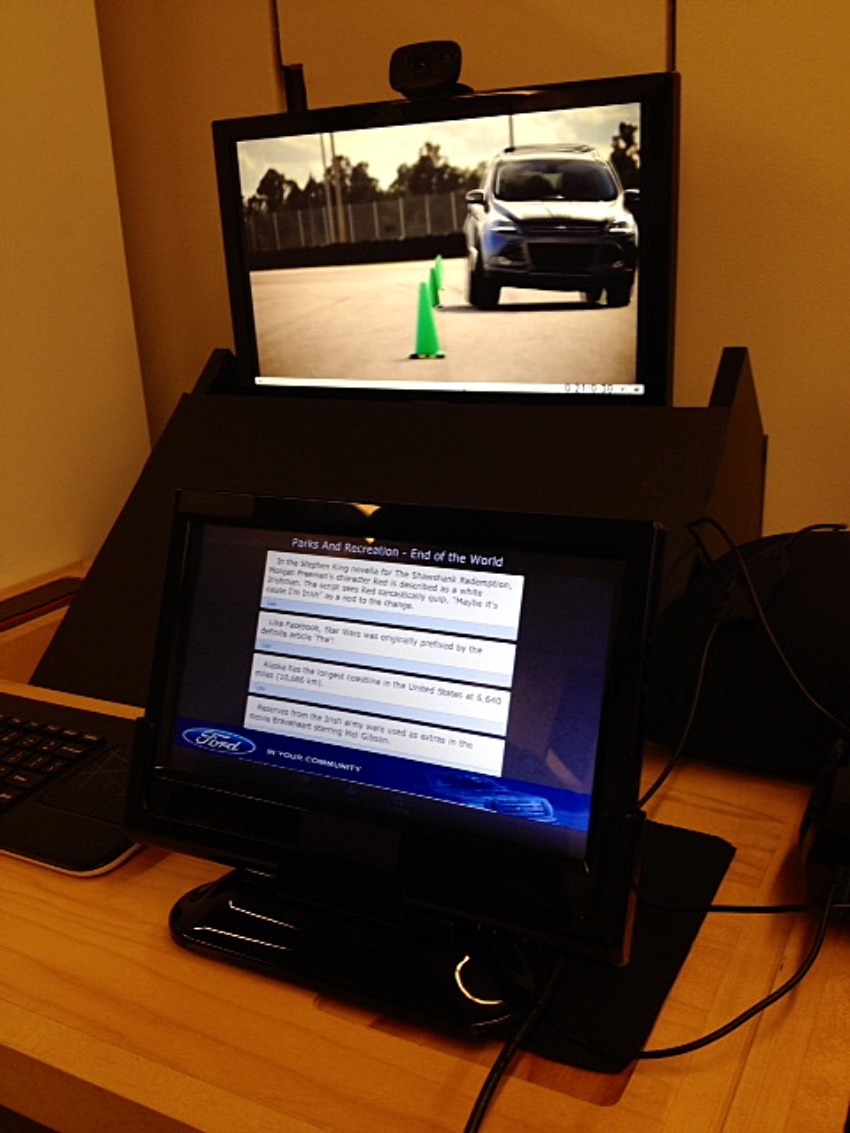
Figure 1. Individual viewing booth with a large computer screen, showing a TV program, and small tablet screen underneath. This example comes from Study 3, in which participants saw large, highly intrusive banners that occupied the full width of the tablet screen.
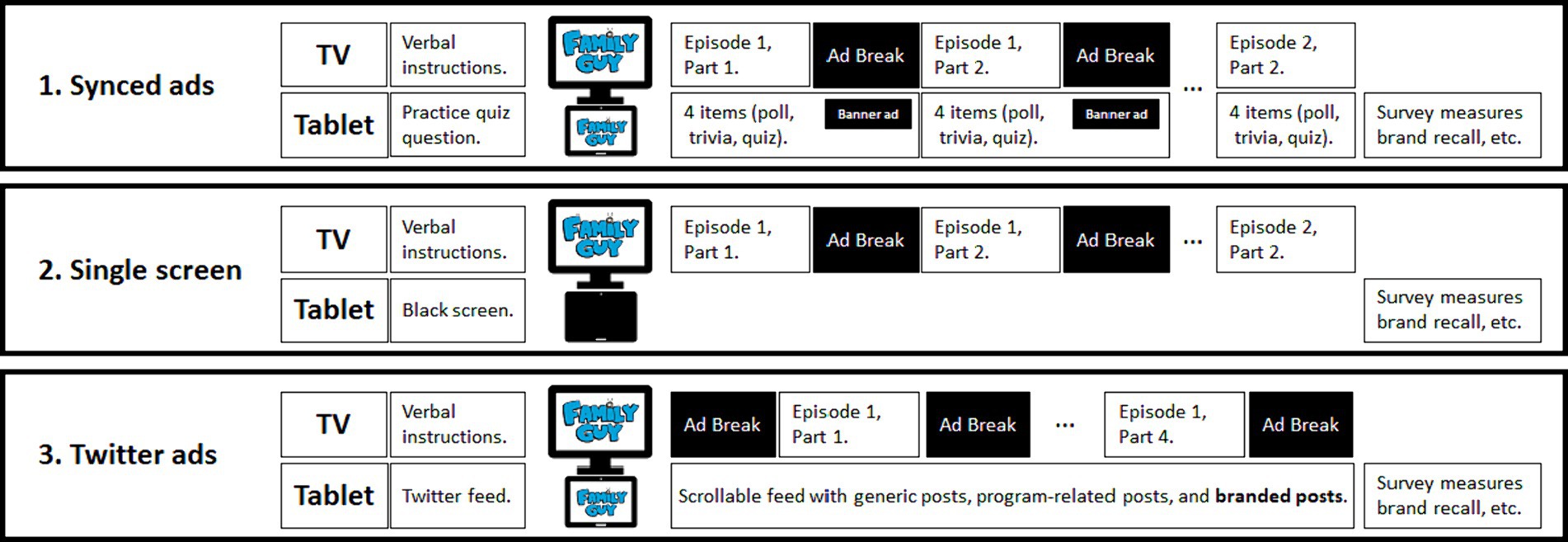
Figure 2. Schematic diagrams of the participant experience, depending on the study and group the participant was assigned to. (1) The synced ads condition replicated media multitasking across a large computer screen, showing a TV program, and a smaller tablet screen showing program-related content. Banner ads related to some of the test TV ads were superimposed over the program-related content on the smaller screen, either (a) simultaneously with the TV ad, or (b) sequentially, before or after the TV ad. Test ads seen without a matching banner ad on the smaller screen tested the effects of multitasking distraction. (2) In the single-screen group’s experience, the same TV content was seen on the larger screen, but the smaller tablet screen showed no content (the screen was black). (3) In the Twitter ads study, the program-related content on the smaller tablet screen was a scrollable Twitter feed with generic posts, program-related posts, and branded posts (i.e., Twitter ads) related to some of the test ads. Participants could scroll at their own pace, so the Twitter ads could appear before, simultaneously, or after their related TV ad. Again, test ads without a matching Twitter ad tested the effects of multitasking distraction. (The Family Guy logo may be obtained from 20th Television Animation).
3.2 Stimuli
Studies 1 to 3 varied the size and intrusiveness of precisely timed synced ads (see Figure 3 and Table 2). All three of these studies were replications using the same ad content and TV programs, allowing for all-else-equal comparisons of the effects of ad size. Figure 3 shows the differences in ad size across the four studies. The ad-size factor was named “intrusiveness” because its levels varied both ad size and the salience of the ad versus its background. In our first study (Study 1), we used synced ads that had a medium level of intrusiveness. The synced ad, which was displayed on the tablet’s screen for the 30-s duration of the TV ad it was aligned with, was a small rectangular floating banner ad, occupying 12% of the tablet screen’s width (but only 4% of its area). Although this ad was small, we coded it as medium in intrusiveness because the app’s content stopped updating and switched to a neutral background color during the ad breaks, and this likely attracted greater attention to the synced ad than the typical situation in which ads compete with other content, and suffer from “banner blindness” (Benway, 1998; Sharakhina et al., 2023). For this reason, we replicated Study 1 with a new Study 2 in which the same sized banner ad (12% of screen width, 4% of its area) had a lower level of intrusiveness because it was superimposed over program-related content. This content continued to automatically scroll behind the banner ad, while it displayed for 30 s. The last of these three studies, Study 3, replicated Study 2 but used a larger superimposed banner ad that occupied 100% of the screen’s width, filling the bottom fifth of the screen (i.e., 20% of its area). As in Study 2, the app’s content continued to scroll above the banner, so potentially this ad was still affected by “banner blindness,” despite its larger size. Nevertheless, in the context of these three studies, we coded this synced ad as having a high level of intrusiveness, although it was still small in comparison with some of the ads used in more recent studies. For comparison, in Segijn et al.’s (2023b) study, the highly intrusive pop-up synced ad (with a close button) occupied a similar 20% of the screen area (62% of the portrait-orientation tablet’s screen width). But the full-screen ads tested by Hoeck and Spann (2020) occupied 100% of the mobile phone’s screen width and area.
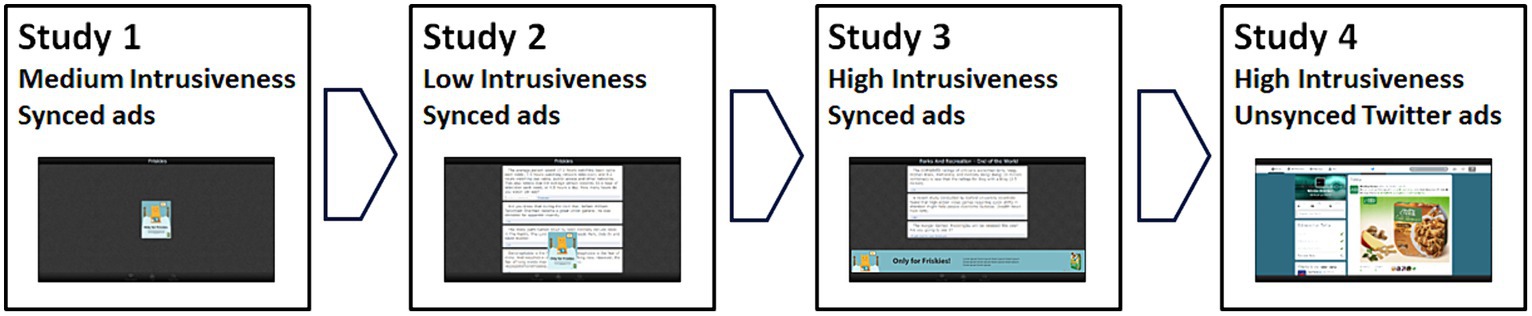
Figure 3. Studies 1 to 3 tested synced ads; Study 4 tested unsynced Twitter ads. In addition, Studies 1 to 4 varied the size (intrusiveness) of the synced or unsynced ad on the tablet screen.
Study 4 was a separate study, using different ads and program content, originally carried out to test the effect of social media multitasking on TV ad effectiveness. Subsequently, we realized that Study 4’s results could be included in the meta-analysis of Studies 1 to 3, to provide evidence about the effect of extreme sequential timing of synced ads. However, the results of Study 4 were not directly comparable with the other three studies, mainly because the TV program was shorter and there were fewer test ads. For this reason, we also report a separate analysis of Study 4’s results. In Study 4, the Twitter interface was replicated on a landscape-orientation tablet screen, with a stationary window on the left and a scrollable news-feed window on the right. The Twitter ads were either highly intrusive large picture posts, like the example in Figure 3, or smaller text posts. Picture posts occupied 44% of screen width (36% of its area). Text posts had the same width but occupied only 7% of screen area. Despite their smaller size, text posts had similar levels of brand recall as picture posts, because the branding text information in both types of ads was the same size (Wedel and Pieters, 2000; Myers et al., 2020). For this reason, we combined results from both types of Twitter ad in the analysis reported below. Similarly, another condition in Study 4 tested the effect of co-viewing (i.e., 2 people who normally watch TV together watching the same TV screen in the same room). In Study 4, unlike previous co-viewing studies (Bellman et al., 2012), there was no difference in brand recall between the co-viewing condition (recall = 20%) and the single-viewing condition (17%), so we combined both into one single-screen TV-only condition.
For participants in the TV-only group in Studies 1 to 3, the tablet screen went black during TV ad breaks, to direct attention to the TV screen, while controlling for the presence of a second screen. Otherwise, the presence or absence of a second screen might have been an alternative explanation for any differences between synced ads and TV-only ads. In the other groups, the tablet screen showed distracting program-related content, including during ad breaks. In Studies 1 to 3, the tablet app showed program-related news, trivia, and quizzes, and in Study 4 the Twitter feed contained generic posts (e.g., from Barack Obama, the US President when these studies were run), TV-network posts related to the TV program, and branded posts related to some of the test ads (see Figure 3). Like the interactive ads in Hoeck and Spann’s (2020) Experiment 2, and the puzzle in Lee et al.’s (2023) Study 2, this interactivity was designed to attract attention away from the TV, to replicate the significant negative multitasking effects reported in most previous studies (Segijn and Eisend, 2019). For example, in Studies 1 to 3, 30-s before the ad break at the end of the first 7-min part of the first episode of Family Guy (“Business Guy”), one of the interactive poll questions was: “Would you let Peter take over a billion-dollar company?” with response options: “Yes, he’s so dumb he’d be good at it!” or “No, he’d run the company into the ground within the first week!” (either response returned “Thanks for participating!”).
In Studies 1 to 3, an online pre-screening survey (see Supplementary Appendix C) measured program fandom (Juckel et al., 2016) to ensure that participants saw their favorite of two programs, to minimize confounding differences in program liking. In Study 4, the same effect was achieved by giving participants a choice of three programs to watch. The one-hour programs used in Studies 1 to 3 were two episodes of the same half-hour sitcom (e.g., 2 episodes of Family Guy). Participants saw a realistic level of TV ad clutter (Hammer et al., 2009; McGranaghan et al., 2022): five ad breaks with five ads in each break (i.e., a total of 25 ads). Recall might have been unrealistically high if attention had been focused on just one test ad, or there was a lack of competitive interference from ads for other brands (Pieters and Bijmolt, 1997; Jin et al., 2022). The potential interfering effects of the surrounding filler brands were controlled by randomizing the order of the test brands across participants. The three middle breaks were the test-ad breaks (to avoid primacy and recency effects), with two test ads in each break (i.e., a total of 6 test ads), in the first and third slots. The other slots were occupied by 19 filler ads for different brands and product categories, shown in a fixed order (i.e., Filler 1 first, Filler 2 s, etc., see Supplementary Appendix A). The six test ads, for familiar brands, were either late (last third) or early (frequently) branded (Newstead and Romaniuk, 2010) with low, medium, or high product category involvement (Mittal, 1995). This increased the external validity of this study by testing the effects of synced ads across a realistic range of differences in branding execution (Hartnett et al., 2016) and familiarity effects from consumer involvement and expertise (Vaughan et al., 2016; Rossiter and Percy, 2017).
For the simultaneous-ad groups in Studies 1 to 3, the tablet showed a synced ad for the entire duration of the matching 30-s TV ad on the larger screen. Three test ads had matching synced ads, the other three tested the distracting effect of multitasking. In the sequential group, synced ads appeared on the tablet before or after the TV ad, with either a 30- or a 60-s gap (relative to the onset of the TV ad). However, it was not possible to show a synced ad before the ad-break, for the test ads that appeared in the first slot of the break. This meant that the sequential-before condition was unevenly distributed across the six test brands, and for this reason, brand was controlled-for in the analysis. The sequential-ad group saw two test ads without synced ads to test multitasking’s distracting effect. Figure 4 shows how order of presentation was controlled for in Studies 1 to 3 by randomizing participants across six content-order variations.
Study 4 used three half-hour sitcom programs, two of which were not used in Studies 1 to 3, and four different test brands. Although the program time was shorter, the forgetting time between seeing the last test ad and answering the brand recall question was controlled to be the same as Studies 1 to 3 (15 min), so the recall results from both studies were comparable (e.g., single-screen TV-only recall was not significantly different). Also, the level of clutter was the same as that used in Studies 1 to 3: five ads in each break (i.e., a total of 20 ads in the 4 ad breaks). One break was before the program started and another was after it finished (see Figure 2). The remaining two breaks were equally spaced mid-roll breaks in the program. Each of the four test ads appeared in the middle position in the 4 ad breaks. Two of the 16 fixed-position filler ads had also been used as fillers in Studies 1 to 3 (see Supplementary Appendix B). Participants were randomly assigned to one of four viewing groups (see Table 2). One group watched only the TV program on the large computer screen (this group combined co-viewing, without a tablet, with single viewing with the tablet switched off). The other three groups multitasked across content on a large computer screen and smaller tablet screen (as in Studies 1 to 3). One of these groups saw a program-related Twitter feed with no synced ads, to test normal multitasking (i.e., the multitasking control group). The remaining two groups saw the same Twitter feed with the addition of branded posts (i.e., synced ads) for the four test ads. The two Twitter ad groups saw either (a) all picture posts (see Figure 3), or (b) all text posts. As was explained above, these two Twitter ad groups were combined in the analysis reported below, because ad-type made little difference to brand recall. Similar to Studies 1 to 3, the four test ads varied in early versus late branding and product category involvement (low vs. high [see Supplementary Appendix B]). Presentation order was controlled by random assignment to one of the 24 possible permutations in which the four test brands could be seen.
3.3 Participants
All four studies, which were conducted in the US in 2013 (Studies 1 to 3) and 2014 (Study 4), used age and sex quotas, based on the US Census, to recruit samples representative of the general population (see Table 3), rather than students. This increased the chances of finding negative effects of multitasking on affective outcomes, such as attitude toward the brand and attitude toward the ad (Segijn and Eisend, 2019). The participants were recruited from The Media Panel in Austin, Texas, and were compensated with a $30 USD American Express gift card for participating in a 90-min study (Studies 1 to 3), or a $25 USD American Express gift card for participating in a 60-min study (Study 4). All four studies were approved by the Murdoch University Research Ethics Office (Approval No. 2011/157). All participants signed informed consent forms. Using G*Power (Faul et al., 2007), the combined sample from Studies 1 to 3 (N = 749) had 99% power of detecting a significant (p < 0.05) medium-sized interaction (f = 0.25) between ad-size (3 levels: small, medium, large) and synced-ad presence and timing (3 levels: no synced ads [TV-only], simultaneous synced-ads, sequential synced ads). The multilevel analyses reported below were even more powerful because for Studies 1 to 3 they included within-subject tests comparing synced ads with another no-synced-ads condition: normal multitasking.
3.4 Procedure
The procedure used mild deception, with ethical review board approval, to replicate, in the lab, a normal low level of attention to advertising. Participants were not told the true purpose of the study until they were debriefed after they had completed answering the post-test questionnaire. Potential participants from The Media Panel were invited to “take part in our next study” (i.e., they were told little about the study before arriving at the lab). On arriving at the lab, participants were given an information sheet, which described the study only in vague, general terms, so that written informed consent could be obtained prior to the viewing session (participants could withdraw their consent at any time). The participant was then shown to the lab where the two screens were set up (as in Figure 1). In Studies 1 to 3, participants were given the following standard instructions:
“In this study we are testing how enjoyable it is to watch different types of TV programs on different types of screens. You have been selected to watch two episodes of [Family Guy/Parks and Recreation (determined by the participant’s fandom score in the pretest)]. The content lasts for about an hour.”
Participants in a synced ad condition received these additional instructions:
“You can interact with additional content related to the program using the computer screen in front of you. You’ll be taken through a brief training program, which will show you how to view this extra content [the training consisted of answering a practice interactive question].”
The procedure was very similar for Study 4, except that participants were invited to participate in a one-hour session, which potentially involved co-viewing TV with the person they normally co-viewed TV with. This meant that pairs of co-viewers needed to attend the lab at the same time, and depending on each pair’s order of arrival, they were assigned to either the co-viewing condition, or the pair was split up and each person was randomly assigned to one of the single-viewing conditions. The standard instructions were:
“In this study we are exploring the differences between viewing TV with someone else compared to viewing TV alone. First, you will practice choosing a program from an electronic program guide using this remote [the participant was then shown which keys did what]. Then you will choose a program to watch [from three available: Family Guy, The Big Bang Theory, Mike & Molly]. The content lasts for about half an hour.”
Participants in a Twitter condition received these additional instructions:
“You are in the Twitter group where you will be able to read tweets about the program on this tablet while you are watching the program on the TV screen.”
Participants were then left alone to complete the viewing session during which they watched either one episode of a half-hour sitcom, including ads (Study 4), or two episodes of the same sitcom (Studies 1 to 3). After the program content ended, the tablet computer was used to answer an online questionnaire about the program and the ads. In Studies 1 to 3, the questionnaire took about 15 min to complete. In Study 4, the questionnaire took slightly longer, as it included filler questions to create a 15-min delay between seeing the last test ad and being asked to recall the advertised brands. After completing the questionnaire, the participant was thanked, debriefed, and given their gift card as compensation.
3.5 Measures
In all four studies, when the program finished, participants completed an online questionnaire (see Supplementary Appendix C). In line with the stated purpose of the experiment, this questionnaire began with items measuring program liking (Coulter, 1998, 3 items, e.g., “I’m glad I had a chance to see this program,” α = 0.91). In Studies 1 to 3, this was immediately followed by a surprise question measuring unaided brand recall. Participants were asked to list all the brands they could remember seeing or hearing ads for during their viewing session (Snyder and Garcia-Garcia, 2016). Correct brands (minor misspellings allowed) were coded 1, otherwise 0. Next, purchase intention was measured, to avoid biasing answers to later questions about attitude toward the brand and attitude toward the ad (Rossiter et al., 2018). Purchase intention was measured as a subjective probability (%) using the 11-point Juster (1966) scale, which has been validated against consumer purchasing data (Wright et al., 2002). Four selected scale points from this scale were used to measure purchase intentions for products expected to have low to moderate involvement levels (Rossiter et al., 2018). In Study 4, attitude toward the brand was measured by the average of four 6-point semantic differential items (Gardner, 1985, e.g., “bad–good,” α = 0.98). In Studies 1 to 3, brand attitude was measured by a 6-point, validated single item (Bergkvist and Rossiter, 2007, 1 = “bad” to 6 = “good”). Finally, attitude toward the ad was measured by another validated single item on a 6-point scale (Bergkvist and Rossiter, 2007, e.g., 6 = “I liked it very much”). The survey ended with a manipulation check of product category involvement (Mittal, 1995, 5 items, e.g., “important–unimportant,” α = 0.89) and demographics measures (e.g., income, occupation). In Study 4, these demographics were measured between the program liking question and the brand recall question, along with other filler questions measuring experience with multitasking and social media, and attitudes toward Twitter. Because participants were randomly assigned to conditions, answers to these questions did not differ between groups and were not included in the analysis below. However, these filler questions helped to create a 15-min delay before measuring brand recall from the last-seen test ad.
3.6 Analysis
Multilevel regression was used to test the significance of the interaction between ad size (intrusiveness) and synced ad presence and timing (synced), using the lme4 package in R (Bates et al., 2015). Logistic regression (glmer) was used for the binary dependent variable, unaided brand recall. In these models, the correlation between repeated measures from the same individual was controlled using a random intercept. A similar random intercept was not used for the stimuli (the test-brand ads) because the manipulation of sequential timing was not shared equally among the test brands. Differences between brands were controlled using fixed effects. Similarly, because there were significant differences between the four studies in participant age (see Table 3), another fixed effect controlled for mean-centered age. A final set of fixed effects controlled for within-subject differences in product category involvement (mean-centered). The analysis and data for this study can be found at the following link: https://osf.io/ed5p4/.
4 Results
4.1 Manipulation checks
Manipulation checks using multilevel regression in R (see the Analysis section above) revealed significant differences in program liking among the four programs used across the four studies [programs 0 to 3, F (3, 508) = 13.3, p < 0.001]. However, there was no significant difference between the two programs used in Studies 1 to 3 [M0 = 5.0, 95% CI (4.8, 5.2) vs. M1 = 5.3, 95% CI (5.1, 5.5), p = 0.06]. The one significant difference was associated with program 2, used in Study 4 [M0 vs. M2 = 3.7, 95% CI (3.3, 4.2), p < 0.001; M0 vs. M3 = 5.0, 95% CI (4.7, 5.4), p = 0.97]. For this reason, among others, the Study 4 data were re-analyzed separately. As would be expected, brand recall was significantly lower for late-branded ads [Mearly = 21%, 95% CI (19%, 23%), vs. Mlate = 6%, 95% CI (6, 7%), b = −0.63, SE = 0.08, p < 0.001]. Similarly, perceived product category involvement increased with ascending levels of expected involvement, with significant differences between all three levels [Mlow = 4.1 (on a 1 to 6 scale), 95% CI (4.1, 4.2) vs. Mmedium = 4.2, 95% CI (4.2, 4.3), p = 0.003, Mmedium vs. Mhigh = 4.6, 95% CI (4.5, 4.6), p < 0.001, F (2, 4,565) = 118.2, p < 0.001]. The manipulations of ad size and timing were objective and observable, and so did not need manipulation checks (Perdue and Summers, 1986).
4.2 Moderating effect of ad size
There was no evidence for a moderating effect of ad size (intrusiveness) as the interaction between intrusiveness and synced ad presence and timing (synced) was not significant, for any of the four dependent variables (unaided brand recall, attitudes toward the ad and the brand, and purchase intention, all F < 1). Table 4 shows the results for unaided brand recall. None of the main effect coefficients for intrusion were significant (apart from the intercept). And none of the coefficients for individual levels of the interaction were significant either. Controlling for significant effects of product category involvement and four (of the six) individual brands, the only significant effects, versus the intercept (multitasking), were for single screen TV ads (p = 0.029), and sequential-after synced ads (p = 0.049).
The effects of ad size on unaided brand recall are illustrated in Figure 5. In this figure, the effect of multitasking on recall (left, red dots) is compared with the effects of other levels of synced ad presence and timing under conditions of low, medium, and high intrusiveness. The recall means for each level of intrusiveness were compared using the emmeans package in R. The single-screen (TV only) mean was at least marginally higher than multitasking for all three intrusiveness levels (low p = 0.029, medium p = 0.076, high p = 0.016). Sequential-after ads were significantly higher for two levels, low (p = 0.049) and medium (p = 0.029; high p = 0.52). Simultaneous ads were marginally higher only for the low level (p = 0.066), and sequential-before ads were never even marginally higher (lowest p = 0.18 for medium intrusiveness). These interaction-test results explain the significant main effects for single screen and sequential-after ads. These findings suggest that ad size makes no difference to the effectiveness of synced ads. In other words, small, normal-sized synced ads are as effective as large, full-screen synced ads. These results also suggest that synced ads work better when they are seen sequentially rather than simultaneously, and that sequential ads work better when seen after the TV ad.
4.3 Moderating effect of timing
Since the interaction-effect test, using the matched data from Studies 1, 2, and 3, revealed no significant moderating effect of ad size, a separate analysis further investigated the main effects of synced ad presence and timing. This analysis added in the data from Study 4, which tested the effects of synced ads in social media (Twitter), as opposed to being in a program-related app that could precisely time the onset of synced ads relative to the scheduled start of the broadcast program. Because Study 4’s participants self-controlled their rate of scrolling through the simulated Twitter feed, the timing of the synced ads relative to the matching TV ad was unknown, and randomly could have occurred before, during, or after the TV ad. (Since Study 4 was carried out, audio content recognition software allows the replacement of any ad a person is about to scroll to by a simultaneous synced ad [Segijn, 2019].) The regression models used for this analysis included an additional four fixed effects to control for Study 4’s different set of test brands.
The results for unaided brand recall largely replicated the significant main effect of synced-ad presence and timing reported in Table 4 [F (5, 2,514) = 3.87, p = 0.002]. Compared with multitasking recall [Mmultitasking = 11%, 95% CI = (9, 13%)], single-screen viewing was significantly higher [Msingle screen = 15%, 95% CI = (13, 17%), p = 0.010]. Recall was also significantly higher for sequential-after ads [Msequential after = 15%, 95% CI = (12, 19%), p = 0.043]. There were no significant differences for simultaneous ads [Msimultaneous = 13%, 95% CI = (11, 17%), p = 0.22] or sequential-before ads [Msequential before = 14%, 95% CI = (10, 20%), p = 0.39], even after controlling for brand. The new result from this analysis was that untimed synced ads (Twitter ads) had a significantly higher recall, compared with multitasking [Muntimed synced ads = 25%, 95% CI = (19, 32%), p < 0.001]. Table 5 reports the estimated marginal means and 95% confidence intervals for the four dependent variables, under various conditions of synced-ad presence and timing. This pattern of results suggests that synced ad timing is not important, and that simultaneous exposure (which potentially divides attention) is best avoided.
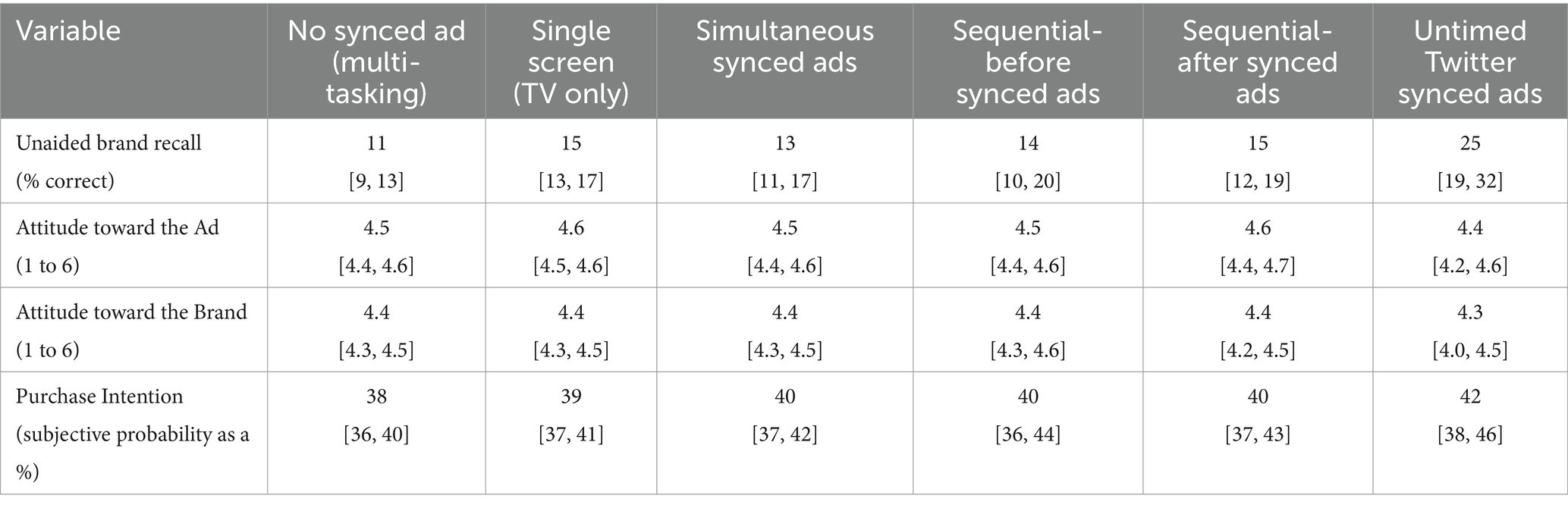
Table 5. Estimated marginal means and 95% confidence intervals for levels of synced-ad presence and timing.
4.4 Separate test of untimed synced ads
Besides the different test brands, Study 4’s participants watched different (and shorter) TV programs, one of which was significantly less liked, and saw different content on the tablet (social media rather than a program app). For these reasons, separate analyses were conducted using the data from Study 4. Interestingly, although there was a significant main effect of synced-ad presence and timing [F (2, 225) = 4.74, p = 0.010], single-screen (TV-only) recall [Msingle screen = 14%, 95% CI = (11%, 18%)] was not significantly higher than multitasking recall in this study [Mmultitasking = 11, 95% CI = (6, 18%), p = 0.55]. This may have been due to the use of fewer ads and a shorter gap before measuring recall. However, recall was significantly higher for untimed synced ads [Muntimed synced ads = 24%, 95% CI = (19, 31%), p = 0.008]. There were no significant effects of untimed synced ads on attitude toward the ad, attitude toward the brand, or purchase intention. This separate analysis of Study 4’s data confirmed the combined-study results reported above.
5 Discussion
Synced ads have the potential to improve the effectiveness of advertising when consumers are multitasking and dividing their attention across two screens (Segijn, 2019). The results of these four studies show that synced ads can improve brand awareness compared with a multitasking control condition. This justifies the growing stream of research into synced ad effectiveness, which has been hampered by many null results. The few studies reporting positive results, relative to multitasking, used unrealistic manipulations (Garaus et al., 2017), or full-screen synced ads (Hoeck and Spann, 2020) that consumers and regulators might reject. Our results show the effects of synced ads do not depend on ad size or timing. Synced ads did not need to be large (e.g., occupying 100% of the mobile device’s screen) to improve brand awareness. In fact, the best-performing synced ads in these four studies were normal-sized social media ads (Twitter posts). Furthermore, these results suggest that synced ads do not have to be precisely timed to coincide with their matching large-screen (TV) ad. In fact, synced ads were more likely to increase awareness when they were not seen simultaneously with the TV ad. The positive effects of non-simultaneous exposure suggest that simultaneous exposures divide attention across ads for the same brand. In three of these studies, synced ads after the TV ad performed better, compared with multitasking, than synced ads seen before the TV ad, or simultaneously with the TV ad. A fourth study showed that synced ads can be effective when they are imprecisely timed Twitter posts, which could be seen before, during, or after the TV ad, depending on how fast people scrolled their social media feed. Synced ads had no improving effects, relative to multitasking, on attitudes toward the ad or the brand, or purchase intention.
5.1 Theoretical implications
These four studies investigated the importance of two potential moderators of synced-ad effectiveness: ad size and timing, under realistic multitasking conditions. The one prior study that reported positive effects of synced ads compared with realistic multitasking used full screen (width and depth) synced ads, combined with low TV ad clutter (1 ad in 5 min; 9 ad minutes per hour) (Hoeck and Spann, 2020). This meant it was unclear, before our research, whether synced ads were effective if they were smaller, normal-sized social media ads, seen in the context of more realistic TV ad clutter (15 ad minutes per hour). Furthermore, prior research often tested a single synced ad for a single brand (e.g., Lee et al., 2023, Study 2), raising questions about whether the positive or null effects reported could be explained by unique brand or execution effects.
First, our four studies had high external validity, because in total they tested synced ads for 10 brands from a range of categories with low, medium, and high product involvement. Second, these studies heightened external validity by using familiar brands, realistic manipulations of multitasking, and non-student samples. In a meta-analysis of multitasking studies, these factors had reduced the chances of finding negative effects of multitasking (Segijn and Eisend, 2019). This made it harder for us to find a positive effect of synced ads, relative to multitasking. But it was very important for us to do so, as synced ads will only have relevance for advertisers, consumers, and regulators if they are effective under realistic conditions.
Second, these results show that ad size does not moderate synced-ad effectiveness. Small, normal-sized social media ads (Twitter posts) were the most effective synced ads, even though they were less than half the width of the tablet screen. We labeled our ad-size variable “intrusiveness” (Li et al., 2002), because in our medium-intrusiveness condition, the synced ad was the same size as the small-ad (low-intrusiveness) condition. The only difference was that in the medium condition, there was no text to read on the screen, so the ad was the only object on the screen. This increased the salience of the ad, making it more intrusive into the viewer’s multi-task of consuming program-related content across two screens. However, this level of intrusion was mild compared with the use of pop-over synced ads in other research. For example, in Segijn et al. (2023b), a pop-over synced ad intruded over whatever magazine content the viewer was reading on a tablet, when characters in the program on the main screen started discussing the advertised product category (frozen yogurt). Because of this high level of intrusiveness, viewers were able to close the pop-over synced ad and all of them had closed it after an average of 6 s. Intrusive pop-up ads likely concentrate attention on the close button rather than the content of the ad (Frade et al., 2022). This short exposure time likely reduced the effectiveness of these highly intrusive synced ads, compared with the less-intrusive long-exposure synced ads used in our four studies. In our studies, synced ads remained on screen for 30 s in Studies 1 to 3. In Study 4, viewers could scroll past the synced ads, but not close them, so exposure time was untimed, but likely longer than 6 s. This effect of exposure time suggests that synced ads need to be consciously noticed, and therefore mere exposure theories (e.g., Zajonc, 1980) cannot explain the effects of synced ads on cognitive measures like brand awareness, especially when the advertised brand is unfamiliar (Simmonds et al., 2020). The need for sustained conscious attention suggests the explanation for synced-ad effectiveness is more likely to be an active processing theory such as multiple source perception (Harkins and Petty, 1981), or forward priming and image transfer effects (Edell and Keller, 1989; Voorveld et al., 2011).
Third, these studies found no evidence for a moderating effect of synced-ad timing, other than a definite lack of evidence for simultaneous synced-ad effectiveness. The media multitasking literature emphasizes related simultaneous exposure (Segijn and Eisend, 2019), because the idea of threaded cognition suggests it is easier to process related tasks (Salvucci and Taatgen, 2008). But in these four studies, simultaneous synced ads were not associated with any significant differences compared with multitasking. Our results contrast with previous research which found that simultaneous synced ads were just as effective, on a 0-to-4 memory scale, as sequential synced ads seen 45 s before or after the onset of the 30-s TV ad (Segijn et al., 2021). In the sequential conditions in that study, there was a 15-s “thread-cutting” gap (the length of a filler commercial) in which no content for the advertised brand appeared on either screen. In three of our studies, the results for sequential ads averaged over two different gap times. The first was a shorter 30-s gap (equal to the duration of the TV commercial), which meant there was no gap between brand-related content across screens. The second was a longer 60-s gap, which meant there was a thread-cutting gap of 30 s (i.e., twice as long as 15 s), in which no brand-related content appeared on either screen. The significant results for sequential-after synced ads and untimed synced ads in our research suggests a role for spaced learning as an explanation and an enhancer of the effects of sequential synced ads (Janiszewski et al., 2003; Segijn et al., 2021). The importance of seeing a synced ad after the TV ad suggests the TV ad may prime attention to the subsequent synced ad (Edell and Keller, 1989; Voorveld et al., 2011). Eye-tracking data from a prior study hint at a mediating role for attention primed by the TV ad, so that attention was marginally higher for synced ads seen simultaneously or after the TV ad, relative to a synced ad seen before the TV ad (Segijn et al., 2021). In summary, the effectiveness of sequential synced ads suggests a diminished role for theories that exclusively explain simultaneous exposure. Our results suggest the effects of synced ads are more likely to be explained by traditional cross-media advertising theories than multitasking theories like threaded cognition (Salvucci and Taatgen, 2008; Segijn et al., 2021).
Our Study 4 used synced ads with imprecise timing, which could have appeared before or after the TV ad with thread-cutting gaps longer than 30 s. This extended the definition of synced ads to include any ad appearing during the same TV program as the TV ad. This may be a realistic definition of synced ads in practice (Kantrowitz, 2014). It is arguable, however, whether this definition of synced ads is too wide (Segijn et al., 2021). More research is needed to define the boundary between synced ads and normal cross-media ads. Synced ads might be better defined as massed cross-media ads, with intervals measured in seconds or minutes, as opposed to spaced cross-media ads, with intervals measured in days or weeks. The boundary might the duration of a TV program (e.g., one hour), or potentially a three-hour binge-watching session (Schweidel and Moe, 2016). Spaced advertising is generally more effective for improving memory (Janiszewski et al., 2003). If synced ads are massed cross-media ads, their effects could be measured like those of other cross-media campaigns, using field experiments, with sales as the outcome variable (Taylor et al., 2013; Srinivasan et al., 2016).
Another question for future research is whether synced ads benefit from even higher levels of massed repetition. Like prior studies of synced ads (Segijn and Voorveld, 2021), this research found no effect of a single synced ad exposure on brand attitude, most likely because improvements in brand attitude require repetition (Schmidt and Eisend, 2015). But repeated synced ads may annoy viewers (Huang and Huh, 2018; Hussain et al., 2018). Repeat exposures delivered outside the same TV program, or outside the same viewing session, would be more like normal spaced exposures, rather than massed exposures. Future research may find that synced ads do not improve brand attitude even when high levels of repetition are used, because the effects of synced ads are mediated by cognitive processes like attention, which can trigger resistance (Fransen et al., 2015), rather than emotional processes like mere exposure (Zajonc, 1980) or affect transfer (Segijn and Eisend, 2019).
5.2 Practical implications
The results of this research suggest that ad size makes no difference to the effectiveness of synced ads, and that simultaneous ad timing is not critical for synced-ad effectiveness. The practical implications of these results should be interpreted from the perspective of the latest, evidence-based approach to marketing, advertising, and media planning (Sharp et al., 2024). Using a five-year planning horizon, most of a brand’s sales over that period will come from light buyers or new buyers, rather than current buyers (Graham and Kennedy, 2022). The main purpose of brand-awareness advertising therefore (as opposed to purchase facilitation advertising), is to refresh, and occasionally build, memories related to the brand in the minds of these light or new buyers (Rossiter et al., 2018; Bergkvist and Taylor, 2022). This requires reaching, if possible, 100% of potential future customers with at least one ad exposure, at a frequency (e.g., one per month) to ensure that forgetting between exposures is minimized (Zielske, 1959; Taylor et al., 2013; Rossiter et al., 2018). Advertisers typically select one primary medium, traditionally TV, which reaches most consumers and can deliver all the communication objectives of the campaign (Rossiter et al., 2018). Then, secondary media (e.g., radio, outdoor) are added if it is impossible to achieve 80% reach after exhausting the potential reach of the primary medium (Romaniuk et al., 2013; Rossiter et al., 2018). However, nowadays, TV receives only one fifth (21%) of ad spending and for most advertisers, the primary medium is digital advertising (Statista, 2023a). This is because TV viewing has declined, in terms of hours per week, among viewers aged less than 35 years (Barwise et al., 2020). In the current advertising and media context, no advertiser should be relying on a single primary medium to achieve all their reach and other communication objectives. All media plans should be multi-media plans.
Synced ads, and the experiments reported in this research, were conceived at a time (before 2014) when TV was still the most-common primary medium, receiving over 40% of advertising expenditure (Statista, 2023c). In those days, TV-ad exposures were considered “wasted” if viewers were media multitasking, and synced ads were invented as a way of restoring those lost TV exposures, or at least replacing them with “make good” exposures on the mobile phone. If synced ads are restoring or replacement exposures, rather than just additional repeat exposures, the business case for synced ads is stronger. Spending money on repeat exposure has diminishing returns and would be better spent delivering a first exposure to a different consumer (Taylor et al., 2013). It was probably the logic of replacing a lost TV ad with closely matching synced ad (same viewer, same time, same program) that drove the initial interest in delivering simultaneous synced ads (Kantrowitz, 2014). This requires knowing (or predicting) whether the viewer was exposed to the TV ad. One way of doing that was to run the ads in a special program-related app, which viewers would start using when the program began so in-app ads could be timed to sync simultaneously. This was the method tested in this article’s Studies 1 to 3. But the likely low take-up of program-related apps inspired another solution, predicting (at least 2 s in advance) that a person was likely a viewer of a TV ad in a specific TV program, based on their demographics and the stationarity of their mobile phone (indicating they were likely sitting at home) (Kantrowitz, 2014). Then ACR databases were expanded to recognize ads as well as music and program content. This allowed companies like Beatgrid (beatgrid.co) to listen to all the ads its consenting panelists hear to estimate the unique reach of each medium in a multi-media plan. But ACR can also be used, without consent, to hear an ad in a primary medium and trigger a matching synced ad (Segijn et al., 2023b). These days, however, the primary medium may be social media, such as Facebook, rather than TV. But if social media are mainly consumed on the phone, they are less likely to be affected by multitasking, and would not need synced ads to remedy multitasking’s negative effects.
The results of these four studies confirm that multitasking has a negative effect on TV ads, reducing their effectiveness, even when representative samples of consumers are used (Segijn and Eisend, 2019). Furthermore, these results confirm that, when TV viewers are multitasking, synced ads can be useful for improving cognitive outcomes like brand awareness. These significant effects of synced ads did not require highly intrusive (pop-up) full-screen ad formats. Intrusive pop-up ads, and interstitial formats, such as Facebook story ads, are more expensive to buy than normal online advertising (e.g., Facebook Feed ads). If synced ads needed these expensive formats, they would be an unrealistic option for most advertisers. And consumers and regulators are more likely to react negatively to large and intrusive synced ads. The finding of no moderating effect of synced ad size suggests synced ads are a viable and acceptable remedy for the negative effects of multitasking on TV advertising (Segijn and Eisend, 2019).
However, these results also suggest that precise simultaneous timing of synced ads is not essential for their effectiveness. In these studies, imprecisely timed social media ads were the most effective synced ads. The practical implication of those results is that advertisers do not have to pay for expensive simultaneous syncing technology (Kantrowitz, 2014), or support unethical dataveillance to control the timing of synced ads, which, could be illegal in some countries (Strycharz and Segijn, 2024). Synced ads with imprecise timing are likely to avoid the perception of intrusive creepiness when viewers notice a relationship between ads on the TV and ads on a mobile device (Segijn et al., 2021), because their coincidence will resemble typical multimedia-campaign cross-media repetition. Moreover, if advertisers are using a multimedia advertising strategy to reach all potential customers (Graham and Kennedy, 2022), imprecisely timed synced ads are being delivered anyway. In other words, if advertisers are using evidence-based best practice when planning their media buys, these results imply there is no need to change their current daily practice, to include buying synced ads.
5.3 Limitations and suggestions for future research
This research has limitations that suggest directions for future research. First, the most important limitation of these studies is that they were carried out over 10 years ago. Many things have changed in the meantime, including the eclipsing of TV by digital media, and the ability to use ACR to target simultaneous synced ads. Future studies should investigate the effects of synced ads when TV is not the primary medium suffering from multitasking, and the potentially heightened effects of synced ads when these are individually targeted (e.g., Bellman et al., 2013), rather than delivered en masse by an experimental manipulation. A second limitation is that these studies had no measures of process variables, such as visual attention (Segijn et al., 2021). Future research should test whether attention, or other variables, such as counterarguing (Jeong and Hwang, 2012; Segijn et al., 2016, 2018), mediate the effects of sequential synced ads, the most effective form of synced ads in this research. Process measures would clarify whether priming effects originate only from the TV ad, or whether a sequential-before synced ad can prime exposure to the TV ad. Second, these studies manipulated multitasking using a program app, or social media (Twitter). Future research should test the effects of more engaging forms of multitasking, such as participants looking at their personal Facebook feed [Facebook has its own ACR technology (SMG, 2014)]. Finally, future research could test other forms of intrusion besides ad size, such as short video ads with sound on TikTok, or audio advertising on a smart speaker.
6 Conclusion
Synced ads are a new form of advertising on mobile devices designed to combat the negative effects of multitasking on TV advertising. In prior research, effective synced ads were intrusively large, occupying the full screen of the mobile device (Hoeck and Spann, 2020). This research investigated whether ad size and ad timing moderated the effects of synced ads. It reports the results of four realistic studies, because realism affects the results of multitasking studies (Segijn and Eisend, 2019). Ad size made no difference to the effectiveness of synced ads, compared with multitasking. The main effect of ad timing was that synced ads were not effective when they were shown simultaneously with the matching TV ad. These results provide guidance for future research, and advertisers buying synced ads.
Data availability statement
The analysis and data for this study can be found at the following link: https://osf.io/ed5p4/.
Ethics statement
The studies involving humans were approved by the Murdoch University Research Ethics Office (Approval No. 2011/157). The studies were conducted in accordance with the local legislation and institutional requirements. The participants provided their written informed consent to participate in this study.
Author contributions
JB: Writing – original draft, Writing – review & editing. DV: Writing – review & editing, Conceptualization, Funding acquisition, Methodology, Resources. BW: Methodology, Resources, Project administration, Supervision, Writing – review & editing. SB: Conceptualization, Formal analysis, Writing – original draft, Writing – review & editing.
Funding
The author(s) declare that financial support was received for the research, authorship, and/or publication of this article. These studies were funded by the Beyond: 30 consortium as Study 59 (Studies 1 to 3) and Study 63 (Study 4).
Conflict of interest
This research was sponsored by a consortium of companies that included Meta, as well as its competitors (e.g., television networks and Google). The authors therefore believe that this balance of interests means that their research is independent, not influenced by any single sponsor or industry group. In addition, SB works for an institute whose corporate sponsors provide funding on the condition that the institute remains completely independent, with written acceptance that sponsors have no influence over data analysis or its interpretation.
The author(s) declared that they were an editorial board member of Frontiers, at the time of submission. This had no impact on the peer review process and the final decision.
Publisher’s note
All claims expressed in this article are solely those of the authors and do not necessarily represent those of their affiliated organizations, or those of the publisher, the editors and the reviewers. Any product that may be evaluated in this article, or claim that may be made by its manufacturer, is not guaranteed or endorsed by the publisher.
Supplementary material
The Supplementary material for this article can be found online at: https://www.frontiersin.org/articles/10.3389/fcomm.2024.1343315/full#supplementary-material
Footnotes
1. ^https://www.facebook.com/business/ads-guide/image/facebook-feed
2. ^https://www.facebook.com/business/ads-guide/image/facebook-story
References
Abdollahi, M., Fang, Y., Liu, H., and Segijn, C. M. (2023). “Examining affect, relevance, and creepiness as underlying mechanisms of consumers’ attitudes toward synced ads in valenced contexts” in Advances in advertising research. eds. A. Vignolles and M. K. Waiguny, vol. XII (Wiesbaden, Germany: European Advertising Academy/Springer Gabler), 65–80.
Barwise, P., Bellman, S., and Beal, V. (2020). Why do people watch so much television and video? Implications for the future of viewing and advertising. J. Advert. Res. 60, 121–134. doi: 10.2501/jar-2019-024
Bates, D., Mächler, M., Bolker, B., and Walker, S. (2015). Fitting linear mixed-effects models using lme4. J. Stat. Softw. 67, 1–48. doi: 10.18637/jss.v067.i01
Bellman, S., Rossiter, J. R., Schweda, A., and Varan, D. (2012). How coviewing reduces the effectiveness of TV advertising. J. Mark. Commun. 18, 363–378. doi: 10.1080/13527266.2010.531750
Bellman, S., Murphy, J., Treleaven-Hassard, S., O’Farrell, J., Qiu, L., and Varan, D. (2013). Using internet behavior to deliver relevant television commercials. J. Interact. Mark. 27, 130–140. doi: 10.1016/j.intmar.2012.12.001
Benway, J. P. (1998). Banner blindness: the irony of attention grabbing on the world wide web. Proc. Hum. Fact. Ergonom. Soc. Ann. Meet. 42, 463–467. doi: 10.1177/154193129804200504
Bergkvist, L., and Rossiter, J. R. (2007). The predictive validity of multiple-item versus single-item measures of the same constructs. J. Mark. Res. 44, 175–184. doi: 10.1509/jmkr.44.2.175
Bergkvist, L., and Taylor, C. R. (2022). Reviving and improving brand awareness as a construct in advertising research. J. Advert. 51, 294–307. doi: 10.1080/00913367.2022.2039886
Bleier, A., and Eisenbeiss, M. (2015). Personalized online advertising effectiveness: the interplay of what, when, and where. Mark. Sci. 34, 669–688. doi: 10.1287/mksc.2015.0930
Boerman, S. C., and Segijn, C. M. (2022). Awareness and perceived appropriateness of synced advertising in Dutch adults. J. Interact. Advert. 22, 187–194. doi: 10.1080/15252019.2022.2046216
Boerman, S. C., Kruikemeier, S., and Borgesius, F. J. Z. (2017). Online behavioral advertising: a literature review and research agenda. J. Advert. 46, 363–376. doi: 10.1080/00913367.2017.1339368
Brasel, S. A., and Gips, J. (2017). Media multitasking: how visual cues affect switching behavior. Comput. Hum. Behav. 77, 258–265. doi: 10.1016/j.chb.2017.08.042
Büchi, M., Festic, N., and Latzer, M. (2022). The chilling effects of digital dataveillance: a theoretical model and an empirical research agenda. Big Data Soc. 9:205395172110653. doi: 10.1177/20539517211065368
Chandon, J.-L., Laurent, G., and Lambert-Pandraud, R. (2022). Battling for consumer memory: assessing brand exclusiveness and brand dominance from citation-list. J. Bus. Res. 145, 468–481. doi: 10.1016/j.jbusres.2022.02.036
Clarke, R. (1988). Information technology and dataveillance. Commun. ACM 31, 498–512. doi: 10.1145/42411.42413
Collins, D., and Gordon, M. (2022). 40 states settle Google location-tracking charges for $392M. Hartford, CN, USA: The Associated Press. Available at: https://apnews.com/article/google-privacy-settlement-location-data-57da4f0d3ae5d69b14f4b284dd084cca
Coulter, K. S. (1998). The effects of affective responses to media context on advertising evaluations. J. Advert. 27, 41–51. doi: 10.1080/00913367.1998.10673568
Duckler, M. (2016). “Nissan: accelerating brand awareness to drive business growth.” LinkedIn, November 21. Available at: https://www.linkedin.com/pulse/nissan-accelerating-brand-awareness-drive-business-growth-duckler
Ebiquity (2021). “The challenge of attention.” April 2021. London: Ebiquity. Available at: https://ebiquity.com/news-insights/research/the-challenge-of-attention/
Edell, J. A., and Keller, K. L. (1989). The information processing of coordinated media campaigns. J. Mark. Res. 26, 149–163. doi: 10.1177/002224378902600202
Eijlers, E., Boksem, M. A. S., and Smidts, A. (2020). Measuring neural arousal for advertisements and its relationship with advertising success. Front. Neurosci. 14:736. doi: 10.3389/fnins.2020.00736
Eysenck, M. W. (1976). Arousal, learning, and memory. Psychol. Bull. 83, 389–404. doi: 10.1037/0033-2909.83.3.389
Faul, F., Erdfelder, E., Lang, A.-G., and Buchner, A. (2007). G*power 3: a flexible statistical power analysis program for the social, behavioral, and biomedical sciences. Behav. Res. Methods 39, 175–191. doi: 10.3758/bf03193146
Frade, J. L. H., Oliveira, J. H. C., and Giraldi, J. M. E. (2022). Skippable or non-skippable? Pre-roll or mid-roll? Visual attention and effectiveness of in-stream ads. Int. J. Advert. 42, 1242–1266. doi: 10.1080/02650487.2022.2153529
Fransen, M. L., Verlegh, P. W. J., Kirmani, A., and Smit, E. G. (2015). A typology of consumer strategies for resisting advertising, and a review of mechanisms for countering them. Int. J. Advert. 34, 6–16. doi: 10.1080/02650487.2014.995284
Garaus, M., Wagner, U., and Bäck, A.-M. (2017). The effect of media multitasking on advertising message effectiveness. Psychol. Mark. 34, 138–156. doi: 10.1002/mar.20980
Gardner, M. P. (1985). Does attitude toward the ad affect brand attitude under a brand evaluation set? J. Mark. Res. 22, 192–198. doi: 10.1177/002224378502200208
Geuens, M., and De Pelsmacker, P. (2017). Planning and conducting experimental advertising research and questionnaire design. J. Advert. 46, 83–100. doi: 10.1080/00913367.2016.1225233
Google (2023). About brand lift. Available at: https://support.google.com/google-ads/answer/9049825
Graham, C., and Kennedy, R. (2022). Quantifying the target market for advertisers. J. Consum. Behav. 21, 33–48. doi: 10.1002/cb.1986
Hammer, P., Riebe, E., and Kennedy, R. (2009). How clutter affects advertising effectiveness. J. Advert. Res. 49, 159–163. doi: 10.2501/s0021849909090217
Harkins, S. G., and Petty, R. E. (1981). Effects of source magnification of cognitive effort on attitudes: an information-processing view. J. Pers. Soc. Psychol. 40, 401–413. doi: 10.1037/0022-3514.40.3.401
Hartnett, N., Kennedy, R., Sharp, B., and Greenacre, L. (2016). Creative that sells: how advertising execution affects sales. J. Advert. 45, 102–112. doi: 10.1080/00913367.2015.1077491
Hoeck, L., and Spann, M. (2020). An experimental analysis of the effectiveness of multi-screen advertising. J. Interact. Mark. 50, 81–99. doi: 10.1016/j.intmar.2020.01.002
Huang, S., and Huh, J. (2018). Redundancy gain effects in incidental exposure to multiple ads on the internet. J. Curr. Iss. Res. Advert. 39, 67–82. doi: 10.1080/10641734.2017.1372324
Hussain, R., Ferdous, A. S., and Mort, G. S. (2018). Impact of web banner advertising frequency on attitude. Asia Pac. J. Mark. Logist. 30, 380–399. doi: 10.1108/APJML-04-2017-0063
Hwang, Y., and Jeong, S.-H. (2021). The role of user control in media multitasking effects. Media Psychol. 24, 79–108. doi: 10.1080/15213269.2019.1659152
Janiszewski, C., Noel, H., and Sawyer, A. G. (2003). A meta-analysis of the spacing effect in verbal learning: implications for research on advertising repetition and consumer memory. J. Consum. Res. 30, 138–149. doi: 10.1086/374692
Jeong, S.-H., and Hwang, Y. (2012). Does multitasking increase or decrease persuasion? Effects of multitasking on comprehension and counterarguing. J. Commun. 62, 571–587. doi: 10.1111/j.1460-2466.2012.01659.x
Jin, H. S., Kerr, G., Suh, J., Kim, H. J., and Sheehan, B. (2022). The power of creative advertising: creative ads impair recall and attitudes toward other ads. Int. J. Advert. 41, 1521–1540. doi: 10.1080/02650487.2022.2045817
Juckel, J., Bellman, S., and Varan, D. (2016). The utility of arc length for continuous response measurement of audience responses to humour. Israeli J. Humor Res. 5, 46–64.
Juster, F. T. (1966). Consumer buying intentions and purchase probability: an experiment in survey design. J. Am. Stat. Assoc. 61, 658–696. doi: 10.1080/01621459.1966.10480897
Kantrowitz, A. (2014). Your TV and phone may soon double team you: Xaxis’ sync wants to show same ads at same time on the tube, mobiles. Advert. Age 85:8.
Lee, G., Sifaoui, A., and Segijn, C. M. (2023). Exploring the effect of synced brand versus competitor brand on brand attitudes and purchase intentions. J. Interact. Advert. 23, 149–165. doi: 10.1080/15252019.2023.2200777
Lee-Won, R. J., White, T. N., Song, H., Lee, J. Y., and Smith, M. R. (2020). Source magnification of cyberhate: affective and cognitive effects of multiple-source hate messages on target group members. Media Psychol. 23, 603–624. doi: 10.1080/15213269.2019.1612760
Li, H., Edwards, S. M., and Lee, J.-H. (2002). Measuring the intrusiveness of advertisements: scale development and validation. J. Advert. 31, 37–47. doi: 10.1080/00913367.2002.10673665
Main, S. (2017). Linear in a digital world: Nielsen’s ACR technology brings interactive ads to smart TVs. Adweek 58:10.
McGranaghan, M., Liaukonyte, J., and Wilbur, K. C. (2022). How viewer tuning, presence, and attention respond to ad content and predict brand search lift. Mark. Sci. 41, 873–895. doi: 10.1287/mksc.2021.1344
Mittal, B. (1995). A comparative analysis of four scales of consumer involvement. Psychol. Mark. 12, 663–682. doi: 10.1002/mar.4220120708
Myers, S. D., Deitz, G. D., Huhmann, B. A., Jha, S., and Tatara, J. H. (2020). An eye-tracking study of attention to brand-identifying content and recall of taboo advertising. J. Bus. Res. 111, 176–186. doi: 10.1016/j.jbusres.2019.08.009
Newstead, K., and Romaniuk, J. (2010). Cost per second: the relative effectiveness of 15- and 30-second television advertisements. J. Advert. Res. 50, 68–76. doi: 10.2501/s0021849910091191
Perdue, B. C., and Summers, J. O. (1986). Checking the success of manipulations in marketing experiments. J. Mark. Res. 23, 317–326. doi: 10.1177/002224378602300401
Pieters, R. G. M., and Bijmolt, T. H. A. (1997). Consumer memory for television advertising: a field study of duration, serial position, and competition effects. J. Consum. Res. 23, 362–372. doi: 10.1086/209489
Romaniuk, J., Beal, V., and Uncles, M. (2013). Achieving reach in a multi-media environment: how a marketer’s first step provides the direction for the second. J. Advert. Res. 53, 221–230. doi: 10.2501/jar-53-2-221-230
Rossiter, J. R., and Percy, L. (2017). Methodological guidelines for advertising research. J. Advert. 46, 71–82. doi: 10.1080/00913367.2016.1182088
Rossiter, J. R., Percy, L., and Bergkvist, L. (2018). Marketing communications: objectives, strategy, tactics. Los Angeles, CA: Sage
Salvucci, D. D., and Taatgen, N. A. (2008). Threaded cognition: an integrated theory of concurrent multitasking. Psychol. Rev. 115, 101–130. doi: 10.1037/0033-295x.115.1.101
Schmidt, S., and Eisend, M. (2015). Advertising repetition: a meta-analysis on effective frequency in advertising. J. Advert. 44, 415–428. doi: 10.1080/00913367.2015.1018460
Schweidel, D. A., and Moe, W. W. (2016). Binge watching and advertising. J. Mark. 80, 1–19. doi: 10.1509/jm.15.0258
Segijn, C. M. (2019). A new mobile data driven message strategy called synced advertising: conceptualization, implications, and future directions. Ann. Int. Commun. Assoc. 43, 58–77. doi: 10.1080/23808985.2019.1576020
Segijn, C. M., and Eisend, M. (2019). A meta-analysis into multi-screening and advertising effectiveness: direct effects, moderators, and underlying mechanisms. J. Advert. 48, 313–332. doi: 10.1080/00913367.2019.1604009
Segijn, C. M., and van Ooijen, I. (2022). Differences in consumer knowledge and perceptions of personalized advertising: comparing online behavioural advertising and synced advertising. J. Mark. Commun. 28, 207–226. doi: 10.1080/13527266.2020.1857297
Segijn, C. M., and Voorveld, H. A. M. (2021). A first step in unraveling synced advertising effectiveness. Int. J. Advert. 40, 124–143. doi: 10.1080/02650487.2020.1778279
Segijn, C. M., Voorveld, H. A. M., and Smit, E. G. (2016). The underlying mechanisms of multiscreening effects. J. Advert. 45, 391–402. doi: 10.1080/00913367.2016.1172386
Segijn, C. M., Voorveld, H. A. M., and Vakeel, K. A. (2021). The role of ad sequence and privacy concerns in personalized advertising: an eye-tracking study into synced advertising effects. J. Advert. 50, 320–329. doi: 10.1080/00913367.2020.1870586
Segijn, C. M., Xiong, X., and Duff, B. R. L. (2018). Manipulating and measuring media multitasking: implications of previous research and guidelines for future research. Commun. Methods Meas. 13, 83–101. doi: 10.1080/19312458.2018.1555797
Segijn, C. M., Kim, E., Sifaoui, A., and Boerman, S. C. (2023a). When you realize that big brother is watching: how informing consumers affects synced advertising effectiveness. J. Mark. Commun. 29, 317–338. doi: 10.1080/13527266.2021.2020149
Segijn, C. M., Kim, E., Lee, G., Gansen, C., and Boerman, S. C. (2023b). The intended and unintended effects of synced advertising: when persuasion knowledge can help or backfire. Int. J. Res. Mark. 41, 156–169. doi: 10.1016/j.ijresmar.2023.07.001
Sharakhina, L., Ilyina, I., Kaplun, D., Teor, T., and Kulibanova, V. (2023). AI technologies in the analysis of visual advertising messages: survey and application. J. Mark. Anal. doi: 10.1057/s41270-023-00255-1
Sharp, B., Dawes, J., and Victory, K. (2024). The market-based assets theory of brand competition. J. Retail. Consum. Serv. 76:103566. doi: 10.1016/j.jretconser.2023.103566
Sifaoui, A., Lee, G., and Segijn, C. M. (2023). “Brand match vs. mismatch and its impact on avoidance through perceived surveillance in the context of synced advertising” in Advances in advertising research. eds. A. Vignolles and M. K. Waiguny, vol. XII (Wiesbaden, Germany: European Advertising Academy/Springer Gabler), 137–147.
Simmonds, L., Bellman, S., Kennedy, R., Nenycz-Thiel, M., and Bogomolova, S. (2020). Moderating effects of prior brand usage on visual attention to video advertising and recall: an eye-tracking investigation. J. Bus. Res. 111, 241–248. doi: 10.1016/j.jbusres.2019.02.062
Singh, S. N., and Cole, C. A. (1985). Forced-choice recognition tests: a critical review. J. Advert. 14, 52–58. doi: 10.1080/00913367.1985.10672958
SMG (2014). Facebook automatic content recognition (ACR) – SMG POV. Emerging Spaces, June 3. Available at: https://web.archive.org/web/20150706092722/http://emergingspaces.co.uk/facebook-automatic-content-recognition-acr/
Snyder, J., and Garcia-Garcia, M. (2016). Advertising across platforms: conditions for multimedia campaigns. A method for determining optimal media investment and creative strategies across platforms. J. Advert. Res. 56, 352–367. doi: 10.2501/jar-2016-042
Srinivasan, S., Rutz, O. J., and Pauwels, K. (2016). Paths to and off purchase: quantifying the impact of traditional marketing and online consumer activity. J. Acad. Mark. Sci. 44, 440–453. doi: 10.1007/s11747-015-0431-z
Statista (2023a). Advertising worldwide – statistics & facts. Available at: https://www.statista.com/topics/990/global-advertising-market/#topicOverview
Statista (2023b). Second screen usage - statistics & facts. Available at: https://www.statista.com/topics/2531/second-screen-usage/#topicOverview
Statista (2023c). “Share of TV in advertising spending worldwide from 2014 to 2022.” Available at: https://www.statista.com/statistics/272939/tv-advertising-expenditure-worldwide/
Strycharz, J., and Segijn, C. M. (2024). Ethical side-effect of dataveillance in advertising: impact of data collection, trust, privacy concerns and regulatory differences on chilling effects. J. Bus. Res. 173:114490. doi: 10.1016/j.jbusres.2023.114490
Taylor, J., Kennedy, R., McDonald, C., Larguinat, L., El Ouarzazi, Y., and Haddad, N. (2013). Is the multi-platform whole more powerful than its separate parts? Measuring the sales effects of cross-media advertising. J. Advert. Res. 53, 200–211. doi: 10.2501/jar-53-2-200-211
Trifilio, E., Tanner, J. T., Butterfield, L., Mangal, P., Maye, J. E., Marsiske, M., et al. (2020). A tale of two stories: validity of an alternative story memory test in a sample of older adults. Clin. Neuropsychol. 34, 158–173. doi: 10.1080/13854046.2018.1538428
Vaughan, K., Beal, V., and Romaniuk, J. (2016). Can brand users really remember advertising more than nonusers? Testing an empirical generalization across six advertising awareness measures. J. Advert. Res. 56, 311–320. doi: 10.2501/jar-2016-037
Voorveld, H. A. M., Neijens, P. C., and Smit, E. G. (2011). Opening the black box: understanding cross-media effects. J. Mark. Commun. 17, 69–85. doi: 10.1080/13527260903160460
Voorveld, H. A. M., and Viswanathan, V. (2015). An observational study on how situational factors influence media multitasking with TV: the role of genres, dayparts, and social viewing. Media Psychol. 18, 499–526. doi: 10.1080/15213269.2013.872038
Wedel, M., and Pieters, R. (2000). Eye fixations on advertisements and memory for brands: a model and findings. Mark. Sci. 19, 297–312. doi: 10.1287/mksc.19.4.297.11794
Wright, M., Sharp, A., and Sharp, B. (2002). Market statistics for the Dirichlet model: using the Juster scale to replace panel data. Int. J. Res. Mark. 19, 81–90. doi: 10.1016/s0167-8116(02)00049-6
Zajonc, R. B. (1980). Feeling and thinking: preferences need no inferences. Am. Psychol. 35, 151–175. doi: 10.1037/0003-066x.35.2.151
Keywords: synced advertising, social media, mobile, size, timing, experiment
Citation: Brechman JM, Varan D, Wooley B and Bellman S (2024) Synced ads: effects of mobile ad size and timing. Front. Commun. 9:1343315. doi: 10.3389/fcomm.2024.1343315
Edited by:
Ashwin Aravindakshan, University of California, Davis, United StatesReviewed by:
Dušan Mladenović, Masaryk University, CzechiaPaula Rosa Lopes, Lusofona University, Portugal
Copyright © 2024 Brechman, Varan, Wooley and Bellman. This is an open-access article distributed under the terms of the Creative Commons Attribution License (CC BY). The use, distribution or reproduction in other forums is permitted, provided the original author(s) and the copyright owner(s) are credited and that the original publication in this journal is cited, in accordance with accepted academic practice. No use, distribution or reproduction is permitted which does not comply with these terms.
*Correspondence: Steven Bellman, steve.bellman@unisa.edu.au