- Department of Languages, University of Helsinki, Helsinki, Finland
This article discusses annotating and querying multimodal corpora from the perspective of Peircean semiotics. Corpora have had a significant impact on empirical research in the field of linguistics and are increasingly considered essential for multimodality research as well. I argue that Peircean semiotics can be used to gain a deeper understanding of multimodal corpora and rethink the way we work with them. I demonstrate the proposed approach in an empirical study, which uses Peircean semiotics to guide the process of querying multimodal corpora using computer vision and vector-based information retrieval. The results show that computer vision algorithms are restricted to particular domains of experience, which may be circumscribed using Peirce's theory of semiotics. However, the applicability of such algorithms may be extended using annotations, which capture aspects of meaning-making that remain beyond algorithms. Overall, the results suggest that the process of building and analysing multimodal corpora should be actively theorized in order to identify new ways of working with the information stored in them, particularly in terms of dividing the annotation tasks between humans and algorithms.
1 Introduction
Multimodality research has been characterized as a form of “applied semiotics” due to its strong orientation to data, which distinguishes the field from mainstream semiotics (Bateman and Hiippala, 2021, p. 66). This orientation may be traced back—at least partially—to the influence of linguistics, which has a long history of studying language and its use from various perspectives and at various levels of abstraction. This kind of broad engagement with language required linguistics to develop robust methodologies for taking on diverse forms of linguistic data and phenomena. Not surprisingly, the field of linguistics was among the first to expand its research interests to considering how language and other modes of communication co-operate in making and exchanging meanings—a phenomenon now conceptualized as multimodality. Bateman (2022b) argues that such an extension beyond the traditional disciplinary borders reflects the nature of multimodality as a stage of development within a discipline, a process that can bring different disciplines concerned with similar data or phenomena into contact with each other. In addition to theories and frameworks, each discipline is likely to bring its own methodologies and ways of working with data to the contact situation.
Bearing this in mind, in this article I seek to problematise certain methodological imports from linguistics to multimodality research, focusing especially on methods for building and analysing multimodal corpora. The success of corpus methods, which form a major pillar of contemporary linguistics, may be ascribed to the availability of increasingly large volumes of annotated data and powerful methods for searching this data for patterns (Bateman, 2014, p. 239). Owing to their success in linguistics, corpus methods have been proposed as being useful for the field of multimodality research as well. Whereas some approaches to corpus-driven research on multimodality draw on corpus linguistic techniques for building annotation frameworks (see e.g. the use of stand-off annotations in Bateman, 2008), others advocate for a more direct application of linguistic corpus methods (see e.g. the use of concordancers in Christiansen et al., 2020). Instead of engaging with debates on which corpus linguistic techniques may be applicable to multimodality research and for what purposes, I take a step back and consider how we secure access to information stored in multimodal corpora more generally, and how this understanding may benefit their annotation and analysis. To do so, I approach the issue by drawing on the theory of semiotics developed by Charles Sanders Peirce (see e.g. Atkin, 2023), which has been previously brought into contact with theories of multimodality (Bateman, 2018) and multimodal corpora (Allwood, 2008).
2 Corpus-driven research on multimodality
Diverse research communities that study multimodality consider annotated corpora to be essential for conducting empirical research on the phenomenon (see e.g. Allwood, 2008; Bateman, 2014; Huang, 2021). In the context of multimodality research, an annotated corpus may be broadly defined as a collection of data about communicative situations or artifacts, which has been enriched with additional information about the data that is considered relevant for the research questions being asked. This kind of ‘data about data' may range from generic metadata associated with individual entries in the corpus (such as information about author(s), date of publication, etc.) to multiple layers of cross-referenced annotations that allow combining information across annotation layers, which are needed for capturing the structure of multimodal discourse (see e.g. Bateman, 2008; Hiippala, 2015). These annotations, which are typically created using standardized markup languages such as XML or JSON, make working with the corpus tractable by allowing users to query the corpus for instances of particular annotations.
Corpus-driven empirical research has been viewed as crucial for establishing a stronger bond between theory and data in multimodality research (Bateman et al., 2004). Writing 20 years ago, Kaltenbacher (2004, p. 202) identified the lack of empiricism as a major weakness of the emerging field of study. Researchers working at that time sought to address this situation by developing annotation frameworks for multimodal corpora (Bateman et al., 2004) and linguistically-inspired concordancers for detecting patterns in transcripts of multimodal data (Baldry, 2004; Thomas, 2007) and identified challenges involved in applying corpus methods in multimodality research (Gu, 2006). More recently, Pflaeging et al. (2021, p. 3–4) have observed that empirical research on multimodality continues to be oriented toward qualitative research and small-scale studies using limited volumes of data (see also Bateman, 2022a). According to Pflaeging et al. (2021, p. 4), many multimodality researchers still hesitate to ‘scale up' and increase the volume of data for various reasons: the work may simply be at a stage of development in which large-scale studies are not yet feasible, or there might be a lack of knowledge how to pursue such analyses altogether.
Although Pflaeging et al. (2021) discuss the nature of empirical multimodality research more generally, any efforts to scale up the volume of data are likely to involve the creation of annotated corpora, as annotations are needed for securing analytical access to the data in the corpus (Bateman et al., 2004, p. 69). However, large annotated corpora have remained elusive, because applying complex annotation frameworks to multimodal data requires time, resources and expertise. Hiippala et al. (2021), for example, present a corpus of 1,000 primary school science diagrams, which are annotated for their expressive resources, compositionality and discourse structure. The annotations were created over a period of six months by five research assistants trained to apply the annotation schema, which cost approximately 50,000€ (Hiippala et al., 2021, p. 673). Given the costs and resources needed for building corpora, it is not surprising that various proposals have been put forward for improving the efficiency of building multimodal corpora. These proposals range from using computational methods for automating parts of the annotation process (Bateman et al., 2016; Hiippala, 2016; O'Halloran et al., 2018; Steen et al., 2018) to paying crowdsourced non-expert workers available on online platforms to perform the annotation tasks (Hiippala et al., 2022).
Despite the recent advances, corpus methods and their application in multimodality research remain a long way from the level of methodological maturity achieved by corpus linguistics, which has established methods for data collection and annotating and querying corpora (see e.g. Lüdeling and Kytö, 2008). In this context, however, it should be noted that multimodality research seeks to apply corpus methods to data with diverse material properties and multiple semiotic modes. Whereas corpus linguistics could exploit the linear structure of spoken and written language for developing methods such as collocation analyses and keyword-in-context queries, multimodality research regularly takes on data whose materialities vary along the dimensions of temporality, space, participant roles and transience (Bateman, 2021). In terms of materiality, compiling a corpus that describes the multimodality of static, 2D page-based documents is radically different from building a corpus of communicative situations involving face-to-face interaction, which unfold in time and are construed dynamically by their participants. These material differences define to what extent a corpus may capture the multimodal characteristics of the artifacts or situations under analysis (Gu, 2006). In addition, this material diversity has implications for developing corpus methods for multimodality research, which must account for the properties of the underlying materiality in order to make potentially meaning-bearing features accessible for analysis.
The importance of making the information stored in multimodal corpora accessible is emphasized by Bateman (2008, p. 251), who observes that:
... corpus-based research is all about searching for reoccurring patterns; the more the format of stored data can be made to support the activity of searching for patterns, then the more valuable that corpus becomes for analysis.
Arguably, the search for patterns may be supported by designing corpus annotation frameworks that adequately ‘expose' the potentially meaning-carrying dimensions of materiality for annotation and analysis. In other words, the frameworks must inherently support annotating and retrieving information about semiotic modes that may be potentially deployed on the underlying materiality. To exemplify, an annotation framework targeting audiovisual media such as film, animation, television or video games must ensure that both temporal and spatial dimensions of the materiality are made available for description, as both may carry meaningful organizations of semiotic modes (see e.g. Stamenković and Wildfeuer, 2021). Along the temporal dimension, the framework must allow segmenting the data into shots, turns, actions or other basic temporal units, whose position along the timeline may be defined using timestamps. At each point in time, the framework must also allow decomposing the spatial dimension into analytical units, whose position in the layout space may be represented using coordinates. Finally, the framework must also allow synchronizing the descriptions across these temporal and spatial “canvases” (Bateman et al., 2017, p. 87) in order to account for their coordinated use for meaning-making. As Bateman et al. (2021, p. 116) note, it is entirely natural for multimodal artifacts to exhibit structures that unfold temporally and spatially, but their joint description is not necessarily supported by contemporary annotation software (see, however, Belcavello et al., 2022).
However, ensuring that the corpus design adequately exposes the material properties of the data is only a starting point for building multimodal corpora, as it provides a foundation for developing more sophisticated annotation frameworks that pick out characteristics of the semiotic modes deployed on these materialities. The functions of such annotations may range, for example, from identifying, categorizing and describing units of analysis to annotating their interrelations (see e.g. Bateman, 2008; Stöckl and Pflaeging, 2022). On a more general level, all annotation frameworks may be treated as semiotic constructs, whose complexity depends on the kinds of phenomena that the annotation framework seeks to capture. Given that these semiotic constructs are designed and reflect properties of the underlying data, I argue that the relationship between the annotations and the underlying data warrants additional attention, as this inevitably affects our ability to retrieve information from corpora.
3 A Peircean perspective to multimodal corpora
Compared to the efforts to build larger multimodal corpora, relatively little attention has been paid to how we are able to secure any kind of access at all to the information stored in annotated corpora. One perspective to theorizing this issue may be provided by Peircean semiotics, which posits that access to “information” is mediated by signs and processes of signification (Bateman, 2018, p. 3). Allwood (2008, p. 209), who approaches multimodal corpora from a semiotic perspective, observes that multimodal corpora often feature signs that belong to three categories defined by Charles Sanders Peirce: icons, indices and symbols. He points out that static images, audiovisual moving images, sound recordings and many other forms of data stored in multimodal corpora are inherently iconic, because they bear resemblance to the original objects that they represent. According to Allwood (2008, p. 209), the iconic signs that make up a corpus may contain further indexical, iconic and symbolic signs—as exemplified by a sound recording (iconic) of human speech (symbolic). In addition, “raw” corpus data may be complemented by symbolic signs in the form of textual annotations, which can add “focus, identification and perspective” (Allwood, 2008, p. 209).
However, icons, indices and symbols cover only a part of Peirce's theory of signs (Atkin, 2023). This is why considering multimodal corpora from a semiotic perspective may benefit from Bateman's (2018) exploration of Peircean semiotics and its relationship to contemporary theories of multimodality. Bateman (2018, p. 3) emphasizes the phenomenological orientation of Peirce's theory, which focuses on the human experience and attempts to capture “the nature of what could be known” (Jappy, 2013, p. 66). In Peirce's view, signs do not reflect some pre-existing body of knowledge, but actively construe our lived experience. This orientation is evident in three categories proposed by Peirce—Firstness, Secondness and Thirdness—that provide a foundation for his theory of semiotics by carving out different ways of accessing information about the world (Bateman, 2018, p. 5). Firstness covers “independent” forms of signification, such as colors, shapes, textures and other qualities that are inherent to whatever is being interpreted. Secondness refers to forms of signification that pick out pairs of phenomena that depend on each other, as exemplified by the way smoke depends on fire. Finally, Thirdness stands for forms of signification based on conventional relations between entities, which can only be established by an external interpreter who construes a sign.
These categories are fundamental for understanding Peirce's theory of semiotics, beginning with his definition of a sign. For Peirce, a sign involves three interrelated roles that need to be fulfilled in order to know more about something: if some role remains unfulfilled, there is no sign (Bateman, 2018, p. 6). First, the sign-vehicle (or representamen) stands for whatever that acts as the source of “information”. The sign-vehicle may range from a puff of smoke or the sound of a raindrop hitting the windowsill to an utterance, a drawing or a shape. Second, the object refers to the entity picked out by the sign-vehicle. The object also places constraints on the sign-vehicle, which the sign-vehicle must meet in order to be associated with the object (Bateman, 2018, p. 6). To exemplify, a sketch of a dog (a sign-vehicle) must have certain qualities associated with dogs to be recognized as such (an object). Finally, the interpretant refers to something that the sign-user construes about the object via the sign-vehicle, which may range from mental constructs to certain feelings and dispositions (Bateman et al., 2017, p. 57).
According to Peirce, all signs necessarily fall under the category of Thirdness, as “signs do not exist in the world, they are made by interpreters” (Bateman, 2018, p. 6). This does not mean, however, that the categories of Firstness and Secondness would be irrelevant, because they provide a foundation for Peirce's first trichotomy of qualisigns, sinsigns and legisigns, which are concerned with the nature of the sign-vehicle. Qualisigns refer to inherent qualities associated with a sign-vehicle, as exemplified by color, shape, texture, etc., which fall under the category of Firstness. However, Bateman (2018, p. 7) emphasizes that according to Peirce, qualisigns cannot exist without something that actually carries these qualities, which invokes the category of Secondness: Peirce uses the term sinsign to describe sign-vehicles that carry such qualities. Finally, a legisign stands for a sign-vehicle that relies on an established convention and thus falls within the category of Thirdness. Legisigns, which operate in a ‘law-like' manner, can generate replicas of themselves, which are instantiated as sinsigns (Jappy, 2013, p. 33).
As pointed out above, the second trichotomy of icons, indices and symbols is arguably more widely known and used than the first trichotomy (cf. Allwood, 2008), although Peirce intended the trichotomies to be combined for describing how signs operate. They pick out different facets of semiosis, which need to be clearly demarcated, especially when applied to multimodal analysis (Bateman, 2018, p. 7–8). The second trichotomy is concerned with the relationship that holds between the sign-vehicle and the object (Bateman, 2018, p. 7). To begin with, icons are often understood as signs that rely on resemblance based on shared properties, although Bateman (2018, p. 4) argues that a more appropriate definition would involve treating iconicity as an “(abductive) hypothesis that a transferral of qualities makes sense”. Such hypotheses are by no means limited to visual properties. Indices, in turn, are commonly understood as being based on causation, that is, there exists a relationship between the object and the sign-vehicle, regardless whether this relation is established by some interpreter or not. In contrast to icons and indices, symbols rely on convention or agreement between sign users, which is why they constitute the least constrained form of signification (Bateman, 2018, p. 5).
Taken together, the first two trichotomies yield six distinct sign types, which are characterized by the kinds of “semiotic work” that they do (Bateman, 2018, p. 10). Here the internal logic of the framework emerges from the relationships that hold between the categories of Thirdness, Secondness and Firstness, which describe the different ways gaining information about the world. Jappy (2013, p. 70) summarizes these interrelations as follows: any instance of Thirdness (a legisign) must be supported by Secondness through a sinsign that we recognize as a replica of the legisign. No sinsign, however, can be recognized as such without having particular qualities, which fall within the domain of Firstness, as they consist of qualisigns. In other words, Thirdness implies Secondness, which in turn implies Firstness (Jappy, 2013, p. 69–70). According to Bateman (2018, p. 10), these relationships can also be understood in terms of semiotic ‘power', which defines just what kinds of “combinations of ways of being signs are licensed by the framework” proposed by Peirce.
The six sign types derived from the first two trichotomies are illustrated in Part A on the left-hand side of Figure 1 and colored according to their degree of semiotic “reach” in terms of Thirdness, Secondness and Firstness. As set out in Bateman (2018, p. 10), Thirdness (red) enables legisigns to be combined with icons, indices and symbols, whereas the Secondness (blue) of sinsigns limits them to icons and indices. Qualisigns (light brown), in turn, are limited to icons only. Note that these six sign types do not constitute a full account of Peirce's sign types, as it lacks the sign types derived from the third trichotomy, which will be discussed shortly below in connection with the example shown in Figure 1B.
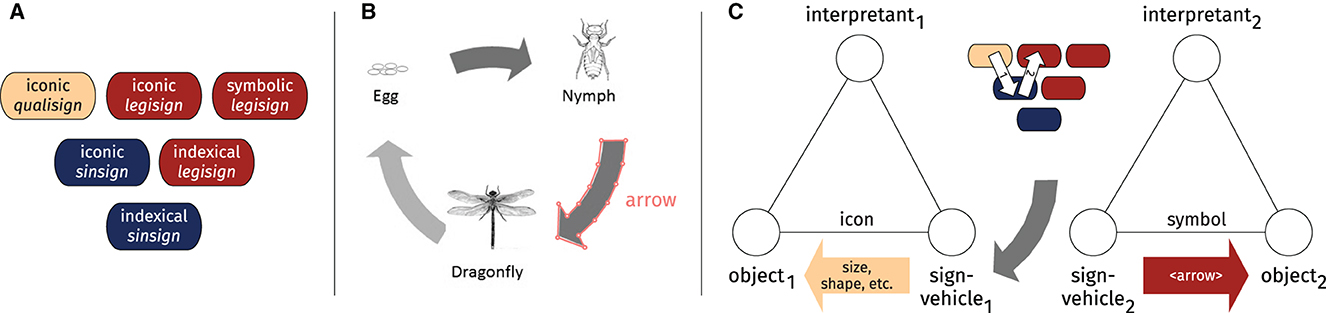
Figure 1. (A) Six distinct sign types based on the first two trichotomies in Peirce's framework. (B) Diagram #100 from the AI2D dataset (Kembhavi et al., 2016). The diagram has been converted into grayscale and annotations have been visualized for a single arrow. (C) Two signs that pick out different facets of the arrow as a sign-vehicle through iconicity.
Figure 1B shows a single diagram from the AI2D dataset, which consists of nearly 5,000 primary school science diagrams that have been annotated for their features (Kembhavi et al., 2016). The diagram, which represents the life cycle of a dragonfly, has been converted from color to grayscale to highlight the annotations. For the purpose of exemplifying the annotations, a single bounding box that surrounds one of the arrows has been drawn on top of the original diagram image. The bounding box that traces the outline of the arrow consists of a polygon, which is essentially a series of coordinate points that indicates the location of the arrow in the diagram layout. This polygon is accompanied by the textual label ‘arrow', which defines the type of the element designated by the polygon. Taken together, the polygon and the textual label represent common types of co-operating annotations found in multimodal corpora that are used to describe parts of the underlying artifact (see e.g. Bateman, 2008; Hiippala et al., 2021).
Having established the six sign types shown in Figure 1A, it is now possible to consider how they can be used to characterize aspects of the sign-making processes involved in annotating the diagram shown in Figure 1B. To do so, Figure 1C uses Peirce's tripartite model of a sign to represent two processes of signification that both pick out the element annotated as an arrow as the sign-vehicle. To begin with the sign on the left-hand side, if this element is taken as the sign-vehicle1 of an iconic qualisign, the interpretant1 construed about the element as an object1 may concern, for example, its shape and texture. However, as pointed about above, qualities such as shape and texture fall within the domain of Firstness, and thus cannot exist independently. They must be inherent to some carrier, which in this case consists of the element as an iconic sinsign (not visualized in Figure 1C). Inferences made about the combined qualities of the sign-vehicle, such as its shape and texture, may lead to the conclusion that the element possesses qualities that are consistent with an arrow. The resulting interpretant may then entail properties inferred about the arrow, such as its direction and thickness.
Acknowledging that the arrow operates as an iconic sinsign can be used to push the analysis even further by considering its function in the diagram, as illustrated by the sign on the right-hand side of Figure 1C. If the annotator recognizes the arrow (sign-vehicle2) as a diagrammatic element (object2) that stands for a process (interpretant2), then this requires treating the arrow as a replica of an iconic legisign that governs the conventionalised use of arrows and lines for representing processes and relations in diagrams (see e.g. Alikhani and Stone, 2018; Lechner, 2020b). As noted above by Jappy (2013, p. 33), legisigns are replicated using sinsigns: they stand in a relationship of instantiation that Peirce described using the terms type and token (Bateman, 2018, p. 11). From a multimodal perspective, the conventionalised use of arrows, lines and other diagrammatic elements may be collectively characterized as an expressive resource commonly deployed within the diagrammatic semiotic mode (Hiippala and Bateman, 2022a). This also resonates with the proposal put forward in Bateman (2018, p. 20), who argues that semiotic modes may be conceptualized as specific kinds of legisigns that enable attributing meaning to forms deployed on some materiality. Overall, the sign-making processes described above underline the continuous nature of semiosis (Jappy, 2013, p. 20) and how processes of signification enable a growth in knowledge (Bateman, 2018, p. 11), which is visualized in the middle of Figure 1C by movement from iconic qualisign to legisign via the sinsign.
The examples discussed above illustrate how Peircean semiotics can be used to describe the kinds of signs that may be construed about data stored in multimodal corpora. The same framework can be naturally applied to the annotations that describe the data as well. Returning to the arrow outlined in Figure 1B, the polygon stored in the corpus may be considered an indexical sinsign, which is a replica of a symbolic legisign. In this case, the symbolic legisign corresponds to a Cartesian coordinate system, which provides the mathematical and geometrical conventions needed for defining points in 2D layout space. The resulting sinsign may be treated as indexical, because its existence presumes that an annotator wanted to use the coordinate system as a symbolic legisign to demarcate a specific area of the diagram. This kind of motivated sign use is particularly important for annotations, which are assumed to reflect signs that the annotator has construed about the underlying data, as exemplified above by the arrow in Figure 1C. Some of these meanings may be captured using textual labels (Allwood, 2008, p. 209). In this case, associating the textual label ‘arrow' with the polygon involves an indexical sinsign of another symbolic legisign, namely that of the English noun “arrow”.
The way these two indexical sinsigns—the polygon and the textual label—co-operate in annotation can be described using the third Peircean trichotomy of rhemes, dicents and arguments. This trichotomy characterizes how the interpretant is shaped by the ‘view' of the object provided by the sign-vehicle (Bateman, 2018, p. 12). To begin with, a rheme refers to something that may be construed about the sign-vehicle, such as a particular quality or a characteristic, but which cannot stand on its own due to its Firstness. To exemplify, the arrow in Figure 1B may be perceived as being wide or facing downward. Rhemes may be picked up in the second category of dicents, which combines rhemes into statements: one may assert, for example, that the arrow is wide and faces down. Dicents are ‘independent' and self-standing, and thus fall within the domain of Secondness. The final category of arguments comprises of multiple dicents combined into something that the individual construing the sign can take a stance on. One could construe an argument, for example, that arrows that are used to represent processes in primary school science diagrams tend to be wider than those used to pick out parts of some depicted object. This argument may be then accepted or rejected by the interpreter.
When viewed from the perspective of the third trichotomy, the polygon and the textual label “arrow” may be treated as rhemes, as they do not make assertions independently. Whereas the interpretant of the textual label defines the type of the element in question, the corresponding interpretant of the polygon determines its location, as visualized in Figure 2A. Due to their proximity in the diagram layout, the textual label and the polygon are likely to be interpreted together. Consequently, the two rhemes may be combined in the sign-vehicle of a dicent, which asserts that the element at the location designated by the polygon belongs to the category of arrows, as shown in Figure 2B. As Bateman (2018, p. 13) points out, Peirce considered the construction of dicents to be functionally constrained: one of the rhemes picked up as a part of the sign-vehicle must function indexically, whereas the other must function iconically. This ensures that any statement or assertion made by the dicent may be verified against the “evidence” provided. In this case, the polygon functions indexically by designating the location of the element, whereas the textual label functions iconically by positing that the qualities of the element marked out by the polygon are consistent with those of an arrow.
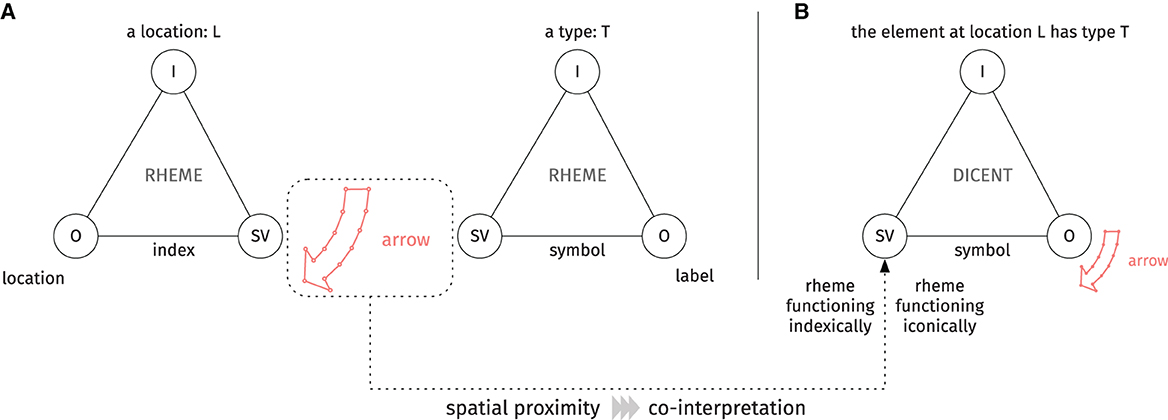
Figure 2. (A) Two rhemes construed about the polygon and the textual label. (B) Combining the two rhemes into a dicent, which asserts that the element at the location designated by the polygon is of the type determined by the textual label.
The example above illustrates how the annotations in multimodal corpora involve the co-operation of multiple sign types and shows how applying all three trichotomies can help sharpen the Peircean perspective to multimodal corpora offered in Allwood (2008). This arguably provides a deeper understanding of the semiotic underpinnings of corpus annotations, which can be used to rethink the way multimodal corpora are accessed and searched for patterns. Multimodal corpora generally rely on textual labels (rhemes) to describe the data, which are combined into dicents involving other rhemes, such as bounding boxes or timestamps. Emphasizing the role of textual labels as an access mechanism, Allwood (2008, p. 209) notes that “most existing multimodal corpora rely on textual identifying information in searching the corpus”, but Thomas (2014, p. 173) argues that “it is not always possible, nor is it necessarily productive, to describe every detail”. In particular, using textual labels to describe iconic qualities—such as size, color and shape—can prove challenging. Firstly, defining an exhaustive set of categories for systematically describing iconic qualities is likely to be difficult, and secondly, individual annotators may adopt different viewpoints to the data that are nevertheless equally valid (Gu, 2006, p. 129), which makes evaluating the reliability of the annotations difficult (see, however, Cabitza et al., 2023). This raises the question whether there are alternatives to using textual labels for accessing the information stored in multimodal corpora. As Allwood (2008) noted in 2008, “present technology mostly does not really allow efficient search using the iconic elements”, but this situation has now changed radically due to parallel work in the field of digital humanities, which has explored the use of computational methods for detecting forms with similar qualities.
4 Computer vision in digital humanities and multimodality research
The rapidly expanding field of digital humanities now regularly engages with visual or multimodal materials, which often involves combining methods developed in the fields of computer vision, natural language processing and machine learning for enriching and exploring large volumes of data (Smits and Wevers, 2023). In addition to methodological explorations that have applied specific computational techniques to different media that range from film (Heftberger, 2018) to photography (Smits and Ros, 2023) and magic lantern slides (Smits and Kestemont, 2021) to mention just a few examples, recent research has sought to couch the application of computational methods to visual and multimodal materials within broader theoretical frameworks, such as the one proposed for “distant viewing” by Arnold and Tilton (2019, 2023). These efforts have also attracted the attention of multimodality researchers, who have argued that computational approaches to multimodal data in digital humanities would benefit from input from relevant theories of multimodality, which can provide the methodological tools needed for pulling apart the diverse materialities and artifacts studied (Bateman, 2017) and annotation schemes required for contextualizing the results of computational analyses (Hiippala, 2021).
On a trajectory parallel to digital humanities, there has been growing interest in the application of computational methods in multimodality research, but the use of these methods has been largely limited to annotating and analysing multimodal corpora. Hiippala and Bateman (2022b), for example, illustrate how combining computer vision and unsupervised machine learning allows describing the diversity of visual expressive resources (e.g. line drawings, colored illustrations) in the corpus of primary school science diagrams presented in Hiippala et al. (2021). Hiippala (2023), in turn, uses the same corpus to show how unsupervised machine learning can be used to identify diagram genres that are characterized by particular multimodal discourse patterns. Computational methods have also been used for automating parts of the annotation process for page-based (Hiippala, 2016) and audiovisual media (Bateman et al., 2016; Steen et al., 2018). O'Halloran et al. (2018), in turn, propose a mixed methods framework that combines qualitative multimodal analysis with quantitative techniques for data mining, whereas Thomas (2020) outlines strategies for applying computational methods in corpus-driven approaches to multimodality.
As pointed out above, much of the computational work in multimodality research is oriented toward analysing existing corpora or automating the creation of annotations. In contrast, many researchers working within the field of digital humanities have focused on developing methods for retrieving information from large collections of visual and multimodal data, which may not be accompanied by extensive metadata or annotations commonly expected of multimodal corpora (cf., however, Arnold and Tilton, 2023). Here computer vision methods have proven especially useful, as they allow querying the data on the basis of formal properties such as texture, color and shape (Wasielewski, 2023, p. 40). One example of such an approach can be found in Lang and Ommer (2018), who show how computer vision methods can support iconographic research on visual arts, manuscripts and images. They present a system that allows the user to select an entire image or its part, which is then used for searching the dataset for visually similar occurrences. In other words, the ‘search term' consists of an instance of data with particular iconic qualities.
The methods described above, which are now finding productive applications in fields such as digital art history (Wasielewski, 2023), have their roots in content-based image retrieval, a subfield of computer vision that develops methods for searching the content of images (see e.g. Smeulders et al., 2000). Because these methods are sufficiently generic to be applied in digital art history, it may be argued that they could also be applied to querying multimodal corpora, in which textual annotations remain the main way of securing access to the data. From a semiotic perspective, this would entail a major shift: instead of querying the data for instances of rhematic indexical sinsigns (e.g. specific instances of textual labels), the search criteria could be based on rhematic iconic qualisigns construed about the object of interest. This process is facilitated by rhematic indexical sinsigns in the form of bounding boxes (polygons or rectangles) that pick out parts of the underlying data. In other words, this would allow searching the corpora for instances of data that are similar in terms of visual qualities or form, as proposed by Lang and Ommer (2018). In the following sections, I explore the potential of such methods for multimodal corpus analysis by implementing a system that allows searching an existing corpus using iconic qualities.
5 Data and methods
The data of this study consists of two interrelated corpora. The first corpus, named AI2D-RST, contains 1,000 diagrams that represent topics in primary school natural sciences (Hiippala et al., 2021). The AI2D-RST corpus is a subset of the second corpus, the Allen Institute for Artificial Intelligence Diagrams dataset (AI2D; see Kembhavi et al., 2016). Whereas AI2D was developed for supporting research on automatic processing of diagrams, AI2D-RST is intended for studying diagrams as a mode of communication (Hiippala and Bateman, 2022a). AI2D contains crowdsourced non-expert annotations for diagram elements, their interrelations and position in diagram layout, which are loosely based on the work of Engelhardt (2002). The AI2D-RST corpus enhances the crowdsourced annotations provided in AI2D with expert annotations for compositionality, or how individual diagram elements are combined into larger units; discourse structure, or what kinds of relations hold between diagram elements; and connectivity, or how arrows and lines are used to set up connections between diagram elements or their groups.
Both AI2D and AI2D-RST use element types originally defined in AI2D: (1) text elements, (2) arrows, lines and other diagrammatic elements, (3) arrowheads and (4) blobs, which is a category that includes all forms of visual representation, such as illustrations, line art, photographs, etc. (Hiippala et al., 2021, p. 665). In total, the 1,000 diagrams in AI2D-RST contain 20,094 elements categorized as text, arrows and blobs. I exclude arrowheads from the current analysis, as they simply augment the annotations for arrow elements. In addition to their type, each element is annotated for its position in the diagram layout. The coordinates for each element are represented using a polygon or a rectangle depending on the element type. The bounding boxes for blobs and arrows are represented using polygons, whereas text elements use rectangles, as illustrated in Figure 3. As such, the combinations of labels and bounding boxes constitute precisely the kinds of dicents described in Section 3 that allow retrieving information from the corpus.
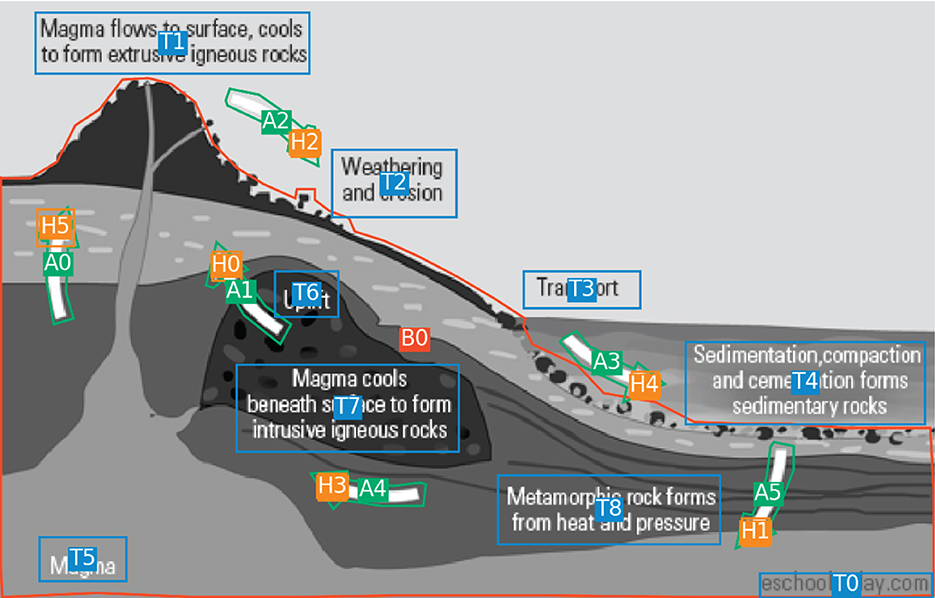
Figure 3. Diagram #4210 from the AI2D dataset. The diagram image has been converted to grayscale to highlight the crowdsourced annotations. The different elements picked out by the crowdsourced annotators are colored according to their type: text (blue), blobs (red), arrows (green) and arrowheads (orange). Each element is also accompanied by a unique identifier, e.g., T1 for the text element positioned in the upper left-hand corner. For a detailed analysis of this example and the AI2D annotation schema, see Hiippala and Bateman (2022a).
I use the information about the position of each element in the diagram layout to extract them from the diagram image and describe their visual appearance using two computer vision algorithms, which approximate two iconic qualities: texture and shape. The first algorithm is Local Binary Patterns (LBP; Ojala et al., 1996), which is implemented in the scikit-image library for Python (van der Walt et al., 2014). The LBP algorithm describes the texture of an image. The operation of the algorithm and its applications in multimodality research have been described in Hiippala and Bateman (2022b, p. 418). More specifically, I use a rotation-invariant version of LBP, which means that the algorithm produces similar descriptions for images with similar textures regardless of their orientation. The output of the LBP algorithm consists of a 26-dimensional vector—a sequence of floating point numbers—that describes the texture of the image. The second algorithm is Zernike moments, which describes the shape of an image. Zernike moments are rotation- and scale-invariant, which means that they can capture similarities among shapes regardless of their size or orientation. I use the implementation of Zernike moments provided in the mahotas library for Python (Coelho, 2013), which yields a 25-dimensional vector that represents the shape of an image.
From a Peircean perspective, computer vision algorithms for low-level feature extraction, such as Local Binary Patterns for texture or Zernike moments for shape, are inherently constrained to the categories of Firstness and Secondness. Given some input data, the algorithms can seek to approximate qualities that fall within the domain of Firstness, which are then encoded into the sequence of numbers in the output vector. The resulting vector, whose existence and properties depend on the input data, may be considered a case of Secondness, because the vectors stand in an indexical relationship to the images they describe. These rhematical indexical sinsigns may be then use to model the iconic properties encoded within them (see Bateman, 2017, p. 37–38), but they are constrained to the domains of Firstness and Secondness (see Figure 1A). As Bateman (2018, p. 10) points out, one cannot “squeeze more semiotic ‘power' out of a sign-situation than that sign-situation is configured to construe” due to the implication principle (Jappy, 2013, p. 69–70). In other words, the category of Thirdness remains beyond the reach of computer vision algorithms, as this would require an external interpreter for sign construction. This is extremely important to keep in mind when considering the capabilities of algorithms.
To store the output from the computer vision algorithms and to search for patterns, I use Milvus, an open-source vector database for storing vectors and other data types, such as textual labels, Boolean values and integers (Wang et al., 2021). Milvus allows querying the database using a vector search, which involves defining a search vector that is then matched to other vectors in the database. For this purpose, Milvus implements various metrics for measuring the similarity of vectors, including Euclidean distance or cosine similarity. For current purposes, I use cosine similarity, which measures the similarity of vectors based on their direction and magnitude. The values for cosine similarity range from 1 for identical vectors to -1 for vectors that are exactly opposite in direction. A value of 0 indicates that the vectors are perpendicular, or at a 90° angle to each other. In addition to vector search, Milvus allows conducting a hybrid search, which searches for matches using both vectors and annotations stored in the database, such as textual labels that describe the type of element or diagram in question. For this reason, I enrich the entry for each diagram element in the database with information on diagram type from both AI2D and AI2D-RST (see Hiippala and Bateman, 2022b, p. 416) and the element type (text, arrow, blob).
For reproducibility, the Python code for extracting data from the corpora, applying the computer vision algorithms and creating and querying the database is provided openly at: https://doi.org/10.5281/zenodo.10566132.
6 Analysis
To assess the potential of using computer vision and vector databases for querying multimodal corpora, I explore the use of arrows and lines as an expressive resource of the diagrammatic semiotic mode (Hiippala and Bateman, 2022a) in the AI2D-RST corpus (Hiippala et al., 2021). Previous research has shown that diagrams regularly use arrows and lines for diverse communicative functions: they can, for example, represent processes and relationships that hold between diagram elements (see e.g. Alikhani and Stone, 2018; Lechner, 2020b). Lechner (2020a, p. 118), who explores how data visualizations use connecting lines to express uncertainty, observes that the iconic qualities of arrows and lines can determine or complement their communicative functions. She identifies various potentially meaning-bearing qualities of arrows and lines, such as orientation, size, color, pattern, etc., which can be used as the basis for annotating these properties (Lechner, 2020a, p. 117). However, as pointed out in Section 3, defining an annotation schema that seeks to capture iconic qualisigns construed about arrows and lines would likely require excessive time and resources due to the number of potentially meaningful qualities and raise questions about the reliability of the annotations (Thomas, 2014, p. 173). Given that the AI2D-RST corpus does not include annotations that describe the form or qualities of individual instances of expressive resources, but simply places them into abstract categories such as text, blobs and arrows, my aim is to evaluate whether the computational methods described in Section 5 can be used to retrieve visually similar arrows and lines from the AI2D-RST corpus, thus sidestepping the need to use textual labels for describing visual qualities.
Figure 4 shows the results of a vector search among the 20,094 elements categorized as text, arrows or blobs in the AI2D-RST corpus. Each element is processed using the LBP algorithm, which yields a 26-dimensional vector that describes the texture of the element. As explicated in Section 5, Milvus compares the search vector to each vector stored in the database and returns those that are closest to the search vector in terms of cosine similarity. In this case, the element that is being searched for is a thick, colored arrow with a solid texture, which is shown in the upper left-hand corner of Figure 4 and has a cosine similarity value of 1.0, which indicates perfect similarity. As Figure 4 shows, the search results include several arrows with similar textures, but also contain numerous instances of other expressive resources, such as illustrations and written language. The diversity of the results reflects the limitations of texture, which represents only one quality that may be construed about arrows and approximated by algorithms. As the results show, texture as a quality is by no means exclusive to arrows as an expressive resource (see Djonov and van Leeuwen, 2011). This suggests that retrieving elements with specific qualities that may correspond to instances of particular expressive resources – such as arrows and lines – requires placing additional constraints on the search in terms of form.
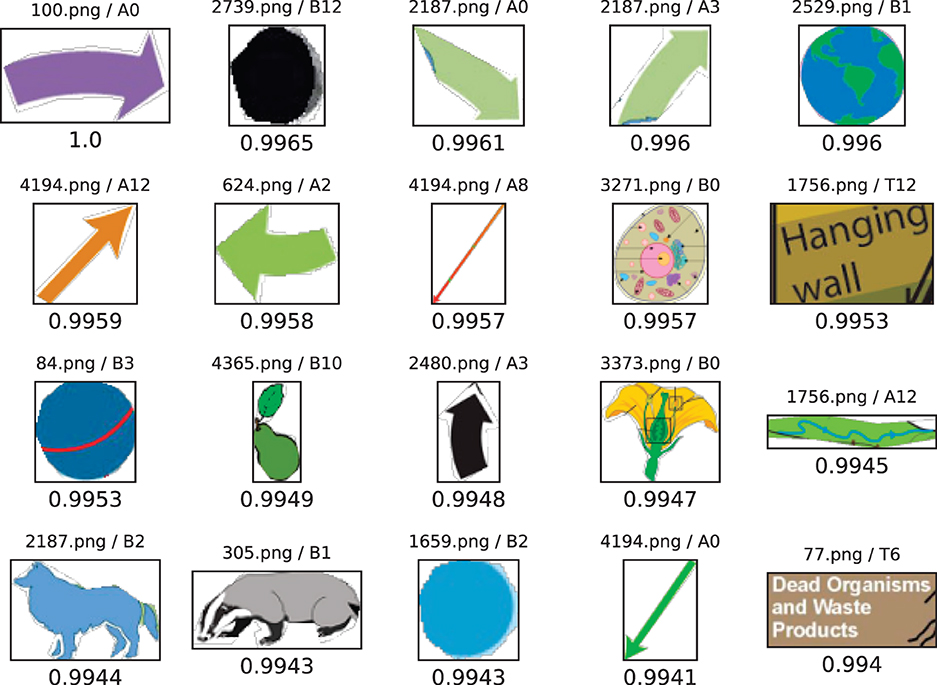
Figure 4. Results for a vector search among the 20,094 diagram elements in the AI2D-RST corpus. Each vector has 26 dimensions that store the output from the LBP algorithm. The search results are organized according to the value for cosine similarity between the result and the search vector. Values for cosine similarity are provided under each thumbnail image of the diagram element. The element in the top left-hand corner is the element searched for, as indicated by a perfect cosine similarity value of 1.0. The name of the diagram image and the unique identifier for each element are given above the thumbnail.
To this end, Figure 5 combines the 26-dimensional vector for LBP that describes the texture of an element with a 25-dimensional vector for Zernike moments, which describes its shape. This combination yields a 51-dimensional vector for each element, which jointly encodes information about both texture and shape. As the results of the query show, combining LBP and Zernike moments yields somewhat different search results than those shown in Figure 4. Just like above, the results are not limited to arrows and lines, but also include instances of other expressive resources, which the computer vision algorithms perceive as having similar visual qualities. This illustrates a challenge that Thomas (2020, p. 84) discusses in relation to supporting empirical research on multimodality using computational methods, which involves moving beyond low-level “regularities of form” and toward higher levels of abstraction. Thomas (2020, p. 84) characterizes this transition from a Peircean perspective as a move from iconic qualisigns to iconic legisigns. As Figure 5 shows, approximating just some iconic qualities that may be attributed to arrows, such as texture and shape, are not sufficient for identifying indexical sinsigns that could be potentially ascribed to the diagrammatic semiotic mode.
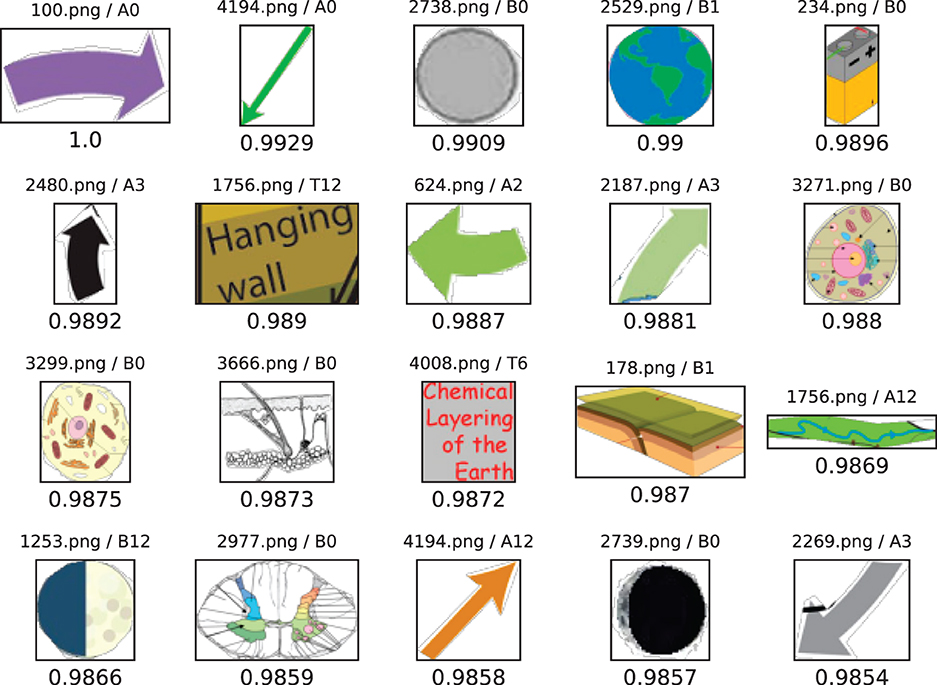
Figure 5. Results of a vector search among the 20,094 diagram elements in the AI2D-RST corpus. Each vector has 51 dimensions that combine the output from the LBP algorithm and Zernike moments. For information on interpreting the figure, see the caption for Figure 4.
In light of the results shown in Figures 4, 5, it should be emphasized that computer vision algorithms, such as LBP or Zernike moments, are inherently restricted to the domains of Firstness and Secondness, as pointed out in Section 5. Unlike humans, computer vision algorithms are not capable of the kind of continuous semiosis that enables the ‘growth' of information (see Figure 1C). This kind of growth, which could entail a move to the domain of Thirdness, would be needed to recognize arrows as indexical sinsigns (replicas) generated by the diagrammatic semiotic mode (Hiippala and Bateman, 2022a). As a particular type of symbolic legisigns—highly conventionalised practices of manipulating materialities for communicative purposes that emerge within communities of users – semiotic modes fall within the domain of Thirdness (Bateman, 2018, p. 20). Because Thirdness remains beyond the reach of algorithms, mapping low-level regularities of form to more abstract categories needs to be supported by annotations, as noted by Thomas (2020, p. 84), in order to bridge what could be conceptualized as the “semiotic gap” (cf. Smeulders et al., 2000). From a Peircean perspective, the annotations needed for this purpose consist of textual labels and bounding boxes, which constitute rhemes that may be combined into a dicent that determines the type of the object at a given location (see Figure 2). In this case, a rhematic indexical sinsign—a replica of the English lexeme “arrow” as a symbolic legisign—provides sufficient “focus, identification and perspective” (Allwood, 2008, p. 209) for recovering indexical sinsigns that may be attributed to the diagrammatic mode.
In this way, the information provided by annotations enables shifting the direction of analysis from Thirdness toward Firstness (see Bateman, 2018, p. 11). Put differently, the annotations enable recovering information that remains unavailable to algorithms and limits their role to the domain of Firstness, that is, to describing iconic qualities. This approach may be implemented in a hybrid search, which combines a vector search with additional categorical or numerical information. Figure 6 shows the results for a hybrid search, which compares the search vector consisting of LBP and Zernike moments to all other vectors in the database, but constrains the search to elements that have been annotated as arrows in the AI2D-RST corpus. As the results show, a hybrid search is able to retrieve arrows with similar iconic qualities in terms of texture and shape, regardless of their size or orientation. This is a notable result, as the application of these algorithms allows sidestepping the annotation of iconic qualities, which can consume excessive time and resources.
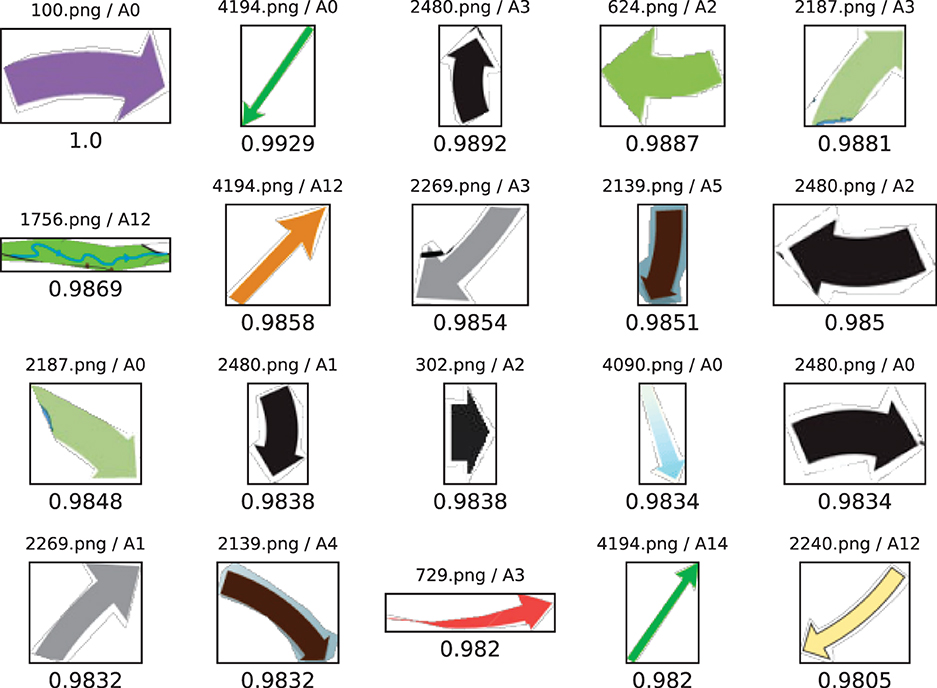
Figure 6. Results of a vector search among the 20,094 diagram elements in the AI2D-RST corpus. Each vector has 51 dimensions that combine the output from the LBP algorithm and Zernike moments. The output is constrained to elements that have been categorized as arrows in the AI2D-RST corpus. For information on how to interpret the results, see the caption for Figure 4.
However, using a hybrid search to retrieve arrows with similar iconic qualities raises questions about quantifying the results, which is a common goal of pursuing corpus-driven analyses. Whereas annotations based on discrete labels can be counted and then subjected to statistical analyses, the results of a vector search are based on a continuous measure, in this case that of cosine similarity, which approximates their visual similarity. It is, however, possible to estimate the degree of similarity between the search vector and other vectors in the database. Figure 7 plots the cosine similarity values between the search vector and (1) all arrows in the AI2D-RST corpus and (2) arrows in diagrams that have been categorized either as cycles or cut-outs (Hiippala et al., 2021, p. 668). As these plots show, the distributions of cosine similarity values do not enable visually identifying a cut-off point that could be used to determine which arrows are considered sufficiently similar to the one being searched for. However, potential differences in the distributions under different conditions can be evaluated statistically. In this case, a Mann-Whitney U-test indicates a statistically significant difference with a medium effect size between the samples for cycles and cut-outs (U = 351359, p = < 0.00, Cliff's d = 0.369), which suggests that these diagrams use arrows with different visual qualities.
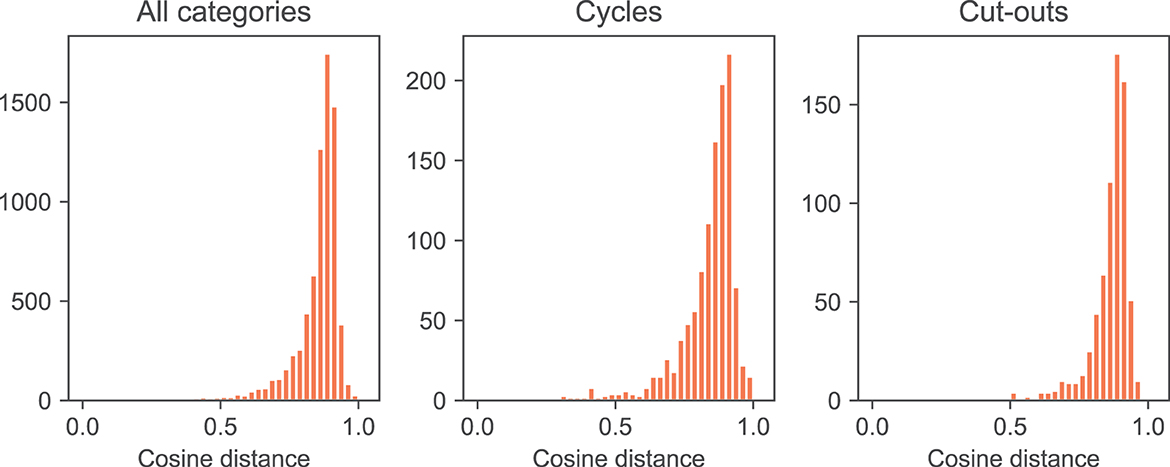
Figure 7. Cosine distances between the search vector and all other vectors in the database for all diagram types in the AI2D-RST dataset and cycle and cut-out diagrams (see Hiippala et al., 2021, p. 668).
When quantifying differences between iconic qualities with the help of computer vision and measures such as cosine similarity, one must naturally also consider the characteristics of the data in the corpus. Previous research has shown that cut-out diagrams are characterized by relatively stable layout patterns in which the depicted object is placed in the center of the layout, whereas the parts of the object are picked out using lines and written labels (Hiippala and Bateman, 2022b; Hiippala, 2023). Given that cut-out diagrams use lines to represent part-whole structures, it may be assumed that they would prefer to use thinner arrows and lines than cycles, which use these elements to represent processes and other phenomena (Lechner, 2020b). However, conducting a hybrid search for arrows among cut-out diagrams by using the same element as in Figure 6 returns mixed results, which are shown in Figure 8.
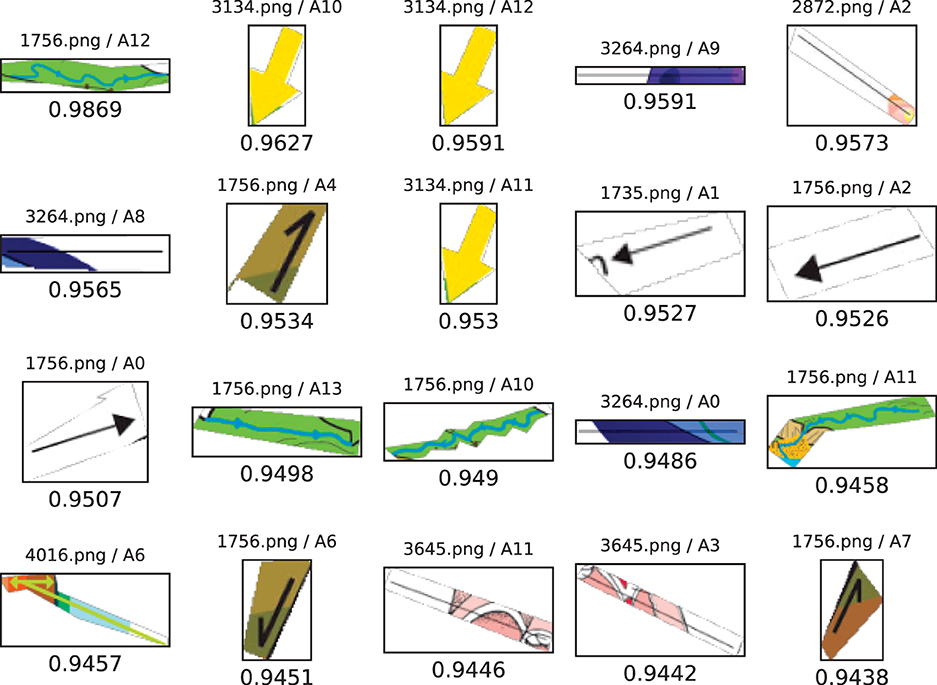
Figure 8. Results of a vector search among the 20,094 diagram elements in the AI2D-RST corpus. Each vector has 51 dimensions that combine the output from the LBP algorithm and Zernike moments. The output is constrained to elements that have been annotated as arrows in the AI2D-RST corpus, which occur in diagrams classified as cut-outs. For information on how to interpret the results, see the caption for Figure 4.
The results show that cut-out diagrams do feature some wide arrows with solid texture, but many of the arrows returned by the vector search are indeed thinner, yet the algorithm considers them similar to the one that is being searched for. This may be traced back to inaccurate bounding boxes drawn by crowdsourced workers who annotated the data for the AI2D dataset (Kembhavi et al., 2016), which do not only include the arrow, but also cover parts of their immediate surroundings in the diagram. In other words, “thinness” is a quality that is difficult to capture using polygons, but which also affects the results of a vector search. These surrounding areas may feature various shapes and textures, as illustrated by the examples in Figure 8. The gray area shows the extent of the bounding box: everything within the bounding box is provided as input to the computer vision algorithms, which results in “noise” that is encoded into the resulting vector representations. This also raises questions about the differences in the distribution of cosine distances in Figure 7, as capturing the property of thinness more accurately might make the differences between cut-outs and cycles more pronounced. To summarize, annotation quality has a significant impact on the applicability of computer vision and vector search methods for querying multimodal corpora.
7 Discussion
The results suggest that considering multimodal corpora from the perspective of Peircean semiotics benefits from a more comprehensive account that extends beyond the trichotomy of icons, indices and symbols (cf. Allwood, 2008). By providing a deeper understanding of corpus annotation frameworks as semiotic constructs that involve diverse types of signs, Peircean semiotics can be used to evaluate in what ways particular types of annotations are able to support access to the information stored in corpora. In particular, the results in Section 6 underline the importance of annotations as dicent indexical sinsigns that not only secure access to the data, but which can also constrain the operation of computer vision algorithms that operate on the elements designated by the annotations (see Figure 2). This information may be particularly useful for dividing the labor involved in annotating multimodal corpora. Although textual labels play a crucial role in securing access to the information stored in multimodal corpora, they may be less useful for describing iconic qualities, as many kinds of iconic qualisigns can be construed about the underlying data (Thomas, 2014, p. 173). This is precisely where computer vision methods may prove particularly useful.
From a Peircean perspective, the application of computer vision algorithms that approximate the qualities of forms present on some materiality is necessarily constrained to the domain of Firstness. Whereas computer vision algorithms can approximate iconic qualities of the data and encode this information into numerical representations (a Second), the domain of Thirdness, which is a prerequisite for signification, remains beyond their reach. Nevertheless, the results show that computer vision algorithms can estimate iconic qualities of the underlying data when supported by annotations that constrain the search by providing information pertaining to the domain of Thirdness. Essentially, the annotations capture aspects of the signs that the human annotators have construed about the instances of data stored in the corpus. Although this process may be mimicked e.g., by training machine learning models to detect objects and predict labels associated with them, it should be noted that predicting a textual label for some entity—which is essentially a rhematic indexical sinsign—is an extremely constrained form of Secondness that does not enable the growth of information commonly attributed to semiosis, which may be considered a capability unique to humans. As such, the capabilities of such models with respect to processing visual and multimodal data should not be overestimated (cf. Arnold and Tilton, 2023). This also raises the question of how to collect high-level information pertaining to Thirdness at scale—using crowdsourced non-expert annotators available on crowdsourcing platforms presents one possible alternative (see Hiippala et al., 2022).
In terms of methodology, vector representations appear to hold much potential for supporting access to the information stored in multimodal corpora. As demonstrated in Section 6, hybrid searches may prove particularly useful, as they allow combining low-level regularities of form captured by the vectors with higher-level information in the form of categorical labels, which has been identified as a key challenge in applying computational methods in multimodality research (Thomas, 2020, p. 84). It may also be argued that complementing traditional searches over annotated data with a vector search increases our capability to search multimodal corpora for patterns (Bateman, 2008, p. 251). However, whether a vector search is able to return results relevant to a query depends on the extent to which the algorithms used for creating the vectors are able to encode the properties of the data under analysis. This is especially important for multimodality research, as the search for patterns may not necessarily target particular kinds of objects, but rather attempts to retrieve instances of specific expressive resources such as written language, colored illustrations, line drawings, etc. As these expressive resources are characterized by particular forms, “traditional” computer vision algorithms based on human-designed heuristics, such as LBP or Zernike moments, may prove more useful than contemporary approaches involving deep neural networks (see e.g., Smits and Wevers, 2023), as these algorithms explicitly target formal properties such as shape or texture, and are less sensitive to rotation- and scale-invariance. It should also be noted that applying similar techniques to audiovisual data is likely to require different solutions (see e.g., Bateman et al., 2016).
For the design of multimodal corpora, the results suggest that additional attention should be paid to ensuring that the corpora support various means of access to the information stored therein, as this facilitates the search for patterns and thus makes the corpora more valuable for research (Bateman, 2008, p. 251). This means that rather than seeking maximum coverage in terms of annotation layers that rely on complex constellations of categories defined using textual labels, corpus design should carefully consider how different types of annotations interact with each other and what kind of information they provide access to. To exemplify, the results presented in Section 6 illustrate how polygons enable using computer vision to effectively extract information about the form of expressive resources directly from the corpus data, but this information only becomes usable when supported by textual labels that “add to, supplement and complement” the information made accessible by bounding boxes (Allwood, 2008, p. 209). Furthermore, the results underline that the quality of annotations remains of great importance: in addition to evaluating the reliability of analytical categories introduced by annotation schemas (Pflaeging et al., 2021, p. 21–22), one must ensure that the bounding boxes used to demarcate objects in the data are accurate, if computer vision methods are to be used for their analysis.
8 Conclusion
In this article, I have examined multimodal corpora from the perspective of Peircean semiotics. I have argued that Peircean semiotics can provide new perspectives on how multimodal corpora support access to the information stored in them. These perspectives are particularly valuable for designing, building and analysing multimodal corpora, as they help to determine what kinds of descriptions are needed for capturing processes of meaning-making in communicative situations and artifacts. Given that creating multimodal corpora consumes excessive time and resources, Peircean semiotics can also be used to inform the division of labor between humans and computers. This kind of input from semiotics will be crucial as multimodal corpora begin to be extended to increasingly complex communicative situations and artifacts. This calls for increased efforts in theorizing the development and use of corpora in multimodality research, rather than considering corpus methods simply as a part of the methodological toolkit carried over from linguistics.
Data availability statement
The original contributions presented in the study are included in the article/supplementary material, further inquiries can be directed to the corresponding author.
Author contributions
TH: Conceptualization, Data curation, Formal analysis, Funding acquisition, Software, Visualization, Writing – original draft.
Funding
The author(s) declare financial support was received for the research, authorship, and/or publication of this article. This research was funded by a University of Helsinki three-year grant to the author.
Acknowledgments
This article has benefitted considerably from discussions with John A. Bateman in the immediate past and over many years. Any errors or misrepresentations remain my responsibility.
Conflict of interest
The author declares that the research was conducted in the absence of any commercial or financial relationships that could be construed as a potential conflict of interest.
Publisher's note
All claims expressed in this article are solely those of the authors and do not necessarily represent those of their affiliated organizations, or those of the publisher, the editors and the reviewers. Any product that may be evaluated in this article, or claim that may be made by its manufacturer, is not guaranteed or endorsed by the publisher.
References
Alikhani, M., and Stone, M. (2018). “Arrows are the verbs of diagrams,” in Proceedings of the 27th International Conference on Computational Linguistics (Santa Fe, New Mexico: International Conference on Computational Linguistics), 3552–3563.
Allwood, J. (2008). “Multimodal corpora,” in Corpus Linguistics: An International Handbook, eds. A. Lüdeling and M. Kytö (Berlin: Mouton de Gruyter), 207–225.
Arnold, T., and Tilton, L. (2019). Distant viewing: analyzing large visual corpora. Digital Scholars. Human. 34, i3–i16. doi: 10.1093/llc/fqz013
Arnold, T., and Tilton, L. (2023). Distant Viewing: Computational Exploration of Digital Images. Cambridge, MA: MIT Press.
Atkin, A. (2023). “Peirce's theory of signs,” in The Stanford Encyclopedia of Philosophy, eds. E. N. Zalta and U. Nodelman (Stanford: Metaphysics Research Lab, Stanford University).
Baldry, A. (2004). “Phase and transition, type and instance: patterns in media texts as seen through a multimodal concordancer,” in Multimodal Discourse Analysis: Systemic Functional Perspectives, ed. K. L. O'Halloran (London: Continuum), 83–108.
Bateman, J. A. (2008). Multimodality and Genre: A Foundation for the Systematic Analysis of Multimodal Documents. London: Palgrave Macmillan.
Bateman, J. A. (2014). “Using multimodal corpora for empirical research,” in The Routledge Handbook of Multimodal Analysis, ed. C. Jewitt (London and New York: Routledge). second edn. 238–252.
Bateman, J. A. (2018). Peircean semiotics and multimodality: towards a new synthesis. Multimodal Commun. 7:21. doi: 10.1515/mc-2017-0021
Bateman, J. A. (2021). “Dimensions of materiality: towards an external language of description for empirical multimodality research,” in Empirical Multimodality Research: Methods, Evaluations, Implications, eds. J. Pflaeging, J. Wildfeuer, and J. A. Bateman (Berlin and Boston: De Gruyter), 35–64.
Bateman, J. A. (2022). Growing theory for practice: empirical multimodality beyond the case study. Multimodal Commun. 11, 63–74. doi: 10.1515/mc-2021-0006
Bateman, J. A. (2022). Multimodality, where next? Some meta-methodological considerations. Multimod. Soc. 2, 41–63. doi: 10.1177/26349795211073043
Bateman, J. A., Delin, J. L., and Henschel, R. (2004). “Multimodality and empiricism: preparing for a corpus-based approach to the study of multimodal meaning-making,” in Perspectives on Multimodality, eds. E. Ventola, C. Charles, and M. Kaltenbacher (Amsterdam: Benjamins), 65–89.
Bateman, J. A., and Hiippala, T. (2021). “From data to patterns: on the role of models in empirical multimodality research,” in Empirical Multimodality Research: Methods, Evaluations, Implications, eds. J. Pflaeging, J. Wildfeuer, and J. A. Bateman (Berlin and Boston: De Gruyter), 65–90.
Bateman, J. A., Thiele, L., and Akin, H. (2021). Explanation videos unravelled: breaking the waves. J. Pragmat. 175, 112–128. doi: 10.1016/j.pragma.2020.12.009
Bateman, J. A., Tseng, C., Seizov, O., Jacobs, A., Lüdtke, A., Müller, M. G., et al. (2016). Towards next generation visual archives: image, film and discourse. Visual Stud. 31, 131–154. doi: 10.1080/1472586X.2016.1173892
Bateman, J. A., Wildfeuer, J., and Hiippala, T. (2017). Multimodality: Foundations, Research and Analysis-A Problem-Oriented Introduction. Berlin: De Gruyter Mouton.
Bateman, J. A. (2017). Multimodale Semiotik und die theoretischen Grundlagen der Digital Humanities. Zeitschrift für Semiotik 39, 11–50.
Belcavello, F., Viridiano, M., Matos, E., and Timponi Torrent, T. (2022). “Charon: A FrameNet annotation tool for multimodal corpora,” in Proceedings of the 16th Linguistic Annotation Workshop (LAW-XVI), eds. S. Pradhan and S. Kuebler (Marseille, France: European Language Resources Association), 91–96.
Cabitza, F., Campagner, A., and Basile, V. (2023). Toward a perspectivist turn in ground truthing for predictive computing. Proc. AAAI Conf. Artif. Intell. 37, 6860–6868. doi: 10.1609/aaai.v37i6.25840
Christiansen, A., Dance, W., and Wild, A. (2020). “Constructing corpora from images and text: an introduction to visual constituent analysis,” in Corpus Approaches to Social Media, eds. S. Rüdiger and D. Dayter (Amsterdam: Benjamins), 149–174.
Coelho, L. P. (2013). Mahotas: Open source software for scriptable computer vision. J. Open Res. Softw. 1:e3. doi: 10.5334/jors.ac
Djonov, E. N., and van Leeuwen, T. (2011). The semiotics of texture: from tactile to visual. Visual Commun. 10, 541–564. doi: 10.1177/1470357211415786
Engelhardt, Y. (2002). The Language of Graphics: A Framework for the Analysis of Syntax and Meaning in Maps, Charts and Diagrams (Ph.D. thesis) Amsterdam: Institute for Logic, Language and Computation, University of Amsterdam.
Gu, Y. (2006). Multimodal text analysis: a corpus linguistic approach to situated discourse. Text & Talk 26, 127–167. doi: 10.1515/TEXT.2006.007
Heftberger, A. (2018). Digital Humanities and Film Studies: Visualising Dziga Vertov's Work. Cham: Springer.
Hiippala, T. (2015). The Structure of Multimodal Documents: An Empirical Approach. New York and London: Routledge.
Hiippala, T. (2016). “Semi-automated annotation of page-based documents within the Genre and Multimodality framework,” in Proceedings of the 10th SIGHUM Workshop on Language Technology for Cultural Heritage, Social Sciences, and Humanities (Berlin, Germany: Association for Computational Linguistics), 84–89.
Hiippala, T. (2021). Distant viewing and multimodality research: prospects and challenges. Multimod. Soc. 1, 134–152. doi: 10.1177/26349795211007094
Hiippala, T., Alikhani, M., Haverinen, J., Kalliokoski, T., Logacheva, E., Orekhova, S., et al. (2021). AI2D-RST: a multimodal corpus of 1000 primary school science diagrams. Lang. Resour. Evaluat. 55, 661–688. doi: 10.1007/s10579-020-09517-1
Hiippala, T., and Bateman, J. A. (2022). “Introducing the diagrammatic semiotic mode,” in Diagrammatic Representation and Inference: 13th International Conference (Diagrams 2022), eds. V. Giardino, S. Linker, R. Burns, F. Bellucci, J.-M. Boucheix, and P. Viana (Cham: Springer), 3–19.
Hiippala, T., and Bateman, J. A. (2022). Semiotically-grounded distant view of diagrams: insights from two multimodal corpora. Digit. Scholars. Human. 37, 405–425. doi: 10.1093/llc/fqab063
Hiippala, T., Hotti, H., and Suviranta, R. (2022). “Developing a tool for fair and reproducible use of paid crowdsourcing in the digital humanities,” in Proceedings of the 6th Joint SIGHUM Workshop on Computational Linguistics for Cultural Heritage, Social Sciences, Humanities and Literature (Gyeongju, Republic of Korea: International Conference on Computational Linguistics), 7–12.
Hiippala, T. (2023). Corpus-based insights into multimodality and genre in primary school science diagrams. Visual Commun. doi: 10.1177/14703572231161829
Huang, L. (2021). Toward multimodal corpus pragmatics: Rationale, case, and agenda. Digit. Scholars. Human. 36, 101–114. doi: 10.1093/llc/fqz080
Kaltenbacher, M. (2004). Perspectives on multimodality: from the early beginnings to the state of the art. Inform. Design J. 12, 190–207. doi: 10.1075/idjdd.12.3.05kal
Kembhavi, A., Salvato, M., Kolve, E., Seo, M. J., Hajishirzi, H., and Farhadi, A. (2016). “A diagram is worth a dozen images,” in Proceedings of the 14th European Conference on Computer Vision (ECCV 2016) (Cham: Springer), 235–251.
Lang, S., and Ommer, B. (2018). Attesting similarity: supporting the organization and study of art image collections with computer vision. Digit. Scholars. Human. 33, 845–856. doi: 10.1093/llc/fqy006
Lechner, V. E. (2020a). “Modality and uncertainty in data visualizations: a corpus approach to the use of connecting lines,” in Diagrammatic Representation and Inference: 11th International Conference (Diagrams 2020), eds. A.-V. Pietarinen, P. Chapman, L. B. de Smet, V. Giardino, J. Corter, and S. Linker (Cham: Springer), 110–127.
Lechner, V. E. (2020b). “What a line can say: Investigating the semiotic potential of the connecting line in data visualizations,” in Data Visualization in Society, eds. H. Kennedy and M. Engebretsen (Amsterdam: Amsterdam University Press), 329–346.
Lüdeling A. and Kytö, M. (2008). Corpus Linguistics: An International Handbook. Berlin: Mouton de Gruyter.
O'Halloran, K. L., Tan, S., Pham, D.-S., Bateman, J. A., and Vande Moere, A. (2018). A digital mixed methods research design: integrating multimodal analysis with data mining and information visualization for big data analytics. J. Mixed Methods Res. 12, 11–30. doi: 10.1177/1558689816651015
Ojala, T., Pietikäinen, M., and Harwood, D. (1996). A comparative study of texture measures with classification based on featured distributions. Pattern Recognition 29, 51–59. doi: 10.1016/0031-3203(95)00067-4
Pflaeging, J., Bateman, J. A., and Wildfeuer, J. (2021). “Empirical multimodality research: the state of play,” in Empirical Multimodality Research: Methods, Applications, Implications, eds. J. Pflaeging, J. Wildfeuer, and J. A. Bateman (Berlin and Boston: De Gruyter), 3–32.
Smeulders, A. W. M., Worring, M., Santini, S., Gupta, A., and Jain, R. (2000). Content-based image retrieval at the end of the early years. IEEE Trans. Pattern Analy. Mach. Intell. 22, 1349–1380. doi: 10.1109/34.895972
Smits, T., and Kestemont, M. (2021). “Towards multimodal computational humanities: using CLIP to analyze late-nineteenth century magic lantern slides,” in Proceedings of the Computational Humanities Research Conference (CHR 2021), 149–158.
Smits, T., and Ros, R. (2023). Distant reading 940,000 online circulations of 26 iconic photographs. New Media Soc. 25, 3543–3572. doi: 10.1177/14614448211049459
Smits, T., and Wevers, M. (2023). A multimodal turn in digital humanities: Using contrastive machine learning models to explore, enrich, and analyze digital visual historical collections. Digit. Scholars. Human. 38, 1267–1280. doi: 10.1093/llc/fqad008
Stamenković, D., and Wildfeuer, J. (2021). “An empirical multimodal approach to open-world video games: a case study of Grand Theft Auto V,” in Empirical Multimodality Research: Methods, Evaluations, Implications, eds. J. Pflaeging, J. Wildfeuer, and J. A. Bateman (Berlin and Boston: De Gruyter), 259–279.
Steen, F. F., Hougaard, A., Joo, J., Olza, I., Cánovas, C. P., Pleshakova, A., et al. (2018). Toward an infrastructure for data-driven multimodal communication research. Linguistics Vanguard 4. doi: 10.1515/lingvan-2017-0041
Stöckl, H., and Pflaeging, J. (2022). Multimodal coherence revisited: notes on the move from theory to data in annotating print advertisements. Front. Commun. 7. doi: 10.3389/fcomm.2022.900994
Thomas, M. (2007). “Querying multimodal annotation: a concordancer for GeM,” in Proceedings of the Linguistic Annotation Workshop (LAW 2007) (Prague, Czech Republic: Association for Computational Linguistics), 57–60.
Thomas, M. (2014). Evidence and circularity in multimodal discourse analysis. Visual Commun. 13, 163–189. doi: 10.1177/1470357213516725
Thomas, M. (2020). “Making a virtue of material values: tactical and strategic benefits for scaling multimodal analysis,” in Multimodality: Disciplinary Thoughts and the Challenge of Diversity, eds. J. Wildfeuer, J. Pflaeging, J. A. Bateman, O. Seizov, and C. Tseng (Berlin: De Gruyter), 69–91.
van der Walt, S., Schönberger, J. L., Nunez-Iglesias, J., Boulogne, F., Warner, J. D., Yager, N., et al. (2014). scikit-image: image processing in Python. PeerJ 2, 453. doi: 10.7717/peerj.453
Wang, J., Yi, X., Guo, R., Jin, H., Xu, P., Li, S., et al. (2021). “Milvus: a purpose-built vector data management system,” in Proceedings of the 2021 International Conference on Management of Data (New York, NY, USA: Association for Computing Machinery), 2614–2627.
Keywords: multimodality, corpora, vector search, computer vision, Peirce, semiotics
Citation: Hiippala T (2024) Rethinking multimodal corpora from the perspective of Peircean semiotics. Front. Commun. 9:1337434. doi: 10.3389/fcomm.2024.1337434
Received: 13 November 2023; Accepted: 29 January 2024;
Published: 12 February 2024.
Edited by:
Claudia Lehmann, University of Potsdam, GermanyReviewed by:
Tiago Timponi Torrent, Federal University of Juiz de Fora, BrazilAlin Olteanu, RWTH Aachen University, Germany
Copyright © 2024 Hiippala. This is an open-access article distributed under the terms of the Creative Commons Attribution License (CC BY). The use, distribution or reproduction in other forums is permitted, provided the original author(s) and the copyright owner(s) are credited and that the original publication in this journal is cited, in accordance with accepted academic practice. No use, distribution or reproduction is permitted which does not comply with these terms.
*Correspondence: Tuomo Hiippala, tuomo.hiippala@helsinki.fi