- 1Institute of Informatics, School of Management, HES-SO Valais-Wallis University of Applied Sciences and Arts Western Switzerland, Sierre, Switzerland
- 2Nuclear Medicine and Molecular Imaging Department, Lausanne University Hospital (CHUV), Lausanne, Switzerland
- 3Faculty of Biology and Medicine, University of Lausanne (UNIL), Lausanne, Switzerland
Technologies based on “artificial intelligence” (AI) are transforming every part of our society, including healthcare and medical institutions. An example of this trend is the novel field in oncology and radiology called radiomics, which is the extracting and mining of large-scale quantitative features from medical imaging by machine-learning (ML) algorithms. This paper explores situated work with a radiomics software platform, QuantImage (v2), and interaction around it, in educationally framed hands-on trial sessions where pairs of novice users (physicians and medical radiology technicians) work on a radiomics task consisting of developing a predictive ML model with a co-present tutor. Informed by ethnomethodology and conversation analysis (EM/CA), the results show that learning about radiomics more generally and learning how to use this platform specifically are deeply intertwined. Common-sense knowledge (e.g., about meanings of colors) can interfere with the visual representation standards established in the professional domain. Participants' skills in using the platform and knowledge of radiomics are routinely displayed in the assessment of performance measures of the resulting ML models, in the monitoring of the platform's pace of operation for possible problems, and in the ascribing of independent actions (e.g., related to algorithms) to the platform. The findings are relevant to current discussions about the explainability of AI in medicine as well as issues of machinic agency.
1 Introduction
Understanding the social implications of “artificial intelligence” (AI) will be one of the most significant topics of this decade. The growing ubiquity of AI-based devices in everyday life and professional settings has prompted newly motivated critical scrutiny and provided an incentive to “describe how AI features in the world as it is” (Brooker et al., 2019; p. 296). AI-based technologies are also rapidly transforming healthcare and medical institutions, and they define the new field of medical imaging analysis known as radiomics—the extracting of large-scale quantitative features from medical imaging by AI algorithms (see Guiot et al., 2022 for a recent review). They furthermore form the basis for this article, which presents an observational user study of work with a radiomics software platform.
Radiomics holds the promise of allowing us to make better use of medical imaging for precision oncology (Gillies et al., 2016). It consists of extracting quantitative information from tumoral and metastatic tissue in order to build diagnostically or prognostically relevant scores that can exceed the capacity of the naked eye when it comes to predictions such as patient survival or risk of recurrence. The resulting scores can provide information that is valuable for orienting treatment options during multidisciplinary tumor board meetings in conjunction with other omics (Luchini et al., 2020). Radiomics relies on the following main steps: Image preprocessing, feature extraction, feature selection, machine-learning (ML) model creation, selection, and validation. The radiomics features can quantify specific visual aspects covering all types of image information: intensity (i.e., minimum, maximum, or average image values), texture, and shape of lesions, often beyond the capabilities of the naked eye. All these steps are carried out within a validation framework to faithfully estimate how the models will perform in the future. After a preprocessing of the initial images to standardize their units and dimensions, a large set of features are computed to quantify the intensity, shape, and texture of a given lesion. This large set of features is then reduced to limit fortuitous correlations and the curse of dimensionality. Finally, ML models are trained to predict the outcome of interest (e.g., overall survival, malignancy) and the best performing models are kept.
The study discussed in this article is based on the premise that the development of any discipline needs to be solidified through its teaching—as Peeken et al. (2018) conclude, it is necessary to “consider adapting the education curricula to include more radiomics related topics” (p. 33). This article explores how a software radiomics platform, QuantImage (v2), can be used in higher education to help students learn about the use of AI in oncology. QuantImage enables the extraction of several types of features from positron emission tomography/computed tomography (PET/CT) images, providing a simple and user-friendly environment that can be further adjusted for more refined analyses (Abler et al., 2023). Positioned within the steps of “explanatory analysis” and “modeling” in the broader workflow of radiomics (see Lambin et al., 2017), QuantImage allows researchers to select and visualize features according to their relationship with the considered outcome, and to construct ML models without the help of computer scientists. In this paper, we are focusing on the situated use of this technology in a medical setting framed as a software trial and educational session. Looking at how novice users work with the platform highlights practical knowledge and aspects of the activity that are taken for granted in the work of experts (see also Lindwall and Lymer, 2014). As noted by Lucy Suchman in the context of human–machine interaction, “by studying what things look like when they are unfamiliar, [we can] understand better what is involved in their mastery” (Suchman, 1987; p. 75). Our study addresses questions such as: How are the actions of the software made sense of and incorporated into the ongoing interaction? What makes the novice users' collaborative work with the platform specifically an instance of radiomics? How is their situated interaction with and around the AI-based platform organized?
Answers to these questions are sought through ethnomethodology and conversation analysis (see Garfinkel, 1967, 2002, 2022; Sacks, 1992; Schegloff, 2007; henceforth EM/CA). This distinctive approach to the study of human sociality has also been influential in research on technology-in-action (Suchman, 1987, 2007; Dourish and Button, 1998; Heath and Luff, 2000, 2022; Crabtree, 2004; Randall et al., 2021). EM/CA describes and explicates practical reasoning and practical action as members' methods in everyday and specialized environments, including the workplace and scientific contexts (Livingston, 1987; Lynch, 1993). With regard to digital technology, it investigates in detail how people work with technical devices, in situ and in real time, drawing on material, bodily, and verbal resources (e.g., Mlynář, 2021; Koivisto et al., 2023). In this article, we present and discuss findings from an EM/CA analysis of video-recorded software trial sessions conducted at Center hospitalier universitaire vaudois (CHUV) in Lausanne, Switzerland, where experts produced and interpreted radiomics results together with novice users with the use of QuantImage. Our study draws from and contributes to video-based interactionist studies of healthcare and hospital settings, which constitute a long-established field (Barnes, 2019; Keel, 2023), including oncology (Singh et al., 2017) and radiology (Rystedt et al., 2011). Although digital and AI-based technologies are often viewed as “tools” (Verma et al., 2021; Mlynář et al., 2022a), we aim to move beyond this reductive understanding. Rather than evaluating or assessing the use of the software, our paper examines how pairs of participants work together, and just what they do to achieve their locally relevant tasks. Focusing on the identification of recurrent patterns of interactional organization distinctive to interacting and working with QuantImage in the educational setting, we explore how novices learn “to see like an expert” (Gegenfurtner et al., 2019). The crux of our contribution is in studying the members' methods of interacting with each other and the platform in real time during situated interactional episodes in a healthcare setting. Moreover, we contribute by examining interactions with and around a non-tangible and non-anthropomorphic AI-powered device—generally understudied in EM/CA, which is mostly preoccupied with human-like, tangible, language-producing technologies such as robots or voice user interfaces (see Mlynář et al., 2022b).
For novice users in trial sessions, the platform is a proxy and a synecdoche of radiomics: radiomics as a wider domain of medical work is embodied and represented in and as the specific platform itself. In learning how to work with the platform, they discover and establish radiomics work procedures as part of the trial session with the help of the co-present expert acting as a tutor. As documented by the analysis below, novice users' troubles might be based in the organization of the current task, in the procedural details of the platform, or in their understanding of the broader domain of radiomics. Most importantly, knowledge of radiomics and knowledge of the specific platform reflexively establish each other, and this has significant implications for the integration of novel technologies into educational and medical praxis.
The article is organized as follows: Section 2 provides an overview of related work and Section 3 describes the setting and method. The analysis in Section 4 specifies the shared practical knowledge that constitutes the common ground of radiomics' work, as well as the “contingencies” (Garfinkel, 2022) of participants' courses of action while they deal with substantive or procedural “trouble,” both in radiomics more generally and in the platform specifically. In Section 5, we conclude that examining how these practices are incorporated into the interaction with and around AI-based platforms is an important step toward understanding how such technologies may not only become trustworthy and efficient as assistive tools, but in the longer run may also possibly be incorporated into more complex medical diagnostics and clinical decision-making.
2 Digital technology and AI in oncology practice
Digital technologies have been described as having a transformative impact on the practice of oncology and radiology in recent years (Brink et al., 2017), but the growing deployment of communication and information technologies in medicine has been underway for several decades (Smith et al., 1998; Heath et al., 2003; Pilnick et al., 2010). Dicker and Jim (2018) propose that technological change will be seen in the transformation of three domains of medical institutions: changes in work, structure, and patient expectations. Digital technology and AI-based devices are becoming increasingly relevant in interactions between medical professionals and patients, but in this paper, we focus on what could be labeled “changes in work.” These include novel workplace practices and communication environments related to the introduction of digital technologies and AI (Aznar et al., 2018), where it is important to specify possible problematic issues with how these technologies are “integrating into existing workflow” (Mun et al., 2021; p. 1). A recent review of the use of ML algorithms in oncology underscores the importance of trust, track records, and the accountability of AI in medical practice, stating that “trust in this technology has to be built one step at a time, based on its capability to make useful and correct predictions” (Nardini, 2020; p. 6). Although AI-powered technology can have a positive impact, e.g., in reducing cancer care inequalities (Arora et al., 2020) or in enhancing the translation between clinical and laboratory work (Yim et al., 2016), there are also threats, for instance in terms of cybersecurity (Joyce et al., 2021; see also Franzoi et al., 2023 for an overview).
The distinctive worksite practices of oncologists and radiologists, often involving work with digital technologies, have also been examined through EM/CA, which is the approach taken in this paper. Following Goodwin's (1994) study of “professional vision” (see also Goodwin, 2018a), Gegenfurtner et al. (2019) explore how experts communicate with novices to allow them to make sense of visualizations of medical data. Their analysis of interactions between an expert in radiology and four laypeople enabled them to identify three recurrent practices—highlighting, zooming, and rotating—as the basis for radiologists' embodied competence for detecting symptoms of pneumothorax. Working from a similar basis, Rystedt et al. (2010) found that professionals in radiology make use of various layered displays of the anatomy, while using “gestures, mouse cursors and individual laser pointers” for “a rapid and precise indexing of anatomical structures and their locations.” Ivarsson (2017) further argues that gestures and embodied actions are important means for organizing expertise in “the enacted production of radiological reasoning” (p. 135). In addition to bodily conduct, analysis of radiological practices has also focused on instructions and their relationship to the recognition and avoidance of errors, while underscoring the shared quality of the expert “perception” (Lymer et al., 2014). Rystedt et al. (2011) point out that expertise involves “discovery work in which visual renderings are made transparent,” enabling radiologists to discern meaningful objects in the images in an “inherently practical and domain-specific” manner (p. 868). Given that “every community is systematically faced with the task of building skilled, knowing members” (Goodwin, 2018b), the domain-specific ways of seeing are also central in training activities. Exploring educational sessions in diagnostic radiology, Ivarsson et al. (2016) argue that in addition to technological novelty, advancement can also be “achieved by a novel set-up of existing technologies and an interactive format that allows for focused discussions between learners with different levels of expertise” (p. 416). Nevertheless, compared to more traditional and established ways of working in oncology or radiology, the introduction of AI-based technologies also creates a requirement for novel ways of “seeing” on the users' part.
To the best of our knowledge, so far, no EM/CA research has addressed the use of AI in oncology, or radiomics as such, though this approach can effectively respond to the calls for rigorous user studies in the emerging domain. Existing literature highlights the fact that one of the main challenges of adopting radiomics in clinical practice is the limited interpretability of the resulting radiomic models, leading to physicians' low confidence in the diagnosis and treatment planning proposed by the model (Liu et al., 2019). While the past few years have seen important improvements in radiomics, not much is known yet about physicians' and researchers' actual conduct while creating, assessing, and interpreting radiomic models (Verma et al., 2023). Detailed insight into experts' practical work can provide important background information for the standardization of data collection procedures, as well as the establishment of evaluation criteria and reporting guidelines (Lambin et al., 2017; Jin et al., 2023). Antoniadi et al. (2021) conclude that there is a lack of user studies “exploring the needs of clinicians.” These must be conducted while keeping in mind the specific position of radiomics in the overall workflows and pipelines of diagnostics and patient care (Chang et al., 2019), while also setting up appropriate expectations for the use of an AI-based technology (Kocielnik et al., 2019). Explainability has been identified as one of the major challenges in the incorporation of AI into medicine and health care in general, and radiomics more specifically (Kundu, 2021; Jin et al., 2022; Chaddad et al., 2023). Calisto et al. (2021, 2022) have conducted a mixed-method study on an AI-powered “assistant” in radiomics that was introduced to translate its findings into clinical practice, finding relatively high levels of trust, acceptance, and satisfaction among the forty-five practitioners involved, as well as higher accuracy levels. Although the reviewed studies point to future possibilities of incorporating radiomics into clinical practice, it is still the case that “radiomic technologies require a systematic evaluation of their properties and effects as decision-making tools in healthcare” (Miles, 2021, p. 929).
This means that understanding the experts' actual practical work with AI-based technologies is a key element for making radiomics respectable in the field and overcoming the resistance to their adoption in clinical practice. A precursor in a different medical domain could be seen in Hartland's (1993) interview-based study on the use of “intelligent machines” for electrocardiograph interpretation, which underscores the important point that considering the use of autonomous technology for the screening of data and the identification of “abnormal” patterns overlooks the fact that “in practice ‘normal' is an achieved rather than a given characteristic” (p. 62, original emphasis). This achievement of “normality” and “routine” operation of an AI system can be illuminated by looking at people interacting with a novel and unfamiliar technology. Our article contributes to the outlined areas of research by examining specific workplace practices previously uninvestigated by EM/CA or interactionist studies, connecting them with the proposal that “AI-facilitated health care requires education of clinicians” (Keane and Topol, 2021). Before we report and discuss our findings from the user study in trial sessions with QuantImage, a brief technical introduction to the platform is needed, alongside a description of the setting and our research methods.
3 Setting and data
The QuantImage platform (Abler et al., 2023) is currently used in clinical research to evaluate the relevance of radiomics by users that do not need any coding knowledge. It covers all the main processes of radiomics and underwent several user studies, conducted to improve the platform as an integral part of the medical field.1 One core component of QuantImage is the “Feature Explorer,” which includes ergonomic workflows to evaluate the relevance of specific feature groups called “collections” in achieving the research tasks at hand. It allows clinical researchers to formulate and test multiple hypotheses in terms of how imaging observations can be used to predict the considered outcomes. The Feature Explorer includes semi-automatic tools that are used to add or remove specific features while simultaneously visualizing their relation to the outcome in a heatmap containing all feature values from all observations (e.g., patients) shown with a standardized color scale. ML models can be built and validated for custom feature collections created by the user in a specific tab called “Model Training.” A screenshot of the Feature Explorer is depicted in Figure 1. The heatmap displays the links between radiomics feature values and outcomes in the “Visualization” tab. The content of the heatmap is dynamic and corresponds to manual and semi-automatic feature selections, which can be saved into “feature collections” to formulate and test specific hypotheses.
Our research reported in this paper broadens the scope and use of the platform by exploring the potential of QuantImage as an educational tool. The potential educational dimensions of this software are related to designing specific curricular activities that would focus on achieving familiarity with radiomics practice through the platform's use and interface, as well as consideration of its limitations. Moreover, it might be that some elements of the platform need to be made more transparent and explainable. To explore these possibilities, in the setting studied in this article, pairs of users (physicians and medical radiology technicians) worked together during collaborative review sessions, focusing on a task aimed at identifying features in the data that help to build a well-performing model through ML algorithms implemented in the platform. Once they had selected the data features that they wanted to include in a collection, they then trained a new model and evaluated its performance in terms of sensitivity and specificity as estimated by the platform for the given model and validation strategy (i.e., repeated stratified train/test splits).
The participants were affiliated with the same hospital (CHUV) and signed an informed consent form before taking part in the session. This document stated that the aim of the project was to improve the platform—including its design, usability, and technical performance—and to design teaching guidelines regarding use of the platform in higher education. They were also informed that the collected data would be used solely for research purposes and that their personal information would be removed from the published outcomes (including possible publication of images with blurred/pixelized faces). In total, we organized 13 sessions with 27 participants, each lasting one hour. For most of the participants (and for all users represented in this paper), it was their first opportunity to work with the platform and in radiomics in general. Their work on the task was solely for the purpose of exploration and was not part of their obligatory study assignments or job duties. A tutor was present to support the participants in achieving the task by providing information about the specific functionalities of QuantImage or general radiomics processes.
The task that the pairs of participants were working on was based on developing a diagnostic model of Pulmonary Lymphangitic Carcinomatosis (PLC), a condition linked to very poor prognosis in the context of lung cancer. Diagnosing PLC is a difficult task for radiologists and usually requires an invasive and risky lung biopsy (Jreige et al., 2020). A collection of over 100 cases representing both positive and negative PLC was assembled at CHUV. Using QuantImage, radiomics features were extracted from PET/CT images. The main task was to use QuantImage's Feature Explorer to identify the feature categories (e.g., intensity, texture, shape) that are most predictive of PLC. Following specific hypotheses concerning which type of features can predict PLC, the user can test them to encourage an interpretable and trustworthy radiomics model. Other datasets, such as the HECKTOR challenge, are available and could be considered variants of this task (Oreiller et al., 2022).
We video-recorded the sessions from two complementary angles, setting up our cameras in opposite corners of the room to capture all potentially relevant details of the setting and activities (see, e.g., Knoblauch et al., 2006; Heath et al., 2010; Broth et al., 2014). We then depersonalized, transcribed, and analyzed the resulting recordings (15 h of video) in detail in accordance with the principles of EM/CA (Jefferson, 2004; Mondada, 2018; see Appendix). Video analysis begins with noticing systematically recurrent interactional phenomena during “unmotivated looking” (Sacks, 1984; Psathas, 1995). This analysis takes into account speech, embodied action (gaze, posture, gestures, touch, movement in space, etc.), as well as the materiality of the setting (see Goodwin, 2018a; Reber and Gerhardt, 2019; Mondada, 2021). The major advantage of direct observational methods such as video analysis is that they allow for discovery of detailed embodied practices that are normally taken for granted by the participants, not reflected upon or talked about, and therefore not easily accessible by standard survey methods (questionnaires or interviews) that rely on recollection (e.g., Verma et al., 2023). Comprehensive knowledge of the studied setting is an important prerequisite for an adequate analysis of video-recordings. Therefore, in addition to the video data, we also collected ethnographic material from the sessions, including documents, textual and visual artifacts, and observational field notes, in order to retain aspects of the setting that were relevant for subsequent analysis of the video-recordings (Grosjean and Matte, 2021).
4 Analysis
In our analysis, we aimed to unpack and explicate the practical work of doing radiomics, including how the platform is made sense of and embedded in the situated activity of the trial sessions. As part of collaborative work, QuantImage is a representative instance of radiomics, and learning about the platform is tied to learning about radiomics more broadly. Although mutually and reflexively constitutive, as we will show, these two dimensions are distinguished by the members and oriented to separately.
While working together with QuantImage, making sense of the platform's operation over the course of that work, the participants came across practical troubles that were intertwined with grasping radiomics as a domain of medical and research practice. Our analysis is structured around the features of the platform, designed and executed as steps in the radiomics procedure, that were encountered as problematic by the participants, ranging from feature selection to model creation and evaluation. In some instances, an absence of fundamental background knowledge—e.g., about performance measures (specificity and sensitivity)—even made it impossible for the participants to proceed with the task on their own, since performance measures are central to evaluating the models. In this section, we focus on three excerpts in which members orient to a lack of practical knowledge that is not fundamental, while still contributing valuable insights about the sensemaking practices related to radiomics during their work with the platform. These excerpts offer illustrative instances of recurrently observed issues that the novice users of QuantImage encountered as part of their collaborative work on the task in the trial sessions.
4.1 Troubles with data visualization
In the first excerpt, we are joining the dyad (Teresa and Hanna2) about 10 min after they begin their independent work on the task. Stephen, the tutor, has spent the previous 20 min of the session introducing radiomics more generally, as well as the platform and the participants' task (see Section 3 above), following which he has provided some further explanations and clarifications. At the beginning of the sequence transcribed below, Stephen is sitting behind Teresa and Hanna and attending to his own laptop screen (see Figure 2; a similar configuration was established in all sessions). The very beginning of Excerpt 1 makes visible the exploratory nature of their work on the task. Hanna and Teresa formulate their activity as “trying” (lines 1 and 4)—they are discovering radiomics and the platform at the same time. Indeed, the setting is framed and organized as a “trial”: the point is to learn something new and try out the platform, and their work (and its possible imperfections) does not have any serious consequences beyond these goals. In selecting the data features that are to be computed by the platform to produce the most efficient model, Teresa and Hanna encounter a certain problem (line 12). Shortly thereafter, in line 19, Teresa raises a more general issue, which also invites Stephen as a recipient. Stephen's involvement in the dyad's work seems to be aimed at overcoming an issue that often leads AI-based digital devices to be described as “black boxes”: they “generate actions—and hence have agency—but knowledge of how they arrive at their outcomes remains hidden” (Huggett, 2021; p. 424). The extract ends with Stephen walking to the large screen; thereafter, following line 29, he goes on to provide an extended explanation of the visualization heatmap (not reproduced in the transcript).
In lines 1–19 of the excerpt, Teresa and Hanna produce their talk in a way that makes it both monitorable by others who are present in the room, and still hearably designed for each other as members of the independently working dyad. One relevant aspect of the talk's production is its volume—there is an amplitude shift (Goldberg, 1978) after the 0.4 s pause in line 19, and the word “mais” (“but” in English) is produced louder than the previous relatively quiet talk. This might be an indication that Teresa's talk is now addressed to a different recipient who is farther away from the speaker. And it seems to be taken as such by Stephen, who turns his head at this precise moment in the direction of the large screen and Teresa with Hanna. This shift does not come unexpectedly, as the exchange between Teresa and Hanna in lines 12–19 builds up to a moment when it is predictable that a recruitment of assistance through a shift in recipiency might occur. The succession of Teresa's audible inhale in line 11, a hesitation marker in line 13 coming after a relatively long pause, and finally her “I can't do it like this” in line 15, already makes relevant the possibility that their work with the platform is encountering trouble. In her next turn (line 16), Hanna aligns with Teresa. In lines 17–18, this alignment is followed by brief shared laughter, which routinely occurs in trouble-related interactional environments (Jefferson, 1984) and may indicate the presence of trouble (Petitjean and González-Martínez, 2015). Stephen's attentive embodied response (turning his head in line 19) is therefore occasioned both by prosodic aspects of the immediately preceding talk and by the recent local history of the monitored interaction of the dyad and its possible recognizability as problematic with regard to working on the given task.
Although the sequential organization and prosodic details of the interaction contribute to the production of a recognizable trouble as something that requires the involvement of the tutor, the encountered problem is also recognizably specific to their activity as an activity in radiomics. The specificity of the occasion is an outcome of its accomplishment as simultaneously a learning situation and a radiomics task. The members' method for recruiting Stephen's assistance thus also provides an opportunity to explore how they make sense of radiomics in the midst of their encounter with a novel technology.
The crux of the problem as formulated by Teresa relates to the “logic” (line 21) behind the very procedure that they are supposed to undertake, i.e., creating a model based on data with “a lot of red” (line 24). Teresa's reference to the color makes relevant the “heatmap” data visualization that is a central component of the Feature Explorer in QuantImage (see above in Section 3; Figure 3 shows the screen at the particular moment). The source of the trouble seems to come from a conventional interpretation of the red color as something “wrong” or “dangerous” (see Gnambs et al., 2015), and novice users might be misled by this cultural convention rather than seeing the colors in ways that are common in the field (e.g., in genomics). In fact, the use of red and blue in the heatmap indicates how the individual features predict a positive or negative outcome after standardization of the features in the statistical sense. The presence of blue and red can therefore be seen as a “good” result, since the features are predictive of the two classes. What seems unclear to the participants at this point is the dynamic relationship between the selection of the features and the visual information contained in the heatmap. The fact that the heatmap is not fully understood as an aspect of QuantImage also means that radiomics processes could be grasped better. This is also what Stephen clarifies afterwards, in an extended explanation sequence that begins after line 32. As formulated by Lymer et al. (2014), eventually, “[t]he reasoned processing of the problematical is ... directed toward re-establishing a new, shared, re-instructed perception” (p. 212), in this case a perception of the heatmap within the Feature Explorer and the colors that are used in it. The excerpt illustrates how members learn radiomics in their collaborative use of a software platform accompanied by running explanations from the tutor, and how common-sense knowledge (e.g., about meanings of colors) could interfere with the established practices (e.g., visual representation) in the professional domain.
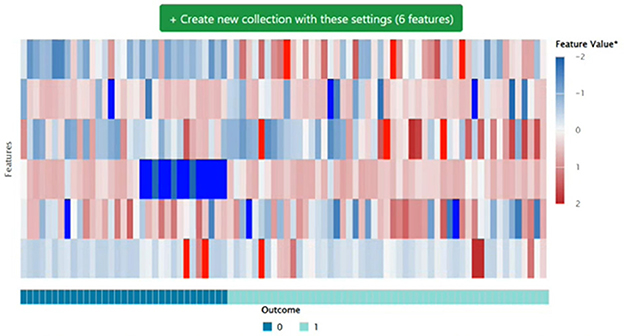
Figure 3. Screenshot of Feature Explorer at line 12 of Excerpt 1.
4.2 Troubles with feature selection
In Excerpt 2, Paul and Carl are about 10 min into their work on the collaborative task. After training the first model on all available features, they had gone back to selecting a subsection of these features to identify those that significantly contributed to proper diagnosis. During this time, Stephen was monitoring and occasionally explaining various aspects of the platform and the reasoning behind it. Excerpt 2 starts as Paul clicks on “train and test” to let the platform develop the next model (line 20).
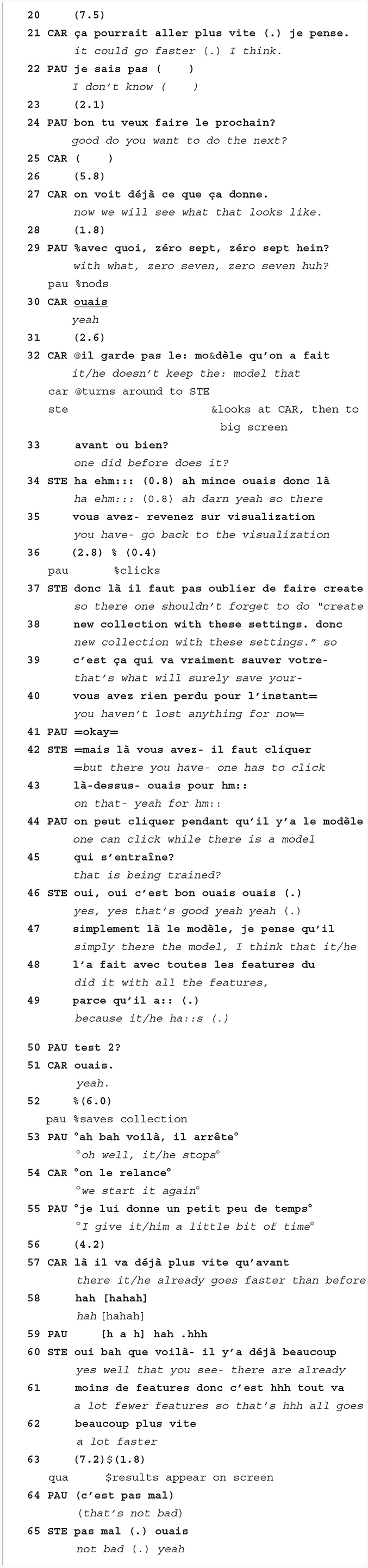
Excerpt 2. “It could go faster” (session of January 26, 2023).3
Lines 20 to 36 take place as the platform is training and testing the new model. In line 21, Carl complains that the platform is taking more time than expected, already noting a potential problem. After a brief and barely audible exchange about the division of work (lines 24–25), they recall the performance measures of the previous model, in order to be able to compare the new model with the previous one. Here, we can note “the practical reasoning involved in ordinary comparisons and contrasts as these are expressed and incarnate in ... conversational actions” (Watson, 2008, p. 211, our emphasis). Paul's “zero seven zero seven” (line 29) relates to the values of specificity (0.7) and sensitivity (0.7) of the model that they have created earlier. The participants thus display their locally established expertise in radiomics by orienting to one of its central aspects, i.e., the performance measures, as a key factor in the assessment of the best model. Thereafter, in line 32, Carl addresses Stephen with a clarification request regarding QuantImage's ability to keep the previous “model.” Although it is not clear whether the question is about the previously selected features or the performance measures of the model trained with these features, which would refer to two distinct steps in the radiomics workflow and correspondingly also to two different sections of the platform, Stephen does not make the ambiguity of the question relevant in his response.
Rather than responding to the content of the question directly, Stephen's response in lines 34–35 treats it as a sign of trouble and he offers instructions on how to solve the problem and proceed correctly. He notes that an important step in the procedure has been omitted—specifically, creating a “new collection” (line 38). This means that the platform uses the same set of features to train the model as it did before—perhaps even all the features, as that is the default setting—rather than a limited set of features that the participants have selected for inclusion in the training and testing of the new model. Creating a new collection is not only a step in working with QuantImage, but also a specific phase in the workflow of radiomics: by creating a collection with particular features, the users also formulate a hypothesis in terms of radiomics (see Section 3 above). Creating a collection with a set of features is equivalent to stating that these features, which model particular aspects of medical imaging, will be useful for making the diagnostic decision, and in the next phase of training the model the hypothesis is then tested. Stephen identifies and explains the problem of not creating a new collection in line 48 (“it/he did it with all the features”), whereafter the participants fix it by creating a new collection with the name “test 2” that is to be correctly used as the basis for training and testing the model.4 In following Stephen's instructions in a skillfully familiar and locally competent way, Carl and Paul exhibit that “the intelligible, determinate, and consequential character of any ‘instruction'... is only to be found and spelled out in its enactment, as its ‘action”' (Sormani and Wolter, 2023, p. 274).
The problem encountered in this excerpt is related to the noticeably “longer” time taken by the platform, which the users orient to as a visible index of a trouble in the expected progression of the work. In particular, the long training time of the ML model indicates that too many features are used, and feature reduction is needed, via the creation of feature collections. The notion of “features,” already prominent in our analysis of Excerpt 1, is relevant not only with regard to the visual aspects of the heatmap in the Feature Explorer, but in a different sense also in the evaluation of the temporality of the platform's operation. Here, the relationship between features and model training that makes the problem visible for the participants seems to be: the more features that are selected to be included in the model, the longer it takes for the model to be calculated during the “training and testing” phase. The machinic temporality of the platform is an integral part of doing radiomics in this setting, and when the temporal features of the platform's work are not aligned with a competent expectation, it indicates that something is “wrong” with the platform itself or with its settings on the users' side.
Therefore, in lines 57–62, the fact that the processing speed has improved is also taken as confirmation that the second attempt has been successful and the platform is now properly creating a new model including only the lower number of selected features. This shows how the interaction around the software platform is temporally aligned with the real-time operation of the platform. In general, machines routinely take a certain “response time” to accomplish their jobs, and people interacting with and around these machines routinely adjust their activities to the temporal requirements of the machine's operation. Yet, as Pelikan and Hofstetter (2023) point out, “from an interactional perspective, delays are not neutral wait time, but … delays do actions in interaction—participants make sense of the delay as doing or meaning something” (p. 4, original emphases). In Excerpt 2, the response time—the projectable time that the machine will take as shown by the progress indicator on the screen—is first taken as a possible sign of trouble (when moving “slow”), and later as a demonstration of the trouble being solved (when moving “faster”). The computer's operation, visibly displayed on the screen, establishes the local temporal structuring of the environment in which the participants work together. The members' activity is tied to the temporal order of the platform's own operation, whose indicators are displayed on the screen and thus also made publicly available for monitoring. In this sense, what is happening on the screen, as a representation of what the machine is doing, becomes an important structuring element of the unfolding situation at every moment. By being able to see the speed of the platform's operation as indicating trouble—or the trouble's disappearance—, the participants display their competence in using QuantImage in routine ways. And once the results are finally shown, in line 63, they also display their radiomics competence in their ability to evaluate the new model's performance measures “at a glance” (Sudnow, 1972, p. 259), in alignment with the tutor (lines 64–65). All these competences are displayed as a matter of course, as unremarkable and taken for granted constituents of the present social setting as specifically radiomics' work.
4.3 Beyond “troubles”: explaining algorithmic agency
Excerpt 3 shows Tom and Yann shortly after they have started their work on the task. The spatial arrangement is similar to the previous two excerpts: Tom and Yann work together as a pair while Stephen sits behind them and tacitly monitors their work. As the excerpt begins, they are using “Feature Explorer” to select 70 features (line 3), save their collection (lines 6–19), and move forward to “Model Training” in line 20. The participants do not encounter trouble in the sense of Excerpts 1 and 2, but they nevertheless recruit Stephen's involvement. He then explains an aspect of radiomics that goes beyond what they need to know to complete the task.
In the first part of the excerpt, Yann repeatedly seeks Stephen's confirmation about the immediate next steps to be taken with the platform: first in line 7, when Yann asks about how to name the new collection while saving it. Afterwards, Tom comments on the three algorithms shown on the screen in the “Model Training” tab: logistic regression, support-vector machines, and random forests.5 With some smiling and laughter, Tom and Yann agree that they do not know any of these algorithms. Nevertheless, when Yann turns to Stephen again in line 24, he doesn't orient to this particular aspect of their knowledge as being insufficient or problematic for their work on the task at hand. In his request for confirmation, Yann instead orients to the procedural organization of their work with the software and the action that needs to be taken next, i.e., clicking the icon ‘train and test' (line 25). After a micro-pause, in line 28, Stephen confirms this proposal and Yann clicks on the icon, which launches the training and testing process in the platform. Thereafter, starting in line 30, Stephen goes on to produce an extended explanation that relates to the more substantive aspects of the participants' work—i.e., these aspects are not related to the procedural organization of their work with the platform, but provide more in-depth insights about radiomics and its logic. In the radiomics workflow, after selecting the features, a user would choose a machine-learning algorithm without knowing in advance which one would provide the best results. To be able to decide which algorithm will yield the best results, the user must test several of them. In the QuantImage platform (as Stephen explains in lines 48–49), this procedure is automated, and the platform selects the most efficient algorithm automatically,6 testing all the possibilities and keeping the best one. Detailed technical knowledge of the three algorithms is not required from the users as a prerequisite, and Tom and Yann orient to this aspect adequately. Tom's questions about the algorithms can be seen more as a sign of competent curiosity and interest going beyond what is needed at this point of the task, rather than signaling or formulating a “trouble.”
Stephen's shift from a practical explanation of the platform's work to an explanation that goes beyond what is needed for the particular task at hand (taking place in line 43) is timed in a way that documents how the sequential structure of interaction is intertwined with the temporality of the platform. As Tom clicks on the icon, the platform starts working on the training and testing of the model—a procedure that is finished only in line 50. We have noted in the analysis of Excerpt 2 that members reflexively adjust to the temporal structuring of the situation as it unfolds, including the machinic temporality of QuantImage. After someone clicks on “train and test,” the platform creates a temporal slot during which the users need to wait—but as our analysis shows, they use this opportunity to do more than just waiting. The time that is available is embedded in the collaborative work as a moment for reflection upon the task underway, and an opportunity to bring up relevant topics or ask additional questions that are not directly connected to the current or following steps. As Stephen produces his turn at talk, the progress indicator on the large screen allows him to estimate how much time he has available before the results of the “train and test” process appear on the screen. Indeed, the temporal slot is also available to the other participants—e.g., in lines 37–38 of Excerpt 3, Tom formulates the gist of Stephen's previous explanation by saying “ha therefore it/he takes any of these three algorithms” (which, as we note below, is then corrected by Stephen). Next, between lines 43 and 50, while the platform is still taking time to finish the model training, Stephen provides a more detailed explanation of QuantImage and the difference it makes compared to the established ways of working in radiomics. Concurrently, Stephen's explanation also accounts for the relatively long time (usually between 15 and 25 s) that the platform takes to accomplish this step, as he makes it clear that the platform is just doing many things on its own.
The algorithmic agency of the platform is deeply embedded in the interactional sequence. Several utterances in lines 30–49 ascribe various kinds of actions to QuantImage. They are, however, not equivalent—although both Tom and Stephen offer accounts of the platform's operation, their category memberships as expert and novice become relevant (see Jacoby and Gonzales, 1991). Stephen—as the tutor and the expert—offers corrections to Tom's candidate explanation, most significantly to Tom's “it/he takes any of these three algorithms” (line 37), which is corrected by Stephen to “it/he will see which one is working best” (line 39). This is offered as a reformulation of “it/he will explore all the models” (line 30) and “it/he will select the best one (.) and then it/he will evaluate it in the test” (lines 34–36), which were offered by Stephen previously. Anthropomorphically described actions of the computer, such as “seeing,” “selecting,” “taking,” and “evaluating,” are presented as interconnected in an ordered stepwise procedure, which is as a whole attributed to the platform as an independent agent—“the model does it automatically” (lines 48–49). Nevertheless, although agency is ascribed to the machine, it does not autonomously produce interactionally relevant actions. The relevance of visible software elements and information provided on screen is worked out by the participants step by step as they move through the procedure of radiomics. Thus, perhaps more markedly than in interactions with tangible devices or anthropomorphic AI-based technologies, “the status of an interactional agent may be highly transient, meaning that its variable forms of agency are accomplished on a moment-by-moment basis” (Pelikan et al., 2022). The excerpt shows that the accountability of algorithmic agency is closely related to the practical explainability of what the platform is doing at each moment in the collaborative work, and as an inherent part of it.
5 Concluding discussion
This article offered insights into novice users' work, in an educationally framed setting, with a radiomics software platform, QuantImage, that uses machine-learning algorithms to extract large-scale quantitative features from medical imaging. Responding to the lack of user studies in radiomics and contributing to EM/CA studies of worksite practices in medicine, the setting provided an opportunity to explore interaction around a tacitly present and situationally constitutive AI-based technology. As Hindmarsh et al. (2007) remind us, “the failure of new technologies often can be attributed to difficulties for users in making technologies ‘at home' in their very practical worlds of work” (p. 5). It is therefore important to understand how radiomics procedures, embodied in the specific platform, are made sense of, in situ and in real time in the collaborative work. Our approach enabled us to observe the novice users' learning and sensemaking procedures with respect to all steps in the radiomics process, and to underscore how their practical actions and reasoning furnish the setting with features that are specifically constitutive of work in radiomics. The analysis of video-recorded materials collected in semi-experimental trial sessions involving pairs of novice users demonstrated that the users learn novel ways of seeing, e.g., colors in the heatmap (Section 4.1). Their ability to use the platform in a competent way is displayed by their orienting to the temporal aspects of its operation as a sign of trouble, as well as their routine assessment of performance measures (Section 4.2). The participants also confirm the proper ways of moving through the stepwise procedure of the task with the tutor, and they make use of opportunities to discuss aspects of radiomics that are not necessarily related to their successful achievement of the task (Section 4.3). In sum, our study contributes to observational user studies of the use of digital technology and AI in oncology practice, examining in detail the sensemaking practices that participants employ while working with unfamiliar software. It shows just how, in concrete interactional detail, troubles might be “located” in the current task, in the platform, and in the broader professional domain.
The analysis indicates that the integration of AI-based technology into medical and educational praxis requires careful consideration of different levels of users' previous understandings of radiomics at various stages of the emerging expertise. This is in line with Hua et al.'s (2023) conclusions based on a scoping literature review of acceptability of AI among healthcare professionals in medical imaging—the authors point out that it is important to design “human-centered AI systems which go beyond high algorithmic performance to consider accessibility to users with varying degrees of AI literacy” (Hua et al., 2023). In the setting examined in this article, the absence of fundamental background knowledge (e.g., of statistical performance measures) can make it impossible for the participants to work efficiently with the platform and complete the task on their own. Conversely, limited knowledge of certain substantive issues (e.g., of specific algorithms in the platform) might not necessarily be detrimental to the successful achievement of the tasks. Furthermore, the analysis indicates that different aspects of the users' competence and knowledge become relevant at different stages of the work, indicating the importance of paying very close attention to the real-time sequential development of actual situations involving interactions with and around AI-powered technologies.
Our empirical observations can also be connected to the explainability, agency, and accountability of AI, issues that are central to human–AI collaboration. These questions are currently hotly debated, as various forms of AI become entrenched in professional and everyday activities. The ability to understand and predict what an AI-based algorithm is doing has important consequences for users' trust in the computational models (Vivacqua et al., 2020). In the setting under investigation in this paper, social interaction happens around the AI rather than directly with it. Autonomous agency is routinely ascribed by the members to the machine involved in the situation even though it features as a passive object rather than an active agent. It is not communicating on its own, in real time or in direct response to humans' turns at talk—not even in a “simulacrum of conversation” (Button and Sharrock, 1995). Explanations functioning as specific social actions are also given among people in the presence of the software platform, and on its behalf (see also Albert et al., 2023). The explanations that are offered by the tutor, or requested by the participants, allow us to identify elements of the platform that may need to be made more transparent. Information objects displayed on the computer screen are turned into events relevant to radiomics in and through the sensemaking work of the participants. In other words, most—if not all—of the “co-operative action” (Goodwin, 2018a) is done by humans rather than the AI. Nevertheless, this is not necessarily to be seen as a limitation. Our study contributes insights into how automated agents that were intentionally not designed to be human-like or anthropomorphic are incorporated into medical work. Understanding how people learn to accommodate the tacit, non-tangible forms of AI and their specific algorithmic agency is crucial before more elaborate and consequential machines (such as robots or chatbots) can be introduced into established medical practices. As proposed by von Eschenbach (2021), one pathway toward “trust in AI” is to “acknowledge that AI is situated within a socio-technical system that mediates trust, and by increasing the trustworthiness of these systems, we thereby increase trust in AI” (p. 1609). As demonstrated in this paper, careful analysis of the “practical trust” that constitutes the very basis of social interaction (González-Martínez and Mlynář, 2019) can aid in identifying and explicating the constitutive elements of the “socio-technical systems” in which understanding, explaining, and transparency are already naturally embedded and situated as members' matters.
Much interaction in medical settings involves communication among actors with different levels of professional expertise and types of competences. We have explored aspects of worksite interaction in which patients have appeared only tangentially; however, large areas of investigation open up as soon as AI-based technologies, or their outputs, move into interdisciplinary contexts and interactions with patients. Research has demonstrated that patients often raise “concerns about fears, uncertainties, and hopes” (Beach et al., 2005, p. 1243; Beach and Dozier, 2015). Singh et al. (2017) have shown that oncologists typically dedicate most of their time with patients to discussions of treatment options, which is also related to shared decision-making (Alby et al., 2015; Tate and Rimel, 2020). In this context, research has been done on how oncologists make recommendations for different cancer treatments (Fatigante et al., 2020), report “bad” and “uncertain” news (Lutfey and Maynard, 1998; Alby et al., 2017), and balance asserting their authority with ensuring that patients are involved in decision-making (Tate, 2019). Divergences among doctors' and patients' understandings can occur, but ambiguities are routinely managed as part of the interaction (Pino et al., 2022; Ross and Stubbe, 2022). How AI-based technology could be adequately incorporated into these complex processes remains an open question, but our study indicates what it means to take AI-based technologies into account in a serious way as part of professional practice. Continued exploration of the organization and reasoning of interdisciplinary communication that takes place, for instance, in multidisciplinary tumor boards (Seuren et al., 2019; cf. Smart and Auburn, 2019; Mano et al., 2021), and the possible incorporation of radiomics results and procedures into interactions with patients, could yield further insights relevant to existing contributions coming from research in doctor–patient interaction, as well as studies of workplace practices in oncology and radiology. Future studies should also explore whether and how AI-based technologies might, to some extent, become participants in complex medical processes involving diagnostic data analysis and decision-making, while keeping human involvement and expertise center stage.
Data availability statement
The datasets presented in this article are not readily available because the video recordings are available only for internal research purposes at the HES-SO Valais-Wallis. Requests to access the datasets should be directed to amFrdWIubWx5bmFyJiN4MDAwNDA7aGV2cy5jaA==.
Ethics statement
Ethical approval was not required for the studies involving humans because the study was conducted as an explorative user study of the software platform prototype under development. The studies were conducted in accordance with the local legislation and institutional requirements. The participants provided their written informed consent to participate in this study. Written informed consent was obtained from the individual(s) for the publication of any potentially identifiable images or data included in this article.
Author contributions
JM, AD, FE, and JP contributed to conception and design of the study. JP, AD, and JM organized the trial sessions. JM, AD, and AM participated in the data collection. RS programmed the software platform. JM conducted the data analysis and wrote the first draft of the manuscript. All authors contributed to the article and approved the submitted version.
Funding
This research was funded by the HES-SO internal fund RCSO-ISNET-119387 Radiomics in higher education: QuantImage as a teaching tool (RADHED) and partially funded by the Swiss National Science Foundation (SNSF) with projects 205320_219430 and 205320_179069, the Swiss Cancer Research foundation with project TARGET (KFS-5549-02-2022-R), the Lundin Center for Neuro-oncology at CHUV, and the Hasler Foundation with the MSxplain project number 21042.
Acknowledgments
We gratefully acknowledge the funding listed above and we thank all participants for their willingness to take part in the trial sessions with QuantImage v2.
Conflict of interest
The authors declare that the research was conducted in the absence of any commercial or financial relationships that could be construed as a potential conflict of interest.
Publisher's note
All claims expressed in this article are solely those of the authors and do not necessarily represent those of their affiliated organizations, or those of the publisher, the editors and the reviewers. Any product that may be evaluated in this article, or claim that may be made by its manufacturer, is not guaranteed or endorsed by the publisher.
Footnotes
1. ^https://medgift.github.io/quantimage-v2-info/
2. ^All participant names used in this article, including the tutor's name, are pseudonyms.
3. ^The French pronouns “il” can be translated to English either as “it” or “he,” depending on what is being referred to. Similarly, “lui” can be translated either as “it” or “him.” We do not see it as our task to decide (on behalf of the participants) which one of these options is more appropriate for referring to an AI-based platform. Therefore, in all cases when the pronoun “il” (or “lui”) seems to refer to QuantImage, we use the translation “it/he” (or “it/him”) to mark the tension between grasping the platform as an object or an agent, which is in many ways characteristic of human–AI interactions (see, e.g., Alač, 2016; Rudaz et al., 2023).
4. ^The user studies reported in this article were quite informative for designing new versions of QuantImage. Observations of participants failing to create a new collection, as happened in Excerpt 2, were later used by the designers of QuantImage to improve it. A new version of the software includes a warning tab when the user moves to the next step (“model training”), and the unsaved state of the feature selection is kept by the platform so it can also be recovered when the user moves back to “visualization.” This way, the user is less likely to lose their set of selected features simply by forgetting to save them in a new collection.
5. ^These three algorithms are listed on the screen next to each other as “classification algorithms.” Underneath, two “data normalization” algorithms are listed—standardization and L2 normalization. Although they are not commented upon by Tom or Yann, Stephen mentions them later (lines 45–46).
6. ^In the earlier versions of QuantImage, users would be asked to select one of the algorithms, but the designers realized that this is too advanced for many users and instead decided to automate the procedure.
References
Abler, D., Schaer, R., Oreiller, V., Verma, H., Reichenbach, J., Aidonopoulos, O., et al. (2023). QuantImage v2: a comprehensive and integrated physician-centered cloud platform for radiomics and machine learning research. Eur. Radiol. Exp. 7, 16. doi: 10.1186/s41747-023-00326-z
Alač, M. (2016). Social robots: things or agents? AI Soc. 31, 519–535. doi: 10.1007/s00146-015-0631-6
Albert, S., Buschmeier, H., Cyra, K., Even, C., Hamann, M., Mlynář, J., et al. (2023). “What ‘counts' as explanation in social interaction? Six observations from an EM/CA approach,” in 2nd TRR 318 Conference Measuring Understanding. Paderborn: Paderborn University, Germany. Available online at: https://saulalbert.net/blog/what-counts-as-explanation-in-social-interaction/
Alby, F., Zucchermaglio, C., and Baruzzo, M. (2015). Diagnostic decision making in oncology: Creating shared knowledge and managing complexity. Mind, Culture Activ. 22, 4–22. doi: 10.1080/10749039.2014.981642
Alby, F., Zucchermaglio, C., and Fatigante, M. (2017). Communicating uncertain news in cancer consultations. J. Cancer Educ. 32, 858–864. doi: 10.1007/s13187-016-1070-x
Antoniadi, A. M., Du, Y., Guendouz, Y., Wei, L., Mazo, C., Becker, B. A., et al. (2021). Current challenges and future opportunities for XAI in machine learning-based clinical decision support systems: a systematic review. Appl. Sci. 11, 5088. doi: 10.3390/app11115088
Arora, S., Ryals, C., Rodriguez, J. A., Byers, E., and Clewett, E. (2020). Leveraging digital technology to reduce cancer care inequities. Am. Soc. Clini. Oncol. Educ. Book 42, 559–566. doi: 10.1200/EDBK_350151
Aznar, M. C., Warren, S., Hoogeman, M., and Josipovic, M. (2018). The impact of technology on the changing practice of lung SBRT. Physica Medica 47, 129–138. doi: 10.1016/j.ejmp.2017.12.020
Barnes, R. K. (2019). Conversation analysis of communication in medical care: Description and beyond. Res. Lang. Soc. Interact. 52, 300–315. doi: 10.1080/08351813.2019.1631056
Beach, W. A., and Dozier, D. M. (2015). Fears, uncertainties, and hopes: patient-initiated actions and doctors' responses during oncology interviews. J. Health Commun. 20, 1243–1254. doi: 10.1080/10810730.2015.1018644
Beach, W. A., Easter, D. W., Good, J. S., and Pigeron, E. (2005). Disclosing and responding to cancer ‘fears' during oncology interviews. Soc. Sci. Med. 60, 893–910. doi: 10.1016/j.socscimed.2004.06.031
Brink, J. A., Arenson, R. L., Grist, T. M., Lewin, J. S., and Enzmann, D. (2017). Bits and bytes: the future of radiology lies in informatics and information technology. Eur. Radiol. 27, 3647–3651. doi: 10.1007/s00330-016-4688-5
Brooker, P., Dutton, W., and Mair, M. (2019). The new ghosts in the machine: ‘Pragmatist' AI and the conceptual perils of anthropomorphic description. Ethnog, Stud. 16, 272–298.
Broth, M., Laurier, E., and Mondada, L., (eds.). (2014). Studies of Video Practices: Video at Work. Abingdon/New York: Routledge. doi: 10.4324/9781315851709
Button, G., and Sharrock, W. (1995). “On simulacrums of conversation: Toward a clarification of the relevance of conversation analysis for human–computer interaction,” in The Social and Interactional Dimensions of Human–Computer Interfaces, ed P. J. Thomas. New York: Cambridge University Press, 107–125.
Calisto, F. M., Santiago, C., Nunes, N., and Nascimento, J. C. (2021). Introduction of human-centric AI assistant to aid radiologists for multimodal breast image classification. Int. J. Human–Comp. Stud. 150, 102607. doi: 10.1016/j.ijhcs.2021.102607
Calisto, F. M., Santiago, C., Nunes, N., and Nascimento, J. C. (2022). Breast screening-AI: evaluating medical intelligent agents for human-AI interactions. Artif. Intell. Med. 127, 102285. doi: 10.1016/j.artmed.2022.102285
Chaddad, A., Peng, J., Xu, J., and Bouridane, A. (2023). Survey of explainable AI techniques in healthcare. Sensors 23, 634. doi: 10.3390/s23020634
Chang, Y., Lafata, K., Sun, W., Wang, C., Chang, Z., Kirkpatrick, J. P., et al. (2019). An investigation of machine learning methods in delta-radiomics feature analysis. PLoS ONE 14, e0226348. doi: 10.1371/journal.pone.0226348
Crabtree, A.ndy. (2004). Taking technomethodology seriously: hybrid change in the ethnomethodology-design relationship. Eur. J. Informat, Syst, 13, 195–209. doi: 10.1057/palgrave.ejis.3000500
Dicker, A. P., and Jim, H. (2018). Intersection of digital health and oncology. JCO Clinical Cancer Informatics 2:1–4. doi: 10.1200/CCI.18.00070
Dourish, P., and Button, G. (1998). On technomethodology: foundational relationships between ethnomethodology and system design. Human–Comp. Interact. 13, 395–432. doi: 10.1207/s15327051hci1304_2
Fatigante, M., Heritage, J., Alby, F., and Zucchermaglio, C. (2020). Presenting treatment options in breast cancer consultations: advice and consent in Italian medical care. Soc. Sci. Med. 266, 113175. doi: 10.1016/j.socscimed.2020.113175
Franzoi, M. A., Gillanders, E., and Vaz-Luis, I. (2023). Unlocking digitally enabled research in oncology: the time is now. Esmo Open Sci. Optimal Canc. Care 8, 101633. doi: 10.1016/j.esmoop.2023.101633
Garfinkel, H. (2002). Ethnomethodology's Program: Working Out Durkheim's Aphorism. Lanham: Rowman & Littlefield.
Gegenfurtner, A., Lehtinen, E., Helle, L., Nivala, M., Svedström, E., and Sälj,ö, R. (2019). Learning to see like an expert: on the practices of professional vision and visual expertise. Int. J. Educ. Res. 98, 280–291. doi: 10.1016/j.ijer.2019.09.003
Gillies, R. J., Kinahan, P. E., and Hricak, H. (2016). Radiomics: Images are more than pictures, they are data. Radiology 278, 563–577. doi: 10.1148/radiol.2015151169
Gnambs, T., Appel, M., and Oeberst, A. (2015). Red color and risk-taking behavior in online environments. PLoS ONE 10, e0134033. doi: 10.1371/journal.pone.0134033
Goldberg, J. A. (1978). “Amplitude shift: a mechanism for the affiliation of utterances in conversational interaction,” in Studies in the Organization of Conversational Interaction, ed. J. Schenkein. New York: Academic Press, 199–218.
González-Martínez, E., and Mlynář, J. (2019). Practical trust. Soc. Sci. Inform. 58, 608–630. doi: 10.1177/0539018419890565
Goodwin, C. (1994). Professional vision. Am. Anthropol. 96, 606–633. doi: 10.1525/aa.1994.96.3.02a00100
Goodwin, C. (2018b). Why multimodality? Why co-operative action? Soc. Interact. 1, 2. doi: 10.7146/si.v1i2.110039
Grosjean, S., and Matte, F., (eds.). (2021). Organizational Video-Ethnography Revisited: Making Visible Material, Embodied and Sensory Practices. London: Palgrave Macmillan.
Guiot, J., Vaidyanathan, A., Deprez, L., Zerka, F., Danthine, D., Frix, A. N., et al. (2022). A review in radiomics: Making personalized medicine a reality via routine imaging. Med. Res. Rev. 42, 426–440. doi: 10.1002/med.21846
Hartland, J. (1993). “The use of intelligent machines for electrocardiograph interpretation,” in Technology in Working Order: Studies of Work, Interaction, and Technology, ed G. Button. London: Routledge, 55–80.
Heath, C., Hindmarsh, J., and Luff, P. (2010). Video in Qualitative Research: Analysing Social Interaction in Everyday Life. London: Sage Publications.
Heath, C., and Luff, P. (2022). “Technology in practice,” in The Ethnomethodology Program: Legacies and Prospects, eds. D. Maynard, and J. Heritage. New York: Oxford University Press, 398–419.
Heath, C., Luff, P., and Svensson, M. S. (2003). Technology and medical practice. Soc. Health Illn. 25, 75–97. doi: 10.1111/1467-9566.00341
Hindmarsh, J., Neil Jenkings, K., and Rapley, T. (2007). Introduction to healthcare technologies in practice. Health Informat. J. 13, 5–8. doi: 10.1177/1460458207073642
Hua, D., Petrina, N., Young, N., Cho, J. G., and Poon, S. K. (2023). Understanding the factors influencing acceptability of AI in medical imaging domains among healthcare professionals: A scoping review. Artif. Intellig. Med. 68, 29–36. doi: 10.1016/j.artmed.2023.102698
Huggett, J. (2021). Algorithmic agency and autonomy in archaeological practice. Open Archaeology 7, 417–434. doi: 10.1515/opar-2020-0136
Ivarsson, J. (2017). Visual expertise as embodied practice. Front. Learn. Res. 5, 123–138. doi: 10.14786/flr.v5i3.253
Ivarsson, J., Rystedt, H., Asplund, S., Åse Allansdotter Johnsson, S., and Båth, M. (2016). The application of improved, structured and interactive group learning methods in diagnostic radiology. Radiat. Protect. Dosimet. 169, 416–421. doi: 10.1093/rpd/ncv497
Jacoby, S., and Gonzales, P. (1991). The constitution of expert/novice in scientific discourse. Issues Appl. Lingu. 2, 149–182. doi: 10.5070/L422005141
Jefferson, G. (1984). “On the organization of laughter in talk about troubles,” in Structures of Social Action: Studies in Conversation Analysis, eds. J. Maxwell Atkinson, and J. Heritage, 346–369. Cambridge: Cambridge University Press.
Jefferson, G. (2004). “Glossary of transcript symbols with an introduction,” in Conversation Analysis: Studies from the First Generation, ed G. Lerner. Amsterdam/Philadelphia: John Benjamins, 13–31.
Jin, W., Li, X., Fatehi, M., and Hamarneh, G. (2023). Guidelines and evaluation of clinical explainable AI in medical image analysis. Med. Image Anal. 84, 102684. doi: 10.1016/j.media.2022.102684
Jin, W., Li, X., and Hamarneh, G. (2022). Evaluating explainable AI on a multi-modal medical imaging task: Can existing algorithms fulfill clinical requirements? Proc, AAAI Conf. Artif. Intell. 36, 11945–11953. doi: 10.1609/aaai.v36i11.21452
Joyce, C., Roman, F. L., Miller, B., Jeffries, J., and Miller, R. C. (2021). Emerging cybersecurity threats in radiation oncology. Adv. Radiat. Oncol. 6, 100796. doi: 10.1016/j.adro.2021.100796
Jreige, M., Dunet, V., Letovanec, I., Prior, J. O., Meuli, R. A., Beigelman-Aubry, C., et al. (2020). Pulmonary lymphangitic carcinomatosis: diagnostic performance of high-resolution CT and 18 F-FDG PET/CT in correlation with clinical pathologic outcome. J. Nucl. Med. 61, 26–32. doi: 10.2967/jnumed.119.229575
Keane, P. A., and Topol, E. J. (2021). AI-facilitated health care requires education of clinicians. Lancet 397, P1254. doi: 10.1016/S0140-6736(21)00722-4
Keel, S., (ed.). (2023). Medical and Healthcare Interactions: Members' Competence and Socialization. London: Routledge.
Knoblauch, H., Schnettler, B., Raav, J., and Soeffner, H. G., (eds.). (2006). Video Analysis: Methodology and Methods. Frankfurt am Main: Peter Lang.
Kocielnik, R., Amershi, S., and Bennett, P. N. (2019). “Will you accept an imperfect AI? Exploring designs for adjusting end-user expectations of AI systems,” in Proceedings of the 2019 CHI Conference on Human Factors in Computing Systems (CHI '19), Paper 411. New York: ACM, 1–14.
Koivisto, A., Vepsäläinen, H., and Virtanen, M. T., (eds.). (2023). Conversation Analytic Perspectives to Digital Interaction: Practices, Resources, and Affordances. Helsinki: Finnish Literature Society. doi: 10.21435/sflin.22
Kundu, S. (2021). AI in medicine must be explainable. Nat. Med. 27, 1328. doi: 10.1038/s41591-021-01461-z
Lambin, P., Leijenaar, R., Deist, T. M., Peerlings, J., de Jong, E. C., van Timmeren, J., et al. (2017). Radiomics: the bridge between medical imaging and personalized medicine. Nature Rev. Clini. Oncol. 14, 749–762. doi: 10.1038/nrclinonc.2017.141
Lindwall, O., and Lymer, G. (2014). Inquiries of the body: novice questions and the instructable observability of endodontic scenes. Discourse Stud. 16, 271–294. doi: 10.1177/1461445613514672
Liu, Z., Wang, S., Dong, D., Wei, J., Fang, C., Zhou, X., et al. (2019). The applications of radiomics in precision diagnosis and treatment of oncology: opportunities and challenges. Theranostics 9, 1303–1322. doi: 10.7150/thno.30309
Luchini, C., Lawlor, R. T., Milella, M., and Scarpa, A. (2020). Molecular tumor boards in clinical practice. Trends in Cancer 6, 738–744. doi: 10.1016/j.trecan.2020.05.008
Lutfey, K., and Maynard, D. W. (1998). Bad news in oncology: How physician and patient talk about death and dying without using those words. Soc. Psychol. Q. 61, 321–341. doi: 10.2307/2787033
Lymer, G., Ivarsson, J., Rystedt, H., Johnsson, A., Asplund, S., and Båth, M. (2014). Situated abstraction: From the particular to the general in second-order diagnostic work. Discour. Stud. 16, 185–215. doi: 10.1177/1461445613514674
Lynch, M. (1993). Scientific Practice and Ordinary Action: Ethnomethodology and Social Studies of Science. New York: Cambridge University Press.
Mano, M. S., Çitaku, F. T., and Barach, P. (2021). Implementing multidisciplinary tumor boards in oncology: a narrative review. Future Oncol. 18, 375–384. doi: 10.2217/fon-2021-0471
Miles, K. (2021). Radiomics for personalised medicine: the long road ahead. Br. J. Cancer 122, 929–930. doi: 10.1038/s41416-019-0699-8
Mlynář, J. (2021). ‘Getting on the page': The practical accord of material resources in educational interaction. Ethnographic Stud. 18:145–172.
Mlynář, J., Kocián, J., and Hofmeisterová, K. (2022a). “How ‘tools' produce ‘data': Searching in a large digital corpus of audiovisual Holocaust testimonies,” in Jewish Studies in the Digital Age, eds. Zaagsma, G., Stökl Ben Ezra, D., Rürup, M., Margolis, M., and Levi, A. S. Berlin: De Gruyter Oldenbourg, 65–88.
Mlynář, J., Liesenfeld, A., de Rijk, L., Stommel, W., Topinková, R., and Albert, S. (2022b). “AI in interaction: EM/CA studies of artificial intelligence,” in Digital Meeting for Conversation Analysis (DMCA), October 31 – November 4, 2022, online.
Mondada, L. (2018). Multiple temporalities of language and body in interaction: challenges for transcribing multimodality. Res. Lang. Soc. Interact. 51, 85–106. doi: 10.1080/08351813.2018.1413878
Mondada, L. (2021). Sensing in Social Interaction: The Taste for Cheese in Gourmet Shops. Cambridge: Cambridge University Press.
Mun, S. K., Wong, K. H., Lo, S. C., Li, Y., and Bayarsaikhan, S. (2021). Artificial intelligence for the future radiology diagnostic service. Front. Mol. Biosci. 7, 614258. doi: 10.3389/fmolb.2020.614258
Nardini, C. (2020). Machine learning in oncology: a review. Ecancermedicalscience 30, 1065. doi: 10.3332/ecancer.2020.1065
Oreiller, V., Andrearczyk, V., Jreige, M., Boughdad, S., Elhalawani, H., Castelli, J., et al. (2022). Head and neck tumor segmentation in PET/CT: The HECKTOR challenge. Med. Image Anal. 77, 102336. doi: 10.1016/j.media.2021.102336
Peeken, J. C., Bernhofer, M., Wiestler, B., Goldberg, T., Cremers, D., Rost, B., et al. (2018). Radiomics in radiooncology – challenging the medical physicist. Physica Medica 48, 27–36. doi: 10.1016/j.ejmp.2018.03.012
Pelikan, H., Broth, M., and Keevallik, L. (2022). When a robot comes to life: The interactional achievement of agency as a transient phenomenon. Soc. Interact. 5, 3. doi: 10.7146/si.v5i3.129915
Pelikan, H., and Hofstetter, E. (2023). Managing delays in human–robot interaction. ACM Trans. Comp.–Human Interact. 30, 50. doi: 10.1145/3569890
Petitjean, C., and González-Martínez, E. (2015). Laughing and smiling to manage trouble in French-language classroom interaction. Classroom Discourse 6, 89–106. doi: 10.1080/19463014.2015.1010556
Pilnick, A., Hindmarsh, J., and Teas Gill, V., (eds.). (2010). Communication in Healthcare Settings: Policy, Participation and New Technologies. Chichester: Wiley-Blackwell.
Pino, M., Fatigante, M., Alby, F., and Zucchermaglio, C. (2022). Two sources of miscommunication in oncology consultations: an observational study using conversation analysis. Appl. Linguist. 43, 249–270. doi: 10.1093/applin/amab036
Randall, D., Rouncefield, M., and Tolmie, P. (2021). Ethnography, CSCW and ethnomethodology. Comp.-Support. Cooper. Work 30, 189–214. doi: 10.1007/s10606-020-09388-8
Reber, E., and Gerhardt, C., (eds.). (2019). Embodied Activities in Face-to-Face and Mediated Settings: Social Encounters in Time and Space. London: Palgrave MacMillan.
Ross, I., and Stubbe, M. (2022). Self-repeats-as-unit-ends: A practice for promoting interactivity during surgeons' decision-related informings. Res. Lang. Soc. Interact. 55, 241–259. doi: 10.1080/08351813.2022.2075641
Rudaz, D., Tatarian, K., Stower, R., and Licoppe, C. (2023). From inanimate object to agent: Impact of pre-beginnings on the emergence of greetings with a robot. ACM Trans. Human–Robot Interact. 12, 29. doi: 10.1145/3575806
Rystedt, H., Ivarsson, J., Asplund, S., Johnsson, A., and Båth, M. (2011). Rediscovering radiology: New technologies and remedial action at the worksite. Soc. Stud. Sci. 41 (6):867–891. doi: 10.1177/0306312711423433
Rystedt, H., Ivarsson, J., and Mäkitalo, A. (2010). “Making professional vision visible: displaying and articulating diagnostic work in medical imaging,” in EARLI SIG 14 Meeting on Diversity in Vocational and Professional Education and Training, München, Germany.
Sacks, H. (1984). “Notes on methodology,” in Structures of Social Action: Studies in Conversation Analysis, eds. J. Maxwell Atkinson and John Heritage. Cambridge: Cambridge University Press, 2–27.
Schegloff, E. A. (2007). Sequence Organization in Interaction: A Primer in Conversation Analysis. Cambridge: Cambridge University Press.
Seuren, L. M., Stommel, W., van Asselt, D., Sir, O., Stommel, M., and Schoon, Y. (2019). Multidisciplinary meetings at the emergency department: A conversation-analytic study of decision-making. Soc. Sci. Med. 242:112589. doi: 10.1016/j.socscimed.2019.112589
Singh, S., Cortez, D., Maynard, D., Cleary, J., DuBenske, L., and Campbell, T. (2017). Characterizing the nature of scan results discussions: insights into why patients misunderstand their prognosis. Journal of Oncology Practice 13, 231–239.
Smart, C., and Auburn, T. (2019). “Theorising multidisciplinary team meetings in mental health clinical practice,” in Interprofessional Care and Mental Health: The Language of Mental Health, eds. C. Smart and T. Auburn. London: Palgrave Macmillan, 79–95.
Smith, C. L., Chu, W.-K., and Enke, C. (1998). A review of digital image networking technologies for radiation oncology treatment planning. Med. Dosimetry 23, 271–277. doi: 10.1016/S0958-3947(98)00026-0
Sormani, P., and Wolter, L. (2023). “Protocol subversion: Staging and stalking ‘Machine Intelligence' at School,” in Instructed and Instructive Actions: The Situated Production, Reproduction, and Subversion of Social Order, eds. M. Lynch and O. Lindwall. London: Routledge, 259–278.
Suchman, L. (1987). Plans and Situated Actions: The Problem of Human-Machine Communication. Cambridge: Cambridge University Press.
Suchman, L. (2007). Human–Machine Reconfigurations: Plans and Situated Actions. Cambridge: Cambridge University Press.
Sudnow, D. (1972). “Temporal parameters of interpersonal observation,” in Studies in Social Interaction, ed. D. Sudnow. Washington: Free Press, 259–279.
Tate, A. (2019). Treatment recommendations in oncology visits: implications for patient agency and physician authority. Health Commun. 34, 1597–1607. doi: 10.1080/10410236.2018.1514683
Tate, A., and Rimel, B. (2020). The duality of option-listing in cancer care. Patient Educ. Couns. 103, 71–76. doi: 10.1016/j.pec.2019.07.025
Verma, H., Mlynář, J., Pellaton, C., Theler, M., Widmer, A., and Evéquoz, F. (2021). “WhatsApp in Politics?!': Collaborative Tools Shifting Boundaries,” in Human-Computer Interaction – INTERACT (2021), eds. C. Ardito et al. Cham: Springer.
Verma, H., Mlynář, J., Schaer, R., Reichenbach, J., Jreige, M., Prior, J., et al. (2023). “Rethinking the role of AI with physicians in oncology: Revealing perspectives from clinical and research workflows,” in Proceedings of the 2023 CHI Conference on Human Factors in Computing Systems (CHI '23), Article 17. New York: ACM.
Vivacqua, A. S., Stelling, R., Garcia, R. A., and Gouvea, L. (2020). “Explanations and sensemaking with AI and HCI,” in Proceedings of the IX Latin American Conference on Human Computer Interaction (CLIHC '19), Article 41. New York: ACM.
von Eschenbach, W. J. (2021). Transparency and the black box problem: Why we do not trust AI. Philos. Technol. 34:1607–1622. doi: 10.1007/s13347-021-00477-0
Watson, R. (2008). Comparative sociology, laic and analytic: Some critical remarks on comparison in conversation analysis. Cahiers de praxématique 50, 197–238. doi: 10.4000/praxematique.967
Yim, W. W., Meliha, Y., Harris, W. P., and Kwan, S. W. (2016). Natural language processing in oncology: a review. JAMA Oncology 2, 797–804. doi: 10.1001/jamaoncol.2016.0213
Appendix
Keywords: artificial intelligence, conversation analysis, ethnomethodology, human–computer interaction, oncology, radiology, radiomics, social interaction
Citation: Mlynář J, Depeursinge A, Prior JO, Schaer R, Martroye de Joly A and Evéquoz F (2024) Making sense of radiomics: insights on human–AI collaboration in medical interaction from an observational user study. Front. Commun. 8:1234987. doi: 10.3389/fcomm.2023.1234987
Received: 05 June 2023; Accepted: 04 December 2023;
Published: 12 February 2024.
Edited by:
Stephanie Fox, Montreal University, CanadaReviewed by:
Jonas Ivarsson, University of Gothenburg, SwedenWill Gibson, University College London, United Kingdom
Copyright © 2024 Mlynář, Depeursinge, Prior, Schaer, Martroye de Joly and Evéquoz. This is an open-access article distributed under the terms of the Creative Commons Attribution License (CC BY). The use, distribution or reproduction in other forums is permitted, provided the original author(s) and the copyright owner(s) are credited and that the original publication in this journal is cited, in accordance with accepted academic practice. No use, distribution or reproduction is permitted which does not comply with these terms.
*Correspondence: Jakub Mlynář, amFrdWIubWx5bmFyJiN4MDAwNDA7aGVzLXNvLmNo