- Department of Language and Linguistic Science, University of York, York, United Kingdom
Introduction: In England and Wales, transcripts of police-suspect interviews are often admitted as evidence in courts of law. Orthographic transcription is a time-consuming process and is usually carried out by untrained transcribers, resulting in records that contain summaries of large sections of the interview and paraphrased speech. The omission or inaccurate representation of important speech content could have serious consequences in a court of law. It is therefore clear that investigation into better solutions for police-interview transcription is required. This paper explores the possibility of incorporating automatic speech recognition (ASR) methods into the transcription process, with the goal of producing verbatim transcripts without sacrificing police time and money. We consider the potential viability of automatic transcripts as a “first” draft that would be manually corrected by police transcribers. The study additionally investigates the effects of audio quality, regional accent, and the ASR system used, as well as the types and magnitude of errors produced and their implications in the context of police-suspect interview transcripts.
Methods: Speech data was extracted from two forensically-relevant corpora, with speakers of two accents of British English: Standard Southern British English and West Yorkshire English (a non-standard regional variety). Both a high quality and degraded version of each file was transcribed using three commercially available ASR systems: Amazon, Google, and Rev.
Results: System performance varied depending on the ASR system and the audio quality, and while regional accent was not found to significantly predict word error rate, the distribution of errors varied substantially across the accents, with more potentially damaging errors produced for speakers of West Yorkshire English.
Discussion: The low word error rates and easily identifiable errors produced by Amazon suggest that the incorporation of ASR into the transcription of police-suspect interviews could be viable, though more work is required to investigate the effects of other contextual factors, such as multiple speakers and different types of background noise.
1. Introduction
Orthographic transcripts of spoken language can be admitted as evidence in courts of law in England and Wales in a number of scenarios. When the speech content of an audio or video recording is used as evidence, e.g., a threatening voicemail message, the recording is often accompanied by a transcript to assist the court in “making out what was said and who said it” (Fraser, 2020). These recordings tend to be of very poor quality such that the speech is often close to unintelligible without the aid of a transcript. However, this means that the transcript can be highly influential on what members of the court believe they hear in the recording, as highlighted by Fraser and Kinoshita (2021; see also Fraser et al., 2011). It is therefore crucial that transcripts presented alongside speech evidence are as accurate as possible since they can play an important role in listeners' perception of speech and speakers, potentially leading to miscarriages of justice in cases where an utterance is inaccurately interpreted as incriminating (Harrison and Wormald, in press).
Another use of orthographic transcripts in the legal system is transcripts of police-suspect interviews, which play an important role in the investigative process and are often admitted as evidence in court (Haworth, 2018). While the audio recording of the police-suspect interview is technically the “real” evidence in this context, the transcript is admissible as a “copy” and is often the only version of the police-suspect interview that is referred to in the courtroom (Haworth, 2018). Given that the court often does not hear the original audio recording, it is important that the transcripts are an accurate representation of the interview's contents. However, Haworth (2018, 2020) has identified issues with the transcripts created by police transcribers, including summarizing large sections of the interview, paraphrasing the speech content and inconsistent representation across transcribers. A verbatim record of the speech would be ideal, but this is a time-consuming and laborious task.
Automatic speech recognition (ASR) technology is rapidly improving and can produce transcripts in a fraction of the time it would take a human to complete the same task. Transcripts produced by an ASR system would require manual checking and correction, but the output would be a verbatim record of the full interview, eliminating the issue of potentially important information being inaccurately paraphrased or omitted. A computer-assisted transcription method could lead to more reliable evidence being presented to courts without a significant increase in the time spent producing the records.
When considering the incorporation of ASR into the transcription process, it is important to take into account factors that have a significant impact on ASR performance, such as audio quality and regional accents. Background noise has been shown to decrease the accuracy of ASR systems in a number of contexts (Lippmann, 1997; Littlefield and Hashemi-Sakhtsari, 2002) including for forensic-like audio recordings (Harrington et al., 2022; Loakes, 2022). In recent years, a growing body of research has focused on systematic bias within automatic systems, i.e., underperformance for certain demographic groups, and significant disparities in performance have been demonstrated across accents. Transcripts tend to be significantly less accurate for non-native speakers (DiChristofano et al., 2022) or speakers of non-standard regional varieties (Markl, 2022). However, a limitation of work in this area is the use of word error rate (WER) for evaluating performance. WER is the ratio of errors in a transcript to the total number of words spoken and can be useful to highlight differences in performance across groups. However, this metric does not provide insights into where and why systems produce errors, or how evidentially significant those errors could be.
This paper presents work on the topic of automatic speech recognition in the context of police-suspect interview transcription, employing a novel method of analysis that combines industry-standard measures alongside detailed phonetic and phonological analysis. While WER is useful for an overview of performance, incorporating fine-grained linguistic analysis into the method permits a deeper understanding of the aspects of speech that prove to be problematic for automatic systems. The performance of three commercial ASR systems is assessed with two regional accents, across different audio qualities; the purpose of this assessment is to evaluate how practical it would be for ASR systems to play a role in the transcription of police-suspect interviews.
2. Background
This section covers a range of topics relevant to the present study. Firstly, Section 2.1 outlines the current situation regarding police-suspect interview transcription in England and Wales, and highlights the issues. Automatic speech recognition (ASR) is offered as part of a potential solution, and Section 2.2 covers a brief history of ASR and its rapid improvement in recent years. Section 2.3 describes research on the use of ASR for transcribing forensic audio recordings, which leads into the potential incorporation of ASR in the transcription of comparatively better quality audio recordings, i.e., police-suspect interviews, in Section 2.4. Section 2.5 addresses potential speaker-related factors that may affect ASR performance, such as regional accent. Finally, Section 2.6 outlines the research aims of the present study.
2.1. Transcription of police-suspect interviews in England and Wales
In England and Wales, police-suspect interviews are recorded according to requirements of the Police and Criminal Evidence Act 1984. The audio recording is subsequently used to produce a Record of Taped Interview (ROTI), and if the case ends up going to trial, the ROTI is often admitted as evidence alongside the original audio recording. However, the transcript itself often becomes effectively “interchangeable [with] and (in essence) identical” (Haworth, 2018, p. 434) to the audio evidence in the eyes of the court, and is often used as a substitute for the original audio recording. Relying on the transcript as the primary source of the interview's contents could be problematic in cases where speech has been omitted or inaccurately represented.
The police interview transcribers, also known as ROTI clerks, tend to be employed as administrative staff, and the job-specific skills required often include proficiency in audio and copy typing and a specific typing speed (Tompkinson et al., 2022). ROTI clerks receive little to no training or guidance on the transcription process (Haworth, 2018), which has the potential to create a systematic lack of consistency in transcription production, even within police forces. This is highlighted by an example provided in Haworth (2018) in which three ROTI clerks transcribe an unanswered question in three unique ways: “no response,” “no audible reply” and “defendant remained silent.” Each representation could potentially generate varying interpretations of the interviewee's character. It is also worth noting that the 43 territorial police forces in England and Wales operate individually, which contributes to the issue of inconsistency in transcription and transcript production across forces.
Another issue with ROTIs is that much of the interview is summarized and the transcriber, untrained in legal issues and protocol, will ultimately decide what is deemed as important and worthy of full transcription. This decision-making process could lead to serious consequences given Section 34 of the Criminal Justice and Public Order Act 1994, which states that the court may draw inferences if something later relied upon as evidence is not mentioned during the initial interview stage.
In accordance with Haworth (2018), this assessment of problematic issues surrounding ROTIs does not serve as a critique of the clerks hired to produce the transcripts, but of the wider process. Transcription, particularly of long stretches of speech, is a time-consuming and labor-intensive task that can take four to five times the length of the audio recording to transcribe for research purposes (Walford, 2001; Punch and Oancea, 2014), and a time factor of 40 to 100 for difficult forensic recordings (Richard Rhodes, personal communication). It is also prone to human error, for example spelling and punctuation mistakes (Johnson et al., 2014) and omission or misrepresentation of short function words, discourse markers and filled pauses (Stolcke and Droppo, 2017; Zayats et al., 2019). Transcribing spoken language, even when producing a verbatim transcript, is a complex and inherently selective process which carries the inevitable risk of systematic and methodological bias (Jenks, 2013; Kowal and O'Connell, 2014). Transcripts carry social and linguistic information, therefore transcribers have an enormous amount of power over the way in which people are portrayed (Jenks, 2013).
Discrepancies concerning the portrayal of speakers have been reported within legal transcripts (e.g., US court reports, UK police interviews), with standardized language and “polished” grammar for professionals such as lawyers, expert witnesses and police interviewers but verbatim transcription or inconsistently-maintained dialect choices for lay witnesses or suspects (Walker, 1990; Coulthard, 2013). Similar inconsistencies were observed in ROTIs (Haworth, 2018), as well as an assumption revealed in focus group discussions with ROTI clerks that the interviewee will be charged with or convicted of an offense, as demonstrated through the use of terms such as “defendant” or “offender” to refer to interviewees (89% of references; Haworth, 2018, p. 440).
The use of ASR could address a number of the concerns regarding the production of police interview transcripts. Automatic systems can process a large amount of data in a fraction of the time it would take a human to do the same task. This could allow for interviews to be transcribed in full, rather than mostly summarized, while saving time, effort and money on behalf of the police. An automatic system would not apply social judgements to the role of interviewer and interviewee, and would therefore likely remain consistent in its treatment of speakers in this regard, given that only the speech content would be transcribed. Furthermore, an ASR system would likely be consistent in its representation of phenomena such as silences; for example, unanswered questions simply would not be transcribed, and therefore the system would not inject potentially subjective statements such as “defendant remained silent.”
2.2. Automatic speech recognition
The field of automatic speech recognition (ASR) has received growing interest over the last decade given its expanding applications and rapid improvements in performance, though this technology has existed in different forms for over 70 years. The first speech recogniser was developed in 1952 at Bell Telephone Laboratories (now Bell Labs) in the United States and was capable of recognizing 10 unique numerical digits. By the 1960's systems were able to recognize individual phonemes and words, and the introduction of linear predictive coding (LPC) in the 1970's led to rapid development of speaker-specific speech recognition for isolated words and small vocabulary tasks (Wang et al., 2019). The 1980's saw the creation of large databases (O'Shaughnessy, 2008) and the implementation of a statistical method called the “Hidden Markov Model” (HMM) which allowed systems to recognize several thousand words and led to substantial progress in the recognition of continuous speech (Wang et al., 2019). Combining HMM with a probabilistic Gaussian Mixture Model (HMM-GMM) created a framework that was thoroughly and extensively researched throughout the 1990's and 2000's, and remained the dominant framework until the last decade when deep learning techniques have become prevalent (Wang et al., 2019). In recent years deep neural networks (DNN) have been implemented to create the HMM-DNN model, achieving performance well beyond its predecessor.
Modern state-of-the-art ASR systems are typically made up of two main components, an acoustic model and a language model, both of which are concerned with calculating probabilities. As a basic summary according to Siniscalchi and Lee (2021), the acoustic model recognizes speech as a set of sub-word units (i.e., phonemes or syllables) or whole word units. It is then tasked with calculating the probability that the observed speech signal corresponds to a possible string of words. The language model then calculates the probability that this string of words would occur in natural speech. This is often evaluated using n-grams, which calculate the probability of the next word in a sequence given the n previous words, based on extensive training on large text corpora. Both models contribute to the estimated orthographic transcription produced by the ASR system.
Adaptations to the architecture of ASR systems have led to huge improvements in accuracy, which can be illustrated by observing the reported word error rates (WER) on a commonly-used dataset for measuring ASR performance, such as the Switchboard corpus (Godfrey and Holliman, 1993). This is a dataset of American English conversational telephone speech that is commonly used to benchmark ASR performance. The first reported assessment of speech recognition performance had a WER of around 78% (Gillick et al., 1993) and by 2005 state-of-the-art systems were yielding WER measures between 20 and 30% (Hain et al., 2005). Thanks to large amounts of training data and the application of machine learning algorithms, huge improvements in speech technology have been demonstrated in recent years. In 2016, Microsoft reported that their automatic system had achieved human parity, with a WER of 5.8% compared with a human error rate of 5.9% on a subset of the Switchboard data (Xiong et al., 2016). In 2021, IBM reported an even lower WER of 5.0% on a subset of the Switchboard data, reaching a new milestone for automatic speech recognition performance (Tüske et al., 2021).
It is crucial to acknowledge, though, that performance is relative to the materials being transcribed. Though trying to mimic spontaneous conversations, the Switchboard corpus contains “inherently artificial” (Szymański et al., 2020) spoken data due to factors such as the predefined list of topics, the localized vocabulary and the relatively non-spontaneous form of the conversations. These factors, paired with the relatively good audio quality, create conditions which are favorable to ASR systems, and while ASR may outperform human transcribers in some cases, there will be circumstances in which the reverse is true, especially in more challenging conditions such as forensic audio.
2.3. Automatic transcription of forensic audio recordings
Some work within the field of forensic transcription has considered whether automatic methods could be incorporated into the transcription of forensic audio samples, such as covert recordings. The audio quality of such recordings is generally poor given the real-world environments in which the recordings are made, and as a result of the equipment being deployed in a covert manner, rather than one designed to capture good-quality audio. They can also be very long, containing only a few sections of interest; it is often necessary to transcribe the recording in full to identify such sections, which is a time-consuming and arduous task for forensic practitioners.
Two studies in particular have explored automatic transcription in forensic-like contexts, the first of which uses an audio recording of a band rehearsal (Loakes, 2022), comparable to a covert recording. Two automatic transcription services (BAS Web Services and Descript) were employed to transcribe the 44 s recording containing the sounds of musical instruments and multiple speakers from a distance. BAS Web Services returned a system error when an orthographic transcription was requested, and when the in-built WebMINNI service was employed to segment the speech into phonemes, many sections of speech were identified as “non-human noise” and instrument noises were labeled as speech. Descript was also unsuccessful in its attempt to transcribe the speech, with the output containing only three distinct words (“yes,” “yeah,” and “okay”), a fraction of the total number of words uttered.
A second study on the topic of forensic transcription compared the performance of 12 commercial automatic transcription services using a 4-min telephone recording of a conversation between five people in a busy restaurant (Harrington et al., 2022). Talkers were positioned around a table upon which a mobile device was placed to record the audio, and all were aware of its presence. The transcripts produced by the automatic systems were of poor quality, making little sense and omitting large portions of speech, although this is not surprising given the high levels of background noise and numerous sections of overlapping speech.
A number of relatively clear single-speaker utterances were selected for further analysis, and results showed that even in cases of slightly better audio quality and more favorable speaking conditions, transcripts were far from accurate. The best performing system (Microsoft) produced transcripts in which 70% of words on average matched the ground truth transcript, though there was a high level of variability across utterances. Microsoft transcribed seven of the 19 utterances with over 85% accuracy, but many of the other transcriptions contained errors that could cause confusion over the meaning, or even mislead readers. For example, “that would have to be huge” was transcribed as “that was absolutely huge,” changing the tense from conditional (something that could happen) to past (something that has happened). In many cases, the automatic transcript would need substantial editing to achieve an accurate portrayal of the speech content.
The findings of such research, though valuable, are unsurprising given that commercial ASR systems are not designed to deal with poor quality audio; they are often trained on relatively good quality materials more representative of general commercial applications. Following recent advances in learning techniques to improve ASR performance under multimedia noise, Mošner et al. (2019) demonstrated that a system trained on clean and noisy data achieved better performance (i.e., higher reductions in WER) than a system trained only on clean data. It seems that training data has a direct effect on the capabilities of ASR systems. There could potentially be a place for automatic systems within the field of forensic transcription if the training data used is comparable to the audio recordings that would ultimately be transcribed. However, it is impractical to expect commercial ASR systems to perform at an appropriate level for the type of data that forensic practitioners handle.
Given the current state of the technology, ASR should therefore not be employed for the transcription of poor quality audio such as covert recordings, though the question remains as to whether it could be incorporated for comparatively better quality audio samples, such as police interviews. This type of audio recording is much better suited to automatic transcription for many reasons. The quality of police-suspect interview recordings tends to be much better since the equipment utilized is built specifically for the purposes of recording audio, and all members present are aware of the recording process. The number of speakers is limited and known, and the question-and-answer format of the interview will most likely result in speech that is easier to transcribe, i.e., less overlapping speech. The level of background noise will also likely be much lower than a busy restaurant or a music practice room, although it must be noted that the audio quality of these interviews is not always ideal or comparable to studio quality audio. Reverberation, broadband noise or interference, the rustling of papers and the whirring of laptop fans (Richard Rhodes, personal communication) are examples of frequently occurring issues encountered within police interview recordings which can make some sections difficult to transcribe.
2.4. Incorporating automatic methods into police transcription
One approach to the use of automatic methods would be the use of an automatically-produced transcript as a starting point to which human judgements could be added i.e., “post-editing” an ASR output. Bokhove and Downey (2018) suggest that using automatic transcription services to create a “first draft” could be worthwhile in an effort to reduce the time and costs involved in human transcription. In their study, many of the errors made by the ASR system for interview data were relatively small and easily rectifiable, while recordings of a classroom study and a public hearing (with many speakers and microphones positioned far away from speakers) resulted in automatic transcriptions that deviated more substantially from the audio content. Nonetheless, Bokhove and Downey (2018) argue that, with little effort, reasonable “first versions” can be obtained through the use of freely available web services, and that these may serve as a useful first draft in a process which would involve multiple “cycles” or “rounds” of transcription (Paulus et al., 2013) regardless of the inclusion of automatic methods.
However, the baseline performance of the ASR system is a key issue in whether combining ASR and human transcription is viable. By artificially manipulating the accuracy of transcripts, Gaur et al. (2016) demonstrated that the time spent correcting an ASR output can exceed the time spent creating a transcript from scratch if the automatically-produced transcript is insufficiently accurate. By manipulating the WER of transcripts at rough intervals of 5% ranging between 15 and 55%, it was found that by the time the WER had reached 30% participants were able to complete the post-editing phase more quickly by typing out the content from scratch. However, participants only realized that the quality of the original transcript was a challenge when the WER reached around 45%. These findings suggest that post-editing an ASR output could reduce the time taken to produce a verbatim transcript provided that the WER does not exceed a certain level; however, if the WER consistently approaches 30% then the incorporation of automatic methods into the transcription process fails to be a worthwhile avenue of research.
There are, however, some issues with using WER as the defining metric of system performance, as highlighted by Papadopoulou et al. (2021). Firstly, WER can be expensive and time-consuming to calculate due to the requirement of manual transcriptions to use as a reference. Secondly, quantified error metrics do not take into account the cognitive effort necessary to revise the ASR transcripts into a “publishable” quality. A more useful metric for analyzing ASR outputs is the post-editing effort required. In their study, a single post-editor with intermediate experience in the field was tasked with post-editing transcripts produced by four commercial ASR systems (Amazon, Microsoft, Trint, and Otter). Both the time taken to edit the ASR output and the character-based Levenshtein distance between the automatic and post-edited transcripts were measured.
An interesting finding by Papadopoulou et al. (2021) is that the number of errors within a transcript does not always correlate with the amount of effort required for post-editing. Systems with the lowest error rates do not necessarily achieve the best scores in terms of the post-editing time and distance. Certain types of errors were shown to take longer to edit, such as those related to fluency, i.e., filler words, punctuation and segmentation. The authors also suggest that deletion and insertion errors are easily detectable and require less cognitive effort to edit than substitution errors. Although little justification for this claim is put forth in the paper, it does seem likely that deletions and insertions could be easier to identify given that the number of syllables will not match up between the speech content and the transcript. The post-editor may find substitutions more challenging to detect, especially if the phonetic content of the target word and transcribed word is similar. It is therefore crucial to consider the types of errors made, not just overall error rates, when assessing the viability of an automatic transcript as a first draft.
The study carried out by Papadopoulou et al. (2021) claims to be one of the first papers to evaluate the post-editing effort required to transform ASR outputs into useable transcripts and to conduct qualitative analysis on ASR transcription errors. Given that WER does not reveal sufficient information regarding the types of errors made and the difficulty of correcting those errors, there is a clear need for additional research on the topic of post-editing and alternative methods of analysis. This is particularly true when evaluating the practicality of incorporating ASR into the transcription process, as the effort required to transform an ASR output into a fit-for-purpose verbatim transcript is the main consideration in whether this approach is advantageous, rather than the number of errors in the initial transcript.
2.5. Automatic systems and speaker factors
Given that the speakers taking part in police-suspect interviews will come from a range of demographics, it is important to consider how this may affect the performance of automatic speech recognition systems. Factors relating to a speaker's linguistic background, such as accent, can prove challenging for an automatic transcription system. Previous work has demonstrated that the performance of ASR systems declines significantly when confronted with speech that diverges from the “standard” variety; this has been found for non-native-accented speech in English (Meyer et al., 2020; DiChristofano et al., 2022; Markl, 2022) and Dutch (Feng et al., 2021), as well as for non-standard regionally-accented speech in Brazilian Portuguese (Lima et al., 2019) and British English (Markl, 2022).
Markl (2022) compared the performance of Google and Amazon transcription services across multiple accents of British English. One hundred and two teenagers from London or Cambridge (South of England), Liverpool, Bradford, Leeds, or Newcastle (North of England), Cardiff (Wales), Belfast (Northern Ireland), or Dublin (Republic of Ireland) were recorded reading a passage from a short story. Both systems demonstrated significantly worse performance, based on WER, for some of the non-standard regional accents compared with the more “standard” Southern English accents. Amazon performed best for speakers from Cambridge and showed a significant decline in performance for those from parts of Northern England (Newcastle, Bradford, and Liverpool) and Northern Ireland (Belfast). Much higher error rates were reported for Google for every variety of British English, likely as a result of much higher rates of deletion errors. Google performed best for speakers of London English and saw a significant drop in performance only for speakers from Belfast.
Many researchers have suggested that the composition of training datasets can cause bias within automatic systems (Tatman, 2017; Koenecke et al., 2020; Meyer et al., 2020; Feng et al., 2021) and that the underrepresentation of certain accents leads to a decline in performance for those varieties. Markl (2022) reports that certain substitution errors identified for speakers of non-standard regional accents of British English suggest that there is an overrepresentation of Southern accents in the training data or that acoustic models are being trained only on more prestigious Southern varieties, such as Received Pronunciation. Similarly, Wassink et al. (2022) claim that 20% of the errors within their data would be addressed by incorporating dialectal forms of ethnic varieties of American English (African American, ChicanX, and Native American) into the training of the automatic systems. The implementation of accent-dependent (or dialect-specific) acoustic models has been found to improve performance, particularly for varieties deviating more substantially from the standard variety, such as Indian English and African American Vernacular English (Vergyri et al., 2010; Dorn, 2019).
2.6. Research aims
The main aim of the present research is to assess ASR transcription errors across accents and audio qualities. The implications of such errors being retained in a transcript presented to the court will be considered, and methods of analysis that are appropriate for this particular context will be employed. This work is centered on the transcription of recordings resembling police interview data, and a further aim of this work is to consider the practicality of incorporating ASR into the transcription of police-suspect interviews.
The present study will explore in much greater detail the types of errors produced across two different accents of British English, and will focus not only on the distribution of three main error categories (deletions, substitutions, and insertions), but also on the distribution of word types that feature in the errors. For example, some substitutions may be more damaging than others, or more difficult to identify in the post-editing of a transcript. Errors will also be assessed from a phonological perspective in order to identify errors resulting from phonological differences across the accents and highlight particularly challenging phonetic variables for the automatic systems. Although both the acoustic and language model will affect ASR performance, the analysis and interpretation of errors in this study will focus on those which are most likely a reflection of the acoustic model.
In this study, recordings that are representative of police interviews in the UK (in terms of speech style and audio quality) are used, which are expected to degrade ASR performance compared with previous studies that have typically used high quality materials. The present study considers, from a practical perspective, whether this technology could be incorporated into the transcription process for police-suspect interviews.
The specific research questions are:
1. How do regional accent and audio quality affect the performance of different ASR systems?
2. What types of errors are produced by the ASR systems, and what are the implications of these errors?
3. To what extent could ASR systems produce a viable first draft for transcripts of police-suspect interviews?
3. Materials and methods
3.1. Stimuli
In order to explore differences in ASR performance across different regional accents, two varieties of British English were chosen for analysis: Standard Southern British English (SSBE) and West Yorkshire English (WYE). SSBE is a non-localized variety of British English spoken mostly in Southern parts of England, and although linguistic diversity is celebrated in contemporary Britain, SSBE is heard more frequently than other accents in public life (e.g., TV programmes and films), especially in media that is seen on an international scale, and acts as a teaching standard for British English (Lindsey, 2019). SSBE is referred to in this study as a “standard” variety. WYE is a non-standard regional variety of British English which shares characteristics with many other Northern English accents1 and whose phonology diverges substantially from SSBE (Hickey, 2015).
Stimuli were extracted from two forensically-relevant corpora of British English: the Dynamic Variability in Speech database (DyViS; Nolan et al., 2009) and the West Yorkshire Regional English Database (WYRED; Gold et al., 2018). DyViS contains the speech of 100 young adult males from the South of England (the majority of whom had studied at the University of Cambridge) taking part in a number of simulated forensic tasks, such as a telephone call with an “accomplice” and a mock police interview. WYRED contains the speech of 180 young adult males from three parts of West Yorkshire (Kirklees, Bradford, and Wakefield) and was created to address the lack of forensically-relevant population data for varieties of British English other than SSBE. The collection procedures employed in the production of the DyViS database were closely followed for WYRED, resulting in very closely matched simulated forensic conditions.
The mock police interview contained a map task in which specific speech sounds were elicited through the use of visual stimuli. Participants assumed the role of a suspected drug trafficker and had to answer a series of questions regarding their whereabouts at the time of the crime, their daily routine and their work colleagues, among other things. Visual prompts were provided during the task, containing information about the events in question and incriminating facts shown in red text. Participants were advised to be cooperative but to deny or avoid mentioning any incriminating information. The speech was conversational and semi-spontaneous, and the question-and-answer format of the task was designed to replicate a police-suspect interview. On account of the focus on police-suspect interview transcription in this paper, the mock police interview task was selected for this study.
Two speakers of each accent were selected and eight short utterances were extracted per speaker, resulting in a total of 32 utterances. Much of the speech content in this task contained proper nouns such as the surnames of colleagues and place names. With the exception of two well-known brands, “Skype” and “Doritos,” proper nouns were not included in the extracted utterances in order to avoid inflated error rates as a result of misspellings or due to the name not featuring in the ASR system's vocabulary. Other than filled pauses, which were extremely common in the spoken data, effort was also made to exclude disfluent sections. Disfluencies have been shown to be problematic for ASR systems (Zayats et al., 2019), therefore sections containing false starts or multiple repetitions were excluded in order to isolate differences in performance due to regional accent. Utterances ranged between 14 and 20 words in length and 3–6 s in duration, each containing a single speaker and unique linguistic content. Some examples of the utterances are included in Table 1 (and reference transcripts for all utterances can be found in Supplementary material).
To investigate the effects of low levels of background noise, such as that commonly found in real police interviews, the studio quality recordings were mixed with speech-shaped noise, derived from the HARVARD speech corpus. This was carried out using Praat (Boersma and Weenink, 2022), and the resulting files had an average signal-to-noise ratio (SNR) of 10 dB, such that intelligibility was not hugely impacted but the background noise was noticeable. The studio quality files had a much higher average SNR of 22 dB, reflecting the lack of background noise in these recordings. To summarize, a studio quality version and a poorer quality version (with added background noise) of each file was created, resulting in a total of 64 stimuli for automatic transcription.
3.2. Automatic transcription
Three commercially-available automatic transcription services were used to transcribe the audio files: Rev AI2, Amazon Transcribe3, and Google Cloud Speech-to-Text4. Many automatic transcription systems acknowledge that background noise and strongly accented speech can decrease transcription accuracy. Rev AI was chosen due to its claims of resilience against noisy audio and its Global English language model which is trained on “a multitude of… accents/dialects from all over the world” (Mishra, 2021). Services from Amazon and Google were chosen due to their frequent use in other studies involving ASR and the prevalent use of products from these technology companies in daily life. When uploading the files for automatic transcription, “British English” was selected as the language for Amazon and Google, and, since this option was not available for the third service, “Global English” was selected for Rev AI.
Reference (i.e., ground truth) transcripts were manually produced by the author for each utterance, using the studio quality recordings. The automatic transcripts produced by Amazon, Google, and Rev were compiled in a CSV file. Amazon and Google offer confidence levels for each word within the transcription but for the purpose of this research, only the final output (i.e., the highest probability word) was extracted.
3.3. Error analysis
Custom-built software was written to align the reference and automatic transcripts on a word-level basis, and each word pairing was assessed as a match or an error. Errors fall into three categories as outlined below:
• Deletion: the reference transcript contains a word but the automatic transcript does not.
• Insertion: the reference transcript does not contain a word but the automatic transcript does.
• Substitution: the words in the reference transcript and automatic transcript do not match.
From a forensic perspective, insertions, and substitutions are potentially more harmful than deletions, on the assumption that reduced information causes less damage than false information in case work (Tschäpe and Wagner, 2012). Table 2 shows an example of two potential transcriptions of the utterance “packet of gum in the car,” and demonstrates the different effect that substitutions can have in comparison with deletions. Both transcripts contain three errors, but the substitutions in transcript 2 could be much more damaging given the change in content and the new potentially incriminating interpretation of the utterance.
Some minor representational errors were observed, such as “steak house” transcribed as a compound noun “steakhouse” and numbers transcribed as digits. Since these substitutions do not constitute inaccuracies, rather slight changes in representation, the word pairing was marked as a match and these were not included as errors in the subsequent analysis. With regards to substitutions spanning multiple words, it was decided that the collective error would be marked as one substitution. For example, “cut and” transcribed as “cutting” was marked as a substitution rather than a combination of a substitution and a deletion, in an attempt to avoid inflated insertion and deletion rates.
Despite the limitations of WER, particularly in a forensic context, this metric can provide a brief overview of system performance across groups that can be used as a starting point for analysis. WER was therefore calculated for each utterance, by dividing the total number of errors (deletions, insertions, and substitutions) by the number of words in the reference transcript. The total number of each type of error in each condition was also calculated and compared to explore the differences across the ASR systems as well as the effects of regional accent and level of background noise. In order to explore in greater detail the types of words involved in errors, each error pairing was manually evaluated as involving content words, function words, filled pauses or a combination of these. Substitutions involving morphological alterations were also highlighted, and transcripts were assessed in terms of the effort required to transform the ASR output into a more accurate, verbatim transcript.
Errors were also assessed on a phonological level in order to explore whether varying phonetic realizations of features across accents could be responsible for transcription errors, with a particular focus on marked vocalic differences across SSBE and WYE. Substitutions involving content words in the Yorkshire English transcripts were analyzed by identifying which of Wells' lexical sets (i.e., group of words all sharing the same vowel phoneme; Wells, 1982) the words in the reference and automatic transcripts belong to as well as transcribing the speaker's production of the word, with the goal of better understanding why the error may have been made.
Four vocalic variables in particular were analyzed due to differences between the SSBE and WYE phonetic realizations (Wells, 1982; Hughes et al., 2005). These are outlined in Table 3, using Wells' (1982) lexical sets as a way of grouping words that share the same phoneme. Words in the BATH lexical set contain a long back vowel in SSBE, but typically contain a short front vowel in WYE, which overlaps with the production of the TRAP vowel [a] in both varieties. Words in the STRUT lexical set contain an unrounded low vowel in SSBE, but a rounded high vowel in WYE; the rounded high vowel [℧] is also produced in words belonging to the FOOT lexical set in both varieties. Words belonging to the FACE and GOAT lexical sets contain diphthongs in SSBE, but typically contain monophthongs in WYE.
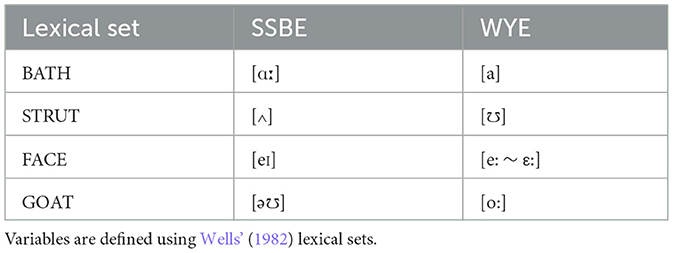
Table 3. Phonetic realizations of four vocalic variables across the two varieties of British English analyzed in this study, Standard Southern British English (SSBE) and West Yorkshire English (WYE).
3.4. Statistical analysis
In order to evaluate which factors had a significant effect on word error rate, three linear mixed effects models were fitted using the lme4 package (Bates et al., 2015) in R. In each model, regional accent, audio quality or ASR system was included as a fixed effect, and all models included Speaker and Sentence as random effects to account for variation across speakers within accent groups and the unique linguistic content of each utterance. A separate “null” model was fitted including only the random effects, and the ANOVA function in R was used to compare each full model with the null model. Results of the model comparisons indicate whether the full model is better at accounting for the variability in the data, and therefore whether the fixed effect has a significant impact on word error rate. Results of the model outputs, containing an Estimate, Standard Error rate and a p-value, were then examined to evaluate the relationship between variables. A threshold of α = 0.05 was used to determine statistical significance.
A three-way comparison was carried out for ASR system and in the first three models Amazon was used as a baseline, meaning that a comparison between Rev and Google had not been carried out. The “ASR system” variable was relevelled such that Rev became the baseline, and a fourth model was then fitted with ASR system as a fixed effect and Speaker and Sentence as random effects.
4. Results
4.1. ASR systems
The three automatic systems tested in this study performed with varying levels of success and were all clearly affected to some degree by the regional accent of the speaker and the level of background noise. Figure 1 shows WER in each condition for the three ASR systems. The four conditions are SSBE speech in studio quality audio, SSBE speech in audio with added speech-shaped noise, WYE speech in studio quality audio and WYE speech in audio with added speech-shaped noise; these will henceforth be referred to as SSBE studio, SSBE SSN, WYE studio and WYE SSN, respectively. Amazon was the best performing system with the lowest word error rate (WER) in each of the four conditions compared with Rev and Google, and achieved its lowest WER (13.9%) in the SSBE studio condition and highest WER (26.4%) in the WYE SSN condition. Google was the worst performing system, achieving the highest WER in every condition except for WYE speech in studio quality, for which Rev performed worst with a WER of 34.1%.
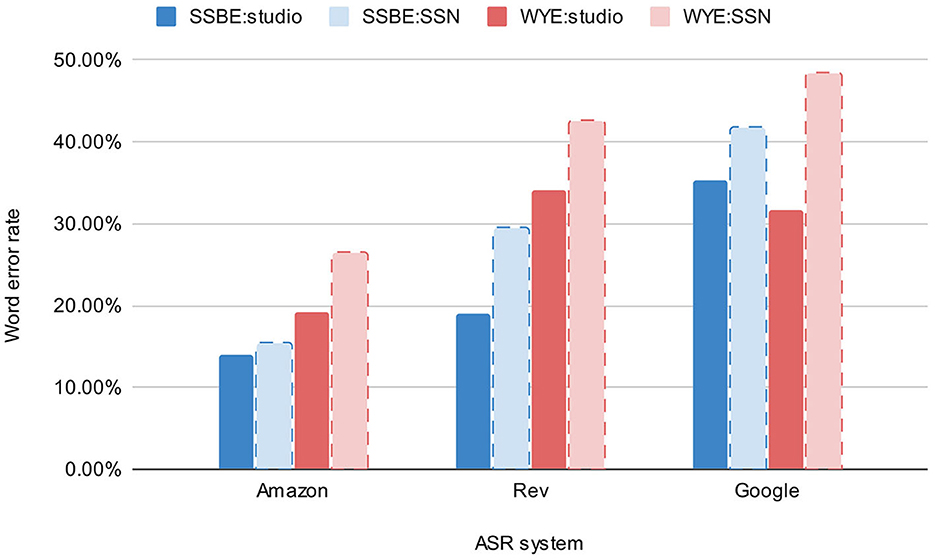
Figure 1. Average word error rate in each of the four conditions (SSBE studio, SSBE SSN, WYE studio, and WYE SSN) for all three ASR systems (Amazon, Rev, and Google). ASR systems are ordered from left to right according to lowest to highest average WER.
Results of a model comparison between the null model and the model with ASR system as a fixed effect revealed that ASR system has a significant impact on WER [ = 50.35, p < 0.0001]. The summary output of the linear mixed effects model revealed that there was a significant difference in error rates between Amazon and both Rev (β = 0.13, SE = 0.26, p < 0.001) and Google (β = 0.20, SE = 0.26, p < 0.001). Rev achieved WERs that were on average 13% higher than those produced by Amazon, while Google produced WERs on average 20% higher than Amazon. When comparing the two worst performing systems, Google was found to produce significantly higher WERs than Rev (β = 0.08, SE = 0.03, p < 0.005).
A notable trend in the data was Google's high tendency toward deletion errors, with over double (and in some cases quadruple) the number of deletions that Amazon produced in the same condition. An example of this is the utterance “not exactly I can't really remember their surnames but I might have known them I don't know” which was transcribed in studio quality by Amazon as “not exactly I can't remember their names but I might have known him I don't you” (with one deletion and three substitutions) and by Google as “not exactly I can't remember this sentence I don't know” (with seven deletions and two substitutions).
4.2. Regional accent
There are some clear differences in performance between the two accents in this study. Word error rate is lower for SSBE than for WYE in all conditions except for Google in the WYE studio condition; however, the results of a model comparison between the null model and the model with regional accent as a fixed effect showed that the difference in performance across accents was not statistically significant [ = 1.28, p = 0.26]. This is likely due to the extremely small sample size and variation in system performance across the speakers of each accent. All ASR systems produced higher WERs for one of the SSBE speakers, which were on average 13 and 20% higher than for the other SSBE speaker in studio quality audio and speech-shaped noise audio, respectively. One of the WYE speakers also proved more challenging for the ASR systems, though the difference was most pronounced in studio quality where WERs were on average 10% higher than for the other WYE speaker. An average difference of 4% was observed between the WYE speakers in speech-shaped noise audio, which is likely a result of the highest WERs in the study being observed in this condition.
The most common type of error also varied across accents, with deletions featuring most frequently for SSBE speech (see Table 4) and substitutions featuring most frequently for WYE speech (see Table 5). As discussed earlier in this paper, substitution errors can be viewed as more harmful than deletion errors in forensic contexts given that incorrect information has the potential to be much more damaging than reduced information. Substitutions may also have a stronger priming effect than other types of errors on the post-editors who are correcting an ASR transcript.
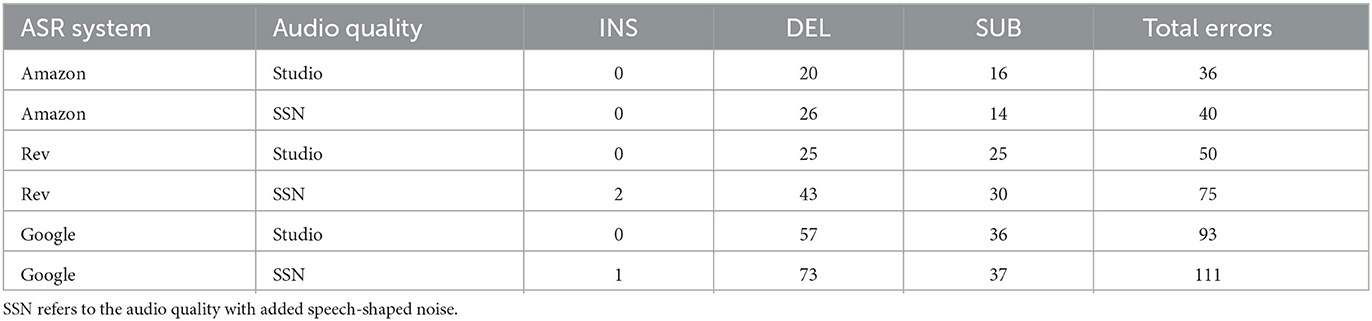
Table 4. Counts of each error type (insertions, deletions, and substitutions) produced by each system for Standard Southern British English speech.
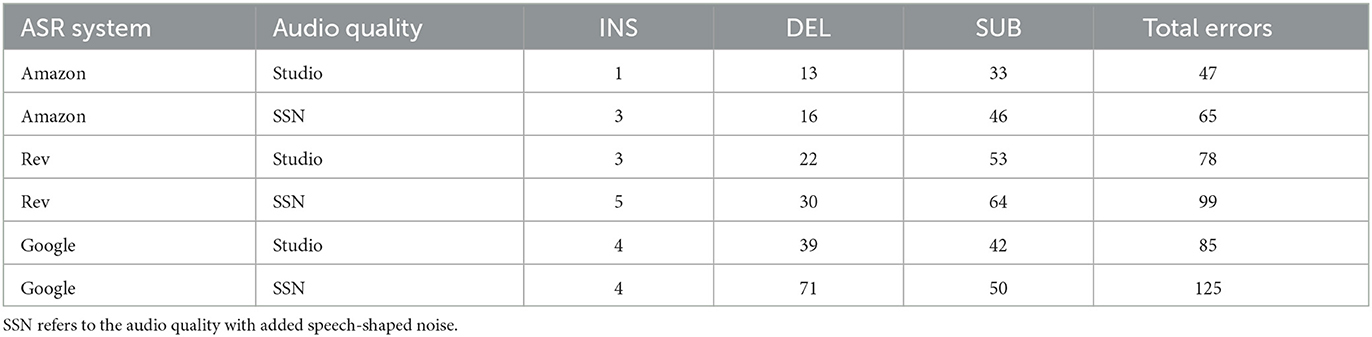
Table 5. Counts of each error type (insertions, deletions, and substitutions) produced by each system for West Yorkshire English speech.
55.6% of SSBE errors in studio quality audio and 62.7% of SSBE errors in speech-shaped noise audio were deletions. The number of deletions in SSBE was consistently higher than in WYE, though occasionally only by a relatively small margin. The majority of deletion errors involved short function words, such as “a” and “to,” which made up between 61.5 and 80% of all deletion errors for Rev and Google. Amazon made the fewest deletion errors out of all the ASR systems, and the majority of the deletions for SSBE speech involved the omission of filled pauses. The deletion of content words was much less frequent, accounting for 17.9% of all deletion errors for Rev and 16.3% of all deletion errors for Google. Amazon was the only system for which content words were never deleted.
Substitutions accounted for the most frequently occurring type of error for West Yorkshire English speech, with an average of 62.5% of all errors in studio condition and 58.5% of all errors in the speech-shaped noise condition involving the substitution of words or phrases. The only condition in which substitutions were not the most frequently occurring type of error for WYE speakers was Google in the speech-shaped noise condition where deletions constituted 71 of the 125 errors. The distribution of word types involved in substitution errors also differed across accents. The majority of substitutions for WYE speech involved content words while most substitutions for SSBE speech involved function words (Figure 2).
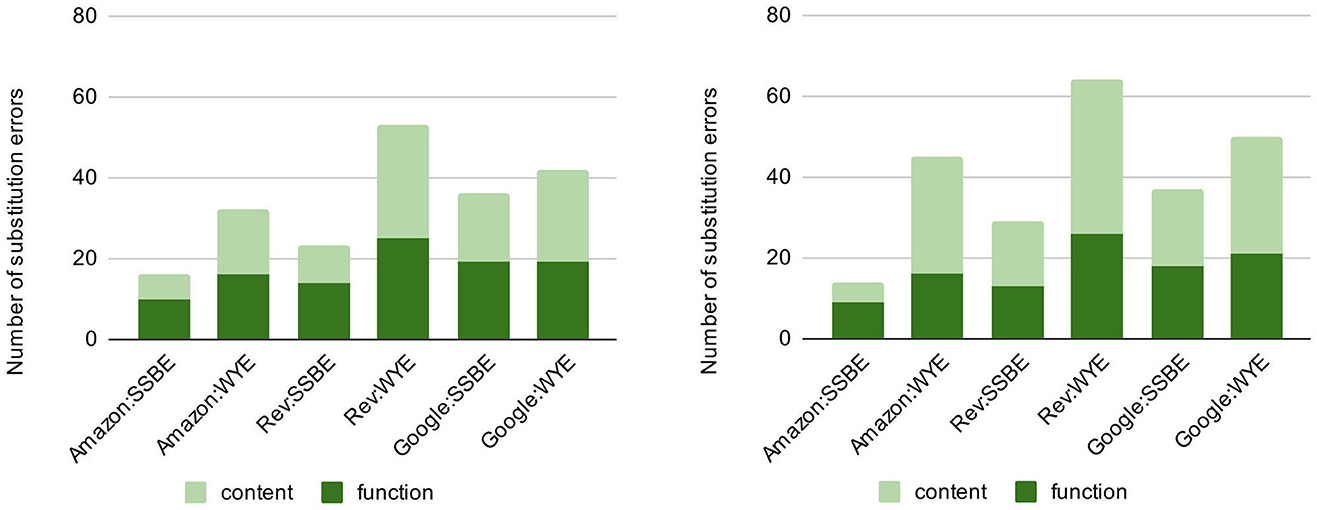
Figure 2. Number of substitution errors produced by each ASR system in each accent, in studio condition (left) and speech-shaped noise condition (right). ASR systems are ordered from left to right according to lowest to highest average WER.
Despite substitutions relating to function words accounting for a minority of substitution errors in WYE, there were more of this type of error in WYE than in SSBE. For Amazon and Rev, the number of content word-related substitutions was between 2 and 5 times higher for Yorkshire English than for SSBE, and the smaller increase for Google was likely a result of higher numbers of substitutions for SSBE speakers.
4.3. Audio quality
Higher error rates (by an average of 8%) were observed in speech-shaped noise audio compared with studio quality audio for all systems and for both accents. The results of a model comparison between the null model and the model with audio quality as a fixed effect showed that this difference was statistically significant [ = 11.42, p < 0.001], and examination of the model output confirmed that WER was significantly higher in the degraded audio condition (β = 0.08, SE = 0.02, p < 0.001). An increase was observed in the number of insertions and deletions in all conditions when comparing the transcripts of the studio quality recordings to the recordings with added speech-shaped noise. Rev and Google in particular show large increases in the number of deletions from studio condition to the speech-shaped noise condition. A very similar number of substitutions was observed across the audio qualities in SSBE, but the number of substitutions in WYE was 19–40% higher in the speech-shaped noise condition. The change in audio quality also affected the distribution of word types involved in substitutions. While the majority of substitution errors in SSBE were related to function words in studio quality audio, a majority involved content words in the speech-shaped noise condition for both Rev and Google. Not only was Amazon the highest performing system overall, it was also the least affected by the addition of background noise.
4.4. Phonological variables
Many errors within the West Yorkshire English data could be explained by a phonetic realization deviating from what might be expected based on the assumed underlying acoustic models. This was especially true in the case of vowels where the phonology deviates markedly from SSBE. Given that previous studies suggest an overrepresentation of more “standard” (in this context, Southern British) varieties in training data, we may expect to see the ASR systems struggling with some of the non-standard pronunciations of words by Yorkshire speakers. To explore this, four vowels which are well-known to differ in quality, length, or number of articulatory targets across SSBE and WYE were chosen for more in-depth analysis.
4.4.1. BATH
Words belonging to the BATH lexical set contain different vowels within the two accents: the long back vowel [ɑː] in SSBE and, like many other varieties from the North of England, the short front vowel [a] in WYE. There were few occurrences of words belonging to the BATH lexical set in the Yorkshire data, though there were two utterances of the word “staff ,” one by each of the Yorkshire speakers, which were produced with a short front vowel, i.e., [staf], rather than a long back vowel, i.e., [stɑːf]. All three systems correctly transcribed this word for one speaker but not for the other. The pronunciations themselves were very similar but the surrounding context of the word was likely the cause of this issue. In the successful case, “staff ” was uttered at the beginning of an intonational phrase but in the other occurrence it was preceded by a non-standard pronunciation of “with” [wIʔ]. Omission of word-final fricatives, most commonly in function words, is a common process in some varieties of Yorkshire English (Stoddart et al., 1999). In this case, the voiced dental fricative /ð/ has been replaced with a glottal stop, resulting in the utterance [wIʔstaf] which Rev and Google both analyzed as one word, transcribing “waste” and “Wigston,” respectively. Amazon mistranscribed the word “staff ” as “stuff ,” a substitution which could be the result of the Yorkshire vowel being replaced with the closest alternative that creates a word in Standard Southern British English. Since [staf] in this case is not recognized as the word “staff ,” the closest SSBE alternative is the word “stuff ” which contains a low central vowel [∧] that is closer within the vowel space to the uttered vowel than [ɑː].
4.4.2. STRUT
There is a systemic difference between SSBE and WYE with regards to the number of phonemes in each accent's phonological inventory, whereby the SSBE STRUT vowel/∧/does not feature in WYE. Instead, [℧] is produced in words belonging to both the STRUT and FOOT lexical sets. Many words containing this vowel were correctly transcribed within the Yorkshire data, though some occurrences resulted in phonologically-motivated substitutions. The word “bus,” pronounced [bʊs] by the Yorkshire speaker, was correctly transcribed by Amazon and Google but proved challenging for Rev which replaced it with “books,” a word containing [℧] in SSBE and belonging to the FOOT lexical set. A similar pattern was observed for the word “cut,” pronounced [kʊʔ], which Amazon and Google transcribed (almost correctly) as the present participle “cutting,” while Rev substituted it with a word from the FOOT lexical set, “couldn't.”
The word “muddy,” pronounced [mʊdI] by the Yorkshire speaker, proved challenging for all three systems. In both audio qualities, Amazon mistranscribed this word as “moody”/mu:di/, retaining the consonants but replacing the vowel with the closest alternative that creates a plausible word. Interestingly, Rev and Google both transcribed “much” in place of “muddy,” correctly recognizing the word uttered as belonging to the STRUT lexical set despite the high rounded quality of the vowel [℧].
Another example of a Yorkshire word belonging to the STRUT lexical set that proved to be challenging for the ASR systems was “haircut,” pronounced [εːkʊʔ], though this was likely due to the h-dropping that takes places in word-initial position. Google semi-successfully transcribed “cut,” ignoring the first vowel in the word, while Amazon and Rev transcribed “airport” and “accurate,” respectively. The lack of /h/ at the beginning of “haircut” had a clear impact on the words consequently transcribed, since both begin with a vowel. This seems to have then had an effect on the vowel transcribed in the second syllable, as these systems transcribed final syllables containing the vowels [ɔ:] or [℧] in SSBE.
4.4.3. FACE
Words belonging to the FACE lexical set are subject to realizational differences across the accents; the FACE vowel is realized as the diphthong [eI] in SSBE but as the long monophthong [e:] in WYE. Most words containing this vowel were transcribed correctly, e.g., “rains” and “place,” despite the monophthongal quality of the vowel produced by the Yorkshire speaker. However, some occurrences of [e:] proved challenging. For example, the word “potatoes,” pronounced [p(ə)te:ʔəZ] with a glottal stop in place of the second alveolar plosive, was incorrectly transcribed as “tears,” “debt is,” and “date is” by Amazon, Rev and Google, respectively. While Google transcribes a word containing the correct vowel [eI] (“date”), the other systems transcribe words containing the vowels [εə] and [ε], which share similar vocalic qualities with the front mid vowel uttered by the speaker in terms of vowel height, frontness and steady state (or very little articulatory movement). Given that Rev and Google both transcribe words containing /t/ after the FACE vowel, it seems unlikely that the mistranscriptions are a result of the glottal stop, and are rather a direct result of the monophthongal realization of the FACE vowel.
4.4.4. GOAT
Words belonging to the GOAT lexical set vary in their phonetic realization across the two accents, such that the diphthong [ə℧] features in SSBE but a long monophthong features in WYE, which can be realized in a number of ways. Traditionally this was produced as a back vowel [o:] but it has undergone a process of fronting (Watt and Tillotson, 2001; Finnegan and Hickey, 2015) to [ɵː] for many younger speakers, including the two Yorkshire speakers in this study. Some words containing this vowel were transcribed without issue, such as “own” and “go,” though it should be noted that the latter was relatively diphthongal in quality given the phonological environment: the following word “in” begins with a vowel therefore a [w]-like sound is inserted, leading to movement during the vowel and creating a sound much closer to the SSBE diphthong [ə℧].
Other words containing the fronted monophthong proved more challenging for the systems, such as “drove” which was mistranscribed as “drew if ,” “do if ,” and “if ” by Amazon, Rev, and Google, respectively. Amazon and Rev replace [ɵː] with words containing the vowel [u:], an alternative long monophthong produced in a relatively similar part of the vowel space, followed by [I] and the voiceless version of the labiodental fricative. Google omitted the GOAT vowel, transcribing only the word “if” in studio quality audio and deleting the word completely in the speech-shaped noise condition. The word “road,” pronounced [ɹɵːḍ], was also mistranscribed by two of the systems as “word” (/wɜ:d/), whereby the central monophthongal quality of the vowel was retained but the height was slightly adjusted to give [ɜ:].
4.5. Post-editing
In order to assess the possibility of incorporating an ASR output into the transcription process, it is necessary to assess the effort required to transform the ASR output into a more accurate (verbatim) transcript. The best performing system, Amazon, was evaluated in terms of the frequency and types of errors produced, as well as the difficulty of error identification within the data. Deletion and insertion errors may be more easily detectable than substitution errors, as suggested by Papadopoulou et al. (2021), in many contexts; in principle, these errors should stand out as missing or extraneous when the transcriber listens to the audio, while substitution errors may be more challenging to identify, especially if closely resembling the speech sounds in the audio recording.
In studio quality, 20 deletions were produced for SSBE speech and 13 for WYE speech, and in both cases, more than half of the deletion errors involved the omission of filled pauses. The rest of the deletions involved function words, and in almost all cases the transcription remained relatively unchanged in terms of semantic meaning, e.g., “I can't really remember” → “I can't remember,” or “half an hour something like that depending on traffic” → “half an hour depending on traffic.” In the speech-shaped noise condition, 26 deletions were produced for SSBE and 16 for WYE. Fifty percent of the errors for SSBE involved filled pauses while the majority of WYE deletions (11/16) involved function words, and most deletions did not affect the semantic meaning of the utterance, e.g., “except for when it rains” → “except when it rains” or “he's a tour guide and I knew him from secondary school” → “he's a tour guide I knew from [a] secondary school.” Furthermore, some of the deletions occurred in instances where a pronoun or determiner, e.g., “I” or “a,” had been repeated, such that the transcript contained only one instance of each word.
Insertions were extremely rare within the data, particularly for Amazon which did not produce any insertions for SSBE and only inserted 1–3 words in the WYE transcripts. In studio quality, the only insertion to be made was “I knew him from secondary school” → “I knew [him] from a secondary school,” which is easily detectable given that the insertion of the determiner sounds unnatural in this context. The same insertion was made in the SSN condition, along with the insertion of first-person pronoun “I” and determiner “a.”
Substitutions may require more cognitive effort to identify, particularly in cases where the word in the transcript closely resembles the word that is uttered. First, the substitution of content words was assessed given that this type of mistranscription could lead to serious errors in forensic contexts, e.g., if a non-incriminating word such as “gum” is substituted with an incriminating alternative like “gun.” In studio quality, six content words in SSBE and 16 in WYE were subject to substitution errors. The majority of SSBE substitutions in this case involved morphological alterations, such as a change in tense (e.g., “finish” → “finished”) or omission of an affix (e.g., “surnames” → “names”). Due to the phonetic similarity of the target and transcribed word, these substitutions could be difficult to notice in a post-editing phase, and an uncorrected change in tense could, in some circumstances, have a significant impact on the meaning of the utterance. However, the morphological alterations in the data were all relatively clear; either the change in tense was held in stark contrast to the tense used in the rest of the utterance, or it was coupled with another error which would indicate that the section needs closer review.
The remaining two errors were relatively easy to identify from the context of the utterance; the utterance-final phrase “I don't know” was mistranscribed as “I don't you” and “a really big yew tree right next to it” was mistranscribed as “a really big utility right next to it.” A much bigger proportion (11/16) of the WYE content-based substitutions involved non-morphological alterations, but the majority of these were easy to identify from context alone, such as the phrase “it's bit uh cut and chop with staff ” which was transcribed by Amazon as “it's bitter cutting chocolate stuff .” The words directly preceding this part of the utterance referenced the frequent hiring of new staff, therefore the reference to “cutting chocolate” seems misplaced in this context. Other WYE substitutions included “airport” in place of “giving him an haircut” and “moody” in place of “when it rains it gets very muddy.”
In the speech-shaped noise condition, there were a very similar number of content-based substitutions in SSBE (5) while the number increased substantially for WYE from 16 to 29, only six of which involved morphological alterations. The rest of the errors were relatively clear from context, e.g., “I had a bit of dessert” → “I had a bit of Giza” when talking about lunch or “did have a sack of potatoes” → “did have a sacrum tears,” making them easy to identify when comparing the audio recording and the ASR transcript, and potentially even from simply reading the transcript through without audio.
The substitution of function words could be more difficult to detect in some cases as short grammatical words are generally paid little conscious attention and glossed over in reading tasks (Van Petten and Kutas, 1991; Chung and Pennebaker, 2007), and the meaning of the utterance often remains unchanged. For example, there is little difference between “go in get my drinks” and “go and get my drinks” in the context of visiting a pub. Substitutions involving function words featured around 10 times in SSBE and 16 times in WYE in both audio qualities, and the majority of these were relatively inconsequential, e.g., “the steak house” → “a steak house” and “that's quite hard to see” → “it's quite hard to see.” However, a number of the errors involved the substitution of pronouns (see Table 6), which could be extremely difficult to notice due to similar pronunciations, but could be problematic within a forensic context if left uncorrected.
5. Discussion
5.1. ASR performance
The present study set out to investigate the reliability of ASR transcripts with simulated police interview recordings by exploring the impact of regional accent and audio quality on the transcription performance of three popular commercially-available ASR systems. Results revealed that the ASR system used and the audio quality of the recording had a significant effect on word error rate, and though regional accent was not found to significantly predict WER, clear differences were observed across the two accents in terms of the frequency and types of errors made.
5.1.1. ASR system and audio quality
With regards to the commercial ASR systems chosen for this study, Amazon Transcribe was clearly the best-performing system, consistently achieving the lowest WER in each condition: 13.9 and 15.4% for SSBE in studio quality and the speech-shaped noise condition, respectively, and 19.2 and 26.4% for WYE in studio quality and the speech-shaped noise condition, respectively. Google Cloud Speech-to-Text achieved the highest WER in almost every condition, and error rates for this ASR system were significantly higher than those for both Amazon and Rev, as well as consistently above 30%. Rev AI had the most variable performance, ranging from 19.0 to 42.5%. The patterns observed across accents and audio qualities were relatively consistent within each system, but the specific reason behind the difference in performance across systems is not clear, especially given the “black box” nature of proprietary automatic systems. The addition of speech-shaped noise to the audio recordings was found to have a significant effect on word error rate, leading to a higher frequency of errors in almost every condition. However, it must be noted that Amazon Transcribe, the best performing system, was the least affected by the addition of speech-shaped noise, with WERs increasing by only 1.5% in SSBE and 7.2% in WYE between the two audio qualities.
5.1.2. Regional accent
Word error rate was not found to be significantly impacted by regional accent in this study, although this was likely due to variation between speakers and the small sample size. A clear pattern emerged whereby one speaker of each accent was favored by the ASR systems, and performance for the best WYE speaker was roughly level with performance for the worst SSBE speaker.
Variation in system performance within an accent group has recently been investigated by Harrison and Wormald (in press), a study in which test data from a sociolinguistically-homogenous group was transcribed using Amazon Transcribe. Despite demographic factors such as age, accent and educational background as well as the content of the recordings being relatively controlled, a high level of variability was observed across speakers, with word error rates ranging from 11 to 33%. The variation across speakers observed in this study is therefore unsurprising, although the systematic effects of variety may emerge on a larger data set, as reported by Markl (2022).
Despite the lack of a statistically significant difference in WER across the accents, a higher number of errors were produced for the West Yorkshire English speech compared with the Standard Southern British English speech, and the majority of errors for the non-standard regional accent involved the substitution of words or phrases. Substitution errors can be extremely damaging in forensic contexts, particularly when the quality of the audio is poor. It is possible that deletion and insertion errors will be easier to identify alongside the audio within a transcript, but if the listeners have been “primed” by an alternative interpretation of a word or phrase (i.e., a substitution) then the identification of that error will in all likelihood be more challenging.
There are a number of factors likely contributing to the disparity in performance between accents. Modern ASR systems tend to involve two components, an acoustic model and a language model. Research on performance gaps between accent groups suggests that many ASR performance issues concerning “accented” speech stem from an insufficiently-trained acoustic model, which is caused by a lack of representation of non-standard accents in training data (Vergyri et al., 2010; Dorn, 2019; Markl, 2022). There were many errors within the Yorkshire data that can be attributed to a phonetic realization deviating from SSBE, a large number of which involved vowels for which phonemic and realizational differences are observed across the accents. Numerous errors were likely the result of a combination of vocalic and consonantal differences between SSBE and WYE; for example, the combination of h-dropping and a Northern realization of the STRUT vowel in “haircut” led to substantial substitutions by two of the systems.
Although the main focus of the fine-grained phonetic analysis was on errors seemingly caused by issues with the acoustic model, there were some errors that could not be attributed to acoustics and instead were likely a reflection of the language model. The language model calculates the conditional probability of words in a sequence, i.e., how likely is it that word D will follow on from words A, B, and C. Utterances containing non-standard grammar are therefore likely to cause problems for ASR systems, a few examples of which were observed in the Yorkshire data. The lack of a subject pronoun in the utterance “did have a sack of potatoes in front” led to the insertion of the pronouns “I” and “you” by Rev and Google respectively, both positioned after the verb “did.” The omission of the determiner in the phrase “in the front” led to the insertion of the verb “is” before this phrase, i.e., “is in front,” by both Rev and Google. Another example of an error likely resulting from the language model is the insertion of the indefinite article into the phrase “from secondary school,” transcribed by Amazon as “from a secondary school.” Having reviewed the audio, there is no phonetic explanation for this insertion given that the nasal [m] is immediately followed by the fricative [s], therefore this insertion is likely due to the language model calculating that the sequence of words including “a” is more probable.
5.1.3. Error analysis
A WER of 5% is generally accepted as a good quality transcript (Microsoft Azure Cognitive Services, 2022) but if the errors within that transcript lead to significant changes to the content, then that transcript could be harmful in a court of law. WER alone cannot indicate whether a system is good enough to use in a legal setting, such as the transcription of police-suspect interviews. Fine-grained phonetic analysis of the errors produced is a much more informative approach that can highlight any major issues with a system such as high rates of substitution errors. This type of analysis could also help to identify common issues in ASR transcripts that could subsequently be built into training for police transcribers, if a computer-assisted approach to police-suspect interview transcription was adopted. However, this method of analysis is extremely labor-intensive in nature and is therefore not feasible for large data sets. A combination of the two approaches, in which WER is calculated for a large data set and a subset of the data is subject to more detailed analysis of the frequency, type and magnitude of the errors, may be more suitable.
5.2. Post-editing
One of the aims of this paper is to investigate the possibility of incorporating automatic transcription into the production of police interview transcripts. The transcripts produced by the three commercial ASR systems in this experiment would not be suitable for use without manual correction, which is to be expected given that this is a commonly acknowledged issue in the field of automatic speech recognition (Errattahi et al., 2018). The question to be addressed is therefore whether the automatic transcripts could act as a first draft which is then reviewed and corrected by a human transcriber.
Gaur et al. (2016) found that editing an ASR output actually takes longer than producing a transcript from scratch once the WER surpasses 30%. Given that the average WER for Google exceeded 30% in every condition, and in all but one condition the WER for Rev was more than 29%, neither of these systems would be adequate for the purpose of producing a first draft of a transcript to be corrected by a human transcriber. In contrast, WERs produced by Amazon ranged from 13.9 to 26.4%, falling into the range of “acceptable but additional training should be considered” according to Microsoft Azure documentation (Microsoft Azure Cognitive Services, 2022). Gaur et al. (2016) found that participants benefitted from the ASR transcript provided the word error rate was low, i.e., below 30%. It is therefore possible that utilizing the Amazon transcripts as a first draft to be edited could reduce the time necessary to produce verbatim transcripts.
Closer inspection of the transcripts produced by Amazon revealed that many of the errors should, in principle, be easy to identify or would be relatively inconsequential if left uncorrected. For example, over 50% of the deletion errors in studio quality audio involved the omission of filled pauses like “uh” and “um,” which is unlikely to have a substantial effect on the reader's perception of the speech and the speaker. Most deletions in speech-shaped noise audio involved short function words, and in almost all cases the meaning of the utterance was unaffected by their omission. Insertions were very rare within the data but were quite easily identifiable from context or were paired with a substitution error. The substitution of content words, particularly for the Yorkshire English speech, was generally evident from context since the resulting transcript was often ungrammatical or non-sensical, and substitution errors involving function words generally made no difference to the meaning of the utterance. The exception to this was the substitution of pronouns and content words with morphologically-related terms (though cases of the latter in this data were relatively easy to identify); these errors would likely be much harder to spot due to the phonetic similarity between the word uttered and the substituted term.
5.2.1. Potential challenges
A potential challenge with the task of correcting a transcript is that post-editors could be “primed” (i.e., heavily influenced) by the content of the ASR output to such an extent that errors go unnoticed. Research in the field of forensic transcription has found that seeing an inaccurate version of a transcript can cause people to “hear” the error in the audio (Fraser et al., 2011; Fraser and Kinoshita, 2021). However, the quality of audio recordings in forensic cases is often extremely poor and the speech is “indistinct,” resulting in a reliance on top-down information such as expectations about the speech content (Fraser, 2003). In the case of police-suspect interviews, where the audio quality is often relatively good in comparison to forensic recordings, transcribers may be less susceptible to the effects of priming. It is also worth noting that many of the errors produced by the ASR systems were easy to identify from contextual knowledge or due to the non-sensical nature of the substitution. For example, one ASR transcript contained “giving him an airport” in place of “given him an (h)aircut” which, despite the similar phonetic content, is unlikely to influence a post-editor due to the implausibility of the utterance. Minor deletion errors, such as the omission of filled pauses, could be more challenging to identify in a transcript, though in many cases this would likely be inconsequential with regards to the readability of the transcript and the reader's perception of the speech and speaker.
Another potential issue is that errors in transcripts with a low WER may be more difficult to identify. As suggested by Sperber et al. (2016), post-editors may miss errors due to a lack of attention, and this effect would likely be increased in cases where the transcript is almost completely accurate and an excessive amount of confidence is placed in the performance of the automatic system. It is possible that the user interface employed could help to address this problem. Sperber et al. (2016) suggest two methods for focusing transcriber attention and therefore decreasing the chance of missing transcription errors: highlighting low-confidence words in red, and typing from-scratch with the ASR hypothesis visible. Both methods were shown to improve the quality of the transcript (i.e., decrease WER) and reduce the time taken, and it was also found that different strategies work best for different levels of WER. Retyping (with the ASR output visible) gave the best results for segments with a high WER, while editing the ASR transcript text gave the best results for low WER segments.
5.3. Future work
This study used a small sample of commercially-available automatic speech recognition systems and has shown that not all ASR systems are suitable for the task of producing a “first draft” transcript, as evidenced by the frequency of errors produced by Rev AI and Google Speech-to-Text. However, promising performance was demonstrated by one of the systems tested and further analysis of the errors suggests that post-editing an ASR transcript, provided it is of adequate quality, is a worthwhile topic to explore in the context of police-suspect interviews. This approach could facilitate the production of verbatim transcripts of interviews without a substantially higher time requirement than the current practice of summarizing the majority of the recording.
Future work on this topic should focus on two areas: ASR performance in a range of audio, speaker and speech conditions, and post-editing. In the present study, the addition of speech-shaped noise to the recordings may not have created an audio quality representative of real police-suspect interview data. It would therefore be interesting to use real recordings to investigate the capabilities of this technology. Other factors that may impact the system's performance and would be present in police-suspect interviews include different levels and types of background noise, multiple speakers, other regional accents, and longer stretches of speech.
More research is also required on the topic of post-editing. Papadopoulou et al. (2021) claims to be one of the first studies to employ qualitative analysis on automatic transcription errors and to evaluate the post-editing effort required in correcting ASR transcripts. Incorporating ASR outputs into the transcription process has been investigated by others, though these studies tend to focus on optimizing efficiency (Sperber et al., 2016, 2017) or simply report on the use of a computer-assisted transcription approach, e.g., for meetings of the National Congress of Japan (Akita et al., 2009) or for speeches in the Icelandic parliament (Fong et al., 2018). Transcripts have been found to be highly influential on the perception of speech content when the audio quality of the recording is extremely poor, but more research is required on the priming effects of ASR transcripts in the context of post-editing police-suspect interviews, i.e., on comparatively better quality audio. Furthermore, it is crucial to investigate the practicalities of correcting an ASR transcript of a police-suspect interview. For example, how many errors are missed by post-editors, and what are the consequences of leaving those errors in the transcript? How long does it take to correct an ASR transcript of a full police-suspect interview, and how does this compare to the current time taken to create ROTIs? Future research should explore these questions as the incorporation of automatic speech recognition into the transcription process could be extremely beneficial.
Data availability statement
The raw data supporting the conclusions of this article will be made available by the authors, without undue reservation.
Ethics statement
Ethical approval was not required for the study involving human data in accordance with the local legislation and institutional requirements. Written informed consent to participate in this study was not required in accordance with the national legislation and the institutional requirements.
Author contributions
The author confirms being the sole contributor of this work and has approved it for publication.
Funding
This work was supported by the Arts and Humanities Research Council (grant number: AH/R012733/1) through the White Rose College of the Arts and Humanities. Open access publication fees were covered by the York Open Access Fund at the University of York.
Conflict of interest
The author declares that the research was conducted in the absence of any commercial or financial relationships that could be construed as a potential conflict of interest.
Publisher's note
All claims expressed in this article are solely those of the authors and do not necessarily represent those of their affiliated organizations, or those of the publisher, the editors and the reviewers. Any product that may be evaluated in this article, or claim that may be made by its manufacturer, is not guaranteed or endorsed by the publisher.
Supplementary material
The Supplementary Material for this article can be found online at: https://www.frontiersin.org/articles/10.3389/fcomm.2023.1165233/full#supplementary-material
Footnotes
1. ^West Yorkshire English shares some features (e.g., lack of TRAP-BATH and FOOT-STRUT splits) with General Northern English (GNE), an emerging variety of Northern British English which is the result of dialect leveling (Strycharczuk et al., 2020). However, there are some features that make WYE distinct from GNE, such as the monophthongization of vowels in words like “face” and “goat.”
2. ^Rev AI accessed 12th November 2021.
3. ^Amazon Transcribe accessed 17th October 2022.
4. ^Google Cloud Speech-to-Text accessed 13th October 2022.
References
Akita, Y., Mimura, M., and Kawahara, T. (2009). “Automatic transcription system for meetings of the japanese national congress,” in Interspeech 2009 (ISCA), 84–87.
Bates, D., Mächler, M., Bolker, B., and Walker, S. (2015). Fitting linear mixed-effects models using ‘lme4.' J. Stat. Softw. 67, 1–48. doi: 10.18637/jss.v067.i01
Boersma, P., and Weenink, D. (2022). Praat: Doing Phonetics by Computer. Available online at: http://www.praat.org/ (accessed November, 2022).
Bokhove, C., and Downey, C. (2018). Automated generation of ‘good enough' transcripts as a first step to transcription of audio-recorded data. Methodol. Innov. 11, 205979911879074. doi: 10.1177/2059799118790743
Chung, C., and Pennebaker, J. W. (2007). The psychological functions of function words. Soc. Commun. 1, 343–359. doi: 10.4324/9780203837702
Coulthard, M. (2013). The official version: audience manipulation in police records of interviews with suspects. Texts Practices 16, 174–186. doi: 10.4324/9780203431382-16
DiChristofano, A., Shuster, H., Chandra, S., and Patwari, N. (2022). Performance Disparities Between Accents in Automatic Speech Recognition. arXiv [cs.CL]. Available online at: http://arxiv.org/abs/2208.01157
Dorn, R. (2019). “Dialect-specific models for automatic speech recognition of African American vernacular English,” in Proceedings of the Student Research Workshop Associated with RANLP 2019. Student Research Workshop Associated with RANLP 2019. (Varna: Incoma Ltd.), 16–20.
Errattahi, R., El Hannani, A., and Ouahmane, H. (2018). Automatic speech recognition errors detection and correction: a review. Proc. Comput. Sci. 128, 32–37. doi: 10.1016/j.procs.2018.03.005
Feng, S., Kudina, O., Halpern, B. M., and Scharenborg, O. (2021). Quantifying Bias in Automatic Speech Recognition. arXiv [eess.AS]. Available online at: http://arxiv.org/abs/2103.15122
Finnegan, K., and Hickey, R. (2015). Sheffield. Researching Northern English. (Amsterdam; Philadelphia, PA: John Benjamins Publishing Company), 227–250. doi: 10.1075/veaw.g55.10fin
Fong, J. Y., Borsky, M., Helgadóttir, I. R., and Gudnason, J. (2018). Manual Post-editing of Automatically Transcribed Speeches from the Icelandic Parliament - Althingi. arXiv [eess.AS]. Available online at: http://arxiv.org/abs/1807.11893
Fraser, H. (2003). Issues in transcription: factors affecting the reliability of transcripts as evidence in legal cases. Int. J. Speech Lang. Law 10, 203–226. doi: 10.1558/sll.2003.10.2.203
Fraser, H. (2020). “Forensic transcription: the case for transcription as a dedicated branch of linguistic science,” in The Routledge Handbook of Forensic Linguistics. Available online at: taylorfrancis.com (accessed October, 2022).
Fraser, H., and Kinoshita, Y. (2021). Injustice arising from the unnoticed power of priming: how lawyers and even judges can be misled by unreliable transcripts of indistinct forensic audio. Crim. Law J. 45, 142–152. Available online at: https://search.informit.org/doi/abs/10.3316/agispt.20210923053902
Fraser, H., Stevenson, B., and Marks, T. (2011). Interpretation of a Crisis Call: persistence of a primed perception of a disputed utterance. Int. J. Speech Lang. Law 18, 261. doi: 10.1558/ijsll.v18i2.261
Gaur, Y., Lasecki, W. S., Metze, F., and Bigham, J. P. (2016). “The effects of automatic speech recognition quality on human transcription latency,” in Proceedings of the 13th International Web for All Conference. (New York, NY: Association for Computing Machinery), 1–8. doi: 10.1145/2899475.2899478
Gillick, L., Baker, J., Baker, J., Bridle, J., Hunt, M., Ito, Y., et al. (1993). “Application of large vocabulary continuous speech recognition to topic and speaker identification using telephone speech,” in 1993 IEEE International Conference on Acoustics, Speech, and Signal Processing, vol. 2, 471–474. doi: 10.1109/ICASSP.1993.319343
Godfrey, J., and Holliman, E. (1993). Switchboard-1 Release 2 LDC97S62. Philadelphia, PA: Linguistic Data Consortium.
Gold, E., Ross, S., and Earnshaw, K. (2018). “The ‘west Yorkshire regional English database': investigations into the generalizability of reference populations for forensic speaker comparison casework,” in Interspeech 2018. (Hyderabad: ISCA), 2748–2752. doi: 10.21437/Interspeech.2018-65
Hain, T., Woodland, P. C., Evermann, G., Gales, M. J. F., Liu, X., Moore, G. L., et al. (2005). Automatic transcription of conversational telephone speech. IEEE Trans. Audio Speech Lang. Process. 13, 1173–1185. doi: 10.1109/TSA.2005.852999
Harrington, L., Love, R., and Wright, D. (2022). “Analysing the performance of automated transcription tools for covert audio recordings,” in Conference of the International Association for Forensic Phonetics and Acoustics, July (Prague).
Harrison, P., and Wormald, J. (in press). “Forensic transcription questioned utterance analysis,” in Oxford Handbook of Forensic Phonetics, eds F. Nolan, T. Hudson, K. McDougall (Oxford: OUP).
Haworth, K. (2018). Tapes, transcripts and trials: The routine contamination of police interview evidence. Int. J. Evid. Proof 22, 428–450. doi: 10.1177/1365712718798656
Haworth, K. (2020). “Police interviews in the judicial process: police interviews as evidence,” in The Routledge Handbook of Forensic Linguistics, eds M. Coulthard, A. May, and R. Sousa-Silva (London: Routledge), 144–158. doi: 10.4324/9780429030581-13
Hickey, R. (2015). “Researching northern English,” in Varieties of English Around the World, G55, ed R. Hickey (Amsterdam: John Benjamins Publishing), 1–493.
Hughes, A., Trudgill, P., and Watt, D. (2005). English Accents and Dialects: An Introduction to Social and Regional Varieties in the British Isles. London: Atlantic Publications, Inc.
Jenks, C. J. (2013). Working with transcripts: an abridged review of issues in transcription. Lang. Linguist. Compass 7, 251–261. doi: 10.1111/lnc3.12023
Johnson, M., Lapkin, S., Long, V., Sanchez, P., Suominen, H., Basilakis, J., et al. (2014). A systematic review of speech recognition technology in health care. BMC Med. Informat. Decision Mak. 14, 94. doi: 10.1186/1472-6947-14-94
Koenecke, A., Nam, A., Lake, E., Nudell, J., Quartey, M., Mengesha, Z., et al. (2020). Racial disparities in automated speech recognition. Proc. Natl. Acad. Sci. U. S. A. 117, 7684–7689. doi: 10.1073/pnas.1915768117
Kowal, S., and O'Connell, D. C. (2014). “Transcription as a crucial step of data analysis,” in The SAGE Handbook of Qualitative Data Analysis, ed U. Flick (Thousand Oaks, CA: Sage), 64–79. doi: 10.4135/9781446282243.n5
Lima, L., Furtado, V., Furtado, E., and Almeida, V. (2019). “Empirical analysis of bias in voice-based personal assistants,” in Companion Proceedings of The 2019 World Wide Web Conference (New York, NY: Association for Computing Machinery), 533–538. doi: 10.1145/3308560.3317597
Lippmann, R. P. (1997). Speech recognition by machines and humans. Speech Commun. 22, 1–15. doi: 10.1016/S0167-6393(97)00021-6
Littlefield, J., and Hashemi-Sakhtsari, A. (2002). The Effects of Background Noise on the Performance of an Automatic Speech Recogniser. Defence Science and Technology Organisation Salisbury (Australia) Info. Available online at: https://apps.dtic.mil/sti/citations/ADA414420 (accessed October, 2022).
Loakes, D. (2022). Does automatic speech recognition (ASR) have a role in the transcription of indistinct covert recordings for forensic purposes? Front. Commun. 7, 803452. doi: 10.3389/fcomm.2022.803452
Markl, N. (2022). “Language variation and algorithmic bias: understanding algorithmic bias in British English automatic speech recognition,” in 2022 ACM Conference on Fairness, Accountability, and Transparency (New York, NY: Association for Computing Machinery), 521–534. doi: 10.1145/3531146.3533117
Meyer, J., Rauchenstein, L., Eisenberg, J. D., and Howell, N. (2020). “Artie bias corpus: an open dataset for detecting demographic bias in speech applications,” in Proceedings of the Twelfth Language Resources and Evaluation Conference (Marseille: European Language Resources Association), 6462–6468.
Microsoft Azure Cognitive Services (2022). Test Accuracy of a Custom Speech Model. Microsoft Azure Cognitive Services. Available online at: https://learn.microsoft.com/en-us/azure/cognitive-services/speech-service/how-to-custom-speech-evaluate-data?pivots=speech-studio (accessed February 2, 2023).
Mishra, A. (2021). What is Rev AI's Accuracy? Available online at: https://help.rev.ai/en/articles/3813288-what-is-rev-ai-s-accuracy (accessed January 23, 2023).
Mošner, L., Wu, M., Raju, A., Parthasrathi, S. H. K., Kumatani, K., Sundaram, S., et al. (2019). “Improving noise robustness of automatic speech recognition via parallel data and teacher-student learning,” in ICASSP 2019 - 2019 IEEE International Conference on Acoustics, Speech and Signal Processing (ICASSP). (Brighton), 6475–6479. doi: 10.1109/ICASSP.2019.8683422
Nolan, F., McDougall, K., de Jong, G., and Hudson, T. (2009). The DyViS database: style-controlled recordings of 100 homogeneous speakers for forensic phonetic research. Int. J. Speech Lang. Law. 16, 31–57. doi: 10.1558/ijsll.v16i1.31
O'Shaughnessy, D. (2008). Invited paper: automatic speech recognition: history, methods and challenges. Pat. Recogn. 41, 2965–2979. doi: 10.1016/j.patcog.2008.05.008
Papadopoulou, M. M., Zaretskaya, A., and Mitkov, R. (2021). “Benchmarking ASR systems based on post-editing effort and error analysis,” in Proceedings of the Translation and Interpreting Technology Online Conference (Ashburn, VA: INCOMA Ltd.), 199–207. doi: 10.26615/978-954-452-071-7_023
Paulus, T., Lester, J., and Dempster, P. (2013). Digital Tools for Qualitative Research. Newcastle upon Tyne: SAGE.
Punch, K. F., and Oancea, A. (2014). Introduction to Research Methods in Education. Newcastle upon Tyne: SAGE.
Siniscalchi, S. M., and Lee, C.-H. (2021). “Automatic speech recognition by machines,” in The Cambridge Handbook of Phonetics, Cambridge Handbooks in Language and Linguistics, eds R. A. Knight and J. Setter (Cambridge: Cambridge University Press), 480–500. doi: 10.1017/9781108644198.020
Sperber, M., Neubig, G., Nakamura, S., and Waibel, A. (2016). “Optimizing computer-assisted transcription quality with iterative user interfaces,” in Proceedings of the Tenth International Conference on Language Resources and Evaluation (LREC'16). (PortoroŽ: European Language Resources Association), 1986–1992.
Sperber, M., Neubig, G., Niehues, J., Nakamura, S., and Waibel, A. (2017). Transcribing against time. Speech Commun. 93, 20–30. doi: 10.1016/j.specom.2017.07.006
Stoddart, J., Upton, C., and Widdowson, J. D. A. (1999). Sheffield Dialect in the 1990s: Revisiting the Concept of NORMs. Urban Voices: Accent Studies in the British Isles (London: Longman), 72–89.
Stolcke, A., and Droppo, J. (2017). Comparing Human and Machine Errors in Conversational Speech Transcription. arXiv [cs.CL]. Available online at: http://arxiv.org/abs/1708.08615
Strycharczuk, P., López-Ibáñez, M., Brown, G., and Leemann, A. (2020). General Northern English. Exploring regional variation in the North of England with machine learning. Front. Artif. Intell. 3, 48. doi: 10.3389/frai.2020.00048
Szymański, P., Zelasko, P., Morzy, M., Szymczak, A., Zyła-Hoppe, M., Banaszczak, J., et al. (2020). WER We Are and WER We Think We Are. arXiv [cs.CL]. Available online at: http://arxiv.org/abs/2010.03432
Tatman, R. (2017). “Gender and dialect bias in YouTube's automatic captions,” in Proceedings of the First ACL Workshop on Ethics in Natural Language Processing (Stroudsburg, PA: Association for Computational Linguistics), 53–59. doi: 10.18653/v1/W17-1606
Tompkinson, J., Haworth, K., and Richardson, E. (2022). “For the record: assessing force-level variation in the transcription of police-suspect interviews in England and Wales,” in Conference of the International Investigative Interviewing Research Group (Winchester).
Tschäpe, N., and Wagner, I. (2012). “Analysis of disputed utterances: a proficiency test,” in Conference of International Association for Forensic Phonetics and Acoustics, August (Santander).
Tüske, Z., Saon, G., and Kingsbury, B. (2021). On the Limit of English Conversational Speech Recognition. arXiv [cs.CL]. Available online at: http://arxiv.org/abs/2105.00982
Van Petten, C., and Kutas, M. (1991). Influences of semantic and syntactic context on open- and closed-class words. Mem. Cogn. 19, 95–112. doi: 10.3758/BF03198500
Vergyri, D., Lamel, L., and Gauvain, J.-L. (2010). Automatic Speech Recognition of Multiple Accented English Data. Available online at: www-tlp.limsi.fr; http://www-tlp.limsi.fr/public/automatic_speech_recognition_of_multiple_accented_english_data_vergyri.pdf (accessed January 20, 2023).
Walker, A. G. (1990). “Language at work in the law,” in Language in the Judicial Process, eds J. N. Levi and A. G. Walker (Boston, MA: Springer US), 203–244.
Wang, D., Wang, X., and Lv, S. (2019). An overview of end-to-end automatic speech recognition. Symmetry 11, 1018. doi: 10.3390/sym11081018
Wassink, A. B., Gansen, C., and Bartholomew, I. (2022). Uneven success: automatic speech recognition and ethnicity-related dialects. Speech Commun. 140, 50–70. doi: 10.1016/j.specom.2022.03.009
Watt, D., and Tillotson, J. (2001). A spectrographic analysis of vowel fronting in Bradford English. Engl. World-Wide 22, 269–303. doi: 10.1075/eww.22.2.05wat
Xiong, W., Droppo, J., Huang, X., Seide, F., Seltzer, M., Stolcke, A., et al. (2016). Achieving Human Parity in Conversational Speech Recognition. arXiv [cs.CL]. Available online at: http://arxiv.org/abs/1610.05256
Keywords: transcription, automatic speech recognition, forensic linguistics, automatic methods, phonetics
Citation: Harrington L (2023) Incorporating automatic speech recognition methods into the transcription of police-suspect interviews: factors affecting automatic performance. Front. Commun. 8:1165233. doi: 10.3389/fcomm.2023.1165233
Received: 13 February 2023; Accepted: 28 June 2023;
Published: 13 July 2023.
Edited by:
Kate Haworth, Aston University, United KingdomReviewed by:
James Tompkinson, Aston University, United KingdomGeorgina Brown, Lancaster University, United Kingdom
Copyright © 2023 Harrington. This is an open-access article distributed under the terms of the Creative Commons Attribution License (CC BY). The use, distribution or reproduction in other forums is permitted, provided the original author(s) and the copyright owner(s) are credited and that the original publication in this journal is cited, in accordance with accepted academic practice. No use, distribution or reproduction is permitted which does not comply with these terms.
*Correspondence: Lauren Harrington, bGF1cmVuLmhhcnJpbmd0b24mI3gwMDA0MDt5b3JrLmFjLnVr