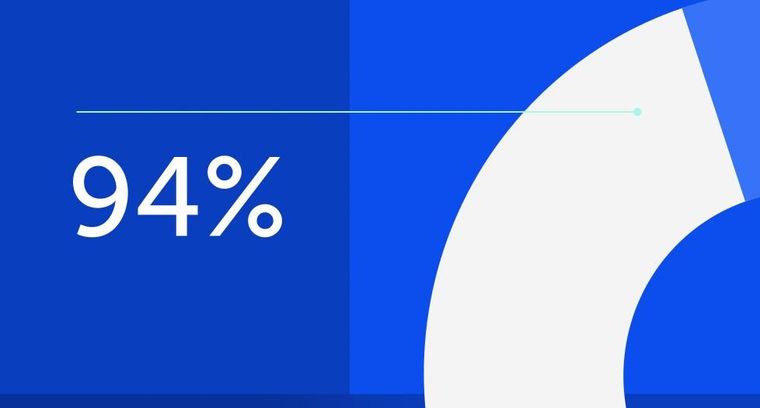
94% of researchers rate our articles as excellent or good
Learn more about the work of our research integrity team to safeguard the quality of each article we publish.
Find out more
REVIEW article
Front. Commun., 02 February 2022
Sec. Psychology of Language
Volume 7 - 2022 | https://doi.org/10.3389/fcomm.2022.792378
In this article, we wish to foster a dialogue between theory-based and classification-oriented stylometric approaches regarding deception detection. To do so, we review how cue-based and model-based stylometric systems are used to detect deceit. Baseline methods, common cues, recent methods, and field studies are presented. After reviewing how computational stylometric tools have been used for deception detection purposes, we show that the he stylometric methods and tools cannot be applied to deception detection problems on the field in their current state. We then identify important advantages and issues of stylometric tools. Advantages encompass quickness of extraction and robustness, allowing for best interviewing practices. Issues are discussed in terms of oral data transcription issues and automation bias emergence. We finally establish future research proposals: We emphasize the importance of baseline assessment and the need for transcription methods, and the concern of ethical standards regarding the applicability of stylometry for deception detection purposes in practical settings, while encouraging the cooperation between linguists, psychologists, engineers, and practitioners requiring deception detection methods.
The general public believes that it is possible to detect lying by observing nonverbal cues, yet these cues do not improve detection abilities (e.g., Bogaard et al., 2016). For these reasons, individuals who have not been trained to detect reliable cues (i.e., based on experimental evidence) typically detect lying at a rate only slightly higher than chance (i.e., 54%; see Bond and DePaulo, 2006, 2008; Hauch et al., 2017). After the meta-analysis ran by DePaulo and colleagues (2003, p. 95), an increase in the interface between language and cognition during deceit has emerged. More specifically, this meta-analysis showed that deceitful narratives differed mainly from truthful ones in terms of content, and slightly in terms of objective vocal and nonverbal cues. Therefore, the analysis of verbal lying behavior has gained interest in several domains: the forensic and legal framework (e.g., Vrij & Fisher, 2016), the insurance framework (e.g., Leal et al., 2015), or the human resources domain (e.g., Schneider et al., 2015) as meta-analyses showed that discourse analysis supported lie detection (e.g., DePaulo et al., 2003; Hauch et al., 2015; Oberlander et al., 2016). To provide an objective assessment of verbal deception, methods have been developed to provide structured analysis grids. Some of these methods have developed around research at the interface between psychology and linguistics. Common tools involve the Statement Validity Analysis (e.g., Amado et al., 2016, for a review), the Reality Monitoring Framework (e.g., Masip et al., 2005; Nahari, 2018a), or the Verifiability Approach (e.g., Nahari et al., 2014; see also Nahari, 2018a, for a review). The goal of these methods is to reduce the subjectivity of human judgments by providing objective indicators from research in cognitive science and psycholinguistics (Zuckerman et al., 1981; Strömwall et al., 2004). These methods tackling verbal deception correctly classify deceptive and authentic narratives about 70% of the time (Vrij, 2018). In this paper, we refer to verbal deception as a kind of deception that is expressed in words, thus including oral-and-transcribed deception and written deception in an interactional context. This type of deception is in contrast to non-verbal deception, which is expressed without words (e.g., through gestures such as head nods) or for which attempts at detection rely on analysis of non-verbal behavior. It is also in contrast with one-sided communication settings, such as online reviews, on which part of the literature that we will discuss here is based and provides interesting insights (see Rastogi and Mehrotra, 2017 for a review).
In 2018, several researchers and practitioners met for a 3-day workshop at Bar-Ilan University (Jérusalem, Israel), and provided an overview of the urgent issues and prospects in verbal deception detection (Nahari et al., 2019). Their goal was to answer the question “In your view, what is the most urgent, unsolved question/issue in verbal lie detection?” (p. 1). The consecutive article answering this question was structured in commentaries, each highlighting a matter that should further be investigated. Some commentaries insisted on the consideration of context and ecological validity of experimental studies (Commentaries 1, 3). Others required a better understanding and a fine-tuning of actual methods (Commentaries 2, 4, 5, 6, 10, 11). Finally, commentaries also regarded the communication between the field and research (Commentaries 7, 8, and 9). These commentaries covered the essential subjects regarding human judgements of deception and highlighted paramount issues on which researchers should focus. However, computational linguistic deception detection is barely mentioned in the publication of this 3-day workshop, while the interest for these tools and methods increases (e.g., Jupe et al., 2018; Kleinberg et al., 2018; Tomas et al., 2021c).
Similarly, in Vrij and Fisher (2016), deception detection tools are considered in regard to their potential application on the field. The article mentions various physiological methods (i.e., Control/comparison Question Test, Concealed Information Test1), nonverbal methods (i.e., Behavioral Analysis Interview2), and cognitive load methods (Imposing cognitive load, Asking unexpected questions, Encouraging the interviewees to say more, Strategic Use of Evidence3). Only one verbal discourse analysis method, the Verifiability Approach, is mentioned (Nahari et al., 2014; Vrij et al., 2016). The Verifiability Approach suggests that liars construct their narratives strategically, and thus attempt to avoid verifiable information that would potentially confound them. No other information however is provided on verbal tools, nor on the extensive literature on computational verbal deception detection published at the time (e.g., Bond and Lee, 2005; Masip et al., 2012; Fornaciari and Poesio, 2013; Hauch et al., 2015; Rubin et al., 2015). The absence of the computational linguistic approach to detect deception from current reviews in the psychology literature is conspicuous as it is currently one of the most useful set of methods and tools when wishing to understand the interface between language and cognition (Jackson et al., 2020). Still, it has been suggested that research should determine whether the coding of verbal data can be operationalized by making computers and humans collaborate to improve deception detection (Biros et al., 2004; Nahari et al., 2019; Zhang et al., 2020).
In the present article, our aim is to inform the large variety of researchers from the fields of communication sciences, psychology, engineering, linguistics about prospects and issues regarding computational approaches to (deceptive) discourse analysis. We wish to provide some considerations regarding the computational approach to verbal deception detection and how it may provide answers and insights regarding the cooperation between humans and computers. To do so, we will rely on a set of scientific literature corpora that rarely mention each other: the theory-based approach and the classification-oriented approach. W e will make use of the theory-based literature, generally proposed by psychology and psycholinguistics researchers and relying on the notion of baselining and the Undeutsch hypothesis (Amado et al., 2015), and combine it with the computational classification -oriented approach supported by computational linguists and engineers. To the best of our knowledge, the combination of both these perspectives has not been recently proposed, and should provide interesting insights and perspectives on deception detection problems. Computational stylometric (i.e., statistical stylistics) measurements and methodologies might help answer some questions often considered in human deception detection methods, such as the standardization of coding commonly encountered in deception (i.e., inter-judge rating discrepancies), or intra-individual baseline-recall comparisons, as stylometry is deep-rooted in document comparison methods.
We thus want to shed light on the prospects and issues of deception detection relying on cue-based (e.g., LIWC) and model-based (e.g., word2vec, GloVe, BERT) computational stylometry, and the technical means provided by computational stylometry to solve certain issues in the deception detection research field. In this review, we shall only discuss the verbal content analysis methods and tools developed to detect deception. In other words, the data considered in this review is either written or transcribed. This is in line with the current research led on the topic indicating that using verbal content deception detection methods might be the most reliable and valid way (Aamodt and Custer, 2006; Hauch et al., 2015; Sternglanz et al., 2019). For this reason, this paper will not concern vocal analyses of deceitful speech, nor on nonverbal cues to deception. Despite their interest in the field, these tend to be less reliable than linguistic content approaches (see Sternglanz et al., 2019 for a review).
This article is divided into three sections. In the first one, we present and define stylometry as a subfield of authorship attribution and outline how it has been adapted to deal with the deception detection problem. This section also includes the main cues and model approaches used to detect deceit, and describes the accuracy of computational stylometric analyses of verbal deception. The second section is focused on the potential applicability to the field of stylometric deception detection methods. In the last section, we detail the advantages and limits of computational stylometric tools and methods for deception detection to finally outline crucial topics for further research.
Stylometry is considered as the statistical analysis of style in textual data (Chen, 2012; Chen et al., 2011; often used as a synonym for Natural Language Processing). It should be considered as a set methodologies and tools for the analysis of linguistic data (Daelemans, 2013). It emerged from the authorship attribution domain, in which an unidentified text is compared to a set of author-attributed groups of texts in order to determine by whom it was written (Love, 2002). Stylometry originates from the literary world but has soon evolved to be concerned with social matters, such as e-mail, text, or forum posts authorship identification (e.g., Afroz et al., 2014; Fatima et al., 2017), document obfuscation (e.g., Brennan et al., 2012), political discourse analysis (e.g., Barlow, 2013), or personality assessment (Verhoeven et al., 2016). Researchers and practitioners in the stylometric domain rely on the idiolect/stylome hypothesis: Every person has its own way to speak about a particular subject, and thus to express oneself on that matter (e.g., Baayen et al., 2002). This implies that while communicating verbally, every person chooses its own words in his/her available lexicon (Hallyday et al., 1964). Although the theoretical reasons for the existence of the idiolect have never been precisely clarified, one can assume an effect of frequency of word usage: The more a word is used by a speaker, the faster it can be accessed, and therefore prevails over other equally valid terms (e.g., O'Malley and Besner, 2008).
Relying on these idiosyncratic elements, stylometry aims to determine objective (i.e., determine the protagonists, the places mentioned, the time, etc.), subjective (i.e., opinions, values, etc.), or meta-knowledge (i.e., information about the author of the text in question, leading to a psychological and sociological profile of the author) from the verbal data analyzed (Daelemans, 2013). Importantly, the term profile is here taken directly from the literature on authorship attribution. Nonetheless, while stylometrics can better identify the psychological characteristics of the author, the notion of profiling has been debated significantly for several decades, and thus should be viewed with caution (see Fox and Farrington, 2018; for a meta-analysis on the issue). To identify the idiosyncrasies of the authors and analyze linguistic data, two approaches have been used to date: cue-based approaches and model-based approaches.
The first perspective, both in terms of historicity and importance, is the cue-based approach often represented by the classical Bag of Words (BoW) set of techniques. This approach considers words as individual atomic entities independently of any context, and covers the most basic semantic information extraction procedures (Zhang et al., 2010). In BoW approaches, one relies on taxonomic structures, and may use dictionaries and ontologies (e.g., Chung and Pennebaker, 2007), function and content words (e.g., Kestemont, 2014), n-grams (e.g., De Vel et al., 2001; Hernández Fulsilier et al., 2015), part-of-speech taggers (e.g., Hitschler et al., 2018), or parsers (e.g., Chen et al., 2015) to determine what every single word taken as an entity may indicate in terms of authorship. Note that it is not simply the word itself, but also its grammatical and syntactic characteristics (Rosso and Cagnina, 2017). But a major issue with these methods concerns the absence of context consideration. This problem can be easily exemplified with the example “I am not happy”, where the unit “happy” will be considered as a word denoting positive emotions when the general sentiment of the sentence is negative. Similarly, the word “bank” has ambiguous meaning, and ontologies working in an atomic perspective will have trouble distinguishing the institution from the side of a river.
To overcome this localist problem, the second perspective (i.e., model-based approaches) was developed. In this perspective, two approaches try to account for the context in which as word is presented. The first one is generally referred to as distributional representations or word embeddings. It relies on the distributional similarity theory according to which meaning is essentially conveyed by the context in which a unit of interest can be found, and thus that words found in a similar context have similar meaning (Harris, 1954; Mikolov et al., 2013). The objective of such models is to provide language models larger than the atomic perspective discussed previously. By commonly relying on recurrent or convolutional neural networks, one is capable of predicting in a sequential fashion words on the basis of context, or the context surrounding a given word (Raaijmakers, 2022). This is done by creating vector representations (also called word embeddings) of a given word from a large corpus, indicating the probability of a word occurring on the basis of its context. To do so, one may rely on training models such as word2vec, GloVe, or fastText (Nam et al., 2020). However, word embeddings have often been criticized for their interpretability problem despite some attempts being made to impart meaning on these vectors (Goodman and Flaxman, 2017; Şenel et al., 2018).
The second approach relies on deep learning and transformer-based language models, and most specifically on BERT (Bidirectional Encoder Representations from Transformers; Devlin et al., 2019). Transformers are algorithms that encode in parallel the input as a whole and combine it with the already produced output to determine the probability of the next word. A recent review regarding the properties of BERT shows that its embeddings incorporate syntactic tree-like structures, parts of speech, and semantic knowledge (Rogers et al., 2020). As for the vector representation approach, transformer-based models require a vast amount of data, and retain this black-box issue (Rogers et al., 2020).
By relying on these methods, stylometry can focus on the extraction of cues allowing to sketch a portrait of the author’s characteristics (i.e., certain socio-demographic characteristics, a potential mood state, a possible ideology, a spatio-temporal context, etc.), or at least suppose stylistic differences between one author’s characteristics and the others’ (Kocher and Savoy, 2018). These involve the evaluation of psychological and sociological traits of the author, and how psychological states may involve information processing, and thus variation in style. For instance, stylometry showed that one’s language was modified in a modified emotional or cognitive state (e.g., cognitive load, Khawaja et al., 2009; depression, Rude et al., 2004; Tackman et al., 2019; for a review, see Chung and Pennebaker, 2007). One can thus consider that this encompasses deception, as it implies for the sender a temporary psychological and emotional state that entails verbal strategies to create a false belief in the receiver (Walczyk et al., 2014). This is commonly expressed in other words by the Undeutsch hypothesis according to which a deceptive narrative will differ in form and content from a truthful narrative (and, by extension, its baseline; Amado et al., 2015). These strategies imply a potential deviation from the idiolect/stylome/baseline. In other words, one would be able to distinguish the deceptive narrative from truthful ones because they imply different states of minds, different verbal strategies, and thus different meta-knowledge cues in the account. To do so, the stylometric analyst may rely on a variety of tools, most of which have emerged for the authorship attribution domain.
Applied stylometric analyses have primarily been deployed by forensic linguists, who empirically rely on the idiolect hypothesis (Johnson and Wright, 2017). Their objective is to evaluate stylometric indices that indicate a deviation from the idiolect. Several types of cues have been used. The most common measures for reporting deviation between one’s idiolect and the questioned text involves BoW methods, and more specifically the frequency of function words (e.g., Argamon et al., 2007; see Kestemont, 2014 for a discussion), n-grams, or sentence/syntactic group complexity (De Vel et al., 2001). Function words refer to a closed and limited category of words such as prepositions, particles, determiners. N-grams correspond to a string of given elements of length n. For example, word bigrams are chains of two words. In the sentence “I saw a blue car driving down the street”, “a car” and “a blue car” are respectively a bigram and a trigram of words. There are also character n-grams: The word “stylometry” is composed of 9 character bigrams (st, ty, yl, lo, om, me, et, tr, ry). The frequency of these bigrams is indicative of the frequent word associations of an author, and thus allows to identify lexical structures. In other words, different authors use different word conjunctions, and can therefore be identified on the basis of the most frequent conjunctions.
More recent approaches involved the model-based perspective. For instance, in Kocher and Savoy (2018), distributional methods have been applied to four different corpora (i.e., Federalist Papers, State of the Union Speeches, Glasgow Herald articles, La Stampa) alongside other cue-based methods. While in some cases, the distributional approach performed similarly or higher in terms of accuracy when compared to vector matrices analyzed via the cosine similarity coefficient or topic modeling approaches (i.e., Latent Dirichlet Allocation), results were reliably in favor of the distributional approach. Chowdhury and colleagues (2019) have also run tests to determine how the use of word embedding representations would apply to authorship attribution in Bengali. Relying on neural networks, they demonstrated that the fastText word embedding methods showed overall excellent results in authorship attribution tasks. Similar methods have been used with satisfactory results in other languages such as Polish (Grzybowski et al., 2019), English (Shrestha et al., 2017), or Bangla (Khatun et al., 2019).
A few studies have also used BERT-like algorithms to determine whether authorship identification could be improved with transformers-based models. For instance, Barlas and Stamatatos (2020) showed that by relying on BERT and other pre-trained language models (e.g., ELMo), authorship attribution in cross-context cases could significantly be improved when compared to supervised, cue-based models. Similarly, when applying transformer-based pre-trained language models to the Enron Email corpus, the Blog Authorship Attribution corpus, and the IMDb Authorship Attribution corpus, a modified version of BERT (i.e., BertAA) outperformed classical methods in authorship attribution tasks (Fabien et al., 2020).
If unsupervised methods have not, to the best of our knowledge, been applied to ongoing forensic problems, certain BoW methods have been applied to the judicial context. Case studies applying forensic authorship attribution methods are rarely academically reviewed and published. However, certain studies indicate how these methods could be applied in a legal context. For instance, Juola (2012) investigated the texts of a journalist in search of asylum in the US. The latter hoped not to have to go back to his home country which policy he allegedly criticized anonymously in his work. Some texts being handwritten were identified as his own (i.e., the established documents), whereas some remained to be proven as his (i.e., the questioned documents). As the alleged author of the articles was the only testable source, the attribution task became rather a verification task: In this case, one source of alleged documents is compared to only one source of verified documents. Juola (2012) first evaluated with two different methods the similarity of trigrams (i.e., three-words strings) between the established document, the questioned documents, and distractor documents that he introduced. He found closer similarity between the established documents and the questioned documents than with the distractors. The results suggested that the author’s claims were correct, and that the texts were indeed his.
These methods and tools began recently to expand in the field of deception detection. In the next part, we shall explain the use of stylometry for deception detection. More precisely, we shall describe how the computational stylometry allow to reach similar-to-better discrimination rates than human judgement methods. We shall also discuss the benefits and disadvantages of such methods, in order to provide future research perspectives.
Most studies relying on computational stylometry to detect deceit based their analyses on BoW and lexical-based approaches (e.g., frequency of specific word categories). For instance, Newman et al. (2003) relied on the Linguistic Inquiry and Word Count (LIWC) software and 29 verbal cues (e.g., word count, pronouns, positive, and negative emotion-related words), and showed it was possible to distinguish true from false narratives at a rate of 67%. They planted the seed for a new interest in verbal deception detection, as many of the following studies later explored narratives using word categories frequency to detect deceit (Bond and Lee, 2005; Dzindolet and Pierce, 2005; Ali and Levine, 2008).
A meta-analysis showed that specific stylometric cues varied as a function of an honesty factor (Hauch et al., 2015). For example, it appears that liars use fewer words, display less vocabulary diversity, build less complex sentences, and express events in less detail. One of the specificities of this meta-analysis was its fit with previously established theories (e.g., Reality Memory paradigm), and the analytical grids supporting human judgment methods. This allowed the authors to show that the results obtained by computational stylometry echoed the results obtained by human coding methods. As a matter of fact, computational stylometric analysis has shown that liars produce less detailed narratives, or more linguistic signs of cognitive load (i.e., less linguistic diversity, fewer words) than truthful people (Hauch et al., 2015, 2017), similar to what non-computational studies had also shown (e.g., Masip et al., 2005; Nahari, 2018b; Nortje and Tredoux, 2019). This cross-validation seems to imply that stylometry is a promising tool for extracting cues and, by extension, detecting deception.
Furthermore, the extraction of deceptive stylometric cues is independent of the modality (i.e., communication channel) used to communicate the deceptive narrative. Indeed, Qin et al. (2005) studied how modality (i.e., face-to-face, auditory conferencing, or text chat) influenced the emergence of verbal deception cues. Twenty-one cues were selected to illustrate concepts such as quantity (e.g., amount of words), complexity (e.g., sentence length), uncertainty (e.g., modal verbs), nonimmediacy (e.g., passive voice), diversity (e.g., lexical diversity), specificity (e.g., spatio-temporal details), and affect (e.g., pleasantness). These cues were extracted computationally. The analysis revealed that the modality used to communicate had little effect on the analysis of the deceptive narratives, suggesting that the extraction of computational stylometric cues seems applicable to any communication modality. This could explain the similarity in the classification results of deceptive written statements between computerized stylometric means and the use of manual discourse analysis grids (e.g., Masip et al., 2012; Almela et al., 2013).
But could more recent approaches than the BoW be used for word-based deception detection accuracy? Compared to the rest of the literature and because of their recent development, a limited amount of studies have analyzed deceptive language by relying on word embeddings and language models. To the best of our knowledge, only a few published studies have focused on the vector representation approaches of verbal deceptive and sincere narratives (e.g., Pérez-Rosas et al., 2017; Nam et al., 2020). Nam and colleagues (2020) covered all three common methods in vector representation by relying on word2vec, GloVe, and fastText (Nam et al., 2020). Similarly, the use of transformers being very recent, only a few studies have relied on them to detect deceit (e.g., Barsever et al., 2020; Raj & Meel, 2021).
Studies using LIWC generally seem to average 70% of correct classifications (e.g., Bond and Lee, 2005; Masip et al., 2012; Fornaciari and Poesio, 2013; Litvinova et al., 2017). This accuracy is particularly interesting as it includes field data and experimental data. If experimental results compose most of these studies (e.g., Masip et al., 2012; Tomas et al., 2021c), other studies have focused on real-life data and shown similar results (Fornaciari and Poesio, 2013; Pérez-Rosas et al., 2015a, 2015b). For instance, an 84% accuracy rate in detecting misleading opinions was reached in a study using lexical (e.g., vocabulary richness) and syntactic (e.g., punctuation) stylometric features, as well as supervised learning methods (i.e., classification by algorithmic methods based on texts labeled as authentic or misleading, Shojaee et al., 2013). Computational stylometry applied to deception has also been used in real-world legal cases. In addition to the aforementioned qualitative study by Juola (2012), Fornaciari and Poesio (2013) used LIWC and other stylometric methods to quantitatively analyze a corpus of Italian court hearings and determine whether a lie could be detected in them. This legal corpus entitled DeCour contains statements made in court hearings labelled a posteriori as true, false, and uncertain. The accuracy of distinguishing between different kinds of statements reached 70%, a classification rate similar to methods for analyzing statement validity or the Reality Memory Paradigm (Masip et al., 2005; Nahari, 2018b). This accuracy rate has been replicated in other studies focusing on real-life cases (e.g., Pérez-Rosas et al., 2015a). By relying solely on BoW approaches, stylometry appears to be as effective on real data than on experimental data, and at the same accuracy level as common human judgment methods used to detect lying (Vrij, 2018).
Beyond these BoW approaches, vector representations and pre-trained transformer-based architectures have also tackled the deception detection problem. Pérez-Rosas and colleagues (2017) focused on identity deception and showed that relying on word2vec lead to interesting classification rates (i.e., 77.51%), and an increase of accuracy of more than 9 points compared to LIWC (63.28%). However, the best approach in this study still was n-grams, with an accuracy of 86.59%. A more recent study re-analysed the data collected by Ott and colleagues (2011, 2012) with word2vec, GloVe, and fastText in the pre-training phase (Nam et al., 2020). Results from their analysis showed that, independently of the neural networks used for classification purposes, accuracy was above 80%. The same data was also analyzed by relying on transformer-based analyzes and reached a staggering 93.6% accuracy rate (Barsever et al., 2020), suggesting that more recent approaches to stylometry and natural language processing provide effective ways to separate truthful reviews from deceitful ones. However, when considering interactional deception, a recent evaluation of BERT-based models show much heterogeneity in the way these models are structured (Fornaciari et al., 2021).
Stylometry would therefore allow the detection of deception in similar-to-higher proportions to methods based on manual discourse analysis. It also surpasses manual processing in terms of speed of execution, reliability and reproducibility of results while suggesting minor drawbacks. These will be developed in a later section of this article, since it is first necessary to determine whether stylometry could potentially be applied to concrete and applied cases. As mentioned above, certain studies have relied on existing material to analyze authorship attribution (e.g., Juola, 2012) or deception (Fornaciari and Poesio, 2013), but no criteria have been assessed to determine whether these methods were sufficiently reliable and transparent to be applied on the field and used, for instance, in a court of law. We thus propose an analysis of the computational approach to deception detection on the basis of previous work regarding manual methods. Manual methods have been analyzed to determine their applicability, and by extension their quality in terms of expertise on concrete cases (Vrij & Fisher, 2016). We therefore propose to subject the stylometric method to the evaluation proposed for other methods.
To determine whether stylometry can be used in the field to reliably detect lying, we propose using a list of criteria developed by Vrij and Fisher (2016). Specifically, this list of criteria assesses 1) whether the items included in the previously presented methods were included as a result of a scientific approach and 2) whether their accuracy is supported by scientific studies.
To this end, this list extends the criteria of the Daubert case used as a benchmark for examining the scientific validity of evidence presented in court (Larreau, 2017). It includes 10 criteria in its final version (five criteria from Daubert and five criteria from Vrij and Fisher, 2016; see Table 1). The first five are used to determine whether evidence presented in court is scientifically admissible, and the last five are specific to lie detection.
TABLE 1. Evaluation of the applicability of stylometric methods to detect deception on the field (based on Vrij & Fisher, 2016).
To the first three criteria (i.e., “Is the scientific hypothesis testable?”, “Has the proposition been tested?”, and “Has the technique been peer-reviewed and published?”), the answer is yes for all of the stylometric methods included in this article. Indeed, lie detection from stylometric cues is a testable hypothesis: It is possible to determine, through human or algorithmic classification methods, whether the use of computational stylometry detects deception or not, in accordance with the falsifiability principle (Popper, 1959). Second, computational stylometry has been tested for its ability to detect deception with various tools and methods (e.g., for LIWC, see Ali and Levine, 2008; Fornaciari and Poesio, 2013; Newman et al., 2003; Tomas et al., 2021c; for named entity recognition, see Kleinberg et al., 2018; for morpho-syntactic labeling, see Banerjee and Chua, 2014; for n-grams, see Cagnina and Rosso, 2017; Hernández Fulsilier et al., 2015; Ott et al., 2013; for vector representations, see Nam et al., 2020; for BERT, see Barsever et al., 2020). Third, it has been the subject of over 20 peer-reviewed publications (e.g., Hauch et al., 2015; Forsyth and Anglim, 2020; Tomas et al., 2021a).
The fourth criterion from the Daubert list, regarding the known error rate of deception detection with stylometric cues, is complex. As we mentioned earlier, the accuracy rate seems to be at least as high as with the SVA or the RM methods for cue-based methods, and higher for model-based methods (see Table 1 for estimated error rates). However, to date, there is no systematic review providing an overview of the hits, misses, false alarms, and correct rejections when trying to classify deceptive narratives with stylometric cues. We thus argue that this criterion is to date not fulfilled because of the absence of general systematic review allowing for correct error rate assessment.
The fifth criterion questions whether the theory upon which the technique is based is globally supported by the scholar community. No clear definition has been given of what constitutes “acceptance in the scientific community”. Vrij and Fisher (2016) define this criterion as the amount of criticism the method has been subjected to. For instance, a lot of criticism has been uttered regarding the use of physiological cues in a polygraph context (e.g., Han, 2016; Vrij et al., 2016). If one considers the field of stylometry in a global perspective, including authorship analysis, there have been some critiques regarding the use of stylometry in court (Clark, 2011). However, while these critiques were accurate, they did not concern computational stylometry as an extraction procedure, but unfounded handmade stylometry, and its potential use of black box machine-learning algorithms for classification (Nortje and Tredoux, 2019). Computational stylometry as an extraction method has evidence-based background, robustness, and results that contradict these criticisms. Moreover, compared to the global literature regarding the stylometric assessment of authorship, the criticism is scarce. There seems to be little doubt regarding the global acceptance of its techniques by the scientific community (see Holmes, 1998, for a review). If one considers the field of forensic linguistics alone, the same global acceptance by the scientific community may be observed (Woolls, 2010). Moreover, the use of computational stylometric cues to detect deceit relies on various theoretical hypotheses commonly accepted by the community, such as the Reality Monitoring framework (e.g., Bond and Lee, 2005), or the Interpersonal Deception Theory combined with the Self-Presentation Perspective (e.g., Hancock et al., 2004). And as highlighted previously, the rationale behind stylometry is the one of the idiolect-baseline hypothesis, which has been supported by numerous studies (e.g., Barlow, 2013; Daelemans, 2013; Johnson and Wright, 2017; Kestemont, 2014; Stoop and van den Bosch, 2014; van Halteren et al., 2005; Wright, 2017). We thus argue that the theories underlying the use of the stylometric methodologies have generally been accepted by the scientific community.
Stylometry also ticks the box for the criteria 6–10. Indeed, it is easy to incorporate in a typical information-gathering setting (Criterion 6) as, up to now, it can only be used after the interviewing phase because of the transcription. This transcription phase is common to all current scientific methods. It tends more and more regularly to be part of the procedure in the case of audiovisual recordings for, for example, hearings of minors (e.g., art. 706-52 of the French code of criminal procedure, art. 4 of the ordinance n° 45-174 on delinquent children in France, articles 92-97 of the Belgian code of criminal instruction, art. 154, §4, let. d. of the Swiss code of criminal procedure) or adults (e.g., suspects in criminal cases, according to art. 715.01-715.2 of the Canadian Criminal Code; art. 64-1 of the French Code of Criminal Procedure; polygraphic credibility assessment according to art. 112ter, §1 of the Belgian Code of Criminal Procedure; Achieving Best Evidence in Criminal Proceedings, N8). Thanks to these transcripts, the use of stylometry during a second part of the hearing procedure allows the investigator to remain focused on the report writing phase and on gathering information during the interview.
Moreover, the use of post-interviewing stylometry will not affect the answer of a truthful interviewee (Criterion 7), and is easy to use (Criterion 8). Regarding Criterion 9, we argue that the automated extraction of stylometric cues protects the truth teller more than the use of any verbal deception detection grid, as the extraction itself is as free of biases as can be. Its analysis, however, may be questioned if one relies on deep learning methods, as they often lack transparency. Finally, regarding counter-measurements (Criterion 10), little is known in the public domain regarding stylometry for deception detection, leaving little room for counter-measurements.
If we follow the arguments developed by Vrij and Fisher (2016), stylometry should be allowed in the field when it comes to deception detection. However, there are a few issues that we wish to highlight to nuance this perspective. T hese criteria, although interesting in terms of scientific value, tend to avoid the main ethical concern behind deception detection, which is its accuracy. Evidently, scientific methods developed to tackle the detection of deceit provide better results than pure reliance on experience and intuition (DePaulo et al., 2003; Hauch et al., 2015; but see Sporer and Ulatowska, 2021 and Stel et al., 2020 for recent developments). Nevertheless, the performance and accuracy of these methods remains practically too dangerous to be put into practice, especially in risky situations such as employment, judicial events, or police interviews. Even in cases where impressive accuracy rates are reached (e.g., 93.6% in Nam et al., 2020), the chances to wrongly blame the sincere remain too high.
Despite this final argument regarding the current impossibility to rely on stylometry to detect deception in a definitie fashion, we still wish to develop the various advantages and caveats that set apart the stylometric way to detect deception from human methods in the next section.
As summarized in Table 2, computational stylometry has a few advantages and caveats when compared to human judgment methods to detect deceit.
In terms of advantages, computational stylometry is defined by its independence from human judgment in coding and extracting cues. In other words, the issue of inter-coder agreement is eliminated here, as researchers using the same software for the same data will extract the same indices. Stylometry thus reduces differences in the collective or collaborative extraction of cues4.
This lack of agreement among coders in data extraction has been raised in multiple studies regarding deceptive discourse analysis, as some criteria in grids proposing manual discourse analysis have ambiguous and poorly articulated definitions (e.g., Vrij et al., 2000; Amado et al., 2016). This robustness in extracting data from stylometry is essential in the context of the so-called reproducibility crisis in psychology (Munafó et al., 2017). Computational stylometry and its automation allow us to take another step on the still very long road to fully reproducible protocols. Thus, it provides a solution to the goals and needs of institutions for valid and reliable ways to detect lying (Nahari et al., 2019).
Automated stylometry also relies on the power of computers, which ensure rapid processing of the collected and transcribed data. Currently, the majority of verbal deception detection methods validated by the scientific literature rely on a transcription phase. This step is currently difficult to avoid, although some methods attempt to provide interesting solutions (e.g., Blandon-Gitlin et al., 2005; Burns and Moffitt, 2014; Masip et al., 2012; Sporer, 1997; see also the section discussing the transcription problem within the limitations of stylometry). Despite this common transcription problem, computational stylometry provides tools to process an impressive amount of data in seconds. This saves a significant amount of time in the coding process compared to manual discourse analysis. This speed of extraction is essential when searching for deception cues, and for the potential operationalization of stylometry: The faster these clues are acquired, the longer they can be scrutinized and thus serve as a basis for the elaboration of a second interview designed to test the existence of the deception.
This change of pace in the deception detection procedure implies a paradigm shift from the “multiple purposes” of interviews (i.e., information gathering, credibility assessment, maintaining rapport with the suspect; Nahari et al., 2019, p. 2), whether conducted in a legal, insurance, or hiring setting. Indeed, because computational stylometry is fast and robust, detection of potentially deceptive elements could be done quickly after the interview, based on a transcript. This is consistent with the notion of separation of multiple objectives, with the interview serving as an unconditional acquisition of information, and credibility assessment occurring afterwards (Nahari et al., 2019). We argue that this separation of purposes distinguishes between objective fact-finding during the interview, and deception-finding afterwards. Stylometry, exactly like statement validity and reality checking methods (e.g., VAS, MR), can therefore help anyone who wishes to focus on applying best practices by freeing them from the cognitive constraints inherent in deception detection, allowing them to focus on establishing report quality and using active listening techniques (Home Office and Department of Health, 2002). It thus allows the deception search to be delayed until after the interview, while providing faster results than current human judgment methods.
Computational stylometry does not appear to have a negative impact on deception detection accuracy. Although no meta-analysis shows an increase or decrease in detection rates, studies have repeatedly shown interesting results (Newman et al., 2003; Bond and Lee, 2005; Fuller et al., 2009; Mihalcea and Strapparava, 2009; Masip et al., 2012; Fornaciari and Poesio, 2013; Litvinova et al., 2017). Computational stylometry for deception detection achieves correct classification results around 70%, similar to other manual methods (Vrij et al., 2016, 2017), but with increased speed and ease of execution.
Thus, the main contribution of computational stylometry for detecting deception is its ease of implementation and speed of execution, coupled with increased objectivity of its method (see next section for a limitation to this objectivity). However, it is not without its critics.
Faced with a growing number of tools and methods for conducting cue-based stylometric analysis, researchers tend to use a smaller number of tools. Indeed, numerous tools and cues from the field of author attribution have been developed to compare texts to one another (Koppel et al., 2009; Stamatatos, 2009). This diversity of cues has given many ways for scientists to tackle a similarity problem (see Reddy et al., 2016 for a exhaustive list of indicator categories). However, with the quantity of proposed indicators, it is often complex to decide whether a stylometric indicator is suitable for a deception detection task.
As a result, according to a meta-analysis based on studies relying on a cue-based approach, half of the studies examining the language of liars with a computer rely on the LIWC and take a frequentist, categorical, and lexical perspective (Hauch et al., 2015). This over-representation of LIWC can be explained in several ways: 1) its ease of use, 2) its power in processing textual data, 3) the interest of the analyzed cues, and 4) the fact that it is a general-purpose tool that can be applied to any domain, including deception detection, while reaching interesting accuracy rates. Additionally, the first study to our knowledge that used stylometric methods to detect deception relied on LIWC, thus setting a precedent on which subsequent studies have relied (Newman et al., 2003).
However, it is important to avoid methodological confirmation bias and to turn away from current methods to explore others. We therefore recommend continuing research to increase the number of indices analyzed in order to ultimately retain only the most efficient methods or protocols (i.e., maximizing the correct classification of truthful vs. deceptive speech, and minimizing false positives and negatives). For example, interesting results have recently been observed when analyzing texts with named entity recognition (i.e., tagging and extracting various named entities, such as location, people’s names, numbers; see Kleinberg et al., 2018) or surface syntactic analysis (i.e., based on the detailed linguistic structure of a sentence and how its parts are related to each other; see Feng et al., 2012; Fornaciari et al., 2020). Stylometry could still benefit from further evaluation of other measures that potentially achieve higher accuracy in deception detection.
Another previously mentioned obstacle to the use of stylometric tools - whether automated or manual - is the transcription of data. Computational stylometry originated in the literary domain and was therefore developed to analyze written data. However, to date, most LIWC studies have relied on the transcription of oral data. This transcription from oral to written is therefore unavoidable, and it has two implications that slow down its application in the field. First, transcribing oral data takes time. In judicial, insurance, or hiring contexts, time is of the essence. Research and engineering must therefore propose methods and tools that facilitate the conversion of oral data into written format so that it is no longer an obstacle to its implementation in the field. Secondly, transcriptions must be accompanied by guidelines and produced according to consensual rules that scientists and users must respect5. For example, it might be interesting to code responses according to previously stated questions, as these can provide interesting insights into the expected length of the response (Dodier and Denault, 2018; Walsh and Bull, 2015; for an example of transcription guidelines, see; Bailey, 2008).
To our knowledge, no standardized method has been consistently applied to transcribe oral data for verbal deception detection. For example, should disfluencies (i.e., full and silent pauses, false starts, and stutters) be transcribed? If so, it is essential to consider how to transcribe them, as these features have been shown to have cognitive and interactional significance (e.g., Reed, 2000; Merlo and Mansur, 2004; Erman, 2007). One solution to this issue would be to delegate the transcription task to a (supervised) automated speech recognition software. Recent developments have shown that these systems provided efficient means to transcribe oral to written data, including pauses (Forman et al., 2017; Hagani et al., 2018; Stolcke and Droppo, 2017) and punctuation (e.g., Alam et al., 2020). This would, again, provide standardized methods that would imply an enhanced reliability in the transcription of the data, and thus in the detection of deception.
A final constraint of computational stylometry is the apparent “perfection” of automation relative to human performance, which is found in the scientific literature under the name the automation bias (for a review, see Goddard et al., 2011). Automation can take different forms, from a fully automated process with no human intervention (coding or decision), to minimal human supervision of the process (Cummings, 2004). In the case of stylometry or other verbal deception detection methods, scientific work uses the minimal stage of automation (i.e., automatic extraction of cues providing information to support human decisions). But even in this case, the automation bias may be present.
The source of automation bias results from over-reliance on automated decision making or decision support systems (Skitka et al., 2000). It is related to the principle of least resistance: In order to reduce information overload and cognitive overload, decision makers tend to adopt various strategies such as using immediately accessible information thus leading to overconfidence in automated signals (Shah and Oppenheimer, 2009).
This over-reliance on automation can lead to two types of errors: errors of omission and errors of commission. Errors of omission occur when, in the absence of problematic signals from an automation system, the user is led to believe that everything is working as intended. This is also referred to as automation-induced complacency, where automation can induce complacency, boredom, and lack of controls (Mosier and Skitka, 2018). This complacency is a pernicious problem in automated situations such as aviation safety or nuclear power plants, where the lack of attention to a visible change can be fatal. In deception detection, this error of omission can be conceived as the absence of deceptive signals from the stylometric tool leading to the inference of honesty of the listener.
Commission error, on the other hand, arises when the cues and decision suggestions from the automatic system steer the final decision toward an erroneous choice, while other indicators distinctly point to the erroneous nature of that choice. For example, a NASA study found that automated checklists resulted in more crew errors during a flight simulation. Indeed, by relying on the automatic system indicating the need for engine shutdown, pilots made a fatal error (e.g., shutting down the engines), when other directly observable indicators suggested that shutting down those engines was an unsafe procedure (Mosier et al., 1992).
Errors of commission may be the consequence of two different cognitive mechanisms: blinding or information rejection bias. The former corresponds to the absence of verification (concordant or discordant) with the information issued by the automatic system, and the latter to the disregard or discrediting of information (see Mosier et al., 1996, for a discussion on human decision and automated decision aids). In automated deception detection, the latter would correspond to a perceived mismatch between conflicting signals where, for example, stylometric signals indicate a deceptive account and a concordant body of evidence shows its honesty. The information rejection bias would be present if, in this case, the person is considered deceptive because the machine indicates it as such (e.g., Kleinberg and Verschuere, 2021).
Yet, despite our knowledge of these errors of omission and commission, it is often wrongly argued that the human-in-the-loop method is probably, to date, the most effective method for working on automation (e.g., Li et al., 2014; Strand et al., 2014; Nahari et al., 2019). Yet, as noted, whether at the level of retrieval or decision making on the basis of automatically analyzed data, human intervention in the retrieval process can lead to human errors. Indeed, a recent study suggests that human intervention for the purpose of correcting the classification of a story as deception or authentic decreases the correct detection rate (Kleinberg and Verschuere, 2021). In other words, humans can misinterpret the extracted data to give it the hue they want. While cue extraction seems to be robust and interesting in the stylometric evaluation of deceptive discourse, it is still necessary to keep in mind these potential biases and errors when automation becomes a decision-making tool.
We provided a review of common problems to deception detection and their potential solutions by relying on a majorly overlooked combination between theory-based and computational approaches to deception. To this day, the psychology and psycholinguistics research angle to deception detection commonly makes use of simple computational word-based stylometric tools while relying on theory-grounded approaches, while the computational approach proposed by the engineering literature relies almost exclusively on powerful algorithms without commonly mentioning the underlying theory explaining the differences between deceitful and sincere narratives. We argue for a combination of the strengths of both approaches (i.e., the understanding of cognitive and social mechanisms behind deception and the power of current algorithmic methods) for future research purposes.
Computational stylometry offers a set of tools that may help scholars and practitioners detect potentially deceptive verbal accounts. Relying on the power of computers and extraction algorithms, stylometry helps extract quickly the desired cues faster and more robustly than when humans use the actual grid to code textual data. The stylometric extracted data, when analyzed by algorithms, show above-chance discrimination rates between deceitful and authentic narratives while bringing interesting advantages for organizational concerns for legal practitioners. Little is known about how stylometric deception detection compares to human judgements, or even to the other methods of analysis of verbal statements, but the current studies seem to indicate at least similar results.
To the best of our knowledge, the present paper is also the first to make use of and extend the criteria developed by Vrij and Fisher (2016) regarding the applicability of stylometric deception detection on the field. Despite the fact that the computational stylometric approach seems to perform as well as the previous manual methods analyzed in the seminal study, and thus allegedly grant their use on the field, we nevertheless extended the list of criteria by considering ethical aspects. We argue that the lack of training datasets and current error rates, even in the best cases, remain insufficient to apply stylometric deception detection as a decision-making tool on the field.
This review also highlighted the limitations of current stylometric methods. To date, many studies in the psychology literature rely on the cue-based method to investigate the theoretical groudns of deception and make use of LIWC, while the other approaches remain explored (Hauch et al., 2015; Holtgraves and Jenkins, 2020; Schutte et al., 2021). It probably is the easiest stylometric tool to use to work on discourse analysis and deception detection. But this ease of use should not make scholars forget to look at other cues. As a matter of fact, a few studies have shown that LIWC involved certain issues that needed to be considered with care. Studies have highlighted for instance that LIWC was a word-based approach and consisted essentially of a list of individual words and could not take into account strings of words (Braun et al., 2019). For this reason, in sentences such as “I am happy” and “I am not happy”, the unigram happy will be considered each time as a sign of positive emotion, while it is not the case in the second sentence. LIWC thus omits context by relying solely on unique words fixed in a predefined ontology.
To counter this effect, a few studies have begun exploring new features to detect deception such as syntactic structure and part-of-speech tagging (Feng et al., 2012; Fornaciari et al., 2020) or Named-Entity Recognition (Kleinberg et al., 2018), and showed interesting insights in the content analysis of deceptive discourse. These encouraging paths need to be explored carefully, relying on evidence-supported linguistic and psychological models and interviewing procedures. But while stylometry shows a promising future for computational deception detection, there remain some methodological aspects that research should investigate.
Regarding the model-based approach, common machine-learning matters have rarely been tackled in the psychology and computational linguistics literature about deception detection. For instance, Kleinberg and colleagues (2019, 2021) explain how the methods developed to detect deceit computationally have rarely been tested on out-of-sample data. Most of the time, the division of the available data is favored to create a training sample (e.g., 20% of the available data) and a testing sample (e.g., 80% of the available data). Moreover, there are very few labeled datasets for deception detection purposes. We have mentioned Ott and colleagues’ dataset on deceptive opinion spam (Ott et al., 2013, Ott et al., 2012). Others have started to develop such as the one developed by Martens and Maalej (2019), the Paraphrased OPinion Spam (POPS; Kim et al., 2017), Ruspersonality (Litvinova et al., 2016), or the corpora made available by Amazon and Yelp. But as language is context-specific, the applications of the use of these datasets could not be transfered to computer-mediated communications, or even interactional deception detection (Sánchez-Junquera et al., 2020). Additionally, deception is, to the best of our knowledge, conceived as a binary variable in these datasets, where truthful and deceptive narratives are voluntarily antagonized. This means that even in the current datasets, a text is either deceptive or truthful. This does not reflect many cases where deception and truth are embedded in one another, representing an instance of the out-of-distribution generalization problem (Liu et al., 2020; Shen et al., 2021; Verigin et al., 2020a). Finally, the datasets artificially created for research purposes might not be reliable for training purposes (Fornaciari et al., 2020).
When considering the cue-based approach, the amount of cues investigated in linguistic deception detection could be widened if one considers the literature on authorship attribution and, to a bigger extent, on pragmatics. For instance, word length has rarely been considered, although it is supported by empirical evidence (Lewis and Frank, 2016). But as we explained above, the reliance on automatically extracted cues may cause an automation bias. We argue that it would be interesting to determine how this automation bias would appear in deception detection contexts, and how legal, human ressources, or insurance practitioners would consider a potentially automated decision-making system regarding deception detection. An adaptation of the aid/no-aid paradigm of Skitka et al. (1999) might be a first step to determining if a decision-supporting design may bias deception detection judgements. A second step would be to determine how trust in deception detection automation may influence decision-making when using computational deception detection methods.
We also wish to highlight how little is known about baselining in stylometric deception detection. In authorship attribution, from which stylometry originates, most studies rely on the comparison between an identified corpus of texts and statements to be attributed to an author. The determination of ground truth of the identified corpus in today’s authorship attribution problems is relatively reliable: Researchers rely often on tweets, blogs, or clearly signed data (Overdorf and Greenstadt, 2016). However such a signature does not exist in the field of deception detection. Still, we notice that little is known on how to adapt this baseline rationale to stylometric deception detection. For instance, could the linguistic style of the interviewer or the presentation of a model statement presented in Commentary 3 of the Nahari et al.‘s paper (2019) influence the language of the interviewee (see Richardson et al., 2014, for an example of Language Style Matching in police interrogation settings; see Porter et al., 2021 for a critical review of the model statement method)? This question bears significant importance, as the measurements used in stylometry and authorship attribution rely on similarity coefficients between identified and questioned documents, or in this case, authentic and deceptive texts. If the language of the interviewee changes and adopts the linguistic structures of the interviewer’s, there is a risk of obtaining inaccurate data, and thus a chance of biasing the samples used. Similarly, if a document guides the authentic or deceptive person’s narrative, it may introduce noise and disrupt the detection of linguistic signals that might indicate that a narrative has been manipulated. These factors should therefore be manipulated in experimental studies to determine their potential impact, but also to propose countermeasures if necessary.
There are other issues to consider when evaluating the baseline. For example, should one only rely on a single point of reference (as is now the case with baselining in deception detection), or use multiple linguistic sources to acquire the best overview of someone’s stylome (as is done in the authorship attribution domain)? Having a single point of reference may be problematic, as there are environmental and idiosyncratic factors that may impact the idiolect-baseline of the interviewee. Moreover, using a single reference point may have other disadvantages since its mere request may influence the subsequent narrative. Indeed, it has been shown that there is an interaction between the baseline and recall. Studies examining the change in order between baseline and recall have shown that the richness of detail and word count of the second statement was altered by the first (Verigin et al., 2020b). A recent study also highlighted this effect of the baseline on the second story (Tomas et al., 2021a).
But relying on a multiple points of reference causes other issues. For instance, ground truth may be harder to assess for each text. Moreover, the practical implications of multiple sources for baselining needs to be mentioned: Legal practitioners do not always have access to numerous documents, and if they do, the standardization of the documents may take a certain time that the legal field does not always have. These questions illustrate how little we actually know about verbal baselining. We argue that researchers should investigate the distinctiveness of verbal baseline establishment, and how to develop best practices combined with flexibility to be applied on the field.
Finally, as mentioned in the limits, modern verbal deception detection techniques rely on verbatim transcripts. Two problems arise: the time necessary for the transcription for oral data, and the method used for transcription. A few solutions should be investigated in order to tackle these limits. First, regarding the time-consuming approach of transcription, a human-in-the-loop approach might be of interest for this purpose6. Transcription softwares might provide a first transcript. Law enforcement officers or academic collaborators could then bring corrections to make it fit the above-mentioned guidelines. This interaction between humans and machines would provide an interesting solution to “meet the organizational time frame” mentioned in Nahari et al. (2019, p. 11). Second, as far as transcription methodology is concerned, we suggest that scholars focus on the emission of guidelines determining the rules to apply in transcribing the verbal discourse for deception detection purposes. More precisely, we suggest that researchers rely on the communication and linguistic domains to test experimentally what is best for verbal, and more particularly, computational stylometric cues extraction (e.g., Easton et al., 2000; Davidson, 2009).
These methodological issues prevent computational stylometry from being currently applied in the field. For this, we recommend that researchers in psychology, linguistics, and engineering cooperate and actively research these topics. This would be a way to expand each other’s knowledge: 1) for psychology scholars to provide evidence-based theory; 2) for linguists to provide the cues on which researchers may rely; and 3) for engineers to supply powerful natural linguistic processing algorithms to extract and analyze verbal data. The knowledge acquired from the coworking of these fields would be fine-tuned by discussing their practical application with legal practitioners to provide them with the best tools possible for verbal deception detection. These practical implications involve, for instance, deception detection in online forms allowing the filing of a report, the analysis of potentially deceptive e-mails, or the quest for truth in post-interview transcribed verbatims.
This tripartite collaboration will need to cover topics beyond the mere detection of deception. Deceptive verbal data can be found in many settings, ranging from insurance claims to opinions about hotels, including film reviews, investigative interviewing, malingering in a medical context. Some involve immediate interaction, while others may foster computer-mediated interaction, or no interaction at all. There is an increasing necessity to determine to what extent the context influences verbal deceit and challenges the idea behind the one-fit-for-all equation solving the deception detection problem (Sánchez-Junquera et al., 2020; Tomas et al., 2021b; Demarchi et al., 2021). The potential implications in the aforementioned contexts may involve computational methods beyond stylometry: If one relies on natural language processing, machine-learning, and artificial intelligence, there is increasing caution required on the ethics behind data collection, and the biases involved in the artificial intelligence literature. Data collected on the field, whether in insurance, investigative interviewing, medical, or in judicial context, are not collected for the purpose of deception detection: They are information gathered with the goal of making the best decision possible. If these private data are used for anything other than the purpose for which they were collected, it is ethically problematic to use them for deception detection, and more importantly so with the current detection rates. An essential discussion needs to happen regarding the treatment of verbal data for deception detection purposes.
Similarly (big) data evaluation through algorithmic and machine-learning means have also been pointed out for their biases. In machine-learning and artificial intelligence, bias refers to a priori knowledge and potential stereotypes that may lead to prejudice. Recent research has suggested that, like human beings, algorithms absorb stereotyped data and may taint, such as flowers and insects, with positive and negative emotions respectively because of the context in which they appear (Caliskan et al., 2017). If the flower-insect question seems trivial, studies have shown that natural processing trained on available data seemed to develop sexist representations of linguistic data, where men are identified as maestros and women as homemakers (Bolubasi et al., 2016; Caliskan et al., 2017; Papakyriakopoulos et al., 2019). Similarly, a race bias has also been observed (Manzini et al., 2019). It is thus extremely important to discuss these ethical issues in case of machine-learning based deception detection. Ethics are little discussed in the deception detection literature, but if, as developed by Vrij and Fisher (2016), stylometry or other verbal deception detection techniques may be used in the field, there is an urgent necessity to consider the ethics of data collection.
Computational stylometry offers a set of tools that can help academics and practitioners in insurance, justice, and human resources settings detect potentially deceptive verbal accounts. By relying on the power of computers and extraction algorithms, stylometry would allow the desired cues to be extracted more quickly and more robustly (agreement, reproducibility) than a solely manual work. Its development will constitute an important theoretical and practical advance in the field of deception detection. The more recent model-based approach, despite being considered as a black box, might provide better accuracy in terms of deception detection. Ethical questions regarding their use on the field need to be considered with absolute necessity. For this, we highly advocate for the combination of knowledge and skill from communication scientists, psychology and (psycho)linguistics researchers, philosophers, and computational scientists.
FT wrote the article after researching the addressed topics. OD provided insights regarding the automation bias, and proofread the manuscript. SD provided structural comments essential to the understanding of the manuscript, and proofread it.
Publication fees will be endorsed by the Tilburg School of Humanities and Digital Sciences.
The authors declare that the research was conducted in the absence of any commercial or financial relationships that could be construed as a potential conflict of interest.
All claims expressed in this article are solely those of the authors and do not necessarily represent those of their affiliated organizations, or those of the publisher, the editors and the reviewers. Any product that may be evaluated in this article, or claim that may be made by its manufacturer, is not guaranteed or endorsed by the publisher.
The authors wish to thank Dr. Prof. Emiel Krahmer for his remarks regarding the content of the manuscript as they helped improve its quality.
1The control/comparison questions test is a polygraph method for assessing physiological differences (e.g., heart rate, skin conductance, breathing rhythm) between a control phase and an eyewitness testimony. It is the archetypal polygraph test. The hidden information test, which is more recent and more efficient, does not have exactly the same objective: it aims at detecting, still in a physiological way, reactions to stimuli that only the guilty person could know (e.g., a photo of the murder weapon or a photo of the crime scene).
2The Behavioral Analysis Interview is a method developed to analyze the language and non-verbal behavior of suspicious individuals through the use of specific questions. Few studies have been published on the subject, and the results do not seem to indicate improved lie detection with this method (Vrij, Mann, and Fisher, 2006).
3Strategic Use of Evidence is a method of structuring the questions asked during a confrontation phase, and revealing them gradually and strategically, starting with less specific questions and ending with the most incriminating and specific questions.
4Nevertheless, stylometry does not preclude the occurrence of some commonly accepted biases in forensic science, such as the influence of context or confirmation bias (see Kassin et al., 2013 for a review; see also Masip & Herrero, 2017; Meissner & Kassin, 2002 for the relationships between contextual biases and deception detection).
5A less cumbersome alternative, more in line with the practices of the scientific community, is to specify and detail the methods and choices of transcriptions. Rules for the presentation of these details should be discussed (what methodological details to report, with what minimum degree of precision, etc.).
6Indeed, if the first interaction between humans and machines concerned decision support in the context of deception detection, with the problems we know, the collaboration between the computerized transcription tool and the subsequent human intervention seems less serious. Nevertheless, the latter should not be exempt from guidelines and recommendations, as discussed below. This will allow the homogenization of the transcriptions, and therefore the reduction of noise in the extraction of stylometric indices.
Aamodt, M. G., and Custer, H. (2006). Who Can Best Catch a Liar? A Meta-Analysis of Individual Differences in Detecting Deception. Forensic Exam 25, 6–11.
Afroz, S., Islam, A. C., Stolerman, A., Greenstadt, R., and McCoy, D. (2014). “Doppelgänger Finder: Taking Stylometry to the Underground,” in 2014 IEEE Symposium on Security and Privacy, SAN JOSE, CA, MAY 18-21, 2014 (IEEE), 212–226. doi:10.1109/SP.2014.21
Alam, T., Khan, A., and Alam, F. (2020). Punctuation Restoration Using Transformer Models for High-and Low-Resource Languages. Proceedings of the 2020 EMNLP Workshop W-NUT: The Sixth Workshop on Noisy User-generated Text, 132–142. doi:10.18653/v1/2020.wnut-1.18
Ali, M., and Levine, T. (2008). The Language of Truthful and Deceptive Denials and Confessions. Commun. Rep. 21, 82–91. doi:10.1080/08934210802381862
Almela, Á., Valencia-García, R., and Cantos, P. (2013). Seeing through Deception: A Computational Approach to Deceit Detection in Spanish Written Communication. Lesli 1, 3–12. doi:10.5195/lesli.2013.5
Amado, B. G., Arce, R., and Fariña, F. (2015). Undeutsch Hypothesis and Criteria Based Content Analysis: A Meta-Analytic Review. The Eur. J. Psychol. Appl. Leg. Context 7, 3–12. doi:10.1016/j.ejpal.2014.11.002
Amado, B. G., Arce, R., Fariña, F., and Vilariño, M. (2016). Criteria-Based Content Analysis (CBCA) Reality Criteria in Adults: A Meta-Analytic Review. Int. J. Clin. Health Psychol. 16, 201–210. doi:10.1016/j.ijchp.2016.01.002
Argamon, S., Whitelaw, C., Chase, P., Hota, S. R., Garg, N., and Levitan, S. (2007). Stylistic Text Classification Using Functional Lexical Features. J. Am. Soc. Inf. Sci. 58, 802–822. doi:10.1002/asi.20553
Baayen, R. H., Halteren, H., van Neijt, A., and Tweedie, F. (2002). An Experiment in Authorship Attribution. Proc. JADT 2002, 29–37.
Bailey, J. (2008). First Steps in Qualitative Data Analysis: Transcribing. Fam. Pract. 25, 127–131. doi:10.1093/fampra/cmn003
Banerjee, S., and Chua, A. Y. (2014). “A Linguistic Framework to Distinguish between Genuine and Deceptive Online Reviews,” in Proceedings of the International Conference on Internet Computing and Web Services. Editors S. I. Ao, O. Castillo, C. Douglas, D. D. Feng, and J. Lee, 501–506. Available at: http://www.iaeng.org/publication/IMECS2014/IMECS2014_pp501-506.pdf.
Barlas, G., and Stamatatos, E. (2020). “Cross-Domain Authorship Attribution Using Pre-trained Language Models,” in Artificial Intelligence Applications and Innovations. AIAI 2020. IFIP Advances in Information and Communication Technology (New York, NY: Springer International Publishing), 255–266. doi:10.1007/978-3-030-49161-1_22
Barlow, M. (2013). Individual Differences and Usage-Based Grammar. Ijcl 18, 443–478. doi:10.1075/ijcl.18.4.01bar
Barsever, D., Singh, S., and Neftci, E. (2020). Building a Better Lie Detector with BERT: The Difference between Truth and Lies. Proc. Int. Jt. Conf. Neural Networks. doi:10.1109/IJCNN48605.2020.9206937
Biros, D. P., Daly, M., and Gunsch, G. (2004). The Influence of Task Load and Automation Trust on Deception Detection. Gr. Decis. Negot. 13, 173–189. doi:10.1023/B:GRUP.0000021840.85686.57
Blandon-Gitlin, I., Pezdek, K., Rogers, M., and Brodie, L. (2005). Detecting Deception in Children: An Experimental Study of the Effect of Event Familiarity on CBCA Ratings. L. Hum. Behav. 29, 187–197. doi:10.1007/s10979-005-2417-8
Bogaard, G., Meijer, E. H., Vrij, A., and Merckelbach, H. (2016). Strong, but Wrong: Lay People's and Police Officers' Beliefs about Verbal and Nonverbal Cues to Deception. PLoS One 11, e0156615. doi:10.1371/journal.pone.0156615
Bolukbasi, T., Chang, K. W., Zou, J. Y., Saligrama, V., and Kalai, A. T. (2016). Man Is to Computer Programmer as Woman Is to Homemaker? Debiasing Word Embeddings. Adv. Neural Inf. Process. Syst. 29, 4349–4357.
Bond, C. F., and DePaulo, B. M. (2006). Accuracy of Deception Judgments. Pers Soc. Psychol. Rev. 10, 214–234. doi:10.1207/s15327957pspr1003_2
Bond, C. F., and DePaulo, B. M. (2008). Individual Differences in Judging Deception: Accuracy and Bias. Psychol. Bull. 134, 477–492. doi:10.1037/0033-2909.134.4.477
Bond, G. D., and Lee, A. Y. (2005). Language of Lies in Prison: Linguistic Classification of Prisoners' Truthful and Deceptive Natural Language. Appl. Cognit. Psychol. 19, 313–329. doi:10.1002/acp.1087
Braun, N., Goudbeek, M., and Krahmer, E. (2019). Language and Emotion - A Foosball Study: The Influence of Affective State on Language Production in a Competitive Setting. PLoS One 14, e0217419. doi:10.1371/journal.pone.0217419
Brennan, M., Afroz, S., and Greenstadt, R. (2012). Adversarial Stylometry. ACM Trans. Inf. Syst. Secur. 15, 1–22. doi:10.1145/2382448.2382450
Burns, M. B., and Moffitt, K. C. (2014). Automated Deception Detection of 911 Call Transcripts. Secur. Inform. 3, 1–9. doi:10.1186/s13388-014-0008-2
Cagnina, L. C., and Rosso, P. (2017). Detecting Deceptive Opinions: Intra and Cross-Domain Classification Using an Efficient Representation. Int. J. Uncertainty, Fuzziness Knowlege-Based Syst. 25, 151–174. doi:10.1142/S0218488517400165
Caliskan, A., Bryson, J. J., and Narayanan, A. (2017). Semantics Derived Automatically from Language Corpora Contain Human-like Biases. Science 356, 183–186. doi:10.1126/science.aal4230
Chen, C., Zhao, H., and Yang, Y. (2015). Deceptive Opinion Spam Detection Using Deep Level Linguistic Features. Lect. Notes Comput. Sci. (including Subser. Lect. Notes Artif. Intell. Lect. Notes Bioinformatics) 9362, 465–474. doi:10.1007/978-3-319-25207-0_43
Chen, X., Hao, P., Chandramouli, R., and Subbalakshmi, K. P. (2011). “Authorship Similarity Detection from Email Messages,” in International Workshop On Machine Learning And Data Mining In Pattern Recognition. Editor P. Perner (New York, NY: Springer), 375–386. doi:10.1007/978-3-642-23199-5_28
Chowdhury, H. A., Haque Imon, M. A., and Islam, M. S. (2019). “A Comparative Analysis of Word Embedding Representations in Authorship Attribution of Bengali Literature,” in 2018 21st International Conference of Computer and Information Technology (ICCIT), Dhaka, Bangladesh, December 21-23, 2018, 21–23. doi:10.1109/ICCITECHN.2018.8631977
Chung, C., and Pennebaker, J. W. (2007). “The Psychological Functions of Function Words,” in Social Communication. Editor K. Fiedler, 343–359.
Clark, A. M. S. (2011). Forensic Stylometric Authorship Analysis under the Daubert Standard. SSRN J. doi:10.2139/ssrn.2039824
Cummings, M. (2004). “Automation Bias in Intelligent Time Critical Decision Support Systems,” in AIAA 1st Intelligent Systems Technical Conference, Chicago, Illinois, 20-24 September 2004, 1–6. doi:10.2514/6.2004-6313
Daelemans, W. (2013). “Explanation in Computational Stylometry,” in International Conference on Intelligent Text Processing and Computational Linguistics (Berlin, Heidelberg: Springer), 451–462. doi:10.1007/978-3-642-37256-8_37
Davidson, C. (2009). Transcription: Imperatives for Qualitative Research. Int. J. Qual. Methods 8, 35–52. doi:10.1177/160940690900800206
de Vel, O., Anderson, A., Corney, M., and Mohay, G. (2001). Mining E-Mail Content for Author Identification Forensics. SIGMOD Rec. 30, 55–64. doi:10.1145/604264.604272
Demarchi, S., Tomas, F., and Fanton, L. (2021). False Rape Allegation and Regret: A Theoretical Model Based on Cognitive Dissonance. Arch. Sex. Behav. 50, 2067–2083. doi:10.1007/s10508-020-01847-z
DePaulo, B. M., Lindsay, J. J., Malone, B. E., Muhlenbruck, L., Charlton, K., and Cooper, H. (2003). Cues to Deception. Psychol. Bull. 129, 74–118. doi:10.1037/0033-2909.129.1.74
Devlin, J., Chang, M. W., Lee, K., and Toutanova, K. (2019). BERT: Pre-training of Deep Bidirectional Transformers for Language Understanding. NAACL HLT 2019 - 2019 Conf. North. Am. Chapter Assoc. Comput. Linguist. Hum. Lang. Technol. - Proc. Conf. 1, 4171–4186.
Dodier, O., and Denault, V. (2018). The Griffiths Question Map: A Forensic Tool for Expert Witnesses' Assessments of Witnesses and Victims' Statements. J. Forensic Sci. 63, 266–274. doi:10.1111/1556-4029.13477
Dzindolet, M. T., and Pierce, L. G. (2005). Using a Linguistic Analysis Tool to Detect Deception. Proc. Hum. Factors Ergon. Soc. Annu. Meet. 49, 563–567. doi:10.1177/154193120504900374
Easton, K. L., McComish, J. F., and Greenberg, R. (2000). Avoiding Common Pitfalls in Qualitative Data Collection and Transcription. Qual. Health Res. 10, 703–707. doi:10.1177/104973200129118651
Erman, B. (2007). Cognitive Processes as Evidence of the Idiom Principle. Ijcl 12, 25–53. doi:10.1075/ijcl.12.1.04erm
Fabien, M., Villatoro-Tello, E., Motlicek, P., and Parida, S. (2020). “BertAA : BERT fine-tuning for Authorship Attribution,” in Proceedings of the 17th International Conference on Natural Language Processing (ICON). Editors P. Bhattacharyya, D. M. Sharma, and R. Sangal (NLP Association of India), 127–137.
Fatima, M., Hasan, K., Anwar, S., and Nawab, R. M. A. (2017). Multilingual Author Profiling on Facebook. Inf. Process. Management 53, 886–904. doi:10.1016/j.ipm.2017.03.005
Feng, S., Banerjee, R., and Choi, Y. (2012). Syntactic Stylometry for Deception Detection. in Proceedings of the 50th Annual Meeting of the Association for Computational Linguistics: Short Papers-Volume 2, Jeju Island Korea, July 8 - 14, 2012eds. H. Li, C. Lin, M. Osborne, G. G. Lee, and J. C. Park (Association for Computational Linguistics), 171–175.
Fornaciari, T., Bianchi, F., Poesio, M., and Hovy, D. (2021). BERTective: Language Models and Contextual Information for Deception Detection. EACL 2021 - 16th Conf. Eur. Chapter Assoc. Comput. Linguist. Proc. Conf., 2699–2708. doi:10.18653/v1/2021.eacl-main.232
Fornaciari, T., Cagnina, L., Rosso, P., and Poesio, M. (2020). Fake Opinion Detection: How Similar Are Crowdsourced Datasets to Real Data? Lang. Resour. Eval. 54, 1019–1058. doi:10.1007/s10579-020-09486-5
Fornaciari, T., and Poesio, M. (2013). Automatic Deception Detection in Italian Court Cases. Artif. Intell. L. 21, 303–340. doi:10.1007/s10506-013-9140-4
Forsyth, L., and Anglim, J. (2020). Using Text Analysis Software to Detect Deception in Written Short-Answer Questions in Employee Selection. Int. J. Sel. Assess. 28, 236–246. doi:10.1111/ijsa.12284
Fox, B., and Farrington, D. P. (2018). What have we Learned from Offender Profiling? A Systematic Review and Meta-Analysis of 40 Years of Research. Psychol. Bull. 144, 1247–1274. doi:10.1037/bul0000170
Fuller, C. M., Biros, D. P., and Wilson, R. L. (2009). Decision Support for Determining Veracity via Linguistic-Based Cues. Decis. Support Syst. 46, 695–703. doi:10.1016/j.dss.2008.11.001
Goddard, K., Roudsari, A., and Wyatt, J. C. (2012). Automation Bias: a Systematic Review of Frequency, Effect Mediators, and Mitigators. J. Am. Med. Inform. Assoc. 19, 121–127. doi:10.1136/amiajnl-2011-000089
Goodman, B., and Flaxman, S. (2017). European Union Regulations on Algorithmic Decision-Making and a "Right to Explanation". AIMag 38, 50–57. doi:10.1609/aimag.v38i3.2741
Grzybowski, P., Juralewicz, E., Juralewicz, E., and Piasecki, M. (2019). Sparse Coding in Authorship Attribution for Polish Tweets. Int. Conf. Recent Adv. Nat. Lang. Process. RANLP, 409–417. doi:10.26615/978-954-452-056-4_048
Halliday, M. A. K., McIntosh, A., and Strevens, P. (1964). The Linguistic Sciences and Language Teaching. London, UK: Longmans.
Han, Y. (2016). Deception Detection Techniques Using Polygraph in Trials: Current Status and Social Scientific Evidence. Contemp. Read. L. Soc. Justice 8, 115–147. doi:10.22381/crlsj8220165
Hancock, J. T., Curry, L. E., Goorha, S., and Woodworth, M. T. (2004). “Lies in Conversation: An Examination of Deception Using Automated Linguistic Analysis,” in Proceedings of the Annual Meeting of the Cognitive Science Society. Editors K. Forbus, D. Gentner, and T. Regier (Mahwah, NJ: Lawrence Erlbaum Associates Inc), 535–540.
Harris, Z. S. (1954). Distributional Structure. WORD 10, 146–162. doi:10.1080/00437956.1954.11659520
Hauch, V., Blandón-Gitlin, I., Masip, J., and Sporer, S. L. (2015). Are Computers Effective Lie Detectors? A Meta-Analysis of Linguistic Cues to Deception. Pers Soc. Psychol. Rev. 19, 307–342. doi:10.1177/1088868314556539
Hauch, V., Sporer, S. L., Masip, J., and Blandón-Gitlin, I. (2017). Can Credibility Criteria Be Assessed Reliably? A Meta-Analysis of Criteria-Based Content Analysis. Psychol. Assess. 29, 819–834. doi:10.1037/pas0000426
Hernández Fusilier, D., Montes-y-Gómez, M., Rosso, P., and Cabrera, R. G. (2015). “Detection of Opinion Spam with Character N-Grams,” in CICLing 2015: Computational Linguistics and Intelligent Text Processing. Editor A. Gelbukh (Springer), 285–294. doi:10.1007/978-3-319-18117-2_21
Hitschler, J., van den Berg, E., and Rehbein, I. (2017). “Authorship Attribution with Convolutional Neural Networks and POS-Eliding,” in Proceedings of the Workshop on Stylistic Variation, 53–58. doi:10.18653/v1/w17-4907
Holmes, D. I. (1998). The Evolution of Stylometry in Humanities Scholarship. Literary Linguistic Comput. 13, 111–117. doi:10.1093/llc/13.3.111
Holtgraves, T., and Jenkins, E. (2020). Texting and the Language of Everyday Deception. Discourse Process. 57, 535–550. doi:10.1080/0163853X.2019.1711347
Home Office and Department of Health (2002). Achieving Best Evidence: Guidance for Vulnerable or Intimidated Witnesses, Including Children. London: Her Majesty’s Stationery Office.
Jackson, J. C., Watts, J., List, J.-M., Drabble, R., Lindquist, K., and Lindquist, K. (2020). From Text to Thought: How Analyzing Language Can Advance Psychological Science. PsyArXiv Prepr. n/a. doi:10.31234/osf.io/nws35
Johnson, A., and Wright, D. (2017). Identifying Idiolect in Forensic Authorship Attribution: an N-Gram Textbite Approach. Lang. Law= Ling. e Direito 1. Available at: https://pentaho.letras.up.pt/index.php/LLLD/article/download/2443/2233.
Juola, P. (2012). Stylometry and Immigration: A Case Study. J. Law Policy 21. Available at: https://brooklynworks.brooklaw.edu/jlp/vol21/iss2/2.
Jupe, L. M., Vrij, A., Leal, S., and Nahari, G. (2018). Are You for Real? Exploring Language Use and Unexpected Process Questions within the Detection of Identity Deception. Appl. Cognit Psychol. 32, 622–634. doi:10.1002/acp.3446
Kestemont, M. (2014). “Function Words in Authorship Attribution. From Black Magic to Theory,” in Proceedings of the 3rd Workshop on Computational Linguistics for Literature (CLFL). Editors A. Feldman, A. Kazantseva, and S. Szpakowicz, 59–66. doi:10.3115/v1/w14-0908
Khatun, A., Rahman, A., Islam, M. S., and Marium-E-Jannat, (2019). Authorship Attribution in Bangla Literature Using Character-Level CNN. 2019 22nd Int. Conf. Comput. Inf. Technol. ICCIT, 18–20. doi:10.1109/ICCIT48885.2019.9038560
Khawaja, M. A., Chen, F., Owen, C., and Hickey, G. (2009). “Cognitive Load Measurement from User's Linguistic Speech Features for Adaptive Interaction Design,” in IFIP Conference on Human-Computer Interaction (Berlin, Germany: Springer), 485–489. doi:10.1007/978-3-642-03655-2_54
Kim, S., Lee, S., Park, D., and Kang, J. (2017). “Constructing and Evaluating a Novel Crowdsourcing-Based Paraphrased Opinion Spam Dataset,” in 26th Int. World Wide Web Conf, Perth, Australia (WWW), 827–836. doi:10.1145/3038912.3052607
Kleinberg, B., Mozes, M., Arntz, A., and Verschuere, B. (2018). Using Named Entities for Computer-Automated Verbal Deception Detection. J. Forensic Sci. 63, 714–723. doi:10.1111/1556-4029.13645
Kleinberg, B., and Verschuere, B. (2021). How Humans Impair Automated Deception Detection Performance. Acta Psychologica 213, 103250. doi:10.1016/j.actpsy.2020.103250
Kocher, M., and Savoy, J. (2018). Distributed Language Representation for Authorship Attribution. Digit. Scholarsh. Humanit. 33, 425–441. doi:10.1093/llc/fqx046
Koppel, M., Schler, J., and Argamon, S. (2009). Computational Methods in Authorship Attribution. J. Am. Soc. Inf. Sci. 60, 9–26. doi:10.1002/asi.20961
Larreau, C. R. (2017). “Daubert V. Merrell Dow Pharmaceuticals, Inc,” in The SAGE Encyclopedia of Abnormal and Clinical Psychology. Editor A. Wenzel (SAGE Publications). doi:10.4135/9781483365817.n374
Leal, S., Vrij, A., Warmelink, L., Vernham, Z., and Fisher, R. P. (2015). You Cannot Hide Your Telephone Lies: Providing a Model Statement as an Aid to Detect Deception in Insurance Telephone Calls. Leg. Crim Psychol. 20, 129–146. doi:10.1111/lcrp.12017
Lewis, M. L., and Frank, M. C. (2016). The Length of Words Reflects Their Conceptual Complexity. Cognition 153, 182–195. doi:10.1016/j.cognition.2016.04.003
Li, W., Sadigh, D., Sastry, S. S., and Seshia, S. A. (2014). “Synthesis for Human-In-The-Loop Control Systems,” in International Conference on Tools and Algorithms for the Construction and Analysis of Systems (Springer), 470–484. doi:10.1007/978-3-642-54862-8_40
Litvinova, O., Seredin, P., Litvinova, T., and Lyell, J. (2017). “Deception Detection in Russian Texts,” in Proceedings of the Student Research Workshop at the 15th Conference of the European (Stroudsburg, PA: USA: Chapter of the Association for Computational Linguistics), 43–52. doi:10.18653/v1/e17-4005
Litvinova, T., Litvinova, O., Zagorovskaya, O., Seredin, P., Sboev, A., and Romanchenko, O. (2016). ““ Ruspersonality”: A Russian Corpus for Authorship Profiling and Deception Detection,” in Artificial Intelligence and Natural Language and Information Extraction, Social Media and Web Search FRUCT Conference (AINL-ISMW FRUCT), St. Petersburg, Russia, 9-14 Nov. 2015. Editors S. Balandin, and T. Tyutina, 29–35. doi:10.1109/fruct.2016.7584767
Liu, C., Sun, X., Wang, J., Tang, H., Li, T., Qin, T., et al. (2020). “Learning Causal Semantic Representation for Out-Of-Distribution Prediction,” in 35th Conference On Neural Information Processing Systems (NeurIPS 2021), 1–16. http://arxiv.org/abs/2011.01681.Available at:
Manzini, T., Chong, L. Y., Black, A. W., and Tsvetkov, Y. (2019). “Black Is to Criminal as Caucasian Is to Police: Detecting and Removing Multiclass Bias in Word Embeddings,” in Proceedings Of the 2019 Conference Of the North American Chapter Of the Association For Computational Linguistics: Human Language Technologies Long and Short Papers. Editors J. Burstein, C. Doran, and T. Solorio (Association for Computational Linguistics), 615–621. doi:10.18653/v1/n19-1062
Martens, D., and Maalej, W. (2019). Towards Understanding and Detecting Fake Reviews in App Stores. Empir Softw. Eng 24, 3316–3355. doi:10.1007/s10664-019-09706-9
Masip, J., Bethencourt, M., Lucas, G., Segundo, M. S.-S., and Herrero, C. (2012). Deception Detection from Written Accounts. Scand. J. Psychol. 53, 103–111. doi:10.1111/j.1467-9450.2011.00931.x
Masip, J., and Herrero, C. (2015). Police Detection of Deception: Beliefs about Behavioral Cues to Deception Are strong Even Though Contextual Evidence Is More Useful. J. Commun. 65, 125–145. doi:10.1111/jcom.12135
Masip, J., Sporer, S. L., Garrido, E., and Herrero, C. (2005). The Detection of Deception with the Reality Monitoring Approach: A Review of the Empirical Evidence. Psychol. Crime L. 11, 99–122. doi:10.1080/10683160410001726356
Meissner, C. A., and Kassin, S. M. (2002). "He's Guilty!": Investigator Bias in Judgments of Truth and Deception. L. Hum. Behav. 26, 469–480. doi:10.1023/A:1020278620751
Merlo, S., and Mansur, L. L. (2004). Descriptive Discourse: Topic Familiarity and Disfluencies. J. Commun. Disord. 37, 489–503. doi:10.1016/j.jcomdis.2004.03.002
Mihalcea, R., and Strapparava, C. (2009). “The Lie Detector: Explorations in the Automatic Recognition of Deceptive Language,” in Proceedings of the ACL-IJCNLP 2009 Conference Short Papers, Suntec, Singapore, 4 August 2009. Editors K. Su, J. Su, J. Wiebe, and H. Li (Association for Computational Linguistics), 309–312.
Mikolov, T., Sutskever, I., Chen, K., Corrado, G., and Dean, J. (2013). Distributed Representations of Words and Phrases and Their Compositionality. Nips’13 Proc. 26th Int. Conf. Neural Inf. Process. Syst. - 2, 3111–3119.
Mosier, K. L., Palmer, E. A., and Degani, A. (1992). Electronic Checklists: Implications for Decision Making. Proc. Hum. Factors Soc. Annu. Meet. 36, 7–11. doi:10.1177/154193129203600104
Mosier, K. L., Skitka, L. J., Burdick, M. D., and Heers, S. T. (1996). Automation Bias, Accountability, and Verification Behaviors. Proc. Hum. Factors Ergon. Soc. Annu. Meet. 40, 204–208. doi:10.4324/9781315095080-1610.1177/154193129604000413
Mosier, K. L., and Skitka, L. J. (2018). “Human Decision Makers and Automated Decision Aids: Made for Each Other,” in Automation And Human Performance: Theory And Applications. Editors R. Parasuraman, and M. Mouloua ((NJ: Erlbaum), 201–220.
Munafò, M. R., Nosek, B. A., Bishop, D. V. M., Button, K. S., Chambers, C. D., Percie du Sert, N., et al. (2017). A Manifesto for Reproducible Science. Nat. Hum. Behav. 1, 21. doi:10.1038/s41562-016-0021
Nahari, G., Ashkenazi, T., Fisher, R. P., Granhag, P. A., Hershkowitz, I., Masip, J., et al. (2019). 'Language of Lies': Urgent Issues and Prospects in Verbal Lie Detection Research. Leg. Crim Psychol. 24, 1–23. doi:10.1111/lcrp.12148
Nahari, G. (2018b). Reality Monitoring in the Forensic Context: Digging Deeper into the Speech of Liars. J. Appl. Res. Mem. Cogn. 7, 432–440. doi:10.1016/j.jarmac.2018.04.003
Nahari, G. (2018a). “The Applicability of the Verifiability Approach to the Real World,” in Detecting Concealed Information and Deception. Editor P. Rosenfield (London, UK: Academic Press), 329–349. doi:10.1016/b978-0-12-812729-2.00014-8
Nahari, G., Vrij, A., and Fisher, R. P. (2014). Exploiting Liars' Verbal Strategies by Examining the Verifiability of Details. Leg. Crim Psychol. 19, 227–239. doi:10.1037/e669802012-21910.1111/j.2044-8333.2012.02069.x
Nam, D., Yasmin, J., and Zulkernine, F. (20202020). “Effects of Pre-trained Word Embeddings on Text-Based Deception Detection,” in Proc. - IEEE 18th Int. Conf. Dependable, Auton. Secur. Comput. IEEE 18th Int. Conf. Pervasive Intell. Comput. IEEE 6th Int. Conf. Cloud Big Data Comput. IEEE 5th Cyber Sci. Technol. Congr. DASC/PiCom/CBDCom/CyberSciTech, Calgary, AB, Canada, 17-22 Aug. 2020, 437–443. doi:10.1109/DASC-PICom-CBDCom-CyberSciTech49142.2020.00083
Newman, M. L., Pennebaker, J. W., Berry, D. S., and Richards, J. M. (2003). Lying Words: Predicting Deception from Linguistic Styles. Pers Soc. Psychol. Bull. 29, 665–675. doi:10.1177/0146167203029005010
Nortje, A., and Tredoux, C. (2019). How Good are We at Detecting Deception? A Review of Current Techniques and Theories. South African J. Psychol. 49, 491–504. doi:10.1177/0081246318822953
O'Malley, S., and Besner, D. (2008). Reading Aloud: Qualitative Differences in the Relation between Stimulus Quality and Word Frequency as a Function of Context. J. Exp. Psychol. Learn. Mem. Cogn. 34, 1400–1411. doi:10.1037/a0013084
Oberlader, V. A., Naefgen, C., Koppehele-Gossel, J., Quinten, L., Banse, R., and Schmidt, A. F. (2016). Validity of Content-Based Techniques to Distinguish True and Fabricated Statements: A Meta-Analysis. L. Hum. Behav. 40, 440–457. doi:10.1037/lhb0000193
Ott, M., Cardie, C., and Hancock, J. T. (2013). “Negative Deceptive Opinion Spam,” in Proceedings of the 2013 Conference of the North American Chapter of the Association for Computational Linguistics: Human language technologies, Georgia, USA, June 9-14, 2013. Editors L. Vanderwende, and H. Daumé III (Atlanta, Georgia: Association for Computational Linguistics), 497–501.In
Ott, M., Cardie, C., and Hancock, J. (2012). Estimating the Prevalence of Deception in Online Review Communities. WWW’12 - Proc. 21st Annu. Conf. World Wide Web, 201–210. doi:10.1145/2187836.2187864
Overdorf, R., and Greenstadt, R. (2016). Blogs, Twitter Feeds, and Reddit Comments: Cross-Domain Authorship Attribution. Proc. Priv. Enhancing Technol. 2016, 155–171. doi:10.1515/popets-2016-0021
Papakyriakopoulos, O., Hegelich, S., Serrano, J. C. M., and Marco, F. (2020). “Bias in Word Embeddings,” in Proceedings of the 2020 Conference on Fairness, Accountability, and Transparency, Barcelona Spain, January 27 - 30, 2020. Editors M. Hildebrandt, and C. Castillo, 446–457. doi:10.1145/3351095.3372843
Pérez-Rosas, V., Abouelenien, M., Mihalcea, R., and Burzo, M. (2015a). “Deception Detection Using Real-Life Trial Data,” in Proceedings of the 2015 ACM on International Conference on Multimodal Interaction, Seattle Washington USA, November 9 - 13, 2015. Editors Z. Zhang, and P. Cohen, 59–66. doi:10.1145/2818346.2820758
Pérez-Rosas, V., Abouelenien, M., Mihalcea, R., Xiao, Y., Linton, C., and Burzo, M. (2015b). “Verbal and Nonverbal Clues for Real-Life Deception Detection,” in Proceedings of the 2015 Conference on Empirical Methods in Natural Language Processing, Lisbon, Portugal, 17-21 September 2015. Editors L. Màrquez, C. Callison-Burch, and J. Su, 2336–2346. doi:10.18653/v1/d15-1281
Pérez-Rosas, V., Davenport, Q., Dai, A. M., Abouelenien, M., and Mihalcea, R. (2017). “Identity Deception Detection,” in Proc. Eighth Int. Jt. Conf. Nat. Lang. Process., 885–894. Available at: https://www.aclweb.org/anthology/I17-1089.
Porter, C. N., Taylor, R., and Salvanelli, G. (2021). A Critical Analysis of the Model Statement Literature: Should This Tool Be Used in Practice? J. Investig. Psychol. Offender Profil 18, 35–55. doi:10.1002/jip.1563
Qin, T., Burgoon, J. K., Blair, J. P., and Nunamaker, J. F. (2005). “Modality Effects in Deception Detection and Applications in Automatic-Deception-Detection,” in Proceedings of the 38th annual Hawaii international conference on system sciences, Big Island, HI, USA, 6-6 Jan. 2005. Editors J. F. Nunamaker, and R. O. Briggs, 23–33. doi:10.1109/hicss.2005.436
Raaijmakers, S. (2022). Deep Learning for Natural Language Processing. New York, NY: Manning Publications. doi:10.1007/978-981-16-0882-7_45
Raj, C., and Meel, P. (2021). “Microblogs Deception Detection Using BERT and Multiscale CNNs,” in 2021 2nd Global Conference for Advancement in Technology (GCAT), Bangalore, India, 1-3 Oct. 2021 (IEEE), 1–6. doi:10.1109/gcat52182.2021.9587698
Rastogi, A., and Mehrotra, M. (2017). Opinion Spam Detection in Online Reviews. J. Info. Know. Mgmt. 16. doi:10.1142/S0219649217500368
Reddy, T. R., Vardhan, B. V., and Reddy, P. V. (2016). A Survey on Authorship Profiling Techniques. Int. J. Appl. Eng. Res. 11 (5), 1750036–1753102.
Reed, M. (2000). He Who Hesitates: Hesitation Phenomena as Quality Control in Speech Production, Obstacles in Non-native Speech Perception. J. Education 182, 72–97. doi:10.1177/002205740018200306
Richardson, B. H., Taylor, P. J., Snook, B., Conchie, S. M., and Bennell, C. (2014). Language Style Matching and Police Interrogation Outcomes. L. Hum. Behav. 38, 357–366. doi:10.1037/lhb0000077
Rogers, A., Kovaleva, O., and Rumshisky, A. (2020). A Primer in Bertology: What We Know about How Bert Works. Trans. Assoc. Comput. Linguistics 8, 842–866. doi:10.1162/tacl_a_00349
Rosso, P., and Cagnina, L. C. (2017). “Deception Detection and Opinion Spam,” in A Practical Guide To Sentiment Analysis. Editors E. Cambria, D. Das, S. Bandyopadhyay, and A. Feraco, 155–171. doi:10.1007/978-3-319-55394-8_8
Rubin, V. L., Chen, Y., and Conroy, N. K. (2015). Deception Detection for News: Three Types of Fakes. Proc. Assoc. Info. Sci. Tech. 52, 1–4. doi:10.1002/pra2.2015.145052010083
Rude, S., Gortner, E.-M., and Pennebaker, J. (2004). Language Use of Depressed and Depression-Vulnerable College Students. Cogn. Emot. 18, 1121–1133. doi:10.1080/02699930441000030
Sánchez-Junquera, J., Villaseñor-Pineda, L., Montes-y-Gómez, M., Rosso, P., and Stamatatos, E. (2020). Masking Domain-specific Information for Cross-Domain Deception Detection. Pattern Recognition Lett. 135, 122–130. doi:10.1016/j.patrec.2020.04.020
Schneider, L., Powell, D. M., and Roulin, N. (2015). Cues to Deception in the Employment Interview. Int. J. Select Assess. 23, 182–190. doi:10.1111/ijsa.12106
Schutte, M., Bogaard, G., Mac Giolla, E., Warmelink, L., Kleinberg, B., and Verschuere, B. (2021). Man versus Machine : Comparing Manual with LIWC Coding of Perceptual and Contextual Details for Verbal Lie Detection. Available at: https://psyarxiv.com/cth58/.
Senel, L. K., Utlu, I., Yücesoy, V., Koç, A., and Cukur, T. (2018). Semantic Structure and Interpretability of Word Embeddings. Ieee/acm Trans. Audio Speech Lang. Process. 26, 1769–1779. doi:10.1109/TASLP.2018.2837384
Shah, A. K., and Oppenheimer, D. M. (2009). The Path of Least Resistance. Curr. Dir. Psychol. Sci. 18, 232–236. doi:10.1111/j.1467-8721.2009.01642.x
Shen, Z., Liu, J., He, Y., Zhang, X., Xu, R., Yu, H., et al. (2021). Towards Out-Of-Distribution Generalization: A Survey. Available at: http://arxiv.org/abs/2108.13624.
Shojaee, S., Murad, M. A. A., Azman, A. B., Sharef, N. M., and Nadali, S. (2013). “Detecting Deceptive Reviews Using Lexical and Syntactic Features,” in 2013 13th International Conference on Intelligent Systems Design and Applications, Salangor, Malaysia, 8-10 Dec. 2013, 53–58. doi:10.1109/isda.2013.6920707
Shrestha, P., Sierra, S., González, F., Montes, M., Rosso, P., and Solorio, T. (2017). Convolutional Neural Networks for Authorship Attribution of Short Texts. 15th Conf. Eur. Chapter Assoc. Comput. Linguist. EACL 2017 - Proc. Conf. 2, 669–674. doi:10.18653/v1/e17-2106
Skitka, L. J., Mosier, K., and Burdick, M. D. (2000). Accountability and Automation Bias. Int. J. Human-Computer Stud. 52, 701–717. doi:10.1006/ijhc.1999.0349
Skitka, L. J., Mosier, K. L., and Burdick, M. (1999). Does Automation Bias Decision-Making? Int. J. Human-Computer Stud. 51, 991–1006. doi:10.1006/ijhc.1999.0252
Sporer, S. L., and Ulatowska, J. (2021). Indirect and Unconscious Deception Detection: Too Soon to Give Up?. Front. Psychol. 12. doi:10.3389/fpsyg.2021.601852
Sporer, S. L. (1997). The Less Travelled Road to Truth: Verbal Cues in Deception Detection in Accounts of Fabricated and Self-Experienced Events. Appl. Cognit. Psychol. 11, 373–397. doi:10.1002/(sici)1099-0720(199710)11:5<373:aid-acp461>3.0.co;2-0
Stamatatos, E. (2009). A Survey of Modern Authorship Attribution Methods. J. Am. Soc. Inf. Sci. 60, 538–556. doi:10.1002/asi.21001
Stel, M., Schwarz, A., van Dijk, E., and van Knippenberg, A. (2020). The Limits of Conscious Deception Detection: When Reliance on False Deception Cues Contributes to Inaccurate Judgments. Front. Psychol. 11, 1–12. doi:10.3389/fpsyg.2020.01331
Sternglanz, R. W., Morris, W. L., Morrow, M., and Braverman, J. (2019). “A Review of Meta-Analyses about Deception Detection,” in The Palgrave Handbook of Deceptive Communication. Editor T. Docan-Morgan (Springer International Publishing), 303–326. doi:10.1007/978-3-319-96334-110.1007/978-3-319-96334-1_16
Stoop, W., and van den Bosch, A. (2014). Improving Word Prediction for Augmentative Communication by Using Idiolects and Sociolects. DuJAL 3, 137–154. doi:10.1075/dujal.3.2.03sto
Strand, N., Nilsson, J., Karlsson, I. C. M., and Nilsson, L. (2014). Semi-automated versus Highly Automated Driving in Critical Situations Caused by Automation Failures. Transportation Res. F: Traffic Psychol. Behav. 27, 218–228. doi:10.1016/j.trf.2014.04.005
Strömwall, L. A., Granhag, P. A., and Hartwig, M. (2004). “Practitioners' Beliefs about Deception,” in The Detection of Deception in Forensic Contexts. Editors P. A. Granhag, and L. Strömwall (Cambridge, UK: Cambridge University Press), 229–250. doi:10.1017/cbo9780511490071.010
Tackman, A. M., Sbarra, D. A., Carey, A. L., Donnellan, M. B., Horn, A. B., Holtzman, N. S., et al. (2019). Depression, Negative Emotionality, and Self-Referential Language: A Multi-Lab, Multi-Measure, and Multi-Language-Task Research Synthesis. J. Personal. Soc. Psychol. 116, 817–834. doi:10.1037/pspp0000187
Tomas, F., Dodier, O., and Demarchi, S. (2021a). Baselining Affects the Production of Deceptive Narratives. Appl. Cognit Psychol. 35, 300–307. doi:10.1002/acp.3768
Tomas, F., Durand, T. C., Boulay, F., and Renard, T. (2021b). Les “Documenteurs”. Nouvelle Arme Dans La Guerre de L’information. Rev. Int. d’Intelligence Économique 13, 119–142.
Tomas, F., Tsimperidis, I., Demarchi, S., and El Massioui, F. (2021c). Keyboard Dynamics Discrepancies between Baseline and Deceptive Eyewitness Narratives. Appl. Cognit Psychol. 35, 112–122. doi:10.1002/acp.3743
van Halteren, H., Baayen, H., Tweedie, F., Haverkort, M., and Neijt, A. (2005). New Machine Learning Methods Demonstrate the Existence of a Human Stylome. J. Quantitative Linguistics 12, 65–77. doi:10.1080/09296170500055350
Verhoeven, B., Daelemans, W., and Plank, B. (2016). “Twisty: a Multilingual Twitter Stylometry Corpus for Gender and Personality Profiling,” in Proceedings of the 10th Annual Conference on Language Resources and Evaluation (LREC 2016), Portorož, Slovenia, May 23-28, 2016. N. Calzolari, K. Choukri, T. Declerck, S. Goggi, M. Grobelnik, B. Maegaardet al. 1632–1637.
Verigin, B. L., Meijer, E. H., and Vrij, A. (2020a). Embedding Lies into Truthful Stories Does Not Affect Their Quality. Appl. Cogn. Psychol. 34, 516–525. doi:10.1002/acp.3642
Verigin, B. L., Meijer, E. H., Vrij, A., and Zauzig, L. (2020b). The Interaction of Truthful and Deceptive Information. Psychol. Crime Law 26, 367–383. doi:10.1080/1068316X.2019.1669596
Vrij, A., Fisher, R. P., and Blank, H. (2017). A Cognitive Approach to Lie Detection: A Meta-Analysis. Leg. Crim Psychol. 22, 1–21. doi:10.1111/lcrp.12088
Vrij, A., and Fisher, R. P. (2016). Which Lie Detection Tools are Ready for Use in the Criminal Justice System?. J. Appl. Res. Mem. Cogn. 5, 302–307. doi:10.1016/j.jarmac.2016.06.014
Vrij, A., Kneller, W., and Mann, S. (2000). The Effect of Informing Liars about Criteria-Based Content Analysis on Their Ability to Deceive CBCA-Raters. Leg. Criminol. Psychol. 5, 57–70. doi:10.1348/135532500167976
Vrij, A., Nahari, G., Isitt, R., and Leal, S. (2016). Using the Verifiability Lie Detection Approach in an Insurance Claim Setting. J. Investig. Psych. Offender Profil. 13, 183–197. doi:10.1002/jip.1458
Vrij, A. (2018). “Verbal Lie Detection Tools from an Applied Perspective,” in Detecting Concealed Information and Deception. Editor P. Rosenfield (London, UK: Academic Press), 297–327. doi:10.1016/b978-0-12-812729-2.00013-6
Walczyk, J. J., Harris, L. L., Duck, T. K., and Mulay, D. (2014). A Social-Cognitive Framework for Understanding Serious Lies: Activation-Decision-Construction-Action Theory. New Ideas Psychol. 34, 22–36. doi:10.1016/j.newideapsych.2014.03.001
Walsh, D., and Bull, R. (2015). Interviewing Suspects: Examining the Association between Skills, Questioning, Evidence Disclosure, and Interview Outcomes. Psychol. Crime L. 21, 661–680. doi:10.1080/1068316x.2015.1028544
Woolls, D. (2010). “Computational Forensic Linguistics* Searching for Similarity in Large Specialised Corpora,” in The Routledge Handbook of Forensic Linguistics. Editors M. Coulthard, and A. Johnson (New York, NY: Routledge), 576–590. doi:10.4324/9780203855607-55
Wright, D. (2017). Using Word N-Grams to Identify Authors and Idiolects. Ijcl 22, 212–241. doi:10.1075/ijcl.22.2.03wri
Zhang, R., Hu, Z., Guo, H., and Mao, Y. (2020). Syntax Encoding with Application in Authorship Attribution. Proc. 2018 Conf. Empir. Methods Nat. Lang. Process. EMNLP, 2742–2753. doi:10.18653/v1/d18-1294
Zhang, Y., Jin, R., and Zhou, Z.-H. (2010). Understanding Bag-Of-Words Model: A Statistical Framework. Int. J. Mach. Learn. Cyber. 1, 43–52. doi:10.1007/s13042-010-0001-0
Keywords: deception detection, stylometry, forensic linguistics, automation bias, computational linguistics
Citation: Tomas F, Dodier O and Demarchi S (2022) Computational Measures of Deceptive Language: Prospects and Issues. Front. Commun. 7:792378. doi: 10.3389/fcomm.2022.792378
Received: 10 October 2021; Accepted: 10 January 2022;
Published: 02 February 2022.
Edited by:
Antonio Benítez-Burraco, Sevilla University, SpainReviewed by:
Tomasz Walkowiak, Wrocław University of Technology, PolandCopyright © 2022 Tomas, Dodier and Demarchi. This is an open-access article distributed under the terms of the Creative Commons Attribution License (CC BY). The use, distribution or reproduction in other forums is permitted, provided the original author(s) and the copyright owner(s) are credited and that the original publication in this journal is cited, in accordance with accepted academic practice. No use, distribution or reproduction is permitted which does not comply with these terms.
*Correspondence: Frédéric Tomas, Zi5qLnkudG9tYXNAdGlsYnVyZ3VuaXZlcnNpdHkuZWR1
Disclaimer: All claims expressed in this article are solely those of the authors and do not necessarily represent those of their affiliated organizations, or those of the publisher, the editors and the reviewers. Any product that may be evaluated in this article or claim that may be made by its manufacturer is not guaranteed or endorsed by the publisher.
Research integrity at Frontiers
Learn more about the work of our research integrity team to safeguard the quality of each article we publish.