- Department of English Studies, University of Graz, Graz, Austria
Converging towards or diverging from an interlocutor's speaking style (i.e., linguistic accommodation) has been investigated in many previous studies and is a highly relevant phenomenon in forensic authorship analysis. Accommodation has also been linked to personality traits, but there is still a lack of investigations of accommodation in computer-mediated communication. The present study thus aims at examining in how far emoji use is subject to accommodation in casual online interactions, and how reliable both emoji and emoticons are as markers of authorship. Further, this study is interested in finding out about connections between both emoji and emoticon use and the Big Five personality traits of agreeableness, emotional stability, conscientiousness, openness to experiences, and extraversion. The results of the analysis show that the frequency of emoji use is indeed strongly influenced by conversation partners, and that both emoji and emoticon use correlate particularly with extraversion and agreeableness. Despite the influence of conversation partners on emoji use, it can further be shown that emoji and emoticons remain valuable markers of authorship to different degrees.
Introduction
The introduction of emoji and emoticons has facilitated digital communication significantly (e.g., Danesi, 2016; Evans, 2017). For example, both emoji and emoticons are said to be substitutes for non-verbal features such as facial expressions and gestures, which are common in face-to-face interaction, but which digital environments are normally deprived of (e.g., Dresner and Herring, 2010; Skovolt et al., 2014; Gawne and McCulloch, 2019). The popularity of emoji in particular has been increasing tremendously ever since their introduction back in 2011 (Evans, 2017), and it has been estimated that 2–3 trillion emoji are sent in text messages every year (Goldman, 2018). Even though the nature of many emoji is ambiguous and leaves much room for misinterpretation and miscommunication (e.g., Miller et al., 2016, 2017), they provide clues to the addressee as to how the respective message is to be interpreted (e.g., Ai et al., 2017). Their increasing popularity is therefore hardly surprising.
With their first recorded appearance in the early 1980s, the emergence of emoticons pre-dates the emergence of emoji. It is an important difference between emoji and emoticons that the latter are created with regular keyboard characters, such as colons, hyphens, and parentheses such as :-), while the former are small pictorial images (e.g., ). Thus, even though long lists of pre-defined emoticons exist, there is no inherent limit to the creativity of users and new emoticons can be generated easily (Goldman, 2018). In contrast, emoji cannot be created at will, even though individuals can submit suggestions for emoji to the Unicode Consortium (Evans, 2017). According to Emojipedia (2021), more than 3,600 different emoji exist as of September 2021, and once an emoji is accepted into the existing list, it is never again deleted (Evans, 2017).
In forensic linguistic contexts, emoji and emoticons have been investigated only marginally despite their popularity and wide-spread use, but have been determined to be useful markers of authorship (Sousa Silva et al., 2011; Marko, 2020). So far, studies have investigated emoji or emoticons in isolated Twitter and Instagram messages but have not addressed them in the context of ongoing conversations between individuals. The present study therefore sets out to investigate emoji and emoticons in two important ways: firstly, it aims at examining whether or not the use of emoji is influenced by interlocutors, i.e., whether or not they are subject to accommodation. Accommodation refers to “behavioral adjustments individuals make during communication” (Bunz and Campbell, 2004, p. 13) toward or away from their interlocutors (see, e.g., Giles, 1984; Coupland and Giles, 1988; Giles et al., 1991; Gallois et al., 2005) and has received little attention in the digital environment so far (Hilte et al., 2020). Secondly, this is the first study to attempt an attribution of individual chat messages to their respective authors based solely on their use of emoji and emoticons in order to reveal how consistent and possibly individuating the use of these multimodal features is. Thus, the contributions of this study are manifold: it increases our knowledge about accommodation in digital environments while simultaneously expanding our knowledge of emoji and emoticons in computer-mediated communication, as well as their role in authorship analysis.
Literature Review
Forensic authorship analysis concerns itself with questions about unknown authorship, for example through authorship comparisons and authorship or sociolinguistic profiling (e.g., Coulthard et al., 2017). In authorship comparison tasks, texts of known and unknown authorship are compared in order to establish whether the authors of the investigated texts are the same or different (e.g., Ehrhardt, 2018, 2021); in authorship profiling tasks, texts of unknown authorship are analyzed to extract sociodemographic features of the author based on their language use (e.g., Nini, 2018a). While many studies of authorship analyses deal exclusively with non-digital texts (e.g., Mosteller and Wallace, 1964; Svartvik, 1968; Holmes, 1994; Coulthard, 2003; Nini, 2018b), modern authorship analysis is increasingly concerned with digital forms of communication (see, e.g., Layton et al., 2010; MacLeod and Grant, 2012; Grant, 2013; Wright, 2013; Johnson and Wright, 2014; Rocha et al., 2016). Digital texts such as social media posts, text messages, or instant messaging chats are not only known to be extremely short (Layton et al., 2010), but also to contain features other than traditionally linguistic ones. That is, language used in digital media is complemented by multimodal and paralinguistic features such as capital letters to indicate shouting (e.g., Zappavigna, 2013; Page et al., 2014), expressive lengthening to reflect tone of voice (McCulloch, 2019), and emoji and emoticons to convey emotions, gestures, and facial expressions (e.g., Gawne and McCulloch, 2019). While the emergence of these features has enriched digital communication (McCulloch, 2019), it has also increased the need for traditional authorship analysis methods to be adapted to new environments (MacLeod and Grant, 2012). Thus, several authors have begun to incorporate digital data into their methodologies and to test existing ones in digital environments and within the constraints imposed by some platforms, such as character limitations (e.g., Orebaugh and Allnutt, 2009; Layton et al., 2010; MacLeod and Grant, 2012; Grant, 2013; Ishihara, 2017).
Previous accommodation research has shown that speakers and writers alike adapt their speaking and writing styles to their interlocutors in a variety of different on- and offline contexts (see, e.g., Bunz and Campbell, 2004; Gnisci, 2005; Michael and Otterbacher, 2014; Muir et al., 2016, 2017; Shin and Doyle, 2018; Hilte et al., 2020). This means that speakers and writers (unintentionally) change their speaking and writing styles in order to appear more similar to their interlocutors if they want to increase solidarity with their conversational partners (convergence), or to appear less similar to achieve dissociation from their conversational partners (divergence) (Giles et al., 1991). Studies in the area of linguistic accommodation have revealed that a large variety of features, such as speech rate, pauses, utterance length, phonological variants, gaze, response latency, posture, and joking, among others, can be subject to both convergence and divergence (see, e.g., Bales, 1950; Matarazzo et al., 1968; Mauer and Tindall, 1983; Street, 1983; Aronsson et al., 1987; Watt et al., 2010). Further, it has been shown that convergence and divergence can be symmetrical or asymmetrical (i.e., one or both conversational partners accommodate their linguistic behavior), upward or downward (i.e., toward or away from a prestigious variety or dialect), full or partial, and subjective or objective, among others (Giles et al., 1991).
Research in the area of communication accommodation theory (CAT) has revealed that accommodation affects non-verbal and paralinguistic features as much as linguistic ones. Despite the general lack of studies investigating accommodation effects in online formats, a few research teams have taken on the task to consider emoji in terms of accommodation. One such study by Kroll et al. (2018), for example, has investigated emoji conversion in instant messaging. While their study only focuses on emoji accommodation in relation to social hierarchies, it does reveal accommodation effects in emoji use, showing that individuals converge toward their interlocutor's use of emoji if they perceive their conversation partners to possess a higher hierarchical status in comparison to themselves. Furthermore, Fullwood et al.'s (2013) research on convergence in the use of emoji1 in online chatrooms has found tendencies for men to converge toward women's use of emoji more so than vice versa. The present study aims at contributing to this line of research by investigating effects of accommodation on emoji in casual digital interactions regardless of hierarchy and age or gender.
Recognizing accommodation effects and possessing knowledge about which linguistic features are susceptible to accommodation can be particularly crucial in authorship analysis tasks that involve dialogic forms of texts. It can, for instance, be critical to know which features are particularly susceptible to accommodation and are thus require careful consideration and treatment in authorship comparison tasks. Accommodation effects have also been observed and investigated in terms of individuals' personality traits. For example, Muir et al. (2016) have revealed that “individuals with a particular configuration of personality traits were especially likely to deviate from the common pattern of divergence” (p. 23)—that is, individuals with high self-confidence, a distinct self-monitoring ability, an exploitative interpersonal style, low scores on agreeableness (i.e., little concern with being liked by others), and low concern for social approval were less likely to accommodate their linguistic behaviors to others. This knowledge can be useful in sociolinguistic profiling and can provide cues to the investigated author's personality.
Connections between the use of emoji and personality traits have also been found in previous studies. In 2018, for example, Li et al. (2018) studied personality traits and emoji use. Their findings indicate that the personality trait of openness to experiences is not related to emoji use, but that high scores on conscientiousness are correlated with lower use of emoji, while a high score on agreeableness is related to higher use of emoji. In their analysis, extraversion is inversely related to emoji use (i.e., highly extraverted individuals use fewer emoji). Marengo et al. (2017), in contrast, have investigated particular types of emoji and have found, for instance, that emoji are strongly related to emotional stability, extraversion, and agreeableness. For example, individuals scoring high on neuroticism (i.e., emotional stability) particularly favor emoji that convey strong emotions, while high scores on extraversion are linked to the use of positively connoted emoji. Even though the use of emoticons has also been investigated in relation to personality features, no comparable results have been obtained (Oleszkiewicz et al., 2017). This line of research has been deemed important in forensic authorship analysis, which is why the connections between emoji and emoticon use will also be investigated in this paper through the use of the Ten-Item Personality Inventory (Gosling et al., 2003), which is based on the Big Five Personality Traits (e.g., Roccas et al., 2002) and has previously been used in linguistic studies.
Based on the literature review, the following research questions were generated for this study:
(1) Is the use of emoji subject to linguistic accommodation in casual instant messaging?
(2) How does the use or lack of use of emoji influence the interlocutor's level of comfort and the perceived naturalness of the conversation?
(3) How is the use of emoji and emoticons related to features of personality?
(4) Is the use of emoji and emoticons in digital conversations distinctive enough to attribute messages to the correct author?
Methodology
The approach taken in this paper is experimental in nature. The experiments took place in the spring and fall semesters of 2021 and involved a 15–20-min online interaction between two people unknown to each other, followed by a short 5-min questionnaire created and distributed with Google Forms. In total, 46 university students (largely undergraduate) participated in this project (see Table 1 for demographic information), resulting in a total of 23 conversations (11 in the emoji condition, 12 in the non-emoji condition). The vast majority of participants (86%) are L1 German speakers, with the remaining 14% being L1 speakers of Bosnian (4.7%), Croatian (4.7%), and Slovenian (4.7%2). All participants have a command of English on at least the B2 level of the Common European Framework of Reference for Languages (CEFR) (Council of Europe, 2021), as this is the level of knowledge required to pass school-leaving exams in Austria, and the level required to study English on a university level. Even though it has been shown that emoji use is culture-specific (e.g., Guntuku et al., 2019), it has also been shown that emoji semantics are generally preserved in a variety of languages (e.g., Barbieri et al., 2016). It is thus not believed that the participants L1's or their cultural backgrounds have a strong influence on the goals of the study. Since the data is not normally distributed, Mann-Whitney-U-Tests for significant differences and Spearman for correlations were used, and the statistical analyses were computed with SPSS 26.
After signing up for participation on a voluntary basis but within the frame of a course, each student was paired with another one, and one of the students in each pair was randomly chosen as the conversation leader. The conversation leader, in contrast to the non-leader, received one of two possible sets of instructions. In Condition 1, the emoji condition, the conversation leader was asked to intersperse the interaction with as many emoji as they would perceive natural. In Condition 2, the non-emoji condition, the leaders were instructed not to use any emoji or emoticons at all. No restrictions were imposed on the topics of the interactions, but all participants talked either about hobbies and their free time, or their fields of studies and work. Thus, it was possible to keep the influence of the discussed topic on the use of emoji and emoticons to a minimum. Prior to the experiment, none of the participants received explicit information about the purpose of the study being an investigation of their use of emoji. This was done in order to prevent any biases in the conversations (e.g., O'Leary, 2014), and to keep the interactions as natural as possible in spite of the experimental setting.
The interactions took place on a university-internal platform called “Unimeet,” which the participants were asked to access with their phones. Each participant was also provided with a user ID upon registration so that they would not use their real names, since previous research has shown that knowing the other person or their gender can influence the use of emoji and other linguistic features (e.g., Turner et al., 1995; Fullwood et al., 2013; Hilte et al., 2020). In order to allow for a natural and easy use of emoji, the participants were asked to access the chatroom with their phones rather than their computers. Data from participants who did not follow one or more of the instructions (e.g., if they provided their names instead of their user IDs, or did not use their phones) was excluded from the analysis. Thus, the dataset was left with 43 participants, i.e., 21.5 chats.
The follow-up questionnaire, the link to which was sent to the participants after the conclusion of their online interactions, included ten questions with a Lickert scale that asked the participants to rate themselves in terms of the Big Five personality criteria (e.g., Roccas et al., 2002, see below). Due to the frame and focus of the study, only the short version of the Big Five Personality questionnaire, the Ten-Item Personality Inventory, or TIPI (Gosling et al., 2003), was implemented. Subsequently, the participants were asked to rate the conversations in terms of their perceived naturalness and level of comfort, and to provide some background information about themselves, such as age, gender, level of education, and ‘normal' amounts of emoji and emoticon use. Lastly, in a follow-up reflection on the task which formed a part of the course, the participants provided useful and valuable insights into their own use of emoji. Some of these comments will also be used to support the findings in the discussion section.
Big Five Personality Traits
In the field of psychology, the Big Five personality traits model is considered to be the “dominant approach for representing the human trait structure today” (Roccas et al., 2002, p. 789). The five factors included in this model are neuroticism, extraversion, openness to experience, conscientiousness, and agreeableness (see Figure 1).
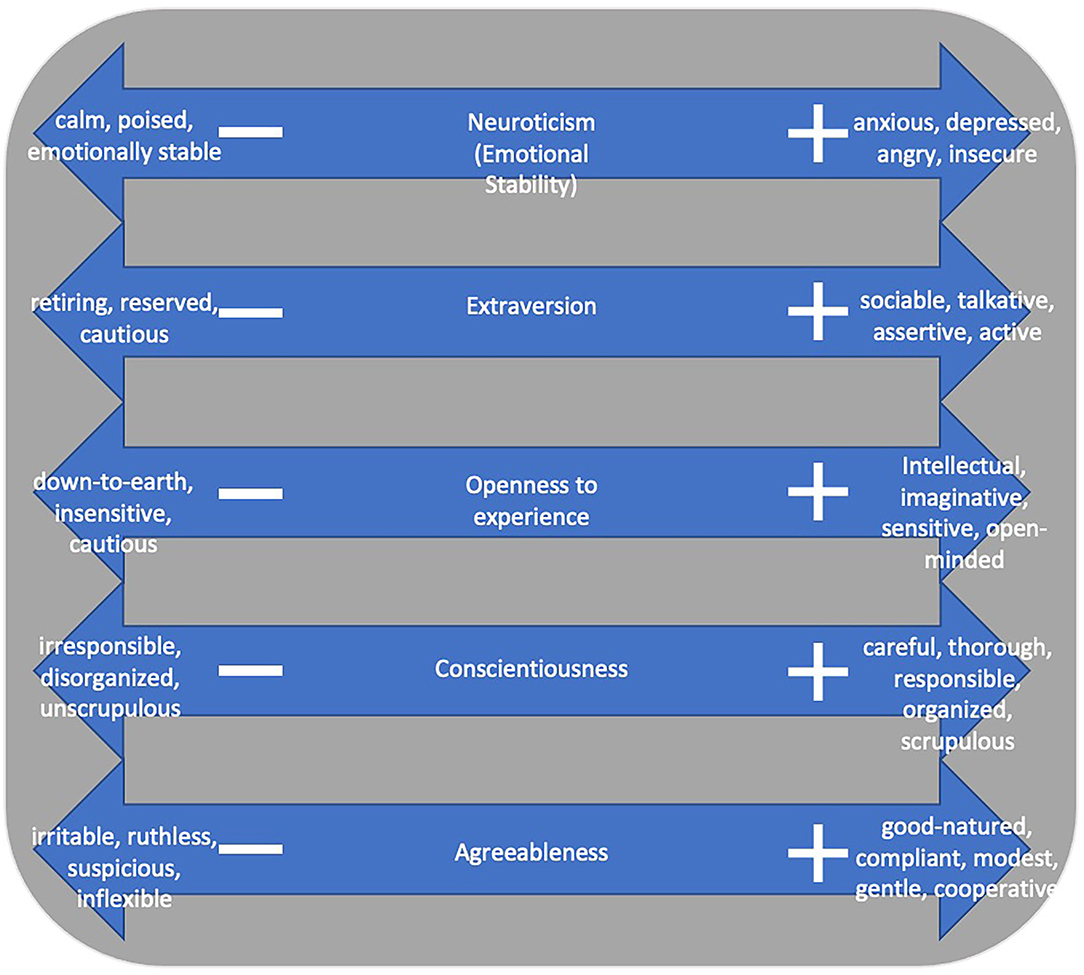
Figure 1. Model of the Big Five personality traits (based on Roccas et al., 2002, p. 792–793).
Usually, an analysis of the Big Five personality traits is rather time-consuming. Thus, Gosling et al. (2003) have devised a short version of the Big Five questionnaire, called the Ten-Item Personality Inventory (TIPI) (see Table 2). This short version is particularly useful in studies that are under time pressure, and/or studies whose main focus of investigation is not in the area of psychology. It has been used in at least one other study investigating relationships between emoji use and personality traits (e.g., Marengo et al., 2017). Gosling et al.'s (Gosling et al., 2003) own analysis has shown that despite the brevity of the questionnaire, it is sufficient to evaluate individuals' personality traits with acceptable reliability.
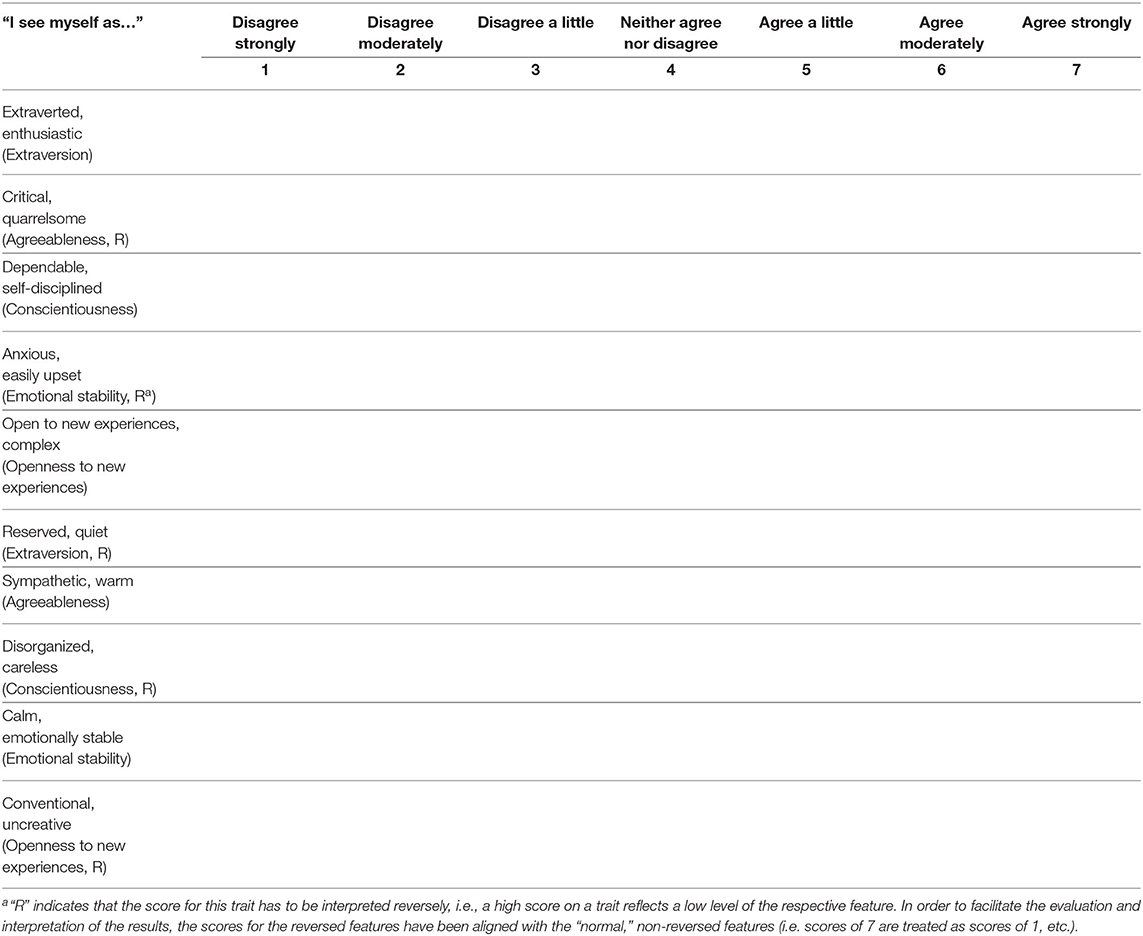
Table 2. Ten-Item Personality Inventory (adapted from Gosling et al., 2003, p. 525).
Authorship Attribution
In order to investigate whether or not emoji and emoticons remain valid markers of authorship despite outside influence through conversation partners, a mock authorship attribution task was designed. For the mock authorship attribution task, which focuses solely on the use of emoji and emoticons, the data was prepared as follows: for each non-leader in the emoji condition, the data was divided into two files with each file containing roughly the same number of turns. Only non-leaders who used four or more emoji spread across the chat protocol were deemed suitable for the analysis. Thus, the data of six individuals remained in the corpus. Table 3 shows the distribution of turns3 per individual in the dataset used for the authorship analysis. Other authorship markers were excluded from the analysis in order to evaluate the performance of emoji and emoticons in authorship tasks in isolation.
Afterwards, the used emoji and emoticons were coded by the author in MaxQDA according to their types based on their Unicode Codes (e.g., U + 1F9704, “Smiling Face with Hearts”), and, based on the classification system used in Marko (2022), the emoji were additionally coded according to their functions5 and special features of use, i.e., whether they were used in compositions (i.e., the adjacent use of different emoji), strings (i.e., the adjacent use of the same emoji), or by themselves (i.e., stand-alone). A total of 122 codes were assigned in the dataset used in the present analysis.
Finally, a similarity matrix and map based on Jaccard's Coefficient was devised in order to see how similar or different the two sections of the chat protocols by the same author are to each other based solely on the used emoji and emoticons. In detail, this means that it was the aim of this part of the analysis to probe whether parts of the same chat protocol would be more similar to each other than to parts of chat protocols of different writers. Texts that appear close to each other on the map or that receive a score close to 1 in the matrix are considered more similar than texts that appear further apart or that receive a score close to 0. Both similarity and distance-based measures have previously been tested and used in forensic linguistic authorship analyses (see, e.g., Gomaa and Fahmy, 2013; Kocher and Savoy, 2017; López-Escobedo et al., 2016), and Jaccard's Coefficient, a similarity measure, was found to be particularly useful for short texts (see, e.g., MacLeod and Grant, 2012; Grant, 2013; Johnson and Wright, 2014; Nini, 2018; Marko, 2020), which is why it was chosen for the present analysis as well.
Ethical Considerations
In order to protect the participants' identities, they were provided with individual IDs consisting of two letters (their initials) and a number (e.g. XY_001). The participants were instructed not to reveal their names or gender in the interactions to prevent biases, as was mentioned above. The chat protocols are stored only in anonymized form and are not accessible by anyone besides the researcher. The provided examples are verbatim, since the data was not taken from online platforms and reverse identification of individuals is impossible (Ayers et al., 2018). Further, the topics of the discussions (i.e., work, hobbies, studies, etc.) are not deemed particularly sensitive, and therefore no harm for the participants is to be expected (Oliver, 2010).
Analysis and Results
Before investigating the data more closely, an overview of some general findings relating to emoji and emoticon use in the investigated dataset will be given. As Table 4 shows, the use of emoji is vastly more common in the emoji condition compared to the non-emoji condition, while the use of emoticons does not seem to be influenced by the condition the participants were assigned to (p = 0.752). It has to be kept in mind, however, that the participants only received explicit instructions to use emoji but not emoticon use, which would have required a third experimental group (i.e., an emoticon condition) and would have made the experiment too complex. An experiment with this condition is thus left to future research.
Further, no significant differences were found between the emoji and non-emoji condition for both the perceived level of comfort (p = 0.412) and naturalness of the interaction (p = 0.941). However, effects of emoji use on the non-leaders' level of comfort are still visible: In the emoji condition, the level of comfort shows a moderate (yet not statistically significant) correlation of 0.486 (p = 0.130) with emoji use (see Figure 2). Due to the fact that only two emoji were used by the non-leaders in the non-emoji condition, no correlations for this groups were found. In relation to emoticon usage, it was found that while non-leaders in the emoji condition felt less comfortable the more emoticons they used (−0.375), the non-leaders in the non-emoji condition felt more comfortable the more emoticons they used (0.308).
In terms of naturalness, a slight effect of emoji and emoticon use can also be observed. In the emoji condition, the non-leaders felt the conversations to be more natural the more emoji (0.252) and emoticons (0.237) they used. The tendency in the non-emoji condition is similar yet weaker (emoji: 0.149; emoticons: 0.082).
Emoji: Accommodation Effects
As mentioned above, the difference in emoji use between the emoji and non-emoji condition is salient. The boxplots in Figure 3 illustrate the statistically significant difference of p = 0.001 in the non-leaders' use of emoji in both the emoji and non-emoji conditions. This result indicates that in the emoji condition, the non-leaders, who did not receive any instructions about their use of emoji, used a significantly larger number of emoji than the non-leaders in the non-emoji condition, in which the conversation leaders were prohibited from using emoji. These findings show that at least the frequency with which emoji are used might be highly susceptible to outside influence (i.e., factors other than personal preferences).
Further credibility for the effect of the leaders' use of emoji (or lack thereof) is provided by the self-reported frequencies6 of emoji and emoticon use by the participants, which is similar across all investigated groups. Participants were asked to rate their normal use of emoji and emoticons on a scale from 0 to 4, where 4 indicates a very frequent use and 0 indicates a lack of use. Overall, the participants rated themselves as being frequent users of emoji (mean: 3), but less frequent users of emoticons (mean: 2.4). No considerable differences emerged between the investigated sub-groups. That is, the non-leaders in the emoji conditions reported frequent use of emoji (mean: 3.4) and slightly less frequent use of emoticons (mean: 2.4). Similar values are reported by the non-leaders in the non-emoji condition (mean normal emoji use: 2.3; mean normal emoticon use: 2.9). These self-reports support the argument that the conversation leaders' behavior considerably influenced the non-leaders' behavior to use or refrain from the use of emoji in the conversation, as despite the similarities in the self-reported, “normal” emoji use, the use of emoji differs significantly depending on whether the leader of the conversation used emoji or not.
In order to illustrate how the use of emoji differed in the emoji and non-emoji condition, two examples will be given. Example (1) is taken from the interaction between FK_029, the conversation leader, and LL_030, the non-leader. In this conversation, the leader was instructed to use as many emoji as they would find natural. No changes have been made to the transcripts and errors in the transcripts are the participants' own.
Example (1): LL_030 (non-leader) & FK_029 (leader) (Emoji Condition)
[13:00] LL_030: Hello!
[13:00] FK_029: Hey
[13:01] LL_030: How are you today?
[13:01] FK_029: I'm fine. A little tired, but okay. You? :)
[13:02] LL_030: I'm good. Also a little tired from uni but that's nothing new
[13:02] FK_029: Haha yep
[13:02] FK_029: What have you been doing today? Have you already been at uni?
[13:04] LL_030: I had a lesson in the morning and after it was over, I went to Hilmteich to relax and enjoy the sunny weather. Then I went home and I've been studying until now and you?
[13:06] FK_029: Ok, sounds nice I don't have lessons on Wednesday. I've also been studying until now
[13:06] FK_029: What have you been studying?
[13:07] LL_030: No classes on Wednesdays also sounds very good
[13:07] LL_030: I've been studying Pedagogical Psychology for an exam on 8. November. And you?
[13:08] FK_029: Sounds interesting I've been studying Latin
This short excerpt of the interaction between FK_029 and LL_030 illustrates that already in the opening of the interaction, emoji are used by both interactants. As the leader of the conversation, FK_029 opens with an emoji and therefore indirectly invites their interlocutor to use emoji as well. As can be seen, almost every turn in this interaction contains at least one emoji. In contrast, consider Example (2), which is taken from a conversation in the non-emoji condition between KM_031, the leader, and MM_032, the non-leader. Next, consider Example (2), which provides insight into a prototypical interaction in the non-emoji condition. Even though the interaction is lively and vivid, neither interactant uses any emoji at all throughout the whole conversation.
Example (2): MM_032 (non-leader) & KM_031 (leader) (Non-emoji Condition)
[13:56] MM_032: Hey, how are you?
[13:56] KM_031: Hey, how are you doing?
[13:56] MM_032: Fine, thanks!
[13:56] KM_031: I'm fine thanks!
[13:57] KM_031: What are you up to today?
[13:57] MM_032: Great to hear. I do have class at a quarter past three, but otherwise not much, how about you?
[13:59] KM_031: Me too, and afterwards another, Wednesdays are my busiest uni days. Looking forward to tomorrow though, free all day to clean my new flat!
[14:00] MM_032: Oh that sucks, it's quite the opposite with me, Wednesdays are not that busy. Did you move to Graz just now, or are you moving from one flat to another?
[14:01] KM_031: From one flat to another, but I love cleaning and now I can decorate everything they way I want to!
[14:01] KM_031: So really looking forward
[14:02] MM_032: I understand, seems pretty nice! Where are you originally coming from?
[14:02] KM_031: I'll have plenty of space for all my plants and books
[14:03] MM_032: So you like to read? Me too!
[14:04] KM_031: I'm from Tyrol, but I've been living in Graz for five years now and hardly ever go home except for Christmas and special occasions
[14:04] KM_031: Nice! Do you have a favorite author? Or genre?
Below, three more examples will serve to illustrate patterns that are deviant from the two examples above and that do not fit into the line of argumentation that it is only the leader of the conversation that influences the emoji use of the interlocutor. Example (3), which is taken from a conversation in the emoji condition, shows JM_014, the non-leader, resistant to PG_013's, the leader's, use of emoji. Only in line [09:12] when the interaction shifts toward JM_014's hobbies did they use an emoji.
Example (3) PG_013 (leader) & JM_014 (non-leader) (Emoji Condition)
[09:04] JM_014: Hi
[09:05] PG_013: Hi, how are you doing today?
[09:05] JM_014: I slept pretty badly, but I'm fine. What about you?
[09:08] PG_013: I'm also a little bit tired, but nothing that a coffee can't fix do you have any big plans today?
[09:10] JM_014: Good point about the coffee, haven't had one yet. ^^ No big plans, just a mercilessly average Wednesday ahead of me, but that's alright
[09:10] JM_014: Do you have any big events ahead of you?
[09:11] PG_013: what is an average Wednesday for you?
[09:12] JM_014: A lecture, a seminar and a trip to the gym
[09:15] PG_013: tbh that sounds really average to me so you're really sporty, do you have like a favorite sport you do?
[09:17] JM_014: Not that sporty really haha, I go bouldering pretty often, used to do climbing competitively as a kid
[09:20] PG_013: that's cool, I tried bouldern once and my hands were numb the next day where do you go bouldering in graz?
Example (4) illustrates a different phenomenon: as the non-leader, BP_021 does not use any emoji throughout the whole conversation, but uses a total of six emoticons, of which the leader uses none. In this case, however, it has to be pointed out that the leader does also not use many emoji in the conversation, even though they opened the conversation with one. Therefore, they have indirectly invited their interlocutor to use emoji as well. Even though the leader's use of emoji is sparse, it is of interest to observe that the non-leader persists in their use of emoticons despite not receiving any from the leader, and further that the non-leader does not switch to the leader's use emoji at any point in the conversation.
Example (4): AS_022 (leader) & BP_021 (non-leader) (Emoji Condition)
[10:00] AS_022: Hi
[10:00] BP_021: Hi there!
[10:00] AS_022: Terrible weather isn't it?
[10:01] BP_021: No not really - right now the sun is shining. I suppose the sun isn‘t shining where you are right now ;)
[10:01] AS_022: Yes. It's very windy.
[10:02] BP_021: I haven‘t been outside today, but I can clearly see that it‘s windy outside
[10:02] AS_022: What's your favorite TV show if I may ask?
[10:03] BP_021: Well that came out ouf the blue ^^. I‘d say it is brooklyn 99. Do you know it?
[10:03] BP_021: What‘s your favorite show?
[10:03] AS_022: I have heard about it. But I wouldn't be able to tell you anything about it
[10:04] BP_021: You should really watch it sometime! It‘s reeeeally funny!
[10:04] AS_022: My favorite show would be criminal minds. I know very basic. But I do love it.
[10:05] BP_021: Ooouu! That‘s nice too! What about Bones?
[10:05] AS_022: I shall give Brooklyn 99 a try then
[10:05] AS_022: Omg I love Bones
The last example, Example (5), is taken from an interaction in the non-emoji condition. As the leader, KG_015, refrains from the use of either emoji or emoticons, but interestingly, the non-leader, DR_016, persists to intersperse the interaction with emoticons despite not receiving any back from the leader. In total, DR_016 uses 16 emoticons across the whole conversation. It can be seen that DR_016 does not give up the use of emoticons at any point, indicating their persistence and confidence in their use of emoticons.
Example (5): KG_015 (leader) & DR_016 (non-leader) (Non-emoji Condition)
[11:00] KG_015: Hello
[11:00] DR_016: I'm ready :)
[11:00] KG_015: Great, how are you today?
[11:01] DR_016: a little bit stressed.. it‘s a busy day for me
[11:01] DR_016: how are you today?
[11:01] KG_015: I‘m sorry to hear that. What are you busy with?
[11:01] KG_015: I‘m good thank you. Just finished breakfast.
[11:02] DR_016: different uni courses.. I have a tight schedule on Wednesdays
[11:02] KG_015: I totally know how you‘re feeling. Uni can be overwhelming at times.
[11:02] DR_016: oh that‘s nice :) what did you have for breakfast? I'm looking for ideas :D
[11:02] KG_015: How many classes are you taking this semester?
[11:03] KG_015: I cut up an apple, sprinkled some cinnamon on it and had some coffee, nothing too special.
[11:03] DR_016: 13 maybe.. and my internship at school
[11:03] KG_015: Woooow that‘s a lot!
[11:04] DR_016: sounds good :) bet it tasted like Christmas
[11:04] KG_015: Yes! Love me some apples with cinnamon
[11:04] KG_015: Are you looking forward to Christmas?
[11:04] DR_016: I hear that a lot :D but sometimes I feel like I'm not doing enough :(
The previously reported analyses have shown that the use of emoji by one person (in that case, the leader) influences the number of emoji used by the other person. However, as Examples (3) to (5) have shown, some non-leaders displayed a different behavior that did not fit the predicted pattern. Thus, the question arises as to which other factors, such as personality traits, have an influence on whether or not an individual adopts another person's use of emoji. For example, as reported in previous studies, extraversion and agreeableness have been found to be related to the use of emoji (e.g., Li et al., 2018; Marengo et al., 2017), and certain constellations of personality traits have been shown to have an influence on whether or not individuals converge toward their interlocutors' speaking or writing styles (e.g., Muir et al., 2016). It is therefore critical to examine whether personality traits, such as the desire for social approvement (i.e., agreeableness), or level of extraversion also influence how likely it is that a person converges toward or diverges from another person's use of emoji and whether these constellations can explain the deviant patterns observed in some non-leaders. This question will be investigated in more detail subsequently.
Emoji: Personality Effects
In order to answer the question of how personality traits influence the use of emoji, the participants' scores on the TIPI are evaluated in relation to their emoji use. Across all participants, the only trait that shows a correlation with emoji use is emotional stability (0.274). No correlation was found for any of the other features. In respect to emoticon use, however, the personality trait of agreeableness shows a similarly slight positive correlation of 0.233 (see Table 5).
Since half of the participants were explicitly instructed to use or not use emoji, it is of higher value to examine more closely only the use of emoji of those participants who did not receive instructions about neither emoji nor emoticon use (i.e., the non-leaders). As Table 5 shows, the results are stronger when the leaders are excluded: there appear to be moderately strong correlations between emoji use and agreeableness (0.507), and emotional stability (0.456), as well as slightly weaker but still moderate correlations with openness to experiences (0.363). Only the personality trait of emotional stability appears to have a moderate effect (0.305) on the frequency of emoticon use, followed by a slightly weaker effect of extraversion (0.208).
Even though the conversation leaders in the emoji condition received the explicit instruction to use emoji, they were neither told how many nor which types to use. That is, they were free in their choice of emoji in both quantity and quality. Thus, it is still valuable to investigate whether the number of emoji used correlates with personality traits, even though these results have to be interpreted more carefully for the reasons outlined above. As indicated in Table 5, it appears as if the more extraverted individuals used fewer emoji (-0.361) but more emoticons (0.303). Similarly, individuals scoring high on conscientiousness used fewer emoji (-0.276) but more emoticons (0.228), and individuals rating themselves as open to experiences used fewer emoji (−0.268) but more emoticons (0.312). Interestingly, at least for the conversation leaders, the use of emoji and emoticons show the exact opposite patterns or trends with all five investigated personality traits, indicating that emoji and emoticons appear to be used differently or for different purposes.
Emoji and Emoticons as Markers of Authorship in Informal Interactions
As outlined above, for the mock authorship analysis, the non-leaders' turns in the conversations were separated into two parts, each part containing an equal number of turns. In order to illustrate the coding process, consider the following examples (comments in brackets indicate the coding of the respective emoji):
(1) FK_029: Hey [“Smiling face with Smiling Eyes,” complementary]
(2) PG_013: tbh that sounds really average to me [“Grinning face with sweat,” complementary] so you're really sporty, do you have like a favorite sport you do?
[“snowboarder,” reinforcement]
[“woman climbing,” reinforcement]
[“woman mountainbiking,” reinforcement] [composition]
(3) EB_010: 30 min per day is [“fire,” substitution]
(4) AK_044: Have a lovely day! [“Smiling face with hearts,” reinforcement]
(5) EL_043: It's warmer than in Graz. Exept the rain. It rains 24/7 [“Face with tears of joy,” complementary] [string]
Afterwards, a similarity matrix was created with MaxQDA to compare the two parts of the same individual's text with each other in order to see whether or not it would be possible to attribute the second part of the chat to the first. Figure 4 depicts the similarity map (left) and matrix (right). It can be seen that for each non-leader, both parts of the chat cluster rather close together, indicating that their use of emoji and emoticons is similar throughout the chats, yet different from the other individuals in the dataset.
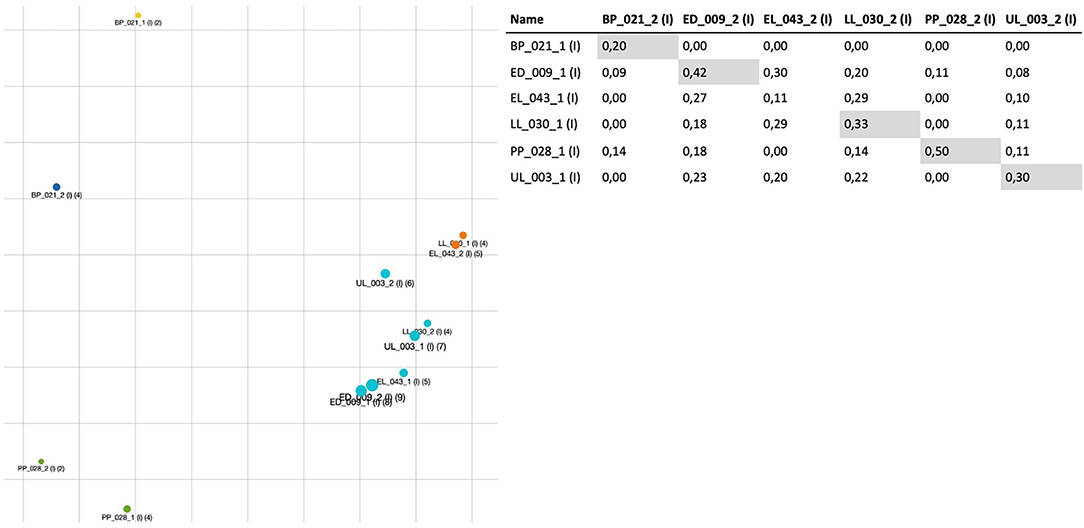
Figure 4. (Left): Similarity map based on Jaccard's Coefficient for all non-leaders in the emoji condition. The distance between the individuals' text excerpts reflects the respective degrees of similarity. (Right): The closer the result is to 1, the more similar the parts of the chats are to each other. Gray indicates “correct” attributions.
On the right hand-side of Figure 4, the results are represented numerically. It can be seen that in 83.3% of the cases (i.e., 5 out of 6 attributions), the classifications were correct. That is, the first parts of the chats of BP_021, ED_009, LL_030, PP_028, and UL_003 were classified correctly as being most similar to the same authors' second parts of the chats, or vice versa. Considering that the texts are very short and that no linguistic features other than emoji and emoticons were included, this result can be regarded as quite promising and strengthens the findings reported in Marko (2020).
In order to arrive at a more differentiated picture and to separately examine the effects of emoji types, functions, and emoticon types on the overall result of the authorship attribution, the results for these categories are separated and presented below. Initially, only the functions of emoji, as well as their specifics of use (i.e., whether they are used in strings, compositions, or as stand-alones) were considered (see Figure 5). The texts of three individuals had to be excluded due to a lack of emoji use. With a vastly reduced identification rate of only 25%, the results of the attribution based on the limited set of variables is highly unsuccessful and below the level of chance. This result clearly supports the idea that the classification system on which this analysis is based requires further improvements and adaptations (Marko, 2022).
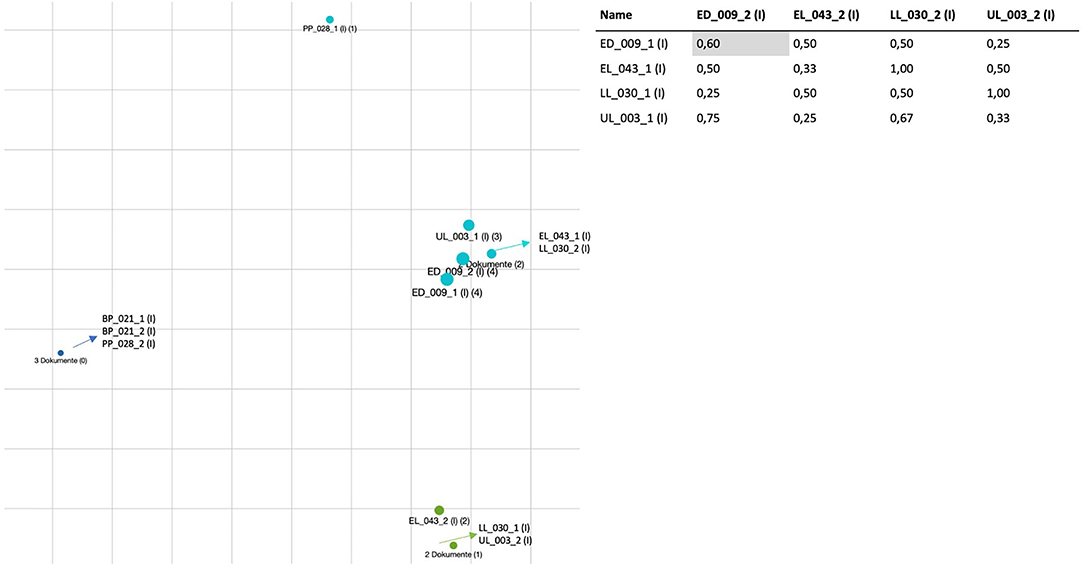
Figure 5. (Left): Similarity map based on Jaccard's Coefficient in the emoji condition, relying only on emoji functions and the specifics of use (strings, compositions, stand-alone). The distance between the individuals' text excerpts reflects the respective degrees of similarity. (Right): The closer the result is to 1, the more similar the parts of the chats are to each other. Gray indicates “correct” attributions.
In a next step, only emoji types were investigated. In this analysis, the results of correct attributions increased to 50%, as illustrated in Figure 6. Even though this result cannot be considered successful either, it has improved from the previous analysis and shows that it is worth taking emoji types as markers of authorship into account as well. In particular, it might be the use of personally preferred emoji that has an effect on this result, which is of special interest in authorship analysis. However, much more research is this area is required.
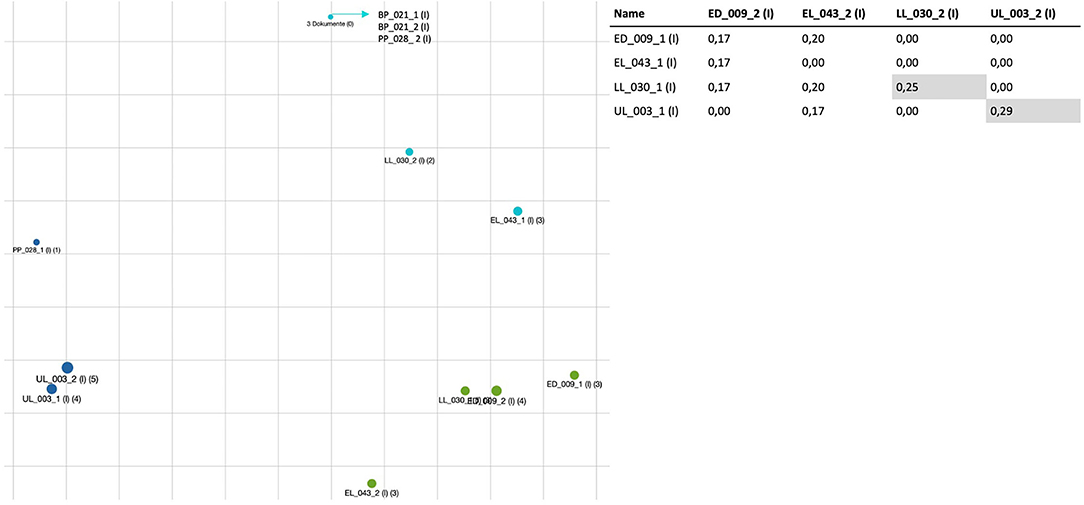
Figure 6. (Left): Similarity map based on Jaccard's Coefficient for all non-leaders in the emoji condition, based on emoji types. The distance between the individuals' text excerpts reflects the respective degrees of similarity. (Right): The closer the result is to 1, the more similar the parts of the chats are to each other. Gray indicates “correct” attributions.
Lastly, the types of emoticons were examined more closely. Only three participants consistently used emoticons, which is why only their chat protocols are considered in this part of the analysis. With this reduced dataset, the authorship attribution yielded the best overall results with a 100% correct identification rate, as shown in Figure 7. Even though the range of used emoticons was rather limited (only eight different emoticons were used in contrast to 50 different emoji), this result supports Sousa Silva et al.'s (Sousa Silva et al., 2011) findings, in whose study emoticons outperformed all other investigated measures of authorship.
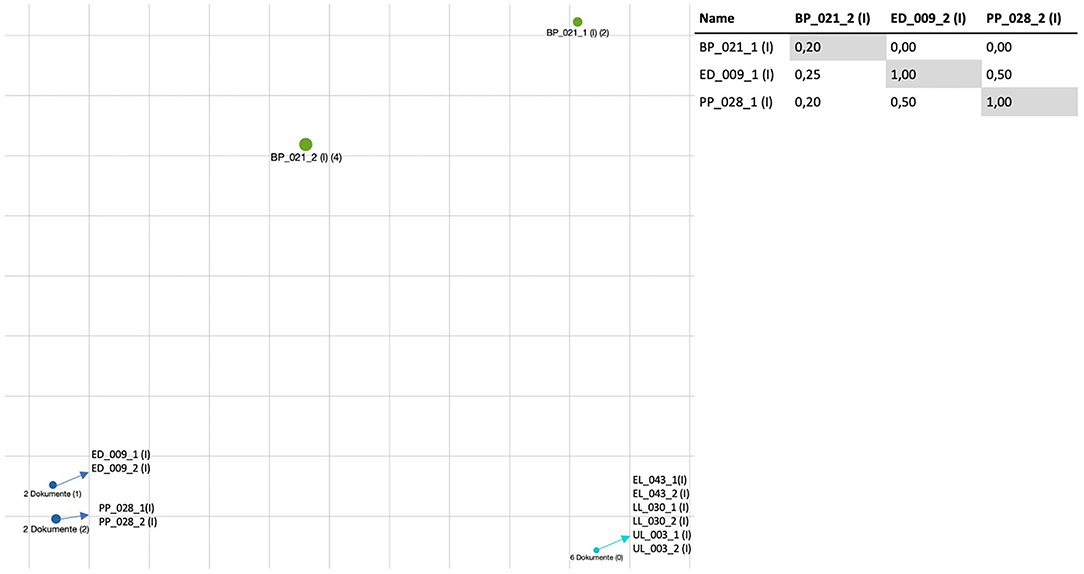
Figure 7. (Left): Similarity map based on Jaccard's Coefficient for all non-leaders in the emoji condition, emoticons only. The distance between the individuals' text excerpts reflects the respective degrees of similarity. (Right): The closer the result is to 1, the more similar the parts of the chats are to each other. Gray indicates “correct” attributions.
The above-mentioned analyses show that the use of emoticons appears to be the most individuating in the present dataset, followed by the types of emoji, and with the emoji functions performing the worst. Further research in the area of emoticon use is thus warranted and a promising avenue for future research. Additionally, it needs to be tested whether a more differentiated classification system of emoji functions can also improve authorship attribution results.
Discussion
Finally, the results of the reported experiment will be summarized and discussed in order to answer the initially proposed research questions. The first research question asked whether or not the use of emoji is subject to accommodation in casual instant messaging. The analysis presented in this paper has clearly demonstrated that, indeed, the use or lack of use of emoji by one person in the interaction influences the frequency with which the interlocutor uses emoji. However, it is yet left unclear whether the quality of emoji (i.e., the types of emoji) is also influenced by the addressee, or whether personal preferences play a more central role. This question is also critical from an authorship analysis perspective and requires in-depth examination.
In order to find out more about the participants' awareness of their own emoji use and accommodation, a follow-up reflection on the experiment was conducted several weeks after the experiment. Some participants provided valuable insights in terms of their awareness of accommodation:
PG_13: I try to adapt to the other person's writing style. Therefore, with some friends I don't use emojis in our conversations, while with others I use some of their favorite emojis/emoticons (my emphasis).
CW_034: If the person I'm writing to doesn't use emojis, I also don't use them. If the person I'm writing with uses a lot of emojis, I use a lot of them too.
MM_032: When I write with someone I don't know that well or with a stranger, I do use more emojis and also different kinds than with people I know well, because they tend to understand you and your emotions without emojis and you kind of develop a liking for emojis which are used regularly (my emphasis).
JP_002: it just really depends on the person I am talking to, the topic of the conversation and also my mood (my emphasis)
DH_001: I'm not sure I really adapt my emoji use to others, I believe that I mostly stick to my selected set of emoji and I simply select out of these based on the situation (my emphasis).
MM_036: I myself fully adapt to my conversation partner when using emojis. If they use them, I do too. If they do not use them, I stop using them.
Several interesting aspects are raised in these comments. Firstly, it appears as if for some individuals, it is a highly conscious choice to adapt to their conversation partners' emoji use, while for others, this choice is less conscious. Secondly, some comments, like MM_032's, imply a differentiation of conversation partners in terms of familiarity upon which a decision for or against emoji use is made; an issue that will be commented on again below. Additionally, it is highly interesting that, as JP_002 points out, the choice of whether or not they use emoji also depends on the topic of the conversation and their mood, while DH_001 and MM_032 provide insights into their awareness of the existence of either having their own personal preferences for specific emoji, or of other people having ‘favorite' emoji, which they adopt into their own style.
Further, the analyses suggest that there might be a difference in use between emoji and emoticons, as also touched upon in some participants' comments. No interlocutor persistently used emoji in the non-emoji condition, but several continued to use emoticons. Emoticons are inherently less prominent or visible in a text than emoji, which possibly explains why individuals are more comfortable and consistent in their use of emoticons compared to their use of emoji. Thus, it might be argued that the use of emoji is more susceptible to “leading” than emoticons. The subsequent comments made by some participants aid in the creation of a more differentiated picture about emoji and emoticon use.
PG_013: they clearly look different visually, but they also convey a different emotion, at least to me (my emphasis).
CW_034: I use emoticons when I write a quick message and don't take the time to look for a suitable emoji. However I also think emoticons are a bit more formal than emojis (my emphasis).
AS_022: I do think there is a difference between using emojis and emoticons. I feel like emoticons are more popular in the gamer community (my gamer friends primarily use emoticons. I do not adapt) while emojis are just something adapted throughout the time and used by everyone (my emphasis).
KA_038: I use them differently, if I write friends on WhatsApp, I will use a lot of emojis, but outside of WhatsApp I much prefer emoticons and will usually mainly use these (my emphasis).
DR_042: For whatever weird reason, emojis just seem “softer” and “friendlier” than emoticons (my emphasis).
LL_030: I often use emojis in informal conversations, for example when I am texting my friends and family, and emoticons for formal conversations, for example when I am texting my boss (my emphasis).
DH_001: Emojis are a bit more elaborate and expressive in my opinion, but I believe my usage of the two depends on what I'm writing on. If I'm on the phone I mainly use emojis and on desktop keyboards I mainly use emoticons because they are swifter and easier to do (my emphasis).
ED_009: I think with emojis you can say so much more than with emoticons (my emphasis).
BK_026: I can't really explain why, but emoticons seem more sincere to me, while emojis often tend to exaggerate, which I personally find a little silly (my emphasis).
As can be seen in the participants' comments, the difference in emoji and emoticon usage is rather complex. For instance, as pointed out by PG_013, DR_042, ED_009, BK_026, and DH_001, emoji and emoticons do not only have a different visual appearance but are also perceived differently in terms of which kinds of emotions they convey and in the amount of information they convey. AS_022 suggests that a group-specific preference for one over the other might exist, and CW_034, KA_038, LL_030, and DH_001 further comment on the fact that the used device influences their choice of either emoji over emoticons or vice versa, and that also the formality or informality of the conversation has an effect on their choice.
The second research question addressed the level or comfort and perceived naturalness of the interaction in connection with emoji use or lack thereof. It was shown that the level of comfort seemed to increase with an increased emoji use in the emoji condition, possibly indicating that the non-leaders felt more comfortable when their own emoji use was mirrored by their conversation partners. This is reflected in the following comment by ED_009, which also shows how well-integrated emoji have become into digital communication: “Using a lot of emojis when writing to a person who does not use emojis at all feels kind of wrong. On the other hand, not using emojis at all sometimes feels rude.” However, it can also indicate that some individuals generally feel uncomfortable when using emoji with a stranger rather than an acquaintance or friend, as is supported by the comment of one participant: CH_040: “Strangers get no emojis, I do not want to seem childish.” In contrast, other students reported that they indeed use more emoji with strangers: NS_027: “I use them more when I don't know people to make sure things come across as intended. With my closest friends I use hardly any emoji.”
In the third part of this paper, the relation between emoji and emoticon use and personality traits was investigated. As has been shown in Li et al. (2018), the present study also supports the finding that extraversion is negatively related to emoji use in all the investigated groups. Indeed, the personality trait of extraversion is the only one that shows consistent negative correlations with emoji use. Interestingly, however, in contrast to emoji use, emoticon use is positively correlated with extraversion in all groups except for the non-leaders in the emoji condition. This result lends further credence to the perception voiced by AS_022 above, who perceives that emoji and emoticons are used by different groups of people. Also, in line with Li et al.'s (2018) study, moderately strong positive correlations were revealed for the uninstructed individuals in both the emoji and non-emoji condition. For emoticon use the results are weak but also generally positive. Thus, it might be concluded that individuals with a desire for social approvement use both more emoji and emoticons. No strong or consistent results were obtained with respect to conscientiousness and either emoji or emoticon use, while the personality traits of emotional stability and openness to experience were largely found to be positively correlated with both emoji and emoticon use. Thus, to summarize, it was possible to support and replicate some findings obtained in previous studies. It is possible, however, that the effect that accommodation has on emoji use is so strong that effects of personality traits are hidden or exaggerated, which would explain some of the mixed findings. Further research is thus necessary.
The last part of the analysis aimed at investigating how distinctive the use of emoji and emoticons is in terms of authorship analysis. As the analysis for answering the first research question has revealed, the frequency with which emoji are used is considerably influenced by the addressee, which has important implications for authorship analysis of interactive texts. In contrast to Marko (2020), which has investigated the distinctiveness of emoji use in Instagram messages, the identification rate based on emoji functions is considerably reduced in the present analysis. This can be due to the fact that the data of the present analysis is more interactive and thus more highly influenced by the interlocutor and processes of accommodation compared to the non-interactive Instagram data. However, it was shown that emoji and emoticon types might still be valuable indicators of authorship in interactive texts and thus warrant further investigation. Further investigation is also warranted in terms of emoji functions, as a more differentiated system of emoji functions might lead to more successful outcomes in authorship attribution tasks. Research into emoticon use in terms of authorship, however, might be even more promising.
The present study also has several limitations. First of all, despite attempts to keep the conversation as natural as possible, it was nevertheless an experimental setting, which might have impacted the participants' use of emoji. As outlined above, some participants reported to use more emoji with strangers while others reported the exact opposite. It also needs to be acknowledged that the participants are university students who likely share many cultural, social, institutional, and even physical circumstances. Thus, the results cannot be generalized to a larger or different group of individuals at this level of research. Further, effects of upward accommodation cannot be ruled out entirely. In detail, this means that it is possible that the non-leaders accommodated to the leaders' use of emoji or lack thereof simply because they were called “leaders.” However, since all participants knew they were interacting with other students, this effect is likely to be negligible. Additionally, even if this effect is present, it still shows that the frequency with which the non-leaders used emoji was significantly influenced by the leaders' use, regardless of whether the use was triggered by upward accommodation or “simple” accommodation of emoji use. In respect to the authorship analysis, it is a limitation that the included texts are rather short and that some participants used only very few emoji and emoticons overall, which poses a particular challenge and might be accountable for the high error rate in the attribution based on emoji functions. However, texts used in forensic authorship analysis generally tend to be very short (e.g., Ehrhardt, 2021) and thus the applied methods are required to function under such extreme conditions as well. Lastly, it needs to be stated that the present study only employed the short version of the Big Five Personality Traits test, the TIPI. More in-depth psychological tests which are outside the scope of the present study might lead to more generalizable results.
Conclusions
The present study has shown that the frequency of emoji use is highly susceptible to outside influence. Future research needs to examine and evaluate in how far this effect is also observable in terms of emoji types. The study additionally implies that emoticons might be good markers of authorship, since they appear to be more robust in authorship attribution. However, future research needs to verify these findings and investigate whether they are similarly susceptible to outside influence and ‘leading'. Overall, as shown in the discussion, the use of emoji and emoticons is far more complex than expected, with their use being not only influenced by the conversation partners, by conscious choices to converge toward or diverge from another person's emoji use, by sub-conscious use of personally preferred emoji and emoticons, and by considerations of the formality of contexts and situations, involved participants, and, finally, devices and platforms.
Data Availability Statement
The original contributions presented in the study are included in the article, further inquiries can be directed to the corresponding author.
Ethics Statement
Ethical review and approval was not required for the study on human participants in accordance with the local legislation and institutional requirements. The participants voluntarily participated in the study.
Author Contributions
The author confirms being the sole contributor of this work and has approved it for publication.
Funding
The author acknowledges the financial support by the University of Graz.
Conflict of Interest
The author declares that the research was conducted in the absence of any commercial or financial relationships that could be construed as a potential conflict of interest.
Publisher's Note
All claims expressed in this article are solely those of the authors and do not necessarily represent those of their affiliated organizations, or those of the publisher, the editors and the reviewers. Any product that may be evaluated in this article, or claim that may be made by its manufacturer, is not guaranteed or endorsed by the publisher.
Acknowledgments
The author would like to thank everyone who has taken the time to participate in the study.
Footnotes
1. ^While the title suggests that the research investigates emoticons, the focus of the study is in fact on emoji.
2. ^The values not adding up to 100% is due to rounding.
3. ^A turn refers to a single contribution by one speaker/writer (Pridham, 2001; Clift, 2016).
4. ^Codes such as these are provided by the Unicode Consortium for each emoji to make them uniquely identifiable (e.g., Emojipedia, 2021).
5. ^Complementation (non-redundant meta-comments to convey an idea not encoded in the words, or used for politeness), Substitution (emoji that replace individual words), Contradiction (emoji to contradict the message), Reinforcement (redundant repetition of an idea that is encoded in the language and replicated through the emoji) (see, e.g., Zanzotto et al., 2011; Danesi, 2016; Evans, 2017; Herring and Dainas, 2017; Schneebeli, 2017; Giannoulis and Wilde, 2020; Siever, 2020).
6. ^While it is known that self-reports are frequently biased and subjective (see, e.g., Koziol and Burns, 1986; Mehta et al., 2016), such self-reports still present a valuable source of information.
References
Ai, W., Lu, X., Liu, X., Wang, N., Huang, G., and Mei, Q. (2017). “Untangling emoji popularity through semantic embeddings,” in Proceedings of the 11th International AAAI Conference on Web and Social Media (Montréal, QC), 2–11.
Aronsson, K., Jonsson, L., and Linell, P. (1987). The courtroom hearing as middle ground: speech accommodation by lawyers and defendants. J. Lang. Soc. Psychol. 6, 99–116. doi: 10.1177/0261927X8700600202
Ayers, J. W., Caputi, T. L., Nebeker, C., and Dredze, M. (2018). Don't quote me: reverse identification of research participants in social media studies. NPJ Digit. Med. 1, 1–2. doi: 10.1038/s41746-018-0036-2
Barbieri, F., Kruszewski, G., Ronzano, F., and Saggion, H. (2016). “How cosmopolitan are emojis? Exploring emojis usage and meaning over different languages with distributional semantics,” in MM '16: Proceedings of the 24th ACM international conference on Multimedia (Amsterdam), 531–535. doi: 10.1145/2964284.2967278
Bunz, U., and Campbell, S. W. (2004). Politeness accommodation in electronic mail. Commun. Res. Rep. 21, 11–25. doi: 10.1080/08824090409359963
Coulthard, M.. (2003). “The official version. Audience manipulation in police record of interviews with suspects,” in Texts and Practices. Readings in Critical Discourse Analysis, eds C. R. Caldas-Coulthard and M. Coulthard (London; New York, NY: Routledge), 166–178.
Coulthard, M., Johnson, A., and Wright, D. (2017). Introduction to Forensic Linguistics. London, England & New York, NY: Routledge.
Council of Europe (2021). Common European Framework of Reference of Languages. Available online at: https://www.coe.int/en/web/common-european-framework-reference-languages/level-descriptions (accessed December 14, 2021).
Coupland, N., and Giles, H., (eds.). (1988). Communicative accommodation: recent developments. Lang. Commun. 8, 175–327. doi: 10.1016/0271-5309(88)90015-8
Dresner, E., and Herring, S. (2010). Functions of the nonverbal in CMC: emoticons and illocutionary force. Commun. Theory 20, 249–268. doi: 10.1111/j.1468-2885.2010.01362.x
Ehrhardt, S.. (2018). “Authorship attribution analysis,” in Handbook of Communication in the Legal Sphere, eds J. Visconti and M. Rathert (Berlin: de Gruyter), 169–200. doi: 10.1515/9781614514664-010
Ehrhardt, S.. (2021). Forensic linguistics in German law enforcement. Lang. Law 8, 6–21. doi: 10.21747/21833745/lanlaw/8_1a1
Emojipedia (2021). FAQ. How Many Emoji Characters Are There? Available online at: https://emojipedia.org/faq/ (accessed December 6, 2021).
Fullwood, C., Orchard, L. J., and Floyd, S. A. (2013). Emoticon convergence in Internet chat rooms. Soc. Semiotics 23, 648–662. doi: 10.1080/10350330.2012.739000
Gallois, C., Ogay, T., and Giles, H. (2005). “Communication accommodation theory: a look back and a look ahead,” in Theorizing about communication and culture, ed W. B. Gudykunst (Thousand Oaks, CA: Sage), 121–148.
Giannoulis, E., and Wilde, L., (eds.). (2020). Emoticons, Kaomoji, and Emoji. New York, NY: Routledge. doi: 10.4324/9780429491757
Giles, H., (ed.). (1984). The Dynamics of Speech accommodations. International Journal of the Sociology of Language. Berlin: Mouton.
Giles, H., Coupland, N., and Coupland, J. (1991). “Accommodation theory: communication, context, and consequence,” in Contexts of Accommodation: Developments in Applied Sociolinguistics, eds H. Giles, J. Coupland, and N. Coupland (Cambridge: Cambridge University Press), 1–68. doi: 10.1017/CBO9780511663673.001
Gnisci, A.. (2005). Sequential strategies of accommodation: a new method in courtroom. Br. J. Soc. Psychol. 44, 621–643. doi: 10.1348/014466604X16363
Gomaa, W. H., and Fahmy, A. A. (2013). A survey of text similarity approaches. Int. J. Comput. Appl. 68, 13–18. doi: 10.5120/11638-7118
Gosling, S. D., Rentfrow, P. J., and Swann, J.r. W. B. (2003). A very brief measure of the Big-Five personality domains. J. Res. Pers. 37, 504–528. doi: 10.1016/S0092-6566(03)00046-1
Grant, T.. (2013). Txt 4n6: method, consistency, and distinctiveness in the analysis of SMS text messages. J. Law Policy 21, 467–494.
Guntuku, S. C., Li, M., Tay, L., and Ungar, L. H. (2019). “Studying cultural differences in emoji usage across the East and the West,” in Proceedings of the 13th International AAAI Conference on Web and Social Media (Münich), 226–235.
Herring, S., and Dainas, A. (2017). ““Nice picture comment!” Graphicons in Facebook comment threads,” in Proceedings of the 50th Hawaii International Conference on System Sciences (Los Alamitos, CA), 1–10. doi: 10.24251/HICSS.2017.264
Hilte, L., Vandekerckhove, R., and Daelemans, W. (2020). Linguistic accommodation in teenagers' social media writing: convergence patterns in mixed-gender conversations. J. Quant. Linguist. 1–28. doi: 10.1080/09296174.2020.1807853
Ishihara, S.. (2017). Strength of linguistic evidence: a fused forensic text comparison system. Forensic Sci. Int. 278, 148–197. doi: 10.1016/j.forsciint.2017.06.040
Johnson, A., and Wright, D. (2014). Identifying idiolect in forensic authorship attribution: an n-gram textbite approach. Lang. Law 1, 37–69.
Kocher, M., and Savoy, J. (2017). Distance measures in author profiling. Inf. Process. Manag. 53, 1103–1119. doi: 10.1016/j.ipm.2017.04.004
Koziol, S., and Burns, P. (1986). Teachers' accuracy in self-reporting about instructional practices using a focused self-report inventory. J. Educ. Res. 79, 205–209. doi: 10.1080/00220671.1986.10885678
Kroll, T., Braun, L., and Stieglitz, S. (2018). “Accomodated emoji usage: influence of hierarch on the adaptation of pictogram usage in instant messaging,” in Australasian Conference on Information Systems (Sydney), 1–11. doi: 10.5130/acis2018.dm
Layton, R., Watters, P., and Dazeley, R. (2010). “Authorship attribution for Twitter in 140 characters of less,” in 2010 Second Cybercrime and Trustworthy Computing Workshop (Ballarat, VIC), 1–8. doi: 10.1109/CTC.2010.17
Li, W., Chen, Y., Hu, T., and Luo, J. (2018). “Mining the relationship between emoji usage patterns and personality,” in Proceedings of the 12th International AAAI Conference on Web and Social Media (Palo Alto, CA), 648–651.
López-Escobedo, F., Solorzano-Soto, J., and Sierra Martínez, G. (2016). Analysis of intertextual distances using multidimensional scaling in the context of authorship attribution. J. Quant. Linguist. 23, 154–176. doi: 10.1080/09296174.2016.1142324
MacLeod, N., and Grant, T. (2012). “Whose tweet? Authorship analysis of micro-blogs and other short-form messages,” in Proceedings of the International Association of Forensic Linguists' 10th Biennial Conference (Birmingham), 210–224.
Marengo, D., Giannotta, F., and Settanni, M. (2017). Assessing personality using emoji: an exploratory study. Pers. Individ Diff. 112, 74–78. doi: 10.1016/j.paid.2017.02.037
Marko, K.. (2020). Exploring the distinctiveness of emoji use for digital authorship analysis. Lang. Law 7, 36−55. doi: 10.21747/21833745/lanlaw/7_1_2a9
Marko, K.. (2022). “You're a Rockstar *heart eyes*” – What the functions of emoji reveal about the age and gender of their users on Instagram. Language@Internet.
Matarazzo, J. D., Weins, A. N., Matarazzo, R. G., and Saslow, G. (1968). “Speech and silence behavior in clinical psychotherapy and its laboratory correlates,” in Research in psychotherapy, eds J. Schlier, H. Hunt, J. D. Matarazzo, and C. Savage (Washington, DC), 347–394. doi: 10.1037/10546-017
Mauer, R. E., and Tindall, J. H. (1983). Effects of postural congruence on client's perceptions of counselor empathy. J. Counsel. Psychol. 30, 158–163. doi: 10.1037/0022-0167.30.2.158
Mehta, D. D., Cheyne, H. A., Wehner, A., Heaton, J. T., and Hillman, R. E. (2016). Accuracy of self-reported estimates of daily voice use in adults with normal and disordered voices. Am. J. Speech Lang. Pathol. 25, 643–641. doi: 10.1044/2016_AJSLP-15-0105
Michael, L., and Otterbacher, J. (2014). “Write like I write: herding in the language of online reviews,” in Proceedings of the 8th International AAAI Conference on Weblogs and Social Medi (Palo Alto, CA), 356–366.
Miller, H., Kluver, D., Thebault-Spieker, J., Terveen, L., and Hecht, B. (2017). “Understanding emoji ambiguity in context: the role of text in emoji-related, miscommunication,” in Proceedings of the 11th International AAAI Conference on Web and Social Media ((Montréal, QC), 152–161.
Miller, H., Thebault-Spieker, J., Chang, S., Johnson, I., Terveen, L., and Hecht, B. (2016). ““Blissfully happy” or “ready to fight”: varying interpretations of emoji,” in Proceedings of the 10th AAAI Conference on Web and Social Media (Palo Alto, CA), 259–268.
Mosteller, F., and Wallace, D. L. (1964). The Federalist: Inference and Disputed Authorship. Addison-Wesley Series in Behavioral Science Quantitative Methods. Reading, MA: Addison-Wesley.
Muir, K., Joinson, A., Cotterill, R., and Dewdney, N. (2016). Characterizing the linguistic chameleon: personal and social correlates of linguistic style accommodation. Hum. Commun. Res. 42, 462–484. doi: 10.1111/hcre.12083
Muir, K., Joinson, A., Cotterill, R., and Dewdney, N. (2017). Linguistic style accommodation shapes impression formation and rapport in computer-mediated communication. J. Lang. Soc. Psychol. 36, 525–548. doi: 10.1177/0261927X17701327
Nini, A.. (2018b). An authorship analysis of the Jack the Ripper letters. Digit. Scholarsh Hum. 33, 612–636. doi: 10.1093/llc/fqx065
Oleszkiewicz, A., Karwowski, M., Pisanski, K., Sorokowski, P., Sobrado, B., and Sorokowska, A. (2017). Who uses emoticons? Data from 86 702 Facebook users. Pers. Individ. Diff. 119, 289–295. doi: 10.1016/j.paid.2017.07.034
Orebaugh, A., and Allnutt, J. (2009). Classification of instant messaging communications for forensic analysis. Int. J. Forensic Comput. Sci. 1, 22–28. doi: 10.5769/J200901002
Page, R., Barton, D., Unger, J. W., and Zappavigna, M. (2014). Researching Language and Social Media. London; New York, NY: Routledge. doi: 10.4324/9781315771786
Roccas, S., Sagiv, L., Schwarty, S., and Knafo, A. (2002). The Big Five personality factors and personal values. PSPB 28, 789–801. doi: 10.1177/0146167202289008
Rocha, A., Scheirer, W., Forstall, C., Cavalcante, T., Theophilo, A., Shen, B., et al. (2016). Authorship attribution for social media forensics. IEEE Trans. Inf. Forensics Secur. 12, 1–30. doi: 10.1109/TIFS.2016.2603960
Schneebeli, C.. (2017). “The interplay of emoji, emoticons, and verbal modalities in CMC: a case study of YouTube comments,” in VINM 2017 Visualizing (in) the Social Media (Neuchâtel), 1–15.
Shin, H., and Doyle, G. (2018). “Alignment, acceptance, and rejection of group identities in online political discourse,” in Proceedings of NAACL_HLT 2018: Student Research Workshop (New Orleans), 1–8. doi: 10.18653/v1/N18-4001
Siever, C. M.. (2020). “‘Iconographic communication' in digital media: emoji in WhatsApp, Twitter, Instagram, Facebook – from a linguistic perspective,” in Emoticons, kaomoji, and emoji, Chapter 7, eds E. Giannoulis and L. Wilde (Routledge).
Skovolt, K., Gronning, A., and Kankaanranta, A. (2014). The communicative functions of emoticons in workplace e-mails: :-). J. Comput. Mediated Commun. 19, 780–797. doi: 10.1111/jcc4.12063
Sousa Silva, R., Laboreiro, G., Sarmento, L., Grant, T., Oliveira, E., and Maia, B. (2011). “‘twazn me!!! ;(‘ Automatic authorship analysis of micro-blogging messages,” in Lecture Notes in Computer Science 6716, eds R. Munoz, A. Montoyo, and E. Métais (Berlin; Heidelberg: Springer), 161–168. doi: 10.1007/978-3-642-22327-3_16
Street, R. L. Jr.. (1983). “Noncontent speech convergence in adult – child interactions,” in Communication Yearbook 7, ed R. N. Bostrom (Beverly Hills, CA: Sage), 369–95. doi: 10.1080/23808985.1983.11678543
Svartvik, J.. (1968). The Evans Statements. A Case for Forensic Linguistics. Gothenburg: University of Gothenburg Press.
Turner, L. H., Dindia, K., and Pearson, J. (1995). An investigation of female/male verbal behaviors in same-sex and mixed-sex conversations. Commun. Rep. 8, 86–96. doi: 10.1080/08934219509367614
Watt, D., Llamas, C., and Johnson, D. E. (2010). Levels of linguistic accommodation across a national border. J. English Linguist. 38, 270–289. doi: 10.1177/0075424210373039
Wright, D.. (2013). Stylistic variation within genre conventions in the Enron email corpus: developing a text-sensitive methodology for authorship research. Int. J. Speech Lang. Law 20, 45–75. doi: 10.1558/ijsll.v20i1.45
Zanzotto, F. M., Pennacchiotti, M., and Tsioutsiouliklis, K. (2011). “Linguistic redundancy in Twitter,” in Proceedings of the Conference on Empirical Methods in Natural Language Processing (Stroudsburg, PA), 659–669.
Keywords: linguistic style accommodation, authorship, forensic linguistics, instant messaging, experiment
Citation: Marko K (2022) “Depends on Who I'm Writing To”—The Influence of Addressees and Personality Traits on the Use of Emoji and Emoticons, and Related Implications for Forensic Authorship Analysis. Front. Commun. 7:840646. doi: 10.3389/fcomm.2022.840646
Received: 21 December 2021; Accepted: 31 January 2022;
Published: 03 March 2022.
Edited by:
Juhani Järvikivi, University of Alberta, CanadaReviewed by:
Julie E. Boland, University of Michigan, United StatesKatarzyna Molek-Kozakowska, Opole University, Poland
Copyright © 2022 Marko. This is an open-access article distributed under the terms of the Creative Commons Attribution License (CC BY). The use, distribution or reproduction in other forums is permitted, provided the original author(s) and the copyright owner(s) are credited and that the original publication in this journal is cited, in accordance with accepted academic practice. No use, distribution or reproduction is permitted which does not comply with these terms.
*Correspondence: Karoline Marko, a2Fyb2xpbmUubWFya29AdW5pLWdyYXouYXQ=