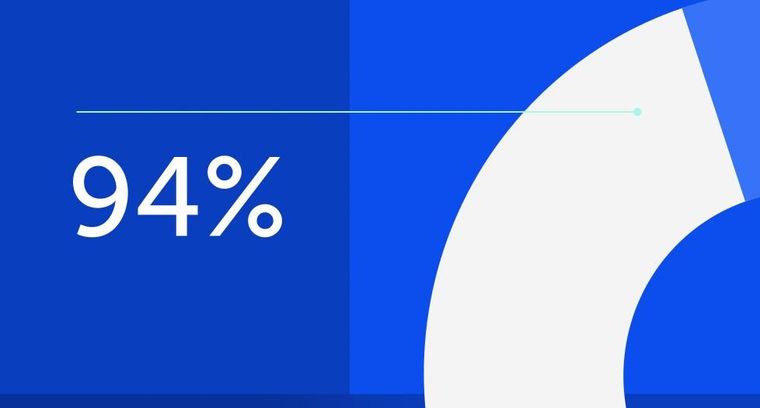
94% of researchers rate our articles as excellent or good
Learn more about the work of our research integrity team to safeguard the quality of each article we publish.
Find out more
ORIGINAL RESEARCH article
Front. Commun., 11 April 2024
Sec. Health Communication
Volume 9 - 2024 | https://doi.org/10.3389/fcomm.2024.1292792
This article is part of the Research TopicExamining Upstream to Understand Downstream: Use of Telehealth and Other Health Equity Measures for Addressing Health DisparitiesView all 8 articles
Introduction: The effectiveness of telehealth strategies toward support for adequate hospital visits is vital. We examined whether individuals who received advice from a physician via an online application subsequently visited hospitals. Further, we examined the background factors associated with their hospital visit behavior.
Methods: We used machine learning to examine whether chief complaint, medical advice, and user background characteristics could be used to predict their subsequent hospital visit.
Results: Among 7,152 participants, those in their 30s were the most frequent users. The proportion of each medical advice was significantly different between the group that did and the one that did not follow physicians’ advice. We further performed supervised machine learning using random forest modeling to categorize those who (1) followed physicians’ advice or (2) did not follow physicians’ advice. The area under the receiver operating characteristic curve was 0.677. Consequently, the aforementioned model soundly categorized whether users followed physicians’ advice. Chief complaint and medical advice were the most important variables to predict whether users followed the advice.
Discussion: The telehealth system to provide support for adequate hospital visits influenced patients’ subsequent hospital visit behavior. Patients’ chief complaint was the most important variable in discriminating whether users followed physicians’ advice.
Disparities in the healthcare system arise from various individual and social system factors. It is vital to discuss issues such as the distribution of limited healthcare resources to reduce these disparities (Solnica et al., 2020). In particular, providing patients with support for adequate hospital visiting is vital for both the ranking regarding prioritization and allocation of limited medical resources (Kucewicz-Czech and Damps, 2020). Therefore, promoting adequate patient behavior toward hospital visits is a crucial public health issue to promote the appropriate use of limited medical resources.
Various strategies to promote appropriate patient hospital visit behavior have been undertaken. In particular, telehealth, which provides health services including consultative and diagnostic services via telecommunication, was widely used to improve workforce capacity and patient accessibility to healthcare during the COVID-19 pandemic. Telehealth provides support for adequate hospital visiting (Hollander and Carr, 2020; Sivarajasingam, 2021). In fact, several studies have reported that an online strategy provided effective support for adequate hospital visiting for emergency patients and patients with burns, heart failure, and cervical cancer (Arrossi et al., 2019; Carmichael et al., 2020; Chai et al., 2021; Beyer et al., 2022; Morrill et al., 2022). A study that conducted telemedicine screening assessments on four diagnostic cohorts—patients with gastroenteritis, psychiatric conditions, burn, or fractures—reported that the median door-to-provider times were reduced through telemedicine screening (Friedman et al., 2021). Hence, telehealth has the potential to improve health communication dramatically. However, compared to their counterparts, these telehealth systems are favored by younger and higher-educated individuals, and their adoption might cause further disparities (Chambers et al., 2019). In fact, the telehealth infrastructure evolved during the COVID-19 era to prevent infections; yet, research surveying the effectiveness of telehealth strategies toward support for adequate hospital visits is lacking (Chambers et al., 2019) especially at the community level.
To promote appropriate patient hospital visit behavior and adequate health communication between patients and medical staff, we examined whether individuals who received advice from a physician via a telehealth application subsequently visited hospitals. Further, we examined the background factors associated with the behavior after they received advice from the physicians. We used machine learning to further examine (1) whether the chief complaints and basic characteristics could be predicted by physicians’ medical advice and (2) whether the chief complaint, medical advice given by the physicians, and users’ background characteristics predicted subsequent user behavior after they received physicians’ advice. The machine learning method was adopted to survey whether the application effectively utilized data to promote adequate patient hospital visit behavior toward saving human healthcare resources. We investigated whether telehealth application strategies including communication between users and physicians could be utilized to provide support for adequate hospital visits by users and patients at the community level.
This was an observational historical cohort study, which applied machine learning with the random forest approach. This study was approved by the Ethics Committee of LEBER Inc. (no. 21-01) and the Ethics Committee of Fukushima Medical University (no. 2021-190). An opt-out consent process was permitted; thus, we waived individual consent.
A telehealth application named “LEBER” was used to provide support for adequate hospital visiting (LEBER Inc., 2022). Users obtained medical advice, such as go to a hospital, stay home, call an ambulance, or others, from a physician through the chat application. The application was launched in January 2018, and was used commercially by over one million people by October 2022 (LEBER Inc., 2022).
The application process differed between “user flow” and “physician flow.” Users were required to complete the subsequent process: (1) download the application, (2) consult with a chat bot system, (3) receive medical advice, and (4) receive the web questionnaire a few days later asking whether they went to the hospital. Contrastingly, physicians completed the following process: (1) download the application, (2) get alarm-matched with a patient by the application, and (3) enter medical advice.
Users could add their chief complaint and symptom(s). Physicians then provided medical advice: stay home, go to the hospital if your condition worthens, go to the hospital emergency ward, or others. Users then made their decision. This application had an advantage as it was used by many people throughout Japan and provided medical advice rapidly by physicians.
A total of 9,552 candidates used the LEBER at least once and answered the online survey about whether they visited the hospital between January 2018 and October 2020. Of these, we included 7,152 participants who had a specific chief complaint and provided all the appropriate data: age, sex, consultation date and time, chief complaint, medical advice, and hospital visit.
Data were retrieved from the data source of the application by the staff of LEBER Co., Ltd. We integrated patients’ chief complaints into 27 major chief complaints (Table 1) and medical advice into three main categories: stay home, go to the hospital if something happens, and go to the hospital emergency ward. Time was classified into four categories: (1) 6 a.m. to 12 p.m., (2) 12 p.m. to 6 p.m., (3) 6 p.m. to 12 a.m., and (4) 12 a.m. to 6 a.m. Authors had access to information that could identify individual participants during or after data collection.
Table 1. Participants’ characteristics by whether users followed physicians’ advice or not (N = 7,152).
The primary outcome was whether the user followed medical advice. This included two categories: (1) went to the hospital after being told “go to the hospital if something happens” or “go to the hospital emergency ward” and (2) did not go to the hospital after the advice “stay home.” Those who did not follow physicians’ advice also included two categories: (1) stayed home after advised “go to the hospital if something happens” or “go to the hospital emergency ward” and (2) went to the hospital after the advice “stay home.”
We examined user background characteristics, which were associated with following physicians’ advice or not, to create a strategy using telehealth to promote appropriate patient consultation behavior. Categorical and continuous variables were analyzed using chi-squared and Mann–Whitney U tests, respectively. Descriptive analysis was performed using STATA IC version 15 (Lightstone, Texas, United States). Random forest approach of machine learning was performed to investigate whether physicians’ advice could be predicted based on the chief complaint, age, sex, and consultation time. Data were divided into 70% of training data and 30% of test data. The model’s sensitivity and specificity generated from the training data were calculated, and the area under the receiver operating characteristic curve (ROC-AUC) was calculated to show that the test data could be predicted by the model. We also examined the importance of each variable. The same machine learning method was used to obtain ROC-AUCs. Machine learning was performed using R studio version 4.1.2.
Among the 7,152 participants, 64.2% were women, and those in their 30s were the most frequent users. The proportion of “go to the hospital” and those who followed physicians’ advice were 34.5 and 40.7%, respectively. A total of 17.5% participants consulted regarding their chief complaint associated with COVID-19, and these were widely diverse. Age, medical advice, and consultation time differed between those who followed and did not follow the advice. A total of 70.6% were advised “go to the hospital (if something happens)” among those who did not follow physicians’ advice. Contrastingly, 49.3% were advised “go to the hospital (if something happens)” among participants who followed physicians’ advice (Table 1).
The proportion of each medical advice was significantly different between the groups (p < 0.001; chi-squared test). The majority of those advised to “go to the hospital (if something happens)” tended to not follow physicians’ advice. Contrastingly, those advised to “stay home” who followed this advice were classified as users who followed physicians’ advice (Figure 1).
Figure 1. The proportion of participants on hospital visit behavior by the groups given medical advice. (A) Hospital visiting on medical advice from physicians using the telehealth application. (B) Followed physicians’ advice or did not follow medical advice from physicians using the online platform.
We performed supervised learning using random forest modeling to categorize the medical advice by physicians. The dataset was randomly divided into the training dataset (70%) and test dataset (30%). A random forest classifier was trained on the training dataset and its performance was assessed using the test dataset. The discriminate model was highly performant with AUC over 0.65. The ROC-AUC was 0.792%. Hence, the aforementioned model soundly categorized the medical advice by physicians (Figure 2). The importance of each variable, which contributed to the model, is shown in Figure 2. Chief complaint was the most important variable to predict medical advice. Details of the chief complaints and consultation time by medical advice are shown in Supplementary Table S1. The variable importance in projection is shown in Supplementary Figure S1A.
Figure 2. Machine-learning based on physicians’ advice (go to the hospital or stay home) discrimination model. (A) A receiver operating curve (ROC) displaying the performance of a representative discrimination model. Area under the curve is 0.7918. (B) A variable importance of the discrimination model.
We performed supervised learning using random forest modeling to categorize (1) followed physicians’ advice and (2) did not follow physicians’ advice. The dataset was randomly divided into the training dataset (70%) and test dataset (30%). A random forest classifier was trained on the training dataset and its performance was assessed using the test dataset. The ROC-AUC was 0.677. Hence, the aforementioned model soundly categorized (1) followed physicians’ advice and (2) did not follow physicians’ advice (Figure 3). The importance of each variable, which contributed to the model, is shown in Figure 3. Chief complaint and medical advice were the most important variables to predict whether the user followed the medical advice. Details of the chief complaints and consultation time by followed or did not follow physicians’ advice are shown in Supplementary Table S1. The variable importance in projection is shown in Supplementary Figure S1B.
Figure 3. Machine-learning based on “followed physicians’ advice” or “did not follow” discrimination model. (A) ROC displaying the performance of a representative discrimination model. AUC is 0.6766. (B) A variable importance of the discrimination model.
Most physicians advised users with COVID-19 symptoms to stay home. Mental problems, headaches, and skin problems may have contributed to a failure to make users follow physicians’ advice in the projection model. The night time (6 p.m. to 12 a.m.) was associated with users not following physicians’ advice in the projection model.
We examined the subsequent hospital visit behavior among those who received physicians’ advice on the need to visit a hospital via a telehealth application to promote appropriate patient visit behavior. The association between physicians’ advice via the application and whether users followed said advice was investigated.
Physicians’ advice influenced users’ subsequent hospital visit behavior. The proportion of users who visited the hospital subsequent to physicians’ advice increased according to the urgency of the advice: stay home, go to the hospital if something happens, and go to the hospital emergency ward. Previous studies showed that telehealth supported adequate hospital visits significantly improved door-to-care times compared to controls (Friedman et al., 2021). However, this was an observational study. Hence, further research with a control group is required to confirm the effectiveness of the advice.
There might be some diseases for which the applications were not suitable. A total of 56.7% users consulted about COVID-19 followed physicians’ advice. Contrastingly, most physicians advised hospital visits for users with mental problems, but only 19.3% users followed the physicians’ advice. Studies that focus more on each specific disease are required to clarify these differences. Further, additional online support or phone call medical interviews to obtain further health information may prove beneficial. This could help physicians avoid excessive recommendations for hospital visiting for chief complaints, which could lead to serious outcomes.
Chief complaint, physicians’ advice, and patient background characteristics predicted whether users would follow physicians’ advice. Previous studies showed that online prehospital triage systems improved outcomes regarding consult-to-provider time and length of stay in the emergency department (Friedman et al., 2021). This study’s findings could be used to improve the outcomes associated with patients’ hospital visit behavior in the real world. Yet, there were some issues, such as older people experiencing difficulties in using such applications (Arcury et al., 2020; Pirhonen et al., 2020). Our previous study, which examined the participant characteristics of LEBER users, showed that older adults used the application at a much lesser frequency than those in their 30s (Kobashi et al., 2023). It is necessary to develop a user-friendly interface for all ages and populations and comprehensively introduce the system by the government and local authorities.
Physicians’ advice could be predicted from the chief complaint and users’ baseline characteristics. The chief complaint was the most important variable to understand whether the user would or would not follow physicians’ advice with machine learning. In the current Japanese healthcare system, patients can freely choose their hospital, and the chief complaint might not be able to be used to optimize the allocation of medical resources. The chief complaint is not well utilized for adequate hospital visit behavior. Therefore, this prediction might be of help in recommending appropriate patient consultations.
This study had several limitations. First, this study used data from an application that is broadly used only in Japan (LEBER Inc., 2022). Yet, it was a suitable application to access the telehealth system to provide support for adequate hospital visiting at the community level in Japan. Second, we only collected sex, age, chief complaint, consultation time. Other variables, such as household finance and educational background, may have been associated with outcomes. Nevertheless, we identified “chief complaint” as the most important variable. Third, we did not have control group to compare the effect of the intervention. Future studies should employ an experimental design. Finally, we defined the medical advice of “go to the hospital if something happens” as that the physicians recommended patients go to the hospital. However, the advice could have been deemed more “neutral.” We decided on this classification after careful discussion between the researchers, physicians, and the system engineering team.
Physicians’ advice influenced users’ subsequent hospital visit behavior. Furthermore, chief complaint, physicians’ advice, and patient background characteristics predicted whether users would follow physicians’ advice. These findings should be effectively utilized for promoting adequate patient hospital visit behavior toward saving human healthcare resources.
The datasets presented in this article are not publicly available because the dataset included personal information. Reasonable requests to access the datasets should be directed to c2h1bkBsZWJlci5qcA==.
The studies involving humans were approved by Fukushima Medical University (no. 2021-190). The studies were conducted in accordance with the local legislation and institutional requirements. Written informed consent for participation was not required from the participants or the participants’ legal guardians/next of kin because an opt-out consent process was permitted.
YK: Formal analysis, Methodology, Project administration, Writing – original draft. MO: Data curation, Writing – review & editing. NN: Supervision, Validation, Writing – review & editing. MT: Methodology, Project administration, Resources, Supervision, Writing – review & editing. SI: Data curation, Writing – review & editing.
The author(s) declare that no financial support was received for the research, authorship, and/or publication of this article.
We thank the physicians at Tsukuba University and all other physicians who greatly cooperated with tele consultation with LEBER users.
YK, MT, MO, and SI have a position in LEBER Inc. with a salary or consultation fee (out of this work). MO and SI provided the database; however, they did not conduct analyses and did not decide the direction of the manuscript. YK and MT conducted analyses and drafted the manuscript as the position of Fukushima Medical University.
The remaining author declares that the research was conducted in the absence of any commercial or financial relationships that could be construed as a potential conflict of interest.
All claims expressed in this article are solely those of the authors and do not necessarily represent those of their affiliated organizations, or those of the publisher, the editors and the reviewers. Any product that may be evaluated in this article, or claim that may be made by its manufacturer, is not guaranteed or endorsed by the publisher.
The Supplementary material for this article can be found online at: https://www.frontiersin.org/articles/10.3389/fcomm.2024.1292792/full#supplementary-material
Arcury, T. A., Sandberg, J. C., Melius, K. P., Quandt, S. A., Leng, X., Latulipe, C., et al. (2020). Older adult internet use and eHealth literacy. J. Appl. Gerontol. 39, 141–150. doi: 10.1177/0733464818807468
Arrossi, S., Paolino, M., Orellana, L., Thouyaret, L., Kohler, R. E., and Viswanath, K. (2019). Mixed-methods approach to evaluate an mHealth intervention to increase adherence to triage of human papillomavirus-positive women who have performed self-collection (the ATICA study): study protocol for a hybrid type I cluster randomized effectiveness-implementation trial. Trials 20:148. doi: 10.1186/s13063-019-3229-3
Beyer, A., Moon, K., Penndorf, P., Hirsch, T., Zahn-Tesch, U., Hoffmann, W., et al. (2022). Triage through telemedicine in paediatric emergency care-results of a concordance study. PLoS One 17:e0269058. doi: 10.1371/journal.pone.0269058
Carmichael, H., Dyamenahalli, K., Duffy, P. S., Lambert Wagner, A., and Wiktor, A. J. (2020). Triage and transfer to a regional burn center-impact of a mobile phone app. J. Burn Care Res. 41, 971–975. doi: 10.1093/jbcr/iraa098
Chai, P. R., Dadabhoy, F. Z., Huang, H. W., Chu, J. N., Feng, A., Le, H. M., et al. (2021). Assessment of the acceptability and feasibility of using mobile robotic systems for patient evaluation. JAMA Netw. Open 4:e210667. doi: 10.1001/jamanetworkopen.2021.0667
Chambers, D., Cantrell, A. J., Johnson, M., Preston, L., Baxter, S. K., Booth, A., et al. (2019). Digital and online symptom checkers and health assessment/triage services for urgent health problems: systematic review. BMJ Open 9:e027743. doi: 10.1136/bmjopen-2018-027743
Friedman, J., Lame, M., Clark, S., Gogia, K., Platt, S. L., and Kim, J. W. (2021). Telemedicine medical screening evaluation expedites the initiation of emergency care for children. Pediatr. Emerg. Care 37, e417–e420. doi: 10.1097/PEC.0000000000002428
Hollander, J. E., and Carr, B. G. (2020). Virtually perfect? Telemedicine for Covid-19. N. Engl. J. Med. 382, 1679–1681. doi: 10.1056/NEJMp2003539
Kobashi, Y., Oguni, M., Tsubokura, M., Kanda, N., Ito, N., and Ito, S. (2023). The disparity of utilization rate among specific groups for a rapid spreading telehealth application called LEBER during the COVID-19 state of emergency in Japan. J. Rural Med. 18, 233–240. doi: 10.2185/jrm.2022-052
Kucewicz-Czech, E., and Damps, M. (2020). Triage during the COVID-19 pandemic. Anaesthesiol. Intensive Ther. 52, 312–315. doi: 10.5114/ait.2020.100564
LEBER Inc. (2022). Available at: https://www.leber.jp/ (Accessed March 15, 2023)
Morrill, J., Qirko, K., Kelly, J., Ambrosy, A., Toro, B., Smith, T., et al. (2022). A machine learning methodology for identification and triage of heart failure exacerbations. J. Cardiovasc. Transl. Res. 15, 103–115. doi: 10.1007/s12265-021-10151-7
Pirhonen, J., Lolich, L., Tuominen, K., Jolanki, O., and Timonen, V. (2020). “These devices have not been made for older people’s needs”–older adults’ perceptions of digital technologies in Finland and Ireland. Technol. Soc. 62:101287. doi: 10.1016/j.techsoc.2020.101287
Sivarajasingam, V. (2021). Total triage is the future for general practice. BMJ 373:n1532. doi: 10.1136/bmj.n1532
Keywords: telehealth, telehealth application, hospital visit behavior, Japan, machine learning
Citation: Kobashi Y, Oguni M, Nakamura N, Tsubokura M and Ito S (2024) A telehealth application for adequate hospital visit advice by physicians affected users’ subsequent hospital visit behavior: a historical cohort study with machine learning. Front. Commun. 9:1292792. doi: 10.3389/fcomm.2024.1292792
Received: 14 September 2023; Accepted: 25 March 2024;
Published: 11 April 2024.
Edited by:
Gulzar H. Shah, Georgia Southern University, United StatesReviewed by:
Alma Nurtazina, Semey State Medical University, KazakhstanCopyright © 2024 Kobashi, Oguni, Nakamura, Tsubokura and Ito. This is an open-access article distributed under the terms of the Creative Commons Attribution License (CC BY). The use, distribution or reproduction in other forums is permitted, provided the original author(s) and the copyright owner(s) are credited and that the original publication in this journal is cited, in accordance with accepted academic practice. No use, distribution or reproduction is permitted which does not comply with these terms.
*Correspondence: Shunichiro Ito, c2h1bkBsZWJlci5qcA==
Disclaimer: All claims expressed in this article are solely those of the authors and do not necessarily represent those of their affiliated organizations, or those of the publisher, the editors and the reviewers. Any product that may be evaluated in this article or claim that may be made by its manufacturer is not guaranteed or endorsed by the publisher.
Research integrity at Frontiers
Learn more about the work of our research integrity team to safeguard the quality of each article we publish.