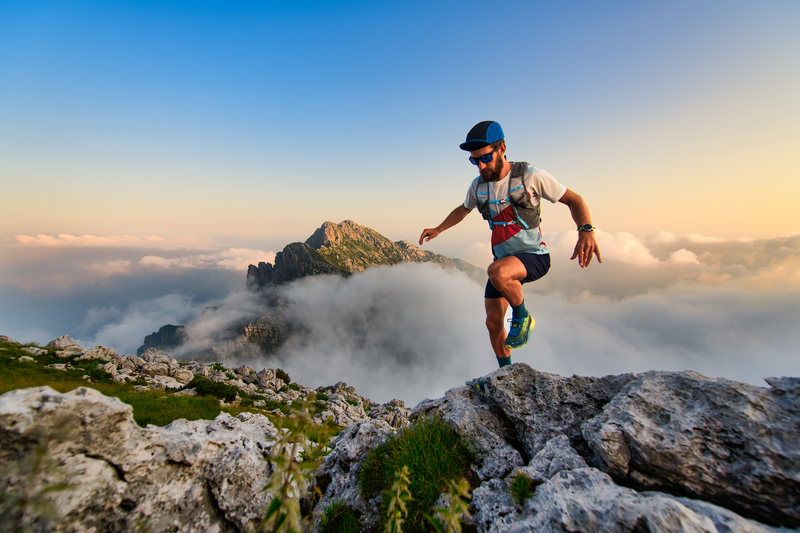
94% of researchers rate our articles as excellent or good
Learn more about the work of our research integrity team to safeguard the quality of each article we publish.
Find out more
ORIGINAL RESEARCH article
Front. Commun. , 27 September 2023
Sec. Health Communication
Volume 8 - 2023 | https://doi.org/10.3389/fcomm.2023.1275127
This article is part of the Research Topic Integrating Digital Health Technologies in Clinical Practice and Everyday Life: Unfolding Innovative Communication Practices View all 9 articles
Background: Chatbots are increasingly being used across a wide range of contexts. Medical chatbots have the potential to improve healthcare capacity and provide timely patient access to health information. Chatbots may also be useful for encouraging individuals to seek an initial consultation for embarrassing or stigmatizing conditions.
Method: This experimental study used a series of vignettes to test the impact of different scenarios (experiencing embarrassing vs. stigmatizing conditions, and sexual vs. non-sexual symptoms) on consultation preferences (chatbot vs. doctor), attitudes toward consultation methods, and expected speed of seeking medical advice.
Results: The findings show that the majority of participants preferred doctors over chatbots for consultations across all conditions and symptom types. However, more participants preferred chatbots when addressing embarrassing sexual symptoms, compared with other symptom categories. Consulting with a doctor was believed to be more accurate, reassuring, trustworthy, useful and confidential than consulting with a medical chatbot, but also more embarrassing and stressful. Consulting with a medical chatbot was believed to be easier and more convenient, but also more frustrating. Interestingly, people with an overall preference for chatbots believed this method would encourage them to seek medical advice earlier than those who would prefer to consult with a doctor.
Conclusions: The findings highlight the potential role of chatbots in addressing embarrassing sexual symptoms. Incorporating chatbots into healthcare systems could provide a faster, more accessible and convenient route to health information and early diagnosis, as individuals may use them to seek earlier consultations.
A chatbot is a “communication simulating computer program” designed to mimic human-to-human conversation by analyzing the user's text-based input, and providing smart, related answers (Dahiya, 2017; Khan et al., 2018). Chatbots are often designed for a specific environment or context, e.g., answering online customers' frequently asked questions, or providing technical support. Chatbots are increasingly being used within healthcare as a way of dealing with growing demands for health information (Rosruen and Samanchuen, 2018; Softić et al., 2021). Medical chatbots can use the information provided by the user regarding their health concerns and/or symptoms and provide advice on the best course of action for treatment or further investigation. Medical chatbots offer two key potential benefits for assisting healthcare: (1) supporting a strained healthcare workforce by increasing system capacity, and (2) encouraging individuals to seek early support for embarrassing and stigmatizing conditions.
Healthcare services are notoriously overworked and understaffed (Bridgeman et al., 2018; Baker et al., 2020). Though this has been especially apparent during the COVID-19 pandemic, it is a longstanding problem that is expected to persist in the future (House of Commons Health Social Care Committee., 2021). In the UK, many patients experience considerable wait times for doctor appointments (McIntyre and Chow, 2020; Oliver, 2022; Appleby, 2023). Therefore, there is a significant need for interventions to help increase health service capacity in a timely and cost-effective manner, without placing further burden on healthcare staff. Every day, people around the world search the internet for health information. It is estimated that Google receives more than 1 billion health questions every day (Drees, 2019). However, it can be difficult for users to know which online sources provide safe and reliable health information (Daraz et al., 2019; Battineni et al., 2020). NHS England has stated that achieving a core level of digital maturity across integrated care systems is a key priority within its 2022/3 operational planning guidance (NHS England, 2022). In the week of the NHS' 75th birthday, a summit was held with the aim of driving digital innovation to help deliver better care for patients and cut waiting times (Department of Health Social Care, 2023). Recent examples of digital innovation in healthcare have included medical chatbots, which provide an opportunity to free up staff time, whilst providing patients with timely and efficient access to trustworthy health information (Gilbert et al., 2023; Lee et al., 2023; Matulis and McCoy, 2023).
Medical chatbots may play another important role in health and wellbeing. There are many conditions which go underdiagnosed and untreated due to individuals feeling stigmatized and/or embarrassed (Sheehan and Corrigan, 2020). It can be difficult for individuals to share information and openly discuss their health with medical professionals when they anticipate stigma or embarrassment in response to disclosing their symptoms (Simpson et al., 2021; Brown et al., 2022a,b). Many people may miss the opportunity for early treatment, which can lead to significant decreases in health and wellbeing. Medical chatbots could provide users with a more accessible initial consultation to discuss health concerns and/or medical symptoms (Bates, 2019). Medical chatbots could be used to encourage users to talk about their symptoms in a relaxed environment, which may act as a positive “first step” to help them on their health journey. Conducting some of the initial awkward discussions about embarrassing and/or stigmatizing symptoms through a medical chatbot could make the difference between someone seeking medical advice or choosing to ignore the issue (or delaying help seeking). Chatbots can also be useful after an initial diagnosis, and can be used to help individuals manage their long-term health (Bates, 2019). It is predicted that in the future, patients will have the ability to share their healthcare records and information with medical chatbots, to help further improve their application and accuracy (Bates, 2019).
Interest in chatbots has further increased with the recent release of ChatGPT. In August 2023, we asked ChatGPT version 3.5 to describe itself, it responded, “ChatGPT is an AI language model developed by OpenAI that can engage in conversations and generate human-like text that is based on the input it receives. It is trained on a large dataset from the Internet and uses deep learning to understand and respond to user queries” (OpenAI., 2023). Originally released in November 2022, healthcare researchers have already begun to explore the potential of ChatGPT and similar tools for improving healthcare. A US online survey of 600 active ChatGPT users found that approximately 7% of respondents were already using it for health-related queries (Choudhury and Shamszare, 2023). Another US-representative survey of over 400 users suggested that laypeople appear to trust the use of chatbots for answering low-risk health questions (Nov et al., 2023). Initial findings suggest that ChatGPT can produce highly relevant and interpretable responses to medical questions about diagnosis and treatment (Hopkins et al., 2023). Despite the potential to assist in providing medical advice and timely diagnosis, concerns have been raised about the accuracy of responses and the continuing need for human oversight (Temsah et al., 2023). It is vital that researchers continue to investigate health-related interactions between chatbots and users to both limit the risk of harm and maximize the potential improvements to healthcare.
In this study, we are interested in exploring preferences for initial consultations about different medical symptoms. We are particularly interested in the impact of potentially embarrassing or stigmatizing symptoms on patient preferences for consultations with doctors and chatbots. Therefore, the aims of this study are threefold: (1) to investigate preferred consultation methods in response to experiencing potentially embarrassing or stigmatizing medical symptoms; (2) to assess participant beliefs about the benefits/limitations of different consultation methods for potentially embarrassing or stigmatizing medical symptoms; and (3) to examine whether a particular consultation method is more likely to result in seeking earlier consultation.
An experimental study was conducted using a quantitative Qualtrics questionnaire that was approved by the Research Ethics Committee at Northumbria University (ethical approval 54282). A UK nationally representative sample of 402 adult participants was recruited via the platform Prolific. To provide nationally representative samples, Prolific screens participants based on age, gender, and ethnicity in proportion to data derived from the UK's national census in 2021 (Office for National Statistics., 2023; Prolific., 2023). Participants were UK residents aged between 18 and 81 years (M = 46.23 years, SD = 15.95 years), 203 were female, 195 were male and four reported a different gender identity. For full details of the sample characteristics, see Table 1.
Participants were asked to imagine they were experiencing a particular set of medical symptoms and then answered questions about their expected response. The health conditions associated with the presented symptoms were categorized into potentially embarrassing or potentially stigmatizing conditions. They were also classified as sexual or non-sexual symptoms. These categories of health conditions and related symptoms were drawn from existing health literature that identified conditions as either potentially embarrassing or stigmatizing and/or sexual or non-sexual (see below). Feelings of embarrassment when discussing health are common among patients living with a range of conditions and symptoms, including: Irritable Bowel Syndrome (IBS) (Duffy, 2020), Psoriasis (Taliercio et al., 2021; Sumpton et al., 2022), low libido (Brandon, 2016), and those experiencing pain during sex (McDonagh et al., 2018; Lee and Frodsham, 2019). These conditions involve symptoms that people may find uncomfortable, awkward, or challenging to discuss openly. These symptoms are often considered personal or intimate aspects of someone's life that may be embarrassing to disclose to others. In contrast, conditions typically associated with experiences of health-related stigma include: Diabetes (Schabert et al., 2013; Liu et al., 2017), Eating Disorders (EDs) (Dimitropoulos et al., 2016; Williams et al., 2018), Human Immunodeficiency Virus (HIV) (Earnshaw and Chaudoir, 2009; Nyblade et al., 2009), and Sexually Transmitted Infections (STIs) (Hood and Friedman, 2011; Lee and Cody, 2020). These conditions are often linked to experiences of societal stigmatization, public misconceptions, and discriminatory attitudes. People experiencing potentially stigmatizing symptoms may face significant challenges, including social exclusion, prejudice, and fear of judgment. We also distinguish between sexual symptoms (low libido, pain during sex, HIV and STIs) and non-sexual symptoms (IBS, Psoriasis, eating disorders and Diabetes). This is an important distinction because previous research has found that patients are often less likely to discuss sexual health issues with medical professionals compared to non-sexual symptoms (Martel et al., 2017; Manninen et al., 2021). The full research team drew on their collective experience in health stigma research to discuss and agree the final selection of symptoms and conditions, and to assign their respective categories. By asking participants to consider experiences of embarrassing and stigmatizing symptoms that are either sexual or non-sexual in nature, we aim to gain insights into the factors that influence medical consultation preferences for each category.
The study protocol is set out in Figure 1. Firstly, participants provided demographic information (age, gender, ethnicity, country of residence, and educational attainment). Participants then indicated whether they had experience working in healthcare or using chatbots (“yes/no”), and how familiar they were with chatbots, texting, and video calls on a four-point Likert scale from “not at all familiar” to “extremely familiar.”
Each participant was then provided with 2 scenarios: 1 from the embarrassing condition group, and then 1 from the stigmatizing condition group. In each scenario, participants were evenly allocated to either the sexual or non-sexual symptom groups, and then randomly presented with a specific set of symptoms. Participants were asked to answer ten questions for each scenario. See Figure 1 for precise categorization and wording of symptoms.
For each scenario, participants were asked how quickly they would seek medical advice about the presented symptoms on a four-point Likert scale from “no hurry to seek advice” to “would seek advice immediately” and how embarrassing they believed it would be to seek medical advice about the presented symptoms on a four-point Likert scale from “not at all” to “extremely embarrassing.”
Participants were also asked to compare doctors and medical chatbots across ten dimensions to determine which would be more: convenient, useful, easy, confidential, stressful, trustworthy, frustrating, reassuring, embarrassing, and accurate. Responses were provided on a five-point continuum from “Chatbot would be a lot more…” to “Doctor would be a lot more...”. Participants also ranked their preferred way to interact with a doctor if experiencing the presented symptoms (“in-person,” “phone call,” “video call,” or “text messaging”) and their preferred method for interacting with a medical chatbot (“phone call”, “video call”, or “text messaging”).
Finally, participants were asked to state their overall preferred method of consultation for the symptoms described in each scenario: “Doctor” or “Chatbot.” Participants were then asked the extent to which their preferred method of initial consultation would be: positive for their life, personally beneficial, beneficial for society, something they intend to use, something they will try, and something others should use. Participants responded on a five-point Likert scale from “strongly disagree” to “strongly agree.” Participants were asked if their preferred method of initial consultation would encourage them to seek medical advice earlier/later, on a five-point Likert scale from “much earlier” to “much later.” Finally, participants indicated whether they had previously experienced the symptoms described (“yes/no”).
All statistical analyses were conducted using R (R Core., 2021). The following packages were used for data processing, analysis, and visualization: nnet (Ripley et al., 2016), psych (Revelle, 2021), Rmisc (Hope, 2013), and tidyverse (Wickham et al., 2020).
We investigated the separate effects of embarrassing vs. stigmatizing conditions and sexual vs. non-sexual symptoms on preferred method of initial consultation. All participants were presented with symptoms associated with both embarrassing (scenario one) and stigmatizing conditions (scenario two). However, participants were randomly assigned sexual or non-sexual symptoms for each scenario, therefore some participants were presented with one sexual and one non-sexual set of symptoms (n = 200), whereas others saw two of the same type (n = 202). Therefore, we conducted different statistical analyses to study the effects of embarrassing vs. stigmatizing conditions, compared to sexual vs. non-sexual symptoms.
Two separate chi-square tests were conducted for scenarios one and two (embarrassing and stigmatizing conditions respectively; n = 402) to determine the effect of sexual vs. non-sexual symptoms on preferred method of initial consultation (chatbot vs. doctor). McNemar's chi-square test was used to determine the effect of embarrassing vs. stigmatizing conditions on preferred method of initial consultation, among those participants that were randomly assigned the same symptom type in both scenarios (i.e., two sexual sets of symptoms, or two non-sexual sets of symptoms; n = 202). This approach enabled us to investigate the effect of embarrassing vs. stigmatizing on preferred method of consultation, whilst controlling for the effect of considering sexual vs. non-sexual symptoms. To evaluate overall consultation preference across scenarios, a new variable was created capturing whether participants chose “Doctor” or “Chatbot” for both scenarios or “Both” (indicating a different preference in each scenario). Multinomial regression was used to determine the impact of age and gender on overall consultation preference (n = 402).
In relation to the survey items where we asked participants to compare doctors and chatbots across 10 dimensions, we combined scores for the positive dimensions (convenient, useful, easy, confidential, trustworthy, reassuring, and accurate) and negative dimensions (stressful, frustrating, and embarrassing) to create two subscales (positive and negative consultation attributes). For each subscale, the scores were summed to produce a single value. Cronbach's Alpha was calculated for each subscale. For the positive attributes subscale α = 0.79 indicating a “high” standard of internal consistency between items (Taber, 2018). The value for Cronbach's Alpha for the negative subscale was α = 0.63 indicating an “adequate” degree of internal consistency between items (Taber, 2018). Independent sample t-tests were conducted to compare positive and negative attributes between those participants presented with sexual vs. non-sexual symptoms. To investigate the effect of embarrassing vs. stigmatizing conditions, paired-sample t-tests were used to compare dimensions between scenarios among those assigned the same symptom type (sexual or non-sexual) in both scenarios.
Finally, separate Welch t-tests for each scenario investigated differences between participants' beliefs about whether their preferred method of initial consultation would influence their expected speed of seeking medical advice, between participants preferring chatbots vs. those preferring doctors.
A summary of sample demographics and characteristics is provided in Table 1.
Across all 8 health conditions, the majority of participants preferred an initial consultation with a doctor rather than a chatbot (Figure 2).
The majority (65.7%) of participants expressed the same preference for a doctor across both the stigmatizing and embarrassing scenarios. Almost 12% of participants preferred a chatbot across both scenarios, but 22.4% expressed a different preference for the stigmatizing and embarrassing scenarios. From these participants with different preferences across scenarios (n = 90), 57.8% (n = 52) chose chatbots for the embarrassing scenario and doctors for the stigmatizing scenario, and 42.2% (n = 38) chose doctors for the embarrassing scenario and chatbots for the stigmatizing scenario.
We compared the effect of scenario (embarrassing vs. stigmatizing conditions) on consultation choice among those participants that responded to the same symptom type (sexual or non-sexual) in both scenarios. A McNemar's chi-square test determined that there was no significant difference in overall consultation choice between embarrassing and stigmatizing conditions [x2(1, 202) = 2.38, p = 0.12; see Supplementary Figure S3].
Multinomial logistic regression analysis found no significant effect of age or gender on overall preferred consultation method in both scenarios. Age had no effect on likelihood of preferring doctors (β = 0.006, SE = 0.008, OR = 1.006 (95% CI [0.991, 1.022]) or chatbots (β = 0.017, SE = 0.011, OR = 1.017 (95% CI [0.995, 1.040]) compared to choosing one of each. Similarly, gender (being female) had no effect on overall preference for doctors (β = 0.073, SE = 0.245, OR = 1.075 (95% CI [0.665, 1.738]) or chatbots (β = 0.291, SE = 0.360, OR = 1.338 (95% CI [0.660, 2.711]). For a comparison of overall consultation choice across age and gender, see Supplementary Figure S4.
When ranking methods for interacting with a doctor, 67.8% of responses chose “in-person” as their preferred choice. This finding was consistent across all symptoms. Interacting with a doctor via text was least preferred, ranked last in 60% of all responses. For chatbots, interacting via text was the preferred method (ranked first in 72% of all responses) and video was the least preferred (ranked last in 59.5% of responses; see Figure 3).
Figure 3. Participant rankings for preferred method to consult with a doctor (left) and medical chatbot (right).
We also investigated whether preference for an initial consultation with a doctor or chatbot differed between sexual and non-sexual symptoms. When comparing within the embarrassing (compared to stigmatizing) conditions, a chi-square test of independence indicated a significant difference in consultation choice between those presented with sexual vs. non-sexual symptoms [x2(1, 402) = 28.17, p < 0.001; see Supplementary Figure S1]. Though doctors were the preferred choice overall, more participants preferred chatbots among those considering embarrassing sexual symptoms than for embarrassing non-sexual symptoms. However, there was no significant difference in consultation choice between sexual and non-sexual symptoms in scenario two (potentially stigmatizing symptoms; x2(1, 402) = 3.79, p = 0.05; see Supplementary Figure S2).
Overall, participants reported believing that an initial consultation with a doctor would be more accurate, reassuring, trustworthy, useful and confidential than consulting with a medical chatbot, but also more embarrassing and stressful. An initial consultation with a medical chatbot was believed to be easier, more convenient but also more frustrating than consulting with a doctor (see Figure 4). T-tests were conducted for each scenario to investigate differences in overall scores for positive attributes (accurate, reassuring, trustworthy, useful, confidential, easy, convenient) and negative attributes (embarrassing, stressful, frustrating). A difference was found when comparing between sexual and non-sexual conditions. In scenario one (embarrassing conditions), those considering non-sexual symptoms (M = 4.76) associated positive attributes with doctors more so than those with sexual symptoms [M = 2.29; t(400) = 5.33, p < 0.001]. Similarly, those with non-sexual symptoms associated negative attributes more with chatbots (M = −0.57) and those with sexual symptoms associated negative dimensions more with doctors [M = 0.89; t(400) = −6.99, p < 0.001]. In scenario two, there was no significant difference between those with sexual/non-sexual symptoms associating positive attributes with chatbots/doctors [t(400) = 1.04, p = 0.30]. However, those with non-sexual symptoms again associated negative attributes more with chatbots (M = −0.31) and those with sexual symptoms associated negative attributes more with doctors [M = 0.91; t(400) = −6.09, p < 0.001].
Paired-sample t-tests showed no significant differences between scenarios (embarrassing vs. stigmatizing conditions) for attributing positive [t(201) = −1.23, p = 0.22] or negative [t(201) = −0.46, p = 0.64] dimensions to consultations with chatbot/doctors. See Supplementary Figures S5–S7 for differences in individual dimensions by condition and scenario).
Participants who preferred an initial consultation with a doctor reported greater belief in the personal benefits of their chosen method compared to those preferring chatbots (see Figure 5). There was no significant difference in the perceived societal benefits of their chosen method between those preferring doctors/chatbots.
We compared participant beliefs about whether their preferred method of initial consultation would influence their expected speed of seeking medical advice. Welch t-tests (conducted to account for unequal groups sizes between those preferring chatbots vs. doctors) found that participants with an overall preference for chatbots indicated that this method would encourage them to seek earlier medical advice than those preferring an initial consultation with a doctor (see Figure 6). A significant difference in expected speed of seeking medical advice between consultation choice groups (chatbot vs. doctor) was found in both scenario one (potentially embarrassing symptoms; t(228) = 14.58, p < 0.001) and scenario two [stigmatizing conditions; t(146.38) = 6.51, p < 0.001].
Figure 6. Participant beliefs about whether their chosen method of consultation would influence speed of seeking medical advice. Colored bars indicate the mean scores from our full sample (n = 402) for expected speed of seeking medical advice for those who preferred a Chatbot (in pink) for each symptom category, and for those who preferred a Doctor (in blue) for each symptom category.
While our results found that the majority of people would prefer to receive an initial consultation from a doctor than from a medical chatbot for all health conditions depicted in this study, more participants preferred chatbots when considering embarrassing symptoms that were also sexual (e.g., low libido, pain during sex). However, doctors were still the more frequent choice for this category. Previous studies into interaction preferences with respect to potentially embarrassing sexual conditions has produced mixed findings. For example, a study of over 300 university students in Canada and South Africa found that men and women would prefer to receive STI test results by talking face-to-face with a doctor rather than via phone, text message, e-mail, or other forms of Internet-based communication (Labacher and Mitchell, 2013). Similarly, an online survey of 148 German adults reported that patients would prefer to disclose medical information to a doctor rather than to a chatbot, and found no difference in the tendency to conceal information from either doctors or chatbots (Frick et al., 2021). However, Holthöwer and van Doorn (2022) recently found that, despite being typically reluctant to interact with service robots, consumers perceive them less negatively when engaging in an embarrassing service encounter (such as acquiring medication to treat a sexually transmitted disease). Similarly, in a study of 179 online participants, Kim et al. (2022) found that people are less willing to interact with a doctor than with a medical chatbot, when answering potentially embarrassing questions (recent sexual activity, habits, and preferences). Whereas, for non-sensitive questions (demographic questions and current state of health), willingness to disclose was higher in the human condition than in the chatbot condition. Participants of a recent online survey in the UK reported neutral-to-positive attitudes toward sexual health chatbots, and were broadly comfortable disclosing sensitive information with further qualitative findings suggesting that chatbots provide a useful means of providing sexual health information (Nadarzynski et al., 2021, 2023). In our present study, participant evaluations of each consultation method revealed that people were generally most positive about the qualities of chatbots when considering embarrassing and sexual symptoms. Those considering sexual symptoms associated negative dimensions more with doctors, whereas those considering non-sexual symptoms attributed negative dimensions more to chatbots. In light of the collective evidence from this study and previous research, medical chatbots may encourage users to seek medical advice relating to potentially embarrassing sexual symptoms, despite the persisting overall preference for human doctor consultations. Furthermore, some participants did indicate a preference for medical chatbots in both presented scenarios. This suggests that providing the option to receive an initial consultation from a medical chatbot would be beneficial to those who are already comfortable with this emerging technology.
Some previous research has suggested that high levels of technology acceptance support the ongoing use of telehealth solutions in healthcare beyond the constraints of the pandemic (Burbury et al., 2022; Desborough et al., 2022; Branley-Bell and Murphy-Morgan, 2023). However, our findings suggest more people still prefer in-person interactions with doctors. Similarly, an online survey of over a thousand Australian patients found that most (62.6%) preferred in-person consultations with their doctor compared to remote consultations, however those in regional and rural areas were less likely to prefer in-person consultations (Rasmussen et al., 2022). Our study did not ask participants to consider the time, cost or logistics involved in accessing an in-person consultation with their doctor. It is possible that considering the practical factors (as well as the social and emotional factors this study investigated) associated with attending an in-person appointment with a doctor may impact real-world decision-making with respect to medical consultations.
For consultations with doctors, participants reported preferring in-person interactions and least preferred interacting via text. Whereas, for consulting with a chatbot, participants reported preferring to interact via text and least preferred video, avatar-based interactions. This is interesting given the increase in avatar-based chatbots as a method to increase their uptake and acceptance in healthcare settings (Ciechanowski et al., 2019; Moriuchi, 2022). There are multiple potential explanations for this finding, including privacy and ease of use—it may simply be easier and feel more confidential for users to interact with a chatbot via text, whereas they may need to find a private space to engage in a ‘conversation' with an avatar-based chatbot. Familiarity will also play a role as users will tend to prefer technology they are more familiar with (e.g., chatbot text based interaction compared to speech based avatar interaction). Communicating by text may also avoid the feeling of another being physically present, which makes sense when we find that users are most likely to use chatbots for embarrassing, sexual symptoms—in this particular situation perhaps being more human-like is a disadvantage for chatbots. Previous research in a consumer setting found that people perceive an increased social presence when a chatbot is more “human-like,” making consumers more likely to feel socially judged (Holthöwer and van Doorn, 2022). Furthermore, research suggests that users may show a preference for text chatbots over video-based chatbots due to the “uncanny valley” effect. This relates to a feeling of unease when interacting with something that seems eerily human (Ciechanowski et al., 2019).
The attribute which differed the most between symptom categories was perceived stressfulness. When considering embarrassing sexual symptoms (pain during sex and low libido), participants reported that consulting with a doctor would be significantly more stressful than with a chatbot. These findings indicate the potential for medical chatbots to alleviate some of the emotional barriers and enhance patient wellbeing in areas traditionally associated with discomfort and anxiety. Furthermore, we found that participants with an overall preference for chatbots believed that this method would encourage them to seek medical advice earlier than those who preferred an initial consultation with a doctor. This was true across all symptom categories. This is particularly relevant for individuals dealing with embarrassing or stigmatizing symptoms, as they may be more likely to avoid discussing their health with others, thereby risking aggravating their symptoms and the possibility of missing out on earlier, more manageable treatment options. Embracing the use of medical chatbots for timely advice and treatment could help to alleviate the current strain on the healthcare system: firstly, by relieving some of the burden of initial consultations and empowering individuals to address their symptoms promptly; and secondly, by tackling the development of more severe conditions resulting from prolonged undiagnosed or untreated symptoms.
Given recent developments in large language models and the increasing capabilities of AI, perceptions toward medical chatbots are likely to change and evolve in the years to come. For example, a recent study asked a team of licensed healthcare professionals to compare doctor and ChatGPT responses to health questions on social media (Ayers et al., 2023). ChatGPT responses were rated significantly higher, in terms of both quality and empathy, than those made by doctors for 78.6% of the 585 evaluations. A recent systematic review of research into the potential healthcare applications of ChatGPT and related tools suggested that they have the potential to revolutionize healthcare delivery (Muftić et al., 2023). Although at present, individuals may experience some concerns or unease with the use of AI, this is not uncommon for new technology (Meuter et al., 2003; Szollosy, 2017; Khasawneh, 2018), as society becomes more familiar with the technology, and awareness of potential benefits and usefulness increases, we may see a shift in preferences and/or a wider uptake of chatbot services. Amidst the wide array of potential benefits and challenges associated with medical chatbots, our findings suggest they could be useful for encouraging patients to seek earlier medical advice, with particular relevance for people experiencing potentially embarrassing sexual symptoms.
We acknowledge the limitations of our study. Firstly, the study focused on hypothetical scenarios and participants' expected preferences, which may not fully reflect their actual choices and behaviors in real-life health situations. Future research should look to assess participant responses to actual interactions with medical chatbots, as well as investigating real-life choices around available consultation methods. It is also important to note that the study did not ask participants to consider practical factors that may influence their decision to choose a particular consultation method or the strength of their preference. Though people may ideally prefer to receive a medical consultation from a doctor, practical factors (such as having to take time off work, cost of traveling to an appointment, caregiving commitments, as well as current state of physical and mental health) may influence the decision to choose a particular method of medical consultation.
Despite the increasing role of technology in healthcare, this study found that more people still prefer consultations with doctors over chatbots. However, when it comes to potentially embarrassing sexual symptoms, chatbots were more accepted and preferred by more participants than when considering other symptom categories. Participants evaluated chatbots more positively when considering potentially embarrassing sexual symptoms, while their evaluations of doctors for the same symptoms were more negative. These findings suggest that chatbots have the potential to encourage individuals to seek earlier medical advice, particularly for symptoms they may find uncomfortable to discuss with their doctor. Incorporating chatbot interventions in healthcare settings—as a compliment, not replacement, to face-to-face consultations could serve as a valuable tool in the patient experience and encourage more timely healthcare seeking behaviors. The chatbot can serve as a first point of call to collect data, particularly relating to embarrassing symptoms. However, it is important to acknowledge that further research is needed to investigate the safety and effectiveness of medical chatbots in real-world health settings.
The data and code that support the findings of this study are openly available as part of the Open Science Framework at https://osf.io/dgwna/.
The studies involving humans were approved by the Northumbria Psychology Ethics Board. The studies were conducted in accordance with the local legislation and institutional requirements. The participants provided their written informed consent to participate in this study.
DB-B: Conceptualization, Data curation, Formal analysis, Investigation, Methodology, Project administration, Supervision, Writing—original draft, Writing—review and editing. RB: Data curation, Formal analysis, Visualization, Writing—original draft, Writing—review and editing. LC: Conceptualization, Funding acquisition, Supervision, Writing—review and editing. ES: Conceptualization, Investigation, Methodology, Supervision, Writing—original draft, Writing—review and editing.
The author(s) declare financial support was received for the research, authorship, and publication of this article. This work was funded by the EPSRC as part of the Centre for Digital Citizens (grant number EP/T022582/1).
The authors declare that the research was conducted in the absence of any commercial or financial relationships that could be construed as a potential conflict of interest.
All claims expressed in this article are solely those of the authors and do not necessarily represent those of their affiliated organizations, or those of the publisher, the editors and the reviewers. Any product that may be evaluated in this article, or claim that may be made by its manufacturer, is not guaranteed or endorsed by the publisher.
The Supplementary Material for this article can be found online at: https://www.frontiersin.org/articles/10.3389/fcomm.2023.1275127/full#supplementary-material
Appleby, J. (2023). Waiting lists: the return of a chronic condition? BMJ 380, 20. doi: 10.1136/bmj.p20
Ayers, J. W., Poliak, A., Dredze, M., Leas, E. C., Zhu, Z., Kelley, J. B., et al. (2023). Comparing physician and artificial intelligence chatbot responses to patient questions posted to a public social media forum. JAMA Int. Med. doi: 10.1001/jamainternmed.2023.1838
Baker, A., Perov, Y., Middleton, K., Baxter, J., Mullarkey, D., Sangar, D., et al. (2020). A comparison of artificial intelligence and human doctors for the purpose of triage and diagnosis. Front. Artif..Int. 3, 543405. doi: 10.3389/frai.2020.543405
Bates, M. (2019). Health care chatbots are here to help. IEEE Pulse 10, 12–14. doi: 10.1109/MPULS.2019.2911816
Battineni, G., Baldoni, S., Chintalapudi, N., Sagaro, G. G., Pallotta, G., Nittari, G., et al. (2020). Factors affecting the quality and reliability of online health information. Dig. Health 6, 2055207620948996. doi: 10.1177/2055207620948996
Brandon, M. (2016). Psychosocial aspects of sexuality with aging. Topics Geriatr. Rehab. 32, 151–155. doi: 10.1097/TGR.0000000000000116
Branley-Bell, D., and Murphy-Morgan, C. (2023). Designing remote care services for eating disorders: HCI considerations and provocations based upon service user experience and requirements. Preprint. doi: 10.31234/osf.io/fxepc
Bridgeman, P. J., Bridgeman, M. B., and Barone, J. (2018). Burnout syndrome among healthcare professionals. The Bullet. Am. Soc. Hosp. Pharm. 75, 147–152. doi: 10.2146/ajhp170460
Brown, R., Coventry, L., Sillence, E., Blythe, J., Stumpf, S., Bird, J., et al. (2022a). Collecting and sharing self-generated health and lifestyle data: understanding barriers for people living with long-term health conditions–a survey study. Digital Health 8, 20552076221084458. doi: 10.1177/20552076221084458
Brown, R., Sillence, E., Coventry, L., Simpson, E., Tariq, S., Gibbs, J., et al. (2022b). Understanding the attitudes and experiences of people living with potentially stigmatised long-term health conditions with respect to collecting and sharing health and lifestyle data. Digital Health. 8, 798. doi: 10.1177/20552076221089798
Burbury, K., Brooks, P., Gilham, L., Solo, I., Piper, A., Underhill, C., et al. (2022). Telehealth in cancer care during the COVID-19 pandemic. J. Telemed. Telecare 14, 1357633X221136305. doi: 10.1177/1357633X221136305
Choudhury, A., and Shamszare, H. (2023). Investigating the impact of user trust on the adoption and use of chatgpt: survey analysis. J. Med. Int. Res. 25, e47184. doi: 10.2196/47184
Ciechanowski, L., Przegalinska, A., Magnuski, M., and Gloor, P. (2019). In the shades of the uncanny valley: an experimental study of human–chatbot interaction. Future Gen. Comput. Syst. 92, 539–548. doi: 10.1016/j.future.2018.01.055
Dahiya, M. (2017). A tool of conversation: Chatbot. Int. J. Comput. Sci. Eng. 5, 158–161. Available online at: https://www.researchgate.net/profile/Menal-Dahiya/publication/321864990_A_Tool_of_Conversation_Chatbot/links/5a360b02aca27247eddea031/A-Tool-of-Conversation-Chatbot.pdf
Daraz, L., Morrow, A. S., Ponce, O. J., Beuschel, B., Farah, M. H., Katabi, A., et al. (2019). Can patients trust online health information? A meta-narrative systematic review addressing the quality of health information on the internet. J. Gen. Int. Med. 34, 1884–1891. doi: 10.1007/s11606-019-05109-0
Department of Health Social Care (2023). NHS Recovery Summit Held to Help Cut Waiting Lists. Available online at: https://www.gov.uk/government/news/nhs-recovery-summit-held-to-help-cut-waiting-lists (accessed August 1, 2023).
Desborough, J., Dykgraaf, S. H., Sturgiss, E., Parkinson, A., Dut, G., Kidd, M., et al. (2022). What has the COVID-19 pandemic taught us about the use of virtual consultations in primary care? Austr. J. Gen. Prac. 51, 179–183. doi: 10.31128/AJGP-09-21-6184
Dimitropoulos, G., Freeman, V. E., Muskat, S., Domingo, A., and McCallum, L. (2016). “You don't have anorexia, you just want to look like a celebrity”: perceived stigma in individuals with anorexia nervosa. J. Mental Health 25, 47–54. doi: 10.3109/09638237.2015.1101422
Drees, J. (2019). Google Receives More Than 1 Billion Health Questions Every Day. Becker's Health IT. Available online at: https://www.beckershospitalreview.com/healthcare-information-technology/google-receives-more-than-1-billion-health-questions-every-day.html#:~:text=An%20estimated%207%20percent%20of%20Google%27s%20daily%20searches,to%2070%2C000%20each%20minute%2C%20according%20to%20the%20report (accessed August 1, 2023).
Earnshaw, V. A., and Chaudoir, S. R. (2009). From conceptualizing to measuring HIV stigma: a review of HIV stigma mechanism measures. AIDS Behav. 13, 1160–1177. doi: 10.1007/s10461-009-9593-3
Frick, N. R., Brünker, F., Ross, B., and Stieglitz, S. (2021). Comparison of disclosure/concealment of medical information given to conversational agents or to physicians. Health Inf. J. 27, 1460458221994861. doi: 10.1177/1460458221994861
Gilbert, S., Harvey, H., Melvin, T., Vollebregt, E., and Wicks, P. (2023). Large language model AI chatbots require approval as medical devices. Nat. Med. 24, 1–3. doi: 10.1038/s41591-023-02412-6
Holthöwer, J., and van Doorn, J. (2022). Robots do not judge: service robots can alleviate embarrassment in service encounters. J. Acad. Market. Sci. 14, 1–18. doi: 10.1007/s11747-022-00862-x
Hood, J. E., and Friedman, A. L. (2011). Unveiling the hidden epidemic: a review of stigma associated with sexually transmissible infections. Sexual Health 8, 159–170. doi: 10.1071/SH10070
Hope, R. (2013). Rmisc: Rmisc: Ryan Miscellaneous. R Package Version 1, 5. Available online at: https://CRAN.R-project.org/package=Rmisc (accessed August 1, 2023).
Hopkins, A. M., Logan, J. M., Kichenadasse, G., and Sorich, M. J. (2023). Artificial intelligence chatbots will revolutionize how cancer patients access information: ChatGPT represents a paradigm-shift. JNCI Cancer Spectr. 7, pkad010. doi: 10.1093/jncics/pkad010
House of Commons Health Social Care Committee. (2021). Workforce Burnout and Resilience in the NHS and Social Care. Available online at: https://committees.parliament.uk/publications/6158/documents/68766/default/ (accessed August 1, 2023).
Khan, R., Das, A., Khan, R., and Das, A. (2018). Introduction to chatbots. Build Comp. Guide Chatbots 4, 1–11. doi: 10.1007/978-1-4842-3111-1_1
Khasawneh, O. Y. (2018). Technophobia: examining its hidden factors and defining it. Technol. Soc. 54, 93–100. doi: 10.1016/j.techsoc.2018.03.008
Kim, T. W., Jiang, L., Duhachek, A., Lee, H., and Garvey, A. (2022). Do You mind if i ask you a personal question? How AI service agents alter consumer self-disclosure. J. Serv. Res. 25, 649–666. doi: 10.1177/10946705221120232
Labacher, L., and Mitchell, C. (2013). Talk or text to tell? How young adults in Canada and South Africa prefer to receive STI results, counseling, and treatment updates in a wireless world. J. Health Commun. 18, 1465–1476. doi: 10.1080/10810730.2013.798379
Lee, A. S., and Cody, S. L. (2020). The stigma of sexually transmitted infections. Nursing Clin. 55, 295–305. doi: 10.1016/j.cnur.2020.05.002
Lee, N. M., and Frodsham, L. C. (2019). Sexual Pain Disorders. Introduction to Psychosexual Medicine. London: CRC Press, 164–171.
Lee, P., Bubeck, S., and Petro, J. (2023). Benefits, limits, and risks of GPT-4 as an AI chatbot for medicine. New England J. Med. 388, 1233–1239. doi: 10.1056/NEJMsr2214184
Liu, N. F., Brown, A. S., Folias, A. E., Younge, M. F., Guzman, S. J., Close, K. L., et al. (2017). Stigma in people with type 1 or type 2 diabetes. Clin. Diab. 35, 27–34. doi: 10.2337/cd16-0020
Manninen, S. M., Kero, K., Perkonoja, K., Vahlberg, T., and Polo-Kantola, P. (2021). General practitioners' self-reported competence in the management of sexual health issues–a web-based questionnaire study from Finland. Scand. J. Prim. Health Care 39, 279–287. doi: 10.1080/02813432.2021.1934983
Martel, R., Crawford, R., and Riden, H. (2017). ‘By the way…. how's your sex life?'–A descriptive study reporting primary health care registered nurses engagement with youth about sexual health. J. Prim. Health Care 9, 22–28. doi: 10.1071/HC17013
Matulis, J., and McCoy, R. (2023). Relief in sight? Chatbots, in-baskets, and the overwhelmed primary care clinician. J. Gen. Int. Med. 7, 1–8. doi: 10.1007/s11606-023-08271-8
McDonagh, L. K., Nielsen, E. J., McDermott, D. T., Davies, N., and Morrison, T. G. (2018). “I want to feel like a full man”: conceptualizing gay, bisexual, and heterosexual men's sexual difficulties. The J. Sex Res. 55, 783–801. doi: 10.1080/00224499.2017.1410519
McIntyre, D., and Chow, C. K. (2020). Waiting time as an indicator for health services under strain: a narrative review. INQUIRY J. Care Org., Prov. Financ. 57, 0046958020910305. doi: 10.1177/0046958020910305
Meuter, M. L., Ostrom, A. L., Bitner, M. J., and Roundtree, R. (2003). The influence of technology anxiety on consumer use and experiences with self-service technologies. J. Bus. Res. 56, 899–906. doi: 10.1016/S0148-2963(01)00276-4
Moriuchi, E. (2022). Leveraging the science to understand factors influencing the use of AI-powered avatar in healthcare services. J. Technol. Behav. Sci. 7, 588–602. doi: 10.1007/s41347-022-00277-z
Muftić, F., Kaduni,ć, M., Mušinbegović, A., and Abd Almisreb, A. (2023). Exploring medical breakthroughs: a systematic review of chatgpt applications in healthcare. Southeast Eur. J. Soft Computi. 12, 13–41.
Nadarzynski, T., Knights, N., Husbands, D., Graham, C. G., Llewellyn, C. D., Buchanan, T., et al. (2023). Chatbot-Assisted Self-Assessment (CASA): Designing a Novel AI-Enabled Sexual Health Intervention for Racially Minoritised Communities. London: BMJ Publishing Group Ltd.
Nadarzynski, T., Puentes, V., Pawlak, I., Mendes, T., Montgomery, I., Bayley, J., et al. (2021). Barriers and facilitators to engagement with artificial intelligence (AI)-based chatbots for sexual and reproductive health advice: a qualitative analysis. Sexual Health 18, 385–393. doi: 10.1071/SH21123
NHS England (2022). 2022./23 Priorities and Operational Planning Guidance. Available online at: https://www.england.nhs.uk/wp-content/uploads/2022/02/20211223-B1160-2022-23-priorities-and-operational-planning-guidance-v3.2.pdf (accessed August 1, 2023).
Nov, O., Singh, N., and Mann, D. M. (2023). Putting ChatGPT's medical advice to the (Turing) test. medRxiv 2001, 23284735. doi: 10.2139/ssrn.4413305
Nyblade, L., Stangl, A., Weiss, E., and Ashburn, K. (2009). Combating HIV stigma in health care settings: what works? J. Int. AIDS Soc. 12, 1–7. doi: 10.1186/1758-2652-12-15
Office for National Statistics. (2023). Data and Analysis From Census 2021. Available online at: https://www.ons.gov.uk/census (accessed August 1, 2023).
Oliver, D. (2022). David Oliver: can the recovery plan for elective care in England deliver? BMJ 376, 724. doi: 10.1136/bmj.o724
OpenAI. (2023). ChatGPT-3, 5. Available online at: https://chat.openai.com/chat (accessed August 1, 2023).
Prolific. (2023). Representative Samples. Available online at: https://researcher-help.prolific.co/hc/en-gb/articles/360019236753-Representative-samples (accessed August 1, 2023).
R Core. (2021). Core R: A Language and Environment for Statistical Computing. Vienna: R Foundation for Statistical Computing.
Rasmussen, B., Perry, R., Hickey, M., Hua, X., Wong, Z. W., Guy, L., et al. (2022). Patient preferences using telehealth during the COVID-19 pandemic in four Victorian tertiary hospital services. Int. Med. J. 52, 763–769. doi: 10.1111/imj.15726
Revelle, W. (2021). psych: Procedures for Personality and Psychological Research (Version R Package Version 2.0, 12.). Evanston, IL: Northwestern University.
Ripley, B., Venables, W., and Ripley, M. B. (2016). nnet: Feed-Forward Neural Networks and Multinomial Log-Linear Models (R Package Version 7, 700). Available online at: https://cran.r-project.org/web/packages/nnet/index.html
Rosruen, N., and Samanchuen, T. (2018). “Chatbot utilization for medical consultant system,” in 2018 3rd Technology Innovation Management and Engineering Science International Conference (TIMES-iCON), London.
Schabert, J., Browne, J. L., Mosely, K., and Speight, J. (2013). Social stigma in diabetes: a framework to understand a growing problem for an increasing epidemic. Patient Cent. Outcomes Res. 6, 1–10. doi: 10.1007/s40271-012-0001-0
Sheehan, L., and Corrigan, P. (2020). Stigma of disease and its impact on health. The Wiley Encycl. Health Psychol. 28, 57–65. doi: 10.1002/9781119057840.ch139
Simpson, E., Brown, R., Sillence, E., Coventry, L., Lloyd, K., Gibbs, J., et al. (2021). Understanding the barriers and facilitators to sharing patient-generated health data using digital technology for people living with long-term health conditions: a narrative review. Front. Pub. Health 9, 1747. doi: 10.3389/fpubh.2021.641424
Softić, A., Husić, J. B., Softić, A., and Baraković, S. (2021). “Health chatbot: design, implementation, acceptance and usage motivation,” in 2021 20th International Symposium INFOTEH-JAHORINA (INFOTEH), Bosnia.
Sumpton, D., Oliffe, M., Kane, B., Hassett, G., Craig, J. C., Kelly, A., et al. (2022). Patients' perspectives on shared decision-making about medications in psoriatic arthritis: an interview study. Arthritis Care Res. 74, 2066–2075. doi: 10.1002/acr.24748
Szollosy, M. (2017). Freud, Frankenstein and our fear of robots: projection in our cultural perception of technology. AI Soc. 32, 433–439. doi: 10.1007/s00146-016-0654-7
Taber, K. S. (2018). The use of cronbach's alpha when developing and reporting research instruments in science education. Res. Sci. Educ. 48, 1273–1296. doi: 10.1007/s11165-016-9602-2
Taliercio, V. L., Snyder, A. M., Webber, L. B., Langner, A. U., Rich, B. E., Beshay, A. P., et al. (2021). The disruptiveness of itchiness from psoriasis: a qualitative study of the impact of a single symptom on quality of life. J. Clin. Aesthet. Dermatol. 14, 42.
Temsah, O., Khan, S. A., Chaiah, Y., Senjab, A., Alhasan, K., Jamal, A., et al. (2023). Overview of early ChatGPT's presence in medical literature: insights from a hybrid literature review by ChatGPT and human experts. Cureus 15, 4. doi: 10.7759/cureus.37281
Wickham, H., Averick, M., Bryan, J., Chang, W., McGowan, L., François, R., et al. (2020). Welcome to the Tidyverse. J. Open Source Softw. 4, 1686. doi: 10.21105/joss.01686
Keywords: health communication, human computer interaction, chatbots, health stigma, health information seeking, artificial intelligence, doctor-patient communication, healthcare
Citation: Branley-Bell D, Brown R, Coventry L and Sillence E (2023) Chatbots for embarrassing and stigmatizing conditions: could chatbots encourage users to seek medical advice? Front. Commun. 8:1275127. doi: 10.3389/fcomm.2023.1275127
Received: 09 August 2023; Accepted: 11 September 2023;
Published: 27 September 2023.
Edited by:
Sylvie Grosjean, University of Ottawa, CanadaReviewed by:
Aleksandra Gaworska-Krzeminska, Medical University of Gdańsk, PolandCopyright © 2023 Branley-Bell, Brown, Coventry and Sillence. This is an open-access article distributed under the terms of the Creative Commons Attribution License (CC BY). The use, distribution or reproduction in other forums is permitted, provided the original author(s) and the copyright owner(s) are credited and that the original publication in this journal is cited, in accordance with accepted academic practice. No use, distribution or reproduction is permitted which does not comply with these terms.
*Correspondence: Richard Brown, cmljaGFyZDYuYnJvd25Abm9ydGh1bWJyaWEuYWMudWs=
Disclaimer: All claims expressed in this article are solely those of the authors and do not necessarily represent those of their affiliated organizations, or those of the publisher, the editors and the reviewers. Any product that may be evaluated in this article or claim that may be made by its manufacturer is not guaranteed or endorsed by the publisher.
Research integrity at Frontiers
Learn more about the work of our research integrity team to safeguard the quality of each article we publish.