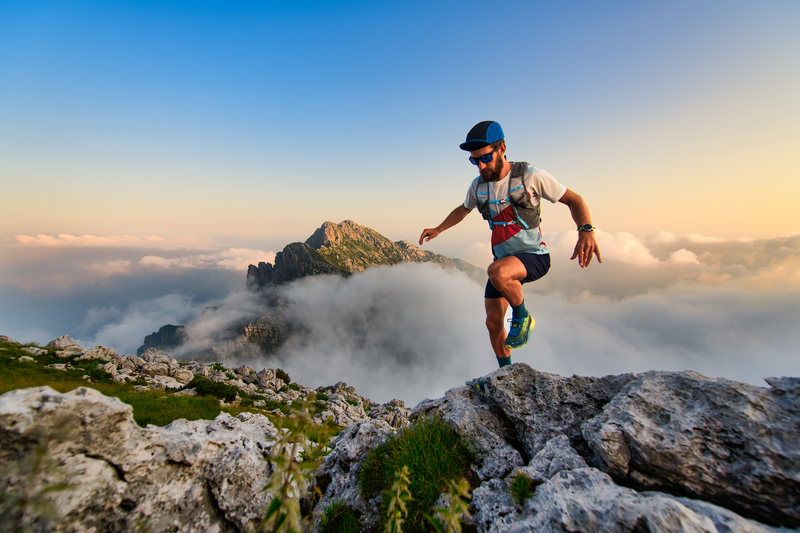
95% of researchers rate our articles as excellent or good
Learn more about the work of our research integrity team to safeguard the quality of each article we publish.
Find out more
PERSPECTIVE article
Front. Commun. , 12 September 2023
Sec. Media Governance and the Public Sphere
Volume 8 - 2023 | https://doi.org/10.3389/fcomm.2023.1243474
The proliferation of artificial intelligence (AI) in digital platforms has complicated the concept of truth in communication studies. The article presents the dichotomic framework of Front-end AI and Back-end AI to tackle the complexity of distinguishing truth. Front-end AI refers to AI technology used up-front, often as the face of a product or service, challenging the authenticity and truthfulness of content. In contrast, Back-end AI refers to AI technology used behind the scenes, which can generate misleading or biased content without disclosing its AI-generated nature. Addressing these challenges requires different approaches, such as verification and ethical guidelines for Front-end AI and algorithmic transparency, bias detection, and human oversight for Back-end AI.
With the proliferation of artificial intelligence (AI) on digital platforms, the concept of truth in communication studies has become increasingly complex. By mimicking the tone, structure, and style of authentic human writing, AI-generated content (Wu et al., 2020), such as news articles and social media posts, can create a false impression of credibility. This complicates the process of distinguishing between authentic and fabricated information. In addition, social bots (Ferrara et al., 2016) can manipulate online discourse by mimicking human behavior, such as liking, sharing, and retweeting content. During political campaigns, for instance, these bots can disseminate false information or biased opinions to influence public sentiment, making it difficult to distinguish between genuine public opinion and perspectives generated artificially. AI is now capable of generating convincingly realistic content (Caporusso, 2021; Lim and Schmälzle, 2023), including text (e.g., GPT), images (e.g., DALL-E), and speech (e.g., Whisper). Deepfakes, synthetic media in which a person's image or voice is digitally altered to resemble another person (Johnson and Diakopoulos, 2021), have further blurred the line between reality and fabrication.
The creation of virtual identities further complicates discerning the truth in communication. These identities can engage in online discourse while obscuring their artificial origins and promoting particular viewpoints (Ross et al., 2019). For instance, virtual influencers on social media platforms can generate followers and shape opinions without the audience realizing they are not interacting with a real person (Kim and Wang, 2023). The opaque nature of AI algorithms can perpetuate and amplify existing societal biases, eroding confidence in the results (Durán and Jongsma, 2021). In hiring processes, for instance, biased AI algorithms may systematically discriminate against minority applicants, reinforcing societal inequalities. The difficulty in evaluating the dependability and precision of AI results (von Eschenbach, 2021) exacerbates the problem by making it difficult to determine whether a given result is trustworthy. This article will introduce a dichotomous framework of Front-end AI and Back-end AI to address these complexities. Front-end AI focuses on enhancing the transparency and explainability of AI-generated content. In contrast, Back-end AI seeks to improve the underlying algorithms to reduce biases and increase precision. By addressing these two facets of AI, distinguishing truth amidst the rapid development of AI technologies will be made more manageable.
There are two main categories of AI regarding truth in communication: Front-end AI and Back-end AI. Front-end AI refers to AI technology used up-front, often as the face of a product or service. One example of Front-end AI is virtual influencers (Kim and Wang, 2023). These computer-generated images of people are intended to appear and behave like real people, complete with distinct personalities and viewpoints. However, since these virtual influencers are not actual people, their authenticity and communication veracity are questioned. For instance, a virtual influencer promoting a product may not have personal experience with the item, leading to endorsements that may be misleading or superficial. Due to the AI-generated nature of virtual influencers' content, it may not be based on genuine opinions or experiences, which makes it difficult to determine the integrity and accountability of communication. It may even lead to psychological problems such as gender stereotypes (Zakharchenko et al., 2020) and body image concerns (Kim and Kim, 2023).
On the other hand, back-end AI refers to AI technology used in the background to influence communication without direct user interaction. An example of a Back-end AI is Chat-GPT, an Open AI-trained language model designed to simulate human conversation (Reynolds and McDonell, 2021). Unlike traditional chatbots (Kim, 2021), followers may be misled when individuals use Chat GPT to express opinions on social media without disclosing the use of AI-generated language. Moreover, although Chat GPT's responses may appear genuine, they may not always be entirely truthful or accurate, particularly if the input it receives is biased or insufficient. This situation can create a false sense of authenticity and authority, which can be exploited to disseminate incorrect information or improperly influence public opinion (Gerlich et al., 2023).
Although their applications are distinct, both Front-end AI and Back-end AI can potentially complicate the notion of truth in communication. As AI technology advances, consumers and businesses must be aware of AI-generated communication's potential biases and limitations to ensure that communication remains as honest as possible. By examining the implications of Front-end AI and Back-end AI on disinformation and privacy, this article aims to shed light on how these two types of AI shape the truth in communication and the challenges they pose in the context of these crucial issues.
Disinformation, a form of communication that intentionally misleads or presents falsehoods, manifests in various ways, such as fabricated news stories, propaganda, and unfounded rumors (Benkler et al., 2018). Disinformation campaigns aim to manipulate public opinion or incite confusion and mistrust. They often proliferate on social media platforms where users rapidly share content without verifying its accuracy (Di Domenico et al., 2021). Consequently, disinformation has spread false information that is difficult to counter and carries significant repercussions for society. Upholding the concept of truth is crucial in combating disinformation. The belief in an objective reality, independent of individual opinions or perspectives, necessitates meticulously examining facts and establishing effective communication strategies (Newman and Gopalkrishnan, 2023).
Front-end AI, including virtual influencers and social bots, contributes to disinformation by raising concerns about the authenticity and truthfulness of the content they generate. As these entities lack human qualities, their content may be perceived as misleading or manipulative (Ferrara et al., 2016; Dwivedi et al., 2022). For instance, during the 2016 US presidential election, social bots disseminated politically-biased misinformation, creating confusion among voters and skewing public discourse (Shao et al., 2018; Rozado, 2023). Audiences often struggle to distinguish virtual influencers' content from real humans, making it unclear whether the opinions expressed are based on genuine experiences or merely marketing tactics. This ambiguity becomes particularly problematic when a social bot amasses a large following, as its views are amplified and widely circulated (Ferrara, 2017), perpetuating disinformation and confusion among consumers.
Back-end AI can further exacerbate disinformation when AI algorithms are trained using incomplete or biased data. The validity of predictions made by these algorithms hinges on the comprehensiveness of the data used for training. For example, a widely-used AI recruitment tool exhibited gender bias (Gross, 2023) due to training on male-dominated resume data, leading to the inadvertent promotion of gender inequality (Dastin, 2018). However, when people encounter such predictions, they may not know if AI generated them, resulting in disinformation and confusion. As disinformation continues to pose a significant challenge to society, countering it requires increased scrutiny of AI-generated content and reinforcing the concept of truth through thorough fact-checking and well-crafted communication strategies.
Privacy, the right to control access to one's personal information, has become increasingly complex in the digital age due to the pervasive collection and use of personal data by both private companies and governments (Schwartz, 2003). This complexity arises from various practices, such as targeted advertising, content personalization, and behavioral monitoring, which rely on extensive data collection. For instance, third-party tracking codes on websites exemplify how privacy can be compromised in the digital landscape. These codes are frequently embedded without users' knowledge or consent, enabling companies to monitor individuals' online activities across multiple platforms, including mobile devices and applications (Mayer and Mitchell, 2012). This invasive tracking can lead to significant risks to personal safety, as demonstrated by cases where individuals' location or medical information has been used against them for insurance purposes (Barocas and Selbst, 2016). Nonetheless, there are alternative digital advertising methods that do not require collecting personal data. Search ads, for example, provide relevant content based on a user's immediate query rather than personal information (Yoo, 2014). This demonstrates that it is possible to maintain privacy while still engaging in effective advertising.
Furthermore, the increasing use of AI technologies on the front-end and back-end raises additional privacy concerns. Open dialogue chatbots, which engage users in free-form conversations, can inadvertently collect and utilize a wealth of personal data (Hasal et al., 2021). This is particularly concerning since these chatbots are often trained on previous conversations, potentially exposing sensitive information to theft or misuse, even when no directly identifiable information is present. Back-end AI technologies, such as facial recognition and other surveillance systems, also present significant privacy concerns. These technologies can surreptitiously collect and use personal data without the knowledge or consent of the individuals involved (Almeida et al., 2022). This compromises individual privacy and raises critical ethical and legal questions regarding the appropriate use of such technologies (Gates, 2011). Consequently, there is an urgent need to develop and implement robust safeguards that protect privacy while allowing for the responsible use of AI and other data-driven technologies in the digital realm (Burr and Leslie, 2022).
The problems of truth in communication with Front-end AI and Back-end AI require different solutions. While concerns regarding Front-end AI are focused on the message receivers, the solutions of Back-end AI are on the message senders. While Front-end AI may require verification and ethical guidelines, Back-end AI may require algorithmic transparency, bias detection and mitigation, and human oversight. By approaching the issues of disinformation and privacy with this framework, we can ensure that their use of AI is responsible, ethical, and authentic.
One approach to addressing the authenticity of Front-end AI-generated content is through verification. This may involve creating a system for verifying virtual influencers' or social bots' authenticity. For example, a digital watermark or certification system could be implemented to confirm the origin of the content (Westerlund, 2019). This can help build trust and ensure that individuals can rely on the information presented to them. Establishing ethical guidelines for using Front-end AI can also help prevent the misuse of these technologies. These guidelines may address issues such as privacy, transparency, and bias. For instance, rules could be established to prohibit using AI-generated deepfakes for malicious purposes (Wojewidka, 2020) or the unauthorized use of personal data in AI-generated content. Consumers' education about the comprehensive implementation of Front-end AI can also help to prevent misunderstandings and promote authentic communication. Individuals can better understand AI-generated content's limitations and potential biases by providing information about how virtual influencers or open-dialogue chatbots are created. For example, interactive educational materials or public awareness campaigns can help inform users about how AI algorithms function (Shin et al., 2022) and the potential pitfalls of relying solely on AI-generated content.
For addressing the truthfulness of Back-end AI-generated content, involving algorithmic transparency in the inner workings of AI algorithms more transparent to users is essential. By providing users with information about how AI systems make decisions, they can better understand the accuracy and reliability of the results. For instance, companies could publish whitepapers detailing the methodologies and data sources used in their AI models or provide user-friendly explanations of the AI's decision-making process (Goodman and Flaxman, 2017). To prevent Back-end AI from perpetuating biases, companies should also prioritize bias detection and mitigation in developing these technologies. This may involve using diverse and representative data sets to train AI systems and implement bias detection algorithms to identify and correct any present biases. For example, companies could collaborate with external organizations specializing in bias detection and fairness in AI (Bellamy et al., 2019) to ensure that their systems do not inadvertently discriminate against specific demographic groups.
Implementing human oversight through human-in-the-loop using Back-end AI can help ensure that interactions remain truthful and authentic. This may involve having human moderators review and approve the results generated by AI systems or having customer service representatives available to intervene when needed (Gillespie, 2020). For example, in a customer support scenario, AI-generated responses could be flagged for review by a human agent before being sent to the customer, ensuring that the information provided is accurate and contextually relevant. Combining human judgment with AI capabilities can achieve a more reliable and authentic communication process.
In conclusion, the complexities surrounding truth in communication caused by the rapid development of AI technologies, such as Front-end AI and Back-end AI, necessitate innovative solutions and heightened vigilance. The process of distinguishing truth can be made more manageable by enhancing the transparency, explainability, and ethical use of AI-generated content in Front-end AI and improving the underlying algorithms to reduce biases and increase precision in Back-end AI. To maintain authentic and ethical communication in the digital age, addressing the obstacles posed by disinformation and privacy concerns is essential. As AI develops, consumers and businesses must be aware of AI-generated communication's possible biases, limitations, and ethical implications. This can be accomplished through verification, ethical guidelines, algorithmic transparency, bias detection and mitigation, and human oversight. By adopting a comprehensive and proactive approach to addressing the challenges posed by AI in communication, society will be able to navigate the blurred lines between reality and fabrication and foster a digital landscape in which truth and authenticity remain paramount.
The original contributions presented in the study are included in the article/supplementary material, further inquiries can be directed to the corresponding author.
DK: study conception, manuscript draft preparation, and final manuscript writing. JK: manuscript revision.
The authors declare that the research was conducted in the absence of any commercial or financial relationships that could be construed as a potential conflict of interest.
All claims expressed in this article are solely those of the authors and do not necessarily represent those of their affiliated organizations, or those of the publisher, the editors and the reviewers. Any product that may be evaluated in this article, or claim that may be made by its manufacturer, is not guaranteed or endorsed by the publisher.
Almeida, D., Shmarko, K., and Lomas, E. (2022). The ethics of facial recognition technologies, surveillance, and accountability in an age of artificial intelligence: a comparative analysis of US, EU, and UK regulatory frameworks. AI and Ethics 2, 377–387. doi: 10.1007/s43681-021-00077-w
Barocas, S., and Selbst, A. D. (2016). Big data's disparate impact. Calif. Law Rev. 2016, 671–732. doi: 10.2139/ssrn.2477899
Bellamy, R. K., Dey, K., Hind, M., Hoffman, S. C., Houde, S., Kannan, K., et al. (2019). AI Fairness 360: An extensible toolkit for detecting and mitigating algorithmic bias. IBM J. Res. Dev. 63, 4-1. doi: 10.1147/JRD.2019.2942287
Benkler, Y., Faris, R., and Roberts, H. (2018). Network Propaganda: Manipulation, Disinformation, and Radicalization in American Politics. Oxford: Oxford University Press.
Burr, C., and Leslie, D. (2022). Ethical assurance: a practical approach to the responsible design, development, and deployment of data-driven technologies. AI and Ethics 2022, 1–26. doi: 10.1007/s43681-022-00178-0
Caporusso, N. (2021). “Deepfakes for the good: A beneficial application of contentious artificial intelligence technology,” in Advances in Artificial Intelligence, Software and Systems Engineering: Proceedings of the AHFE 2020 Virtual Conferences on Software and Systems Engineering, and Artificial Intelligence and Social Computing, July 16-20, 2020, USA. Cham: Springer International Publishing, 235–241.
Dastin, J. (2018). “Amazon scraps secret AI recruiting tool that showed bias against women,” in Ethics of Data and Analytics. Boca Ratoon: Auerbach Publications, 296–299.
Di Domenico, G., Sit, J., Ishizaka, A., and Nunan, D. (2021). Fake news, social media and marketing: a systematic review. J. Bus. Res. 124, 329–341. doi: 10.1016/j.jbusres.2020.11.037
Durán, J. M., and Jongsma, K. R. (2021). Who is afraid of black box algorithms? On the epistemological and ethical basis of trust in medical AI. J. Med. Ethics 47, 329–335. doi: 10.1136/medethics-2020-106820
Dwivedi, Y. K., Hughes, L., Baabdullah, A. M., Ribeiro-Navarrete, S., Giannakis, M., Al-Debei, M. M., et al. (2022). Metaverse beyond the hype: multidisciplinary perspectives on emerging challenges, opportunities, and agenda for research, practice and policy. Int. J. Inf. Manage. 66, 102542. doi: 10.1016/j.ijinfomgt.2022.102542
Ferrara, E. (2017). Disinformation and Social Bot Operations in the Run Up to the 2017 French Presidential Election, Vol. 22. First Monday. doi: 10.5210/fm.v22i8.8005
Ferrara, E., Varol, O., Davis, C., Menczer, F., and Flammini, A. (2016). The rise of social bots. Commun. ACM. 59, 96–104. doi: 10.1145/2818717
Gates, K. A. (2011). Our biometric future: Facial recognition technology and the culture of surveillance (Vol. 2). NYU Press.
Gerlich, M., Elsayed, W., and Sokolovskiy, K. (2023). Artificial intelligence as toolset for analysis of public opinion and social interaction in marketing: identification of micro and nano influencers. Front. Commun. 8, 1075654. doi: 10.3389/fcomm.2023.1075654
Gillespie, T. (2020). Content moderation, AI, and the question of scale. Big Data Soc. 7, 2053951720943234. doi: 10.1177/2053951720943234
Goodman, B., and Flaxman, S. (2017). European Union regulations on algorithmic decision-making and a “right to explanation”. AI Magazine 38, 50–57. doi: 10.1609/aimag.v38i3.2741
Gross, N. (2023). What ChatGPT tells us about gender: a cautionary tale about performativity and gender biases in AI. Soc. Sci. 12, 435. doi: 10.3390/socsci12080435
Hasal, M., Nowaková, J., Ahmed Saghair, K., Abdulla, H., Snášel, V., and Ogiela, L. (2021). Chatbots: Security, privacy, data protection, and social aspects. Concurr. Comput. 33, e6426. doi: 10.1002/cpe.6426
Johnson, D. G., and Diakopoulos, N. (2021). What to do about deepfakes. Commun. ACM. 64, 33–35. doi: 10.1145/3447255
Kim, D. (2021). Siri as an Animated Agent: Intention to Disclose Personal Information to an Intelligent Virtual Assistant (Doctoral dissertation). University of Texas at Austin.
Kim, D., and Kim, S. (2023). Social media affordances of ephemerality and permanence: social comparison, self-esteem, and body image concerns. Soc. Sci. 12, 87. doi: 10.3390/socsci12020087
Kim, D., and Wang, Z. (2023). The ethics of virtuality: navigating the complexities of human-like virtual influencers in the social media marketing realm. Front. Commun. 8, 1205610. doi: 10.3389/fcomm.2023.1205610
Lim, S., and Schmälzle, R. (2023). Artificial intelligence for health message generation: an empirical study using a large language model (LLM) and prompt engineering. Front. Commun. 8, 1129082. doi: 10.3389/fcomm.2023.1129082
Mayer, J. R., and Mitchell, J. C. (2012). “Third-party web tracking: policy and technology,” in 2012 IEEE Symposium on Security and Privacy. San Francisco, CA: IEEE, 413–427.
Newman, S. A., and Gopalkrishnan, S. (2023). The prospect of digital human communication for organizational purposes. Front. Commun. 8, 1200985. doi: 10.3389/fcomm.2023.1200985
Reynolds, L., and McDonell, K. (2021). “Prompt programming for large language models: beyond the few-shot paradigm,” in Extended Abstracts of the 2021 CHI Conference on Human Factors in Computing Systems, 1–7.
Ross, B., Pilz, L., Cabrera, B., Brachten, F., Neubaum, G., and Stieglitz, S. (2019). Are social bots a real threat? An agent-based model of the spiral of silence to analyse the impact of manipulative actors in social networks. Eur. J. Infor. Syst. 28, 394–412. doi: 10.1080/0960085X.2018.1560920
Schwartz, P. M. (2003). Property, privacy, and personal data. Harv. L. Rev., 117, 2056. doi: 10.2307/4093335
Shao, C., Ciampaglia, G. L., Varol, O., Yang, K. C., Flammini, A., and Menczer, F. (2018). The spread of low-credibility content by social bots. Nat. Commun. 9, 1–9. doi: 10.1038/s41467-018-06930-7
Shin, D., Kee, K. F., and Shin, E. Y. (2022). Algorithm awareness: why user awareness is critical for personal privacy in the adoption of algorithmic platforms? Int. J. Inf. Manage. 65, 102494. doi: 10.1016/j.ijinfomgt.2022.102494
von Eschenbach, W. J. (2021). Transparency and the black box problem: why we do not trust AI. Philos. Technol. 34, 1607–1622. doi: 10.1007/s13347-021-00477-0
Westerlund, M. (2019). The emergence of deepfake technology: a review. Technol. Innov. Manag. Rev. 9, 11. doi: 10.22215/timreview/1282
Wojewidka, J. (2020). The deepfake threat to face biometrics. Biometric Technol. Today 2020, 5–7. doi: 10.1016/S0969-4765(20)30023-0
Wu, Y., Mou, Y., Li, Z., and Xu, K. (2020). Investigating American and Chinese subjects' explicit and implicit perceptions of AI-generated artistic work. Comput. Human Behav. 104, 106186. doi: 10.1016/j.chb.2019.106186
Yoo, C. Y. (2014). Branding potentials of keyword search ads: the effects of ad rankings on brand recognition and evaluations. J. Advert. 43, 85–99. doi: 10.1080/00913367.2013.845541
Keywords: truth, artificial intelligence, privacy, disinformation, communication, ethics, algorithmic transparency, bias detection
Citation: Kim D and Kong J (2023) Front-end AI vs. Back-end AI: new framework for securing truth in communication during the generative AI era. Front. Commun. 8:1243474. doi: 10.3389/fcomm.2023.1243474
Received: 20 June 2023; Accepted: 24 August 2023;
Published: 12 September 2023.
Edited by:
Pradeep Nair, Central University of Himachal Pradesh, IndiaReviewed by:
Solomiia Fedushko, Lviv Polytechnic, UkraineCopyright © 2023 Kim and Kong. This is an open-access article distributed under the terms of the Creative Commons Attribution License (CC BY). The use, distribution or reproduction in other forums is permitted, provided the original author(s) and the copyright owner(s) are credited and that the original publication in this journal is cited, in accordance with accepted academic practice. No use, distribution or reproduction is permitted which does not comply with these terms.
*Correspondence: Donggyu Kim, ZG9uZ2d5dWtAdXNjLmVkdQ==
Disclaimer: All claims expressed in this article are solely those of the authors and do not necessarily represent those of their affiliated organizations, or those of the publisher, the editors and the reviewers. Any product that may be evaluated in this article or claim that may be made by its manufacturer is not guaranteed or endorsed by the publisher.
Research integrity at Frontiers
Learn more about the work of our research integrity team to safeguard the quality of each article we publish.