- 1Institute of Psychology, University of Graz, Graz, Austria
- 2Institute of Education and Psychology at Szombathely, Eötvös Loránd University, Budapest, Hungary
- 3Department of Cognitive Science, Budapest University of Technology and Economics, Budapest, Hungary
- 4MTA-BME Momentum Language Acquisition Research Group, Eötvös Loránd Research Network (ELKH), Budapest, Hungary
Purpose: Studies on the interface between statistical learning and language are dominated by its role in word segmentation and association with grammar skills, while research on its role in lexical development is scarce. The current study is aimed at exploring whether and how statistical learning and verbal short-term memory are associated with lexical skills in typically developing German-speaker primary school children (Experiment 1) and Hungarian-speaking children with developmental language disorder (DLD, Experiment 2).
Methods: We used the language-relevant Peabody Picture Vocabulary Tests to measure individual differences in vocabulary. Statistical learning skills were assessed with the Weather Prediction task, in which participants learn probabilistic cue-outcome associations based on item-based feedback. Verbal short-term memory span was assessed with the Forward digit span task.
Results: Hierarchical linear regression modelling was used to test the contribution of different functions to vocabulary size. In TD children, statistical learning skills had an independent contribution to vocabulary size over and above age, receptive grammatical abilities and short-term memory, whereas working memory did not have an independent contribution. The pattern was reverse in SLI: Vocabulary size was predicted by short-term memory skills over and above age, receptive grammar and statistical learning, whereas statistical learning had no independent contribution.
Conclusion: Our results suggest that lexical development rely on different underlying memory processes in typical development and in developmental language disorder to different degrees. This qualitative difference is discussed in the light of different stages of lexical development, as well as the contribution of the different human memory systems to vocabulary acquisition.
Introduction
Our environment serves as a rich source of statistical information. We face a huge number of incoming stimuli, some of which are random, but most of them are organized by an underlying pattern. These patterns are not always easy to detect. This process of pattern extraction is supported by a domain general learning mechanism: statistical learning, which is the ability of identifying structure and patterns based on distributional environmental information (Saffran et al., 1996; Frost et al., 2015). Statistical learning has been associated with a wide variety of cognitive skills and mechanisms, including reading (Arciuli and Simpson, 2012; Schmalz et al., 2019), language development (Weiss et al., 2010; Hsu and Bishop, 2014), language processing (Conway et al., 2010), computational thinking (Kandlhofer et al., 2019), music cognition (Pearce, 2018) and numerical cognition (Levy et al., 2020). The current study focuses on the role of statistical learning in lexical development, and tests how statistical learning and verbal short term memory skills contribute to vocabulary knowledge in typically developing children (Experiment 1) and children with developmental language disorder (Experiment 2).
The contribution of statistical learning to language acquisition has always been among the key topics of research within the field of SL. The first study to use the concept of statistical learning was developed to understand what role linguistic experience plays in word segmentation (Saffran et al., 1996). Later research diverged into various directions both within lexical and grammatical acquisition. Studies addressed how adjacent and non-adjacent dependencies (Uddén et al., 2012; Gervain and Werker, 2013; Hsu et al., 2014) are acquired, how specific structural information is learned (Wonnacott et al., 2008; Wonnacott, 2011) vs. how general patterns are extracted from repeated exposure (Friederici et al., 2006; Saffran, 2002; for a review see; Gomez and Gerken, 2000).
Lexical acquisition relies on distributional information and is supported by statistical learning on many levels. It is important in reorganizing the initial phoneme space of infants to fit the phoneme inventory of their mother tongue (e.g., Kuhl, 2000; Werker and Curtin, 2005), which is a prerequisite of effective word learning. The cornerstone study motivating a wealth of later research on segmentation by Saffran et al. (1996) tested whether babies can identify word boundaries in continuous sound streams based on statistical information alone: Differences between transitional probabilities between syllables within words vs. across word boundaries. The extraction of language specific distributions of phonemes (phonotactics) also supports segmentation (e.g., Jusczyk et al., 1994). Beyond establishing word boundaries, distributional information is also useful in mapping words onto their referents and in resolving referential ambiguity. There are usually multiple candidates available in the environment for a novel word, and repeated exposure with cross situational correlations between words and referents is required to resolve ambiguity (Yu and Smith, 2007; Scott and Fisher, 2012; Monaghan et al., 2015; Rebuschat et al., 2021).
The seminal word segmentation task by Saffran et al. (1996) modelled how infants extract word-like units from speech. In this task, participants (infants, children and adults, see e.g., Aslin et al., 1999) are exposed to a continuous stream of CV syllables (composed of a consonant and a vowel). Unknown to the participants, the syllables are ordered into triplets. Within a triplet the probability of syllable transition is high, whereas between-triplet transitions have a low probability. Infants are able to differentiate between triplet units (with both transitions being high) and triplet fragments (one transition being high, the other one low, Saffran et al., 1996).
Beyond the extraction of word boundaries, SL also operates in establishing object-label mappings, as demonstrated by studies of using the cross-situational word learning paradigm. In cross-situational word learning, participants are exposed to a number of novel objects and their corresponding labels at the same time, without any explicit mapping between objects and labels. After providing all labels, a new set of objects is presented with new labels. Participants should identify which label corresponds to which object across a number of sets, in which the same object-label pair is provided along with different other objects and labels. This type of word learning mechanism has been shown to operate in infants (Yu and Smith, 2007; Smith and Yu, 2008, 2013), children (Suanda et al., 2014) and adults as well (Yu and Smith, 2007; Vouloumanos, 2008).
Few studies addressed the role of statistical learning plays in vocabulary growth, and most of them had a different primary focus, and were only tangentially related to our research question. A modelling study by Yu (2008) examined how lexical knowledge at 2 years of age contributes to later statistical vocabulary learning. Results showed that the more words a child stores in their vocabulary, the easier to utilize statistical learning in further vocabulary acquisition. That is, while statistical learning in fact contributed to vocabulary acquisition, this effect was moderated by vocabulary knowledge. A further large scale study by Spencer and colleagues (Spencer et al., 2015) addressed how statistical learning on a word segmentation task is related to written language skills in 4–10-year-old children. While they primarily focused on literacy skills, most relevant to the current study, word segmentation was associated with several vocabulary measures.
Along with statistical learning, comprehensive models of vocabulary acquisition also emphasize the role of other skills such as short-term memory and categorization in the acquisition of lexical knowledge (Tomasello, 2000; Hirsch et al., 2016). In the following section, we will provide a brief overview of how these cognitive abilities contribute to lexical development.
The associations between verbal-short term memory capacity and early language acquisition are well-documented (Adams and Gathercole, 1995, 2000; Adams, 1996; Baddeley et al., 1998). Gathercole and Baddeley (1993) specifically argued that the main function of the phonological loop is to store new phonological forms for learning new words. In line with this proposal, vocabulary development has been found to be associated with verbal short-term memory in both monolingual and bilingual children (Masoura and Gathercole, 2005; Gathercole, 2006; Verhagen and Leseman, 2016). The significance of verbal short term and working memory deficits in developmental language disorder as clinical markers is also well known (Gathercole and Baddeley, 1990; Bishop et al., 1996; Botting and Conti‐Ramsden, 2001; Conti-Ramsden et al., 2001).
Category learning is important in every aspect of language learning from the acquisition of speech sound categories through the emergence of syntactic categories to forming conceptual categories and labelling them. In many cases, categorisation processes are probabilistic and rely on statistical information available to the learner. Previous studies have addressed how categorization is associated with vocabulary growth and found that both the knowledge of (Borovsky et al., 2016), and the interest in (Ackermann et al., 2020) the category domain help learning new words related to the given category. Individual differences in the early spurt of vocabulary growth have been linked with differences in the appearance of toddlers’ ability of exhaustive sorting of objects into categories (Gopnik and Meltzoff, 1987). Similarly, categorization performance has been found to predict later vocabulary in infants (Ferguson et al., 2015).
In this paper we will focus on how statistical category learning contributes to vocabulary knowledge. As discussed above, there is ample evidence for the association between developmental language disorder and verbal short-term memory. This strong evidence is complemented with sporadic evidence suggesting positive (Andrade and Baddeley, 2011) as well as negative associations (Virag et al., 2015; Conway, 2020) between statistical learning and short-term memory/working memory. Due to the inconsistency of the findings, we extended our focus to the relative contribution of statistical category learning and short-term memory to vocabulary knowledge in typically developing school-age children and in children with developmental language disorder.
To this end we used the Weather Prediction Task, which is a probabilistic category learning paradigm (Knowlton et al., 1994; Lukács and Kemény, 2015). In this task, participants are exposed to various combinations of geometric shapes and have to decide whether they predict sunshine or rain. At the beginning of the task, participants are unaware that each cue is associated with one of the outcomes. Two of the cues have a strong predictive value (being associated with one of the outcomes in 85.7% of all cases), the other two are weaker in prediction (leading to one outcome in 70% of the cases). Participants are not informed about the distributional nature of the task, but receive feedback after each decision. To achieve optimal performance, participants not only have to identify the association between each cue and outcome, but also have to cumulate the predictive values of the cues that are present at the same time. We expect to see that statistical learning efficiency on the Weather Prediction Tasks would be associated with individual variance in vocabulary size over and above age, grammar skills, fluid intelligence and verbal short-term memory. This association is tracked in typically developing primary school children (Experiment 1) and children with Developmental Language Disorder (Experiment 2).
Experiment 1
Methods
Participants
Altogether 50 children participated in Experiment 1, three children were later excluded from the analyses due to missing data, resulting in a pool of 47 participants, whose data was considered in the analyses. Descriptive statistics of the participants are provided in Table 1. All children had German as their native language and were considered monolingual. Children were recruited from first and third grades of primary schools in and around Graz, Austria. All children were tested individually in their schools. Parents of children provided a written informed consent in accordance with the stipulations of the Institutional Ethical Committee, as well as the Declaration of Helsinki.
Procedure
All children were tested individually in quiet rooms in their schools. Tasks were administered in a random order in a single session that lasted for a maximum of 1 h. Children could have a self-paced break between the tasks.
Tasks
Vocabulary. We used a standardized German measure of the Peabody Picture Vocabulary Test (PPVT-4, Lenhard et al., 2015) to assess the vocabulary knowledge of children. In the test children see four pictures while hear a single word. They have to point at the picture that matches the given word. We used the raw score of the PPVT-4 which is the number of the last correctly responded item minus the number of errors.
Statistical Learning. The short version of the Weather Prediction Task (Gluck et al., 2002; Kemény and Lukács, 2010) was used as a measure of statistical learning. The Weather Prediction task is a probabilistic categorization paradigm. Participants see one, two or three out of four possible stimuli, and have to decide whether it would be sunshine or rain. Cues are simple geometric shapes: a square, a triangle, a pentagon and a rhombus. Since these geometric shapes have no general common associations to weather conditions, participants are initially expected to guess. Immediately after their choice, the correct outcome is revealed (that is, whether it was sun or rain). Unknown to the participants, each stimulus has a pre-set probability with which it is associated with the outcome. Cue1 (square) predicts sunshine in 85.7% of all its appearances, Cue2 (triangle) in 70%, Cue3 (pentagon) in 30%, Cue4 (rhombus) in 14.3%. In all other cases, the cue is associated with rain. Participants are not informed about the predictive values, they are expected to learn these contingencies based on feedback. Participants are exposed to one block of 50 items. The association of items and outcome probabilities are provided in Table 2. Statistical learning performance is characterized by the percentage of items with correct answers, i.e., items in which the participants chose the more probable outcome.
Verbal Short-Term Memory (STM). We used a Digit Span task to assess verbal STM (Racsmány et al., 2005). Participants heard a sequence of numbers and had to repeat the numbers in the same order. Numbers in the sequence were presented at a rate of one number per second. At the beginning of the task, sequences were composed of three items, and increased in length by blocks. For each length, there were four sequences presented in a block. If a participant successfully repeated at least half of the sequences (i.e., two out of four), the task continued with increased sequence length. Verbal STM span is characterized by the highest sequence length the participant was able to repeat.
Grammar Skills. Since previous studies have shown that 1) statistical learning is associated with grammar skills (Misyak and Christiansen, 2012; Kidd and Arciuli, 2016), 2) syntactic information supports meaning acquisition (Fisher et al., 1994) and 3) lexical and grammatical development are closely related (Dale et al., 2000; Moyle et al., 2007; but see; Brinchmann et al., 2019), we decided to control for grammar skills while examining the association of vocabulary and statistical learning. We used the standardized German version of the Test for the Reception of Grammar (TROG-D, Fox, 2016) to assess grammar skills. Like in the PPVT-4, children see four pictures, hear one utterance, and have to match the utterance with one of the pictures. The TROG-D is composed of 21 blocks of increasing syntactic complexity with each block containing four items. Completion of a block is considered successful if the participant responds correctly to at least three out of four items are correctly solved. We used the raw score number of the correctly solved successful blocks as the measure of grammar skills.
Fluid Intelligence. Vocabulary is a part of crystallized intelligence (Ullstadius et al., 2002; Kaufman et al., 2015), and fluid intelligence is argued to serve as a basis for crystallized intelligence (Cattell, 1987; Rindermann et al., 2010). Consequently, we also included fluid intelligence on Raven’s Colored Matrices (Raven et al., 1987) as a control variable. Participants are exposed to a picture with a missing part. There are six possibilities, and participants have to choose by pointing at the correct one. There are altogether 36 items, we used the raw score, which is the number of correct responses.
Results
First, we tested whether the experimental group performed above chance on the statistical learning task. We conducted a one-sample T-test on the mean statistical learning performance with 0.5 as target value. The mean score of the group (60.64%, Sd 14.06%) was significantly above chance level (50%), t (46) = 5.186, p < 0.001.
Second, we correlated the target (Statistical learning performance and Verbal Short-Term Memory measure) and control variables (Age, Grammar skills and Fluid intelligence) with the vocabulary measure. Details of the correlations are provided in Table 3. The Vocabulary measure correlated significantly with Statistical learning, r (46) = 0.421, p = 0.003, but did not correlate with Verbal Short-term Memory, r (46) = 0.136, p = 0.362. In terms of the control measures, Vocabulary correlated significantly with age, r (46) = 0.531, p < 0.001, Grammar skills, r (46) = 0.546, p < 0.001, and Fluid intelligence, r (47) = 0.467, p < 0.001.
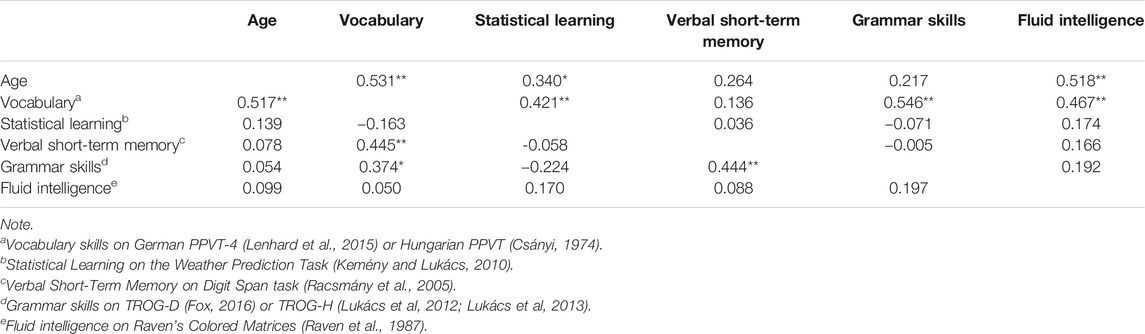
TABLE 3. Bivariate correlation between variables. Results from the typically developing children of Experiment 1 are provided above the diagonal, results from children with developmental language disorder of Experiment 2 are provided below the diagonal.
Third, we assessed the individual contribution of statistical learning and verbal short-term memory to vocabulary measures over and above age, grammar skills and fluid intelligence. We have conducted two hierarchical linear regressions, both with vocabulary as dependent variable. In the first regression analysis, we entered the control variables of Age, Fluid Intelligence and Grammar Skills as well as Verbal STM in Step 1, and additionally Statistical Learning in Step 2. The two models are compared with an analysis of the R2 change, which provides the individual contribution of Statistical Learning to vocabulary over and above the controlled variables. In the second regression analysis we tested the individual contribution of Verbal STM to vocabulary. We entered the control measures and statistical learning in Step 1, and Verbal STM in Step 2. Then we report the analysis of the R2 change. Details of the regression models and coefficients are presented in Table 4.
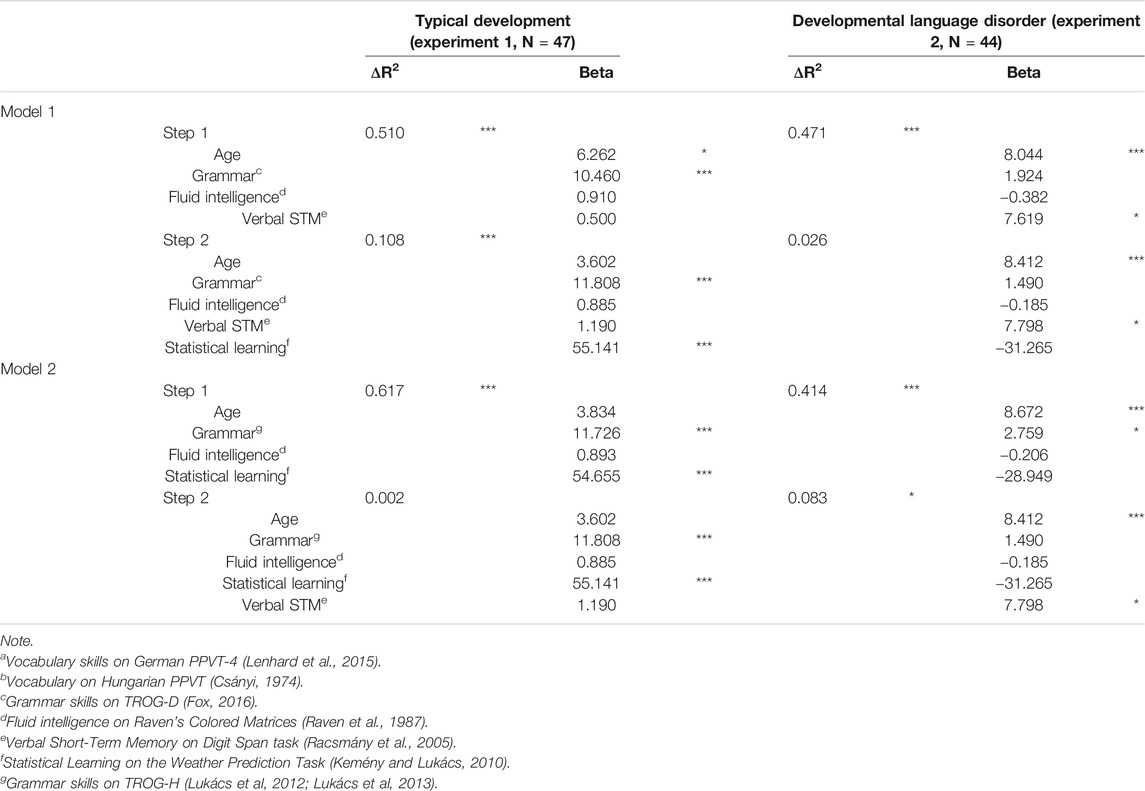
TABLE 4. Results of the hierarchical linear regressions predicting Vocabulary in Experiments 1a and 2b.
Statistical Learning and Vocabulary. Most importantly, a significant regression equation was found in both steps, F (4,46) = 10.944, p < 0.001 for Step 1, and F (5,46) = 13.311, p < 0.001 for Step 2. There was a significant R2 change in Step 2, F (1,41) = 11.663, p = 0.001, ΔR2 = 10.8. The coefficients of Grammar skills and Statistical learning were significant in Step 2 model, t (46) = 4.871, p < 0.001 ß = 11.808 for Grammar skills and t (46) = 3.415, p = 0.001 ß = 55.141 for Statistical Learning. Verbal STM was not a significant predictor, t (46) = 0.421, p = 0.676, ß = 1.190.
Verbal STM and Vocabulary. A significant regression equation was found in both steps, F (4,46) = 16.926, p < 0.001 for Step 1 and F (5,46) = 13.311, p < 0.001 for Step 2. The R2 change in Step 2 was not significant, F (1,41) = 0.177, p = 0.676, ΔR2 = 0.002. Coefficients of Step 2 are identical to those of the previous analysis.
Discussion
Experiment 1 suggests that statistical learning abilities, more specifically, probabilistic categorization skills are significant factors in vocabulary development, explaining 10.8% of additional variance in differences in receptive vocabulary over and above age, grammar skills, fluid intelligence and verbal short-term memory. On the other hand, verbal STM did not have an independent contribution to predicting differences in receptive vocabulary in school-age typically developing children. These results provide evidence that statistical learning, more specifically probabilistic categorization is an important factor in lexical development. This is in line with previous suggestions reviewed in the introduction about the relationship between word learning and statistical learning: that is, objects and their labels are associated numerous times, however, noise and incongruent mappings have to be discounted for (e.g., Roembke et al., 2018).
Verbal short-term memory did not affect vocabulary size in our study. This is in contrast with previous findings in the literature on the role of VSTM in language development. Previous studies observed both an association between verbal short-term memory and vocabulary (Baddeley et al., 1998), as well as verbal short-term memory and statistical learning (e.g., Misyak and Christiansen, 2012). A possible explanation is a methodological one. VSTM spans may not have enough variance when it comes to individual differences. The small variance may account for the lack of association with other skills. However, this is unlikely, since span tasks have generally been developed to reflect individual differences (Daneman and Carpenter, 1980; Engle et al., 1992).
The lack of association between verbal short-term memory and statistical learning is also in contrast with previous findings, but that can be explained by the choice of tasks. On the one hand, most studies used phonological short-term memory as a measure of verbal STM (among others: Andrade and Baddeley, 2011; Gathercole and Baddeley, 1990; Masoura and Gathercole, 2005). The use of the digit span task may explain the lack of association. This task loads more on semantic/declarative information instead of phonological processes. On the other hand, we also used a non-typical task to assess statistical learning. Although the Weather Prediction task clearly relies on distributional information, it has not been widely used as a statistical learning task. Most statistical learning tasks focus on the acquisition of sequential information, where the frequencies of transitions between elements are central (e.g., Saffran et al., 1996). Misyak and Christiansen (2012) examined the association between statistical learning, working and short-term memory and language learning, and found only statistical learning of adjacent dependencies to be related to verbal short-term memory. No association was observed with statistical learning of non-adjacent dependencies. That is, even for sequential learning, task characteristics had an important impact on the association with short-term memory.
A number of previous neuropsychological studies have used the Weather Prediction task to assess learning in patients with brain injury (Knowlton et al., 1994; Hopkins et al., 2004). A number of these studies focused on patients with anterograde amnesia, that is, patients with a difficulty of acquiring new knowledge (Knowlton et al., 1994; Knowlton et al., 1996). Results of more studies have shown that despite a serious deficit in verbal short-term memory, amnesic patients with a deficit of the mediotemporal lobe performed identical to control participants (Knowlton et al., 1994; Knowlton and Squire, 1993; but see; Hopkins et al., 2004; Zaki, 2005). Such neuropsychological studies argue for the independence of short-term memory functions and statistical learning performance, at least in the case of the Weather Prediction task. Despite the use of a non-sequential statistical learning task that is relatively independent of verbal short-term memory, we still found a positive association with language measures.
Overall, Experiment 1 suggests that statistical learning on a probabilistic category learning task is associated with vocabulary. The aim of Experiment 2 is to assess this association in developmental language disorder, as both statistical learning and vocabulary measures are reduced in this population.
Experiment 2
Studying cognitive functions in atypical linguistic development can also contribute to our understanding of the cognitive bases of linguistic competence. Developmental Language Disorder is a neuro-developmental disorder with below age-level spoken language comprehension and expression (Leonard, 2014; McGregor et al., 2020), while other cognitive functions are relatively spared (Leonard, 2014), developmental language disorder shows highly comorbidity with reading impairment and some other developmental disorders (Young et al., 2002), such as developmental coordination disorder (Beitchman et al., 1996) and ADHD (Hill, 2001). A number of theoretical accounts have been proposed to explain the core deficit underlying DLD. These explanations range from specific language impairments to domain-general deficits explaining both the core linguistic problem as well as co-occuring problems in other domains (Ullman and Pierpont, 2005; Leonard, 2014). In the current study we focus on two accounts that suggest core deficits in phonological working memory (Gathercole and Baddeley, 1990) and statistical learning (Evans et al., 2009).
Phonological working memory was among the first candidates to explain linguistic deficits (Gathercole and Baddeley, 1990). Children with developmental language disorder have significantly shorter spans than their typically developing peers. Phonological working memory was also found to constrain vocabulary acquisition, at least in typical development (Gathercole and Baddeley, 1993). Later studies proposed that short-term or working memory deficits are clinical markers of developmental language disorder (Conti-Ramsden et al., 2001), and they also assumed that reduced STM capacity has a direct role in impaired acquisition of structural aspects of language (Adani et al., 2014; Friedmann et al., 2009; Stavrakaki, 2020; Stavrakaki and Van der Lely, 2010; but see; Bishop, 2006). In accordance, we expect verbal short-term memory to play a stronger role in vocabulary acquisition in developmental language disorder than in typical development.
Similarly, while numerous studies showed a robust statistical learning deficit in children with DLD in different tasks and domains (Gabriel et al., 2012; Hedenius et al., 2011; Hsu et al., 2014; Kemény and Lukács, 2010; Lukács and Kemény, 2014; Lum et al., 2010; Lum et al., 2012, for meta-analyses, see; Lum et al., 2014; Lammertink et al., 2017; Obeid et al., 2016), the focus in most studies was on statistical learning, and results on its association with lexical knowledge are few. One exception is the study by Evans and colleagues (2009), who argue that statistical learning is a central factor in lexical development. They found a positive relationship between vocabulary size and statistical learning abilities (in a word segmentation task) in typically developing children, but no association was observed with the same parameters in developmental language disorder. Association was found, however, when the length of the training was increased (Experiment 2 of Evans et al., 2009). Haebig et al. (2017) tested two key mechanisms of word learning: Statistical learning and fast mapping in children with developmental language disorder, children with Autism Spectrum Disorder, and typically developing children. Children with DLD showed impaired statistical learning performance, and no association between segmentation skills and word learning abilities, while a comparable level of statistical learning was found in ASD and TD, as well as an association between SL and word learning in both groups. In sum, we expect statistical learning to play a smaller role in the vocabulary acquisition of children with developmental language disorder.
Experiment 2 addresses the same questions and uses the same methods as Experiment 1 in a group of monolingual Hungarian-speaking children with Developmental Language Disorder. Based on previous results we expected to see no association of statistical learning and vocabulary size in DLD (in accordance with Evans et al., 2009; Haebig et al., 2017). On the other hand, we expect a stronger association between verbal short-term memory and vocabulary. This expectation is supported by the observation that children with developmental language disorder are slower in their vocabulary acquisition (and in language acquisition more generally), and verbal short-term memory skills may be stronger predictors of lexical development in earlier stages/at smaller vocabulary sizes.
Methods
Participants
Altogether 45 children were included in the Developmental Language Disorder group, one child had to be excluded due to missing data. Descriptive statistics are provided in Table 1. All children had Hungarian as their native language and were considered monolingual. Children were recruited from two special schools for children with language impairment. Children were referred to these groups and classes by speech and language therapists working in clinical practice. In each institution, recruitment took between 2 and 3 months. No eligible children declined participation. All children met inclusive and exclusive criteria for DLD that are standardly used in selecting DLD children in research (Leonard, 1997; Tager-Flusberg, 2000). Each child scored above 85 on the Raven Coloured Progressive Matrices (Raven et al., 1987), a measure of fluid intelligence. No child had a hearing impairment or a history of neurological impairment. No children in the SLI group had any known comorbidities. Each child scored at least 1.25 SDs below age norms on at least two of four language tests administered. The four tests included two receptive tests: the Hungarian standardizations of the Peabody Picture Vocabulary Test (PPVT, Csányi, 1974) and the Test for Reception of Grammar (TROG-H, Lukács et al., 2012; Lukács et al., 2013) and two expressive tests: the Hungarian Sentence Repetition Test (Magyar Mondatutánmondási Teszt, MAMUT, Kas and Lukács, 2011), and a nonword repetition test (Racsmány et al., 2005). Table 5 provides further information about the tests on which children with DLD were significantly below age expectations. All children were tested with the informed consent of their parents, in accordance with the principles set out in the Declaration of Helsinki and the stipulations of the local Institutional Review Board.
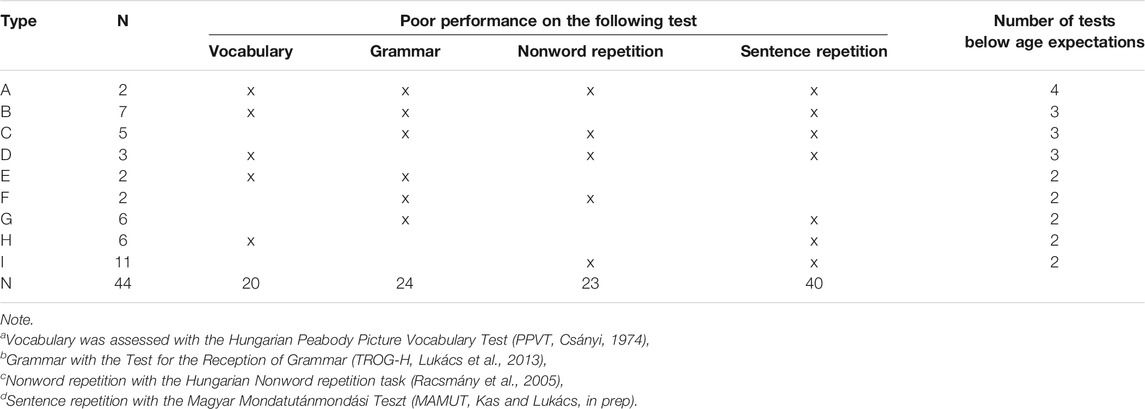
TABLE 5. Description of the DLD group. Participants scored 1.25 SD below average on at least two of four language tests. Each line provides a unique constellation of poor performance patterns across tests together with the number of participants showing the pattern, and the number of tests. The final line provides the sum of participants performing poorly on each of the tests.
Methods and Procedure
Methods and procedure were equivalent to those of Experiment 1, however, Hungarian language tests were used. We used the Hungarian adaptation of the Test for the Reception of Grammar (Lukács et al., 2012) as well as the Hungarian version of the Peabody Picture Vocabulary Test (Csányi, 1974). The tasks, procedures and calculation of raw scores are identical despite language differences.
Results
Similar to experiment 1, we first conducted a one-sample T-test on Statistical learning performance (dependent variable) with 50% (chance level) as target value. Children with DLD scored significantly above chance, t (43) = 4.245, p < 0.001, showing a mean performance of 57.13% with a standard deviation of 11.27%.
We computed bivariate correlations between vocabulary and target variables (Statistical learning and Verbal STM), as well as vocabulary and control variables (Age, Grammar skills and Fluid intelligence). Details of correlations are provided in Table 3. Vocabulary correlated significantly with verbal short-term memory, r (43) = 0.445, p = 0.002, but not with statistical learning, r (43) = −0.165, p = 0.287. Considering the control variables, vocabulary correlated significantly with age, r (43) = 0.509, p < 0.001, grammar skills, r (43) = 0.374, p = 0.012, but not with fluid intelligence, r (43) = −0.050, p = 0.746.
Statistical Learning and Vocabulary. As in Experiment 1, we used hierarchical linear regression with Vocabulary as dependent variable. Age, Fluid Intelligence, Grammar Skills and Verbal STM were entered in Step 1 and Statistical learning was additionally entered in Step 2. Details of the hierarchical regression are provided in Table 4. Both steps resulted in significant models, F (4,43) = 8.682, p < 0.001 for Step 1 and F (5,43) = 7.502, p < 0.001 for Step 2. Unlike in Experiment 1, entering statistical learning scores did not significantly increase the explained variance of in vocabulary measures, F (1,38) = 1.941, p = 0.172, ΔR2 = 0.026. Age and Verbal STM were significant coefficients of the Step 2 model, t (43) = 4.352, p < 0.001, ß = 8.412 for Age and t (43) = 2.503, p = 0.017, ß = 7.798 for Verbal STM.
Verbal STM and Vocabulary. We conducted a hierarchical linear regression analysis with Vocabulary as dependent variable. Age, Fluid Intelligence, Grammar Skills and Statistical Learning were entered in Step 1, and Verbal STM in Step 2. Again both steps resulted in significant models, F (4,43) = 6.882, p < 0.001 for Step 1 and F (5,43) = 7.502, p < 0.001 for Step 2. Unlike Experiment 1, entering Verbal STM significantly increased the explained variance of vocabulary, F (1,38) = 6.264, p = 0.017, ΔR2 = 0.083.
General Discussion
The current study investigated the contribution of statistical categorization abilities and verbal short-term memory to vocabulary knowledge. Statistical learning was tested with a probabilistic category learning task, the Weather Prediction task (Knowlton et al., 1994). Forward digit span was used as a measure of verbal short-term memory. Both factors have been suggested to contribute significantly to vocabulary growth, and both have been shown to be impaired in developmental language disorder. We expected statistical learning in probabilistic categorization to play an important role in vocabulary acquisition in typically developing children, whereas a greater contribution of verbal short-term memory was expected in language impaired population, due to their slower pace of vocabulary development.
These hypotheses were only partially supported by our results. These suggest that statistical learning plays an important role in typical lexical development, explaining over 10% of the variance in receptive vocabulary scores. This contribution was observed after controlling for the effects of grammar skills and fluid intelligence. In contrast, verbal short-term memory was not associated with vocabulary measures in typically developing children. Children with developmental language disorder showed the reverse pattern. There was no effect of statistical learning, whereas verbal short-term memory explained more than 8% of the variance in vocabulary knowledge.
The first and most important result is that statistical learning plays an important role in vocabulary development, at least in typical development. This result supports and extends previous findings on the importance of different forms of statistical learning in lexical acquisition, even with the use of probabilistic categorization. This type of learning has features analogous to cross-situational learning where participants have to identify word-object mappings across several trials, and similar learning has been observed across different age-groups (Yu and Smith, 2007; Smith and Yu, 2008, 2013; Vouloumanos, 2008; Suanda and Namy, 2012; Suanda et al., 2014; Roembke et al., 2018). One study examined specifically how cross-situational statistical word learning is affected by reduced working memory resources (Roembke and McMurray, 2021), which were modelled with a dual-task setting, resulting in lower word learning performance. Although the reported performance decrease was small, this finding is an important step towards understanding how statistical word learning may be affected in developmental language disorder, a clinical population with reduced working memory. While a reduced working memory span may be an important factor in explaining smaller vocabulary sizes and lower rates of lexical development in DLD, we found no association between this form of statistical learning and working memory in either of the experiments, arguing for differential effects of different forms of statistical learning to vocabulary.
It is also important to note that the novelty of the current study was to rely on a statistical learning task, which primarily focuses on categorization instead of the typical word segmentation task (Saffran et al., 1996). We used the Weather Prediction task (Knowlton et al., 1994; Kemény and Lukács, 2013), which is a probabilistic category learning task. The task has traditionally been considered to tax procedural memory functions, and shows intact performance in amnesia (Knowlton et al., 1994, 1996; but see; Zaki, 2005; Lagnado et al., 2006; Newell et al., 2007; Kemény and Lukács, 2013; Kemény, 2014), which suggests independence of this form of statistical learning from short-term memory functions. Similar to this dissociation, the relation between statistical learning and vocabulary measures may also depend on the choice of the task. This is in line with previous studies showing low correlations even between different versions of the same statistical learning tasks (Siegelman et al., 2017a; Siegelman et al., 2017b).
We observed a different pattern of associations between skills in DLD, where vocabulary acquisition showed a stronger association with short-term memory capacity. These results are in contrast with the wealth of previous studies highlighting the role of verbal short-term memory in vocabulary acquisition. A potential explanation for the failure to observe such a connection in our TD group lies in the age of participants: The association between verbal STM and vocabulary may be especially strong at the beginning and earlier stages of lexical acquisition, and indeed, previous studies reported significant associations in younger children (Masoura & Gathercole, 2005; Ferguson et al., 2015; Verhagen & Leseman, 2016). The strength of the association may decrease with age and/or it may also decrease with the growth of the lexicon, which could also account for the presence of such an association in the DLD group. Developmental Language Disorder is often characterized by slower linguistic development (Leonard, 1997; but see; Larkin et al., 2013). If children with DLD lag behind their typically developing peers in their linguistic development, one could argue that the observed association between verbal short-term memory and vocabulary measures is characteristic of TD language development at the DLD groups’ language age. Children with DLD show a pattern of language and cognitive abilities of younger TD children in the developmental phase where lexical development relies more heavily on verbal short-term memory. This hypothesis is supported by the fact that 20 of the children with DLD scored at least 1.25 SD below their age expectations on the PPVT.
Since the probabilistic categorization task tests procedural learning (Knowlton et al., 1996; but see; Lagnado et al., 2006), with direct comparisons across DLD and typical groups, our design can provide important implications for the Procedural Deficit Hypothesis of Specific Language Impairment (Ullman and Pierpont, 2005) and for the different patterns of cooperation and competition between the memory systems in clinical populations. This hypothesis suggests that language impairment is the consequence of a deficit in domain-general procedural memory functions. Procedural memory is the memory responsible for the acquisition and storage of process-like information, like riding a bike (Squire et al., 1993), categorization (Knowlton et al., 1996) or grammar use (Ullman et al., 1997), and has also been assumed to underlie statistical learning (Cleeremans et al., 1998; Perruchet and Pacton, 2006; Simor et al., 2019). Procedural memory is complemented by Declarative memory, which is responsible for the acquisition and storage of fact-like information, like dates, phone numbers, etc (e.g. Squire et al., 1993). Children with developmental language disorder are assumed to be primarily deficient in their procedural memory functions, and use their declarative memory to compensate for the loss (Ullman and Pullman, 2015).
Since the two groups of the current study differ along several factors, direct comparison of the groups does not allow directly assessing the procedural deficit hypothesis or the declarative compensation. The level of statistical learning performance, however, was above chance in both groups, and only slightly differ from each other. That is, we have no evidence for a general procedural deficit, even if we consider that the clinical group was on average 1 year older than the typical group. Instead, we provide evidence that children with developmental language disorder benefit less from their statistical learning abilities when it comes to vocabulary development. This does not imply that training procedures relying on statistical learning are not beneficial for children with DLD, instead it highlights the importance of focusing on higher exposure and more repetitions of trials with difficult patterns during training (Evans et al., 2009; Plante and Gómez, 2018). Perhaps training in statistical learning would not only enhance core statistical learning abilities, but would also support the utilization of distributional regularities within the linguistic domain.
Instead of statistical learning, children with DLD rely more on verbal short-term memory. While one could argue that since verbal STM is the input of the declarative memory system (Blumenfeld and Ranganath, 2007), such a result might reflect mediation by declarative compensation, such a suggestion should be handled with care. While statistical learning was comparable across the two groups, verbal STM is clearly reduced in developmental language disorder, which is in line with previous assumptions of verbal STM being a marker DLD (Gathercole & Baddeley, 1993). If verbal STM is deficient in DLD, whereas statistical learning is at least not clearly deficient, it could be misleading to assume such reliance being compensatory. However, the association pattern between statistical learning abilities and vocabulary is different in the two groups.
Our study is not without limitations. One of these is the lack of a direct comparison between the clinical and the typical groups: that the typical group of Experiment 1 and the clinical group of Experiment 2 came from different countries, spoke different languages and were not matched on age. This made it impossible to directly compare the two groups on their linguistic skills, verbal short-term memory skills or statistical learning abilities. As a result in this study we could not provide evidence either in favour or against the procedural deficit hypothesis (Ullman & Pierpont, 2005) or the verbal short-term memory deficit (Gathercole & Baddeley, 1993) by comparing the two groups. On the other hand, the aim of the current study was to examine how memory skills contribute to linguistic abilities, which contribution should be relatively independent of the target language, and the analyses themselves should be done separately even if the two groups were matched on age. Our study focused on vocabulary knowledge in school-age children at a relatively late stage of lexical development. It would also be interesting to test the contribution of the same set of skills in younger children, where stronger associations might be expected. Similarly, repeating the study, especially Experiment 1, with more age-groups could provide further insights on how the relative contribution of verbal short-term memory and statistical learning to vocabulary.
A further limitation of the study is that the dependent variable (PPVT) of Experiment 2, as well as one of the control variables (TROG-H) were selection variables for developmental language disorder. As explained above, our research focus was how statistical learning and verbal short-term memory contribute to vocabulary development. Both these skills have been found impaired in developmental language disorder (Evans et al., 2009; Masoura & Gathercole, 2005; Lukács et al., 2016; but see; Lukács & Kemény, 2014). Consequently, performance on these variables is on the lower end of the population’s variance. One might argue that the reduced variability of the measures could lead to invalid results. While it is true in principle, we did not observe lower variability in the DLD group than in the TD group (see Table 1 for Descriptives). Variance in Vocabulary (SD = 21.81 in TD, 20.83 in DLD) and Statistical Learning (14.05% in TD and 11.37% in DLD) is slightly smaller in the clinical group, while variance in Verbal Short-Term Memory is slightly larger in DLD (0.77 in TD, 0.86 in DLD). The difference of variance is considerably larger in the case of Grammar skills, with larger variance in DLD (0.91 in TD and 2.40 in DLD). That is, the similarity of variances would not support a conclusion that the atypical pattern in DLD is due to the use of selection variables. On the other hand, the comparison of a typical (TD) and an extreme group (DLD) was the central aim of the current study, which could not be achieved otherwise.
Data Availability Statement
The raw data supporting the conclusions of this article will be made available by the authors upon request, without undue reservation.
Ethics Statement
The studies involving human participants were reviewed and approved by the Ethics committee of the University of Graz (Experiment 1) and the Ethics committee of the Budapest University of Technology and Economics (Experiment 2). Written informed consent to participate in this study was provided by the participants’ legal guardian/next of kin.
Author Contributions
FK and ÁL contributed equally to the design of the study, data collection and publication. Statistical analyses were conducted by FK.
Funding
This work was supported by the Momentum Research Grant of the Hungarian Academy of Sciences (Momentum 96233 “Profiling learning mechanisms and learners: individual differences from impairments to excellence in statistical learning and in language acquisition,” PI: ÁL). The authors acknowledge the financial support by the University of Graz.
Conflict of Interest
The authors declare that the research was conducted in the absence of any commercial or financial relationships that could be construed as a potential conflict of interest.
Publisher’s Note
All claims expressed in this article are solely those of the authors and do not necessarily represent those of their affiliated organizations, or those of the publisher, the editors and the reviewers. Any product that may be evaluated in this article, or claim that may be made by its manufacturer, is not guaranteed or endorsed by the publisher.
References
Ackermann, L., Hepach, R., and Mani, N. (2020). Children Learn Words Easier when They Are Interested in the Category to Which the Word Belongs. Developmental Sci. 23 (3), e12915. doi:10.1111/desc.12915
Adams, A. M., and Gathercole, S. E. (2000). Limitations in Working Memory: Implications for Language Development. Int. J. Lang. Commun. Disord. 35 (1), 95–116. doi:10.1080/136828200247278
Adams, A. M., and Gathercole, S. E. (1995). Phonological Working Memory and Speech Production in Preschool Children. J. Speech Lang. Hear. Res. 38 (2), 403–414. doi:10.1044/jshr.3802.403
Adams, A. M., and Gathercole, S. E. (1996). Phonological Working Memory and Spoken Language Development in Young Children. The Q. J. Exp. Psychol. Section A 49 (1), 216–233. doi:10.1080/027249896392874
Adani, F., Forgiarini, M., Guasti, M. T., and Van Der Lely, H. K. J. (2014). Number Dissimilarities Facilitate the Comprehension of Relative Clauses in Children with (Grammatical) Specific Language Impairment. J. Child. Lang. 41 (4), 811–841. doi:10.1017/s0305000913000184
Andrade, J., and Baddeley, A. (2011). The Contribution of Phonological Short-Term Memory to Artificial Grammar Learning. Q. J. Exp. Psychol. 64 (5), 960–974. doi:10.1080/17470218.2010.533440
Arciuli, J., and Simpson, I. C. (2012). Statistical Learning Is Related to Reading Ability in Children and Adults. Cogn. Sci. 36 (2), 286–304. doi:10.1111/j.1551-6709.2011.01200.x
Aslin, R. N., Saffran, J. R., and Newport, E. L. (1999). “Statistical Learning in Linguistic and Nonlinguistic Domains,” in The Emergence of Language. Editor B. MacWhinney (Mahwah, NJ: Lawrence Erlbaum Associates Publishers), 359–380.
Baddeley, A., Gathercole, S., and Papagno, C. (1998). The Phonological Loop as a Language Learning Device. Psychol. Rev. 105 (1), 158–173. doi:10.1037/0033-295x.105.1.158
Beitchman, J. H., Brownlie, E. B., Inglis, A., Wild, J., Ferguson, B., Schachter, D., et al. (1996). Seven-Year Follow-Up of Speech/Language Impaired and Control Children: Psychiatric Outcome. J. Child. Psychol. Psychiat 37 (8), 961–970. doi:10.1111/j.1469-7610.1996.tb01493.x
Bishop, D. V. M., North, T., and Donlan, C. (1996). Nonword Repetition as a Behavioural Marker for Inherited Language Impairment: Evidence from a Twin Study. J. Child. Psychol. Psychiat 37 (4), 391–403. doi:10.1111/j.1469-7610.1996.tb01420.x
Bishop, D. V. M. (2006). What Causes Specific Language Impairment in Children?. Curr. Dir. Psychol. Sci. 15 (5), 217–221. doi:10.1111/j.1467-8721.2006.00439.x
Blumenfeld, R. S., and Ranganath, C. (2007). Prefrontal Cortex and Long-Term Memory Encoding: An Integrative Review of Findings from Neuropsychology and Neuroimaging. Neuroscientist. 13 (1), 280–291. doi:10.1177/1073858407299290
Borovsky, A., Ellis, E. M., Evans, J. L., and Elman, J. L. (2016). Lexical Leverage: Category Knowledge Boosts Real-Time Novel Word Recognition in 2-Year-Olds. Dev. Sci. 19 (6), 918–932. doi:10.1111/desc.12343
Botting, N., and Conti-Ramsden, G. (2001). Non-word Repetition and Language Development in Children with Specific Language Impairment (SLI). Int. J. Lang. Commun. Disord. 36 (4), 421–432. doi:10.1080/13682820110074971
Brinchmann, E. I., Braeken, J., and Lyster, S. A. H. (2019). Is There a Direct Relation between the Development of Vocabulary and Grammar?. Dev. Sci. 22 (1), e12709. doi:10.1111/desc.12709
Cleeremans, A., Destrebecqz, A., and Boyer, M. (1998). Implicit Learning: News from the Front. Trends Cogn. Sci. 2, 406–416. doi:10.1016/s1364-6613(98)01232-7
Conti‐Ramsden, G., Botting, N., and Faragher, B. (2001). Psycholinguistic Markers for Specific Language Impairment (SLI). J. Child Psychol. Psychiatry 42 (6), 741–748. doi:10.1111/1469-7610.00770
Conway, C. M., Bauernschmidt, A., Huang, S. S., and Pisoni, D. B. (2010). Implicit Statistical Learning in Language Processing: Word Predictability Is the Key☆. Cognition 114 (3), 356–371. doi:10.1016/j.cognition.2009.10.009
Conway, C. M. (2020). How Does the Brain Learn Environmental Structure? Ten Core Principles for Understanding the Neurocognitive Mechanisms of Statistical Learning. Neurosci. Biobehavioral Rev. 112, 279–299. doi:10.1016/j.neubiorev.2020.01.032
Dale, P. S., Dionne, G., Eley, T. C., and Plomin, R. (2000). Lexical and Grammatical Development: A Behavioural Genetic Perspective. J. Child. Lang. 27 (3), 619–642. doi:10.1017/s0305000900004281
Daneman, M., and Carpenter, P. A. (1980). Individual Differences in Working Memory and reading. J. Verbal Learn. Verbal Behav. 19 (4), 450–466. doi:10.1016/s0022-5371(80)90312-6
Engle, R. W., Cantor, J., and Carullo, J. J. (1992). Individual Differences in Working Memory and Comprehension: A Test of Four Hypotheses. J. Exp. Psychol. Learn. Mem. Cogn. 18 (5), 972–992. doi:10.1037/0278-7393.18.5.972
Evans, J. L., Saffran, J. R., and Robe-Torres, K. (2009). Statistical Learning in Children with Specific Language Impairment. J. Speech Lang. Hear. Res. 52 (2), 321–335. doi:10.1044/1092-4388(2009/07-018910.1044/1092-4388(2009/07-0189)
Ferguson, B., Havy, M., and Waxman, S. R. (2015). The Precision of 12-Month-Old Infants’ Link between Language and Categorization Predicts Vocabulary Size at 12 and 18 Months. Front. Psychol. 6, 1319. doi:10.3389/fpsyg.2015.01319
Fisher, C., Hall, D. G., Rakowitz, S., and Gleitman, L. (1994). When it Is Better to Receive Than to Give: Syntactic and Conceptual Constraints on Vocabulary Growth. Lingua 92, 333–375. doi:10.1016/0024-3841(94)90346-8
Fox, A. V. (2016). TROG-D: Test zur Überprüfung des Grammatikverständnisses. 7 edition. Idstein: Schulz-Kirchner.
Friederici, A. D., Bahlmann, J., Heim, S., Schubotz, R. I., and Anwander, A. (2006). The Brain Differentiates Human and Non-human Grammars: Functional Localization and Structural Connectivity. Pnas 103, 2458–2463. doi:10.1073/pnas.0509389103
Friedmann, N., Belletti, A., and Rizzi, L. (2009). Relativized Relatives: Types of Intervention in the Acquisition of A-Bar Dependencies. Lingua 119 (1), 67–88. doi:10.1016/j.lingua.2008.09.002
Frost, R., Armstrong, B. C., Siegelman, N., and Christiansen, M. H. (2015). Domain Generality versus Modality Specificity: The Paradox of Statistical Learning. Trends Cogn. Sci. 19 (3), 117–125. doi:10.1016/j.tics.2014.12.010
Gabriel, A., Stefaniak, N., Maillart, C., Schmitz, X., and Meulemans, T. (2012). Procedural Visual Learning in Children with Specific Language Impairment. Am. J. Speech Lang. Pathol. 21 (4), 329–341. doi:10.1044/1058-0360(2012/11-0044)
Gathercole, S. E., and Baddeley, A. D. (1990). Phonological Memory Deficits in Language Disordered Children: Is There a Causal Connection?. J. Mem. Lang. 29, 336–360. doi:10.1016/0749-596x(90)90004-j
Gathercole, S. E., and Baddeley, A. D. (1993). Phonological Working Memory: A Critical Building Block for reading Development and Vocabulary Acquisition?. Eur. J. Psychol. Educ. 8 (3), 259–272. doi:10.1007/bf03174081
Gathercole, S. E. (2006). Nonword Repetition and Word Learning: The Nature of the Relationship. Appl. Psycholinguistics 27 (4), 513–543. doi:10.1017/s0142716406060383
Gervain, J., and Werker, J. F. (2013). Learning Non-adjacent Regularities at Age 0 ; 7. J. Child. Lang. 40 (4), 860–872. doi:10.1017/S0305000912000256
Gluck, M. A., Shohamy, D., and Myers, C. (2002). How Do People Solve the “Weather Prediction” Task?: Individual Variability in Strategies for Probabilistic Category Learning. Learn. Mem. 9, 408–418. doi:10.1101/lm.45202
Gómez, R. L., and Gerken, L. (2000). Infant Artificial Language Learning and Language Acquisition. Trends Cogn. Sci. 4, 178–186. doi:10.1016/s1364-6613(00)01467-4
Gopnik, A., and Meltzoff, A. (1987). The Development of Categorization in the Second Year and its Relation to Other Cognitive and Linguistic Developments. Child. Development 58, 1523–1531. doi:10.2307/1130692
Haebig, E., Saffran, J. R., and Ellis Weismer, S. (2017). Statistical Word Learning in Children with Autism Spectrum Disorder and Specific Language Impairment. J. Child. Psychol. Psychiatr. 58 (11), 1251–1263. doi:10.1111/jcpp.12734
Hedenius, M., Persson, J., Tremblay, A., Adi-Japha, E., Veríssimo, J., Dye, C. D., et al. (2011). Grammar Predicts Procedural Learning and Consolidation Deficits in Children with Specific Language Impairment. Res. Developmental Disabilities 32 (6), 2362–2375. doi:10.1016/j.ridd.2011.07.026
Hill, E. L. (2001). Non-specific Nature of Specific Language Impairment: A Review of the Literature with Regard to Concomitant Motor Impairments. Int. J. Lang. Commun. Disord. 36, 149–171. doi:10.1080/13682820010019874
Hirsch, L., Jette, N., Frolkis, A., Steeves, T., and Pringsheim, T. (2016). The Incidence of Parkinson's Disease: A Systematic Review and Meta-Analysis. Neuroepidemiology 46 (4), 292–300. doi:10.1159/000445751
Hopkins, R., Myers, C. E., Shohamy, D., Grossman, S., and Gluck, M. (2004). Impaired Probabilistic Category Learning in Hypoxic Subjects with Hippocampal Damage. Neuropsychologia 42, 524–535. doi:10.1016/j.neuropsychologia.2003.09.005
Hsu, H. J., and Bishop, D. V. M. (2014). Sequence-specific Procedural Learning Deficits in Children with Specific Language Impairment. Developmental Sci. 17 (3), 352–365. doi:10.1111/desc.12125
Hsu, H. J., Tomblin, J. B., and Christiansen, M. H. (2014). Impaired Statistical Learning of Non-adjacent Dependencies in Adolescents with Specific Language Impairment. Front. Psychol. 5, 175. doi:10.3389/fpsyg.2014.00175
Jusczyk, P. W., Luce, P. A., and Charles-Luce, J. (1994). Infants′ Sensitivity to Phonotactic Patterns in the Native Language. J. Mem. Lang. 33 (5), 630–645. doi:10.1006/jmla.1994.1030
Kandlhofer, M., Steinbauer, G., Menzinger, M., Halatschek, R., Kemény, F., and Landerl, K. (2019). “MINT-robo: Empowering Gifted High School Students with Robotics,” in IEEE Frontiers in Education Conference (FIE) (IEEE). 1–5. doi:10.1109/fie43999.2019.9028478
Kas, B., and Lukács, Á. (2011). Magyar Mondatutánmondási Teszt Budapest: Magyar Mondatutánmondási Teszt.
Kaufman, A. S., Raiford, S. E., and Coalson, D. L. (2015). Intelligent Testing with the WISC-V. Hoboken, NJ: John Wiley & Sons.
Kemény, F., and Lukács, Á. (2010). Impaired Procedural Learning in Language Impairment: Results from Probabilistic Categorization. J. Clin. Exp. Neuropsychol. 32, 249–258. doi:10.1080/13803390902971131
Kemény, F., and Lukács, Á. (2013). Stimulus Dependence in Probabilistic Category Learning. Acta Psychologica 143 (1), 58–64. doi:10.1016/j.actpsy.2013.02.008
Kemény, F. (2014). Self-insight in Probabilistic Categorization – Not Implicit in Children Either. Front. Psychol. 5, 233. doi:10.3389/fpsyg.2014.00233
Kidd, E., and Arciuli, J. (2016). Individual Differences in Statistical Learning Predict Children's Comprehension of Syntax. Child. Dev. 87 (1), 184–193. doi:10.1111/cdev.12461
Knowlton, B. J., Squire, L. R., and Gluck, M. A. (1994). Probabilistic Classification Learning in Amnesia. Learn. Mem. 1, 106–120. doi:10.1101/lm.1.2.106
Knowlton, B. J., Mangels, J. A., and Squire, L. R. (1996). A Neostriatal Habit Learning System in Humans. Science 273, 1399–1402. doi:10.1126/science.273.5280.1399
Knowlton, B., and Squire, L. (1993). The Learning of Categories: Parallel Brain Systems for Item Memory and Category Knowledge. Science 262, 1747–1749. doi:10.1126/science.8259522
Kuhl, P. K. (2000). A New View of Language Acquisition. Proc. Natl. Acad. Sci. 97 (22), 11850–11857. doi:10.1073/pnas.97.22.11850
Lagnado, D. A., Newell, B. R., Kahan, S., and Shanks, D. R. (2006). Insight and Strategy in Multiple-Cue Learning. J. Exp. Psychol. Gen. 135, 162–183. doi:10.1037/0096-3445.135.2.162
Lammertink, I., Boersma, P., Wijnen, F., and Rispens, J. (2017). Statistical Learning in Specific Language Impairment: A Meta-Analysis. J. Speech Lang. Hear. Res. 60 (12), 3474–3486. doi:10.1044/2017_JSLHR-L-16-0439
Larkin, R. F., Williams, G. J., and Blaggan, S. (2013). Delay or Deficit? Spelling Processes in Children with Specific Language Impairment. J. Commun. Disord. 46 (5–6), 401–412. doi:10.1016/j.jcomdis.2013.07.003
Lenhard, A., Lenhard, W., Segerer, R., and Suggate, S. (2015). Peabody Picture Vocabulary Test—Revision 4 (PPVT-4), Deutsche Version. Frankfurt am Main: Pearson Assessment.
Leonard, L. B. (2014). Children with Specific Language Impairment. Cambridge, Mass: MIT press. doi:10.7551/mitpress/9152.001.0001
Levy, S., Turk-Browne, N., and Goldfarb, L. (2020). Impaired Statistical Learning with Mathematical Learning Difficulties. Chicago: PsyArXiv.
Lukács, Á., Győri, M., and Rózsa, S. (2012). A TROG Pszichometriai Jellemzőinek Magyar Vizsgálata, a Normák Kialakítása,” In BISHOP, D. V. M.(2012): TROG-Test for Reception of Grammar. Kézikönyv Budapest: OS Hungary TesztfejlesztőKft.
Lukács, Á., Győri, M., and Rózsa, S. (2013). TROG-H: Új Sztenderdizált Módszer a Nyelvtani Megértés Fejlődésének Vizsgálatára. Gyógypedagógiai Szemle 41 (1), 1–22.
Lukács, Á., and Kemény, F. (2015). Development of Different Forms of Skill Learning throughout the Lifespan. Cogn. Sci. 39 (2), 383–404. doi:10.1111/cogs.12143
Lukács, Á., and Kemény, F. (2014). Domain-general Sequence Learning Deficit in Specific Language Impairment. Neuropsychology 28 (3), 472–483. doi:10.1037/neu0000052
Lukács, Á., Ladányi, E., Fazekas, K., and Kemény, F. (2016). Executive Functions and the Contribution of Short-Term Memory Span in Children with Specific Language Impairment. Neuropsychology 30 (3), 296–303. doi:10.1037/neu0000232
Lum, J. A. G., Conti-Ramsden, G., Morgan, A. T., and Ullman, M. T. (2014). Procedural Learning Deficits in Specific Language Impairment (SLI): A Meta-Analysis of Serial Reaction Time Task Performance. Cortex 51, 1–10. doi:10.1016/j.cortex.2013.10.011
Lum, J. A. G., Conti-Ramsden, G., Page, D., and Ullman, M. T. (2012). Working, Declarative and Procedural Memory in Specific Language Impairment. Cortex 48 (9), 1138–1154. doi:10.1016/j.cortex.2011.06.001
Lum, J. A. G., Gelgic, C., and Conti-Ramsden, G. (2010). Procedural and Declarative Memory in Children with and without Specific Language Impairment. Int. J. Lang. Commun. Disord. 45, 96–107. doi:10.3109/13682820902752285
Masoura, E. V., and Gathercole, S. E. (2005). Phonological Short-Term Memory Skills and New Word Learning in Young Greek Children. Memory 13 (3–4), 422–429.
McGregor, K. K., Arbisi-Kelm, T., Eden, N., and Oleson, J. (2020). The Word Learning Profile of Adults with Developmental Language Disorder. Autism Developmental Lang. Impairments 5, 2396941519899311. doi:10.1177/2396941519899311
Misyak, J. B., and Christiansen, M. H. (2012). Statistical Learning and Language: An Individual Differences Study. Lang. Learn. 62 (1), 302–331. doi:10.1111/j.1467-9922.2010.00626.x
Monaghan, P., Mattock, K., Davies, R. A. I., and Smith, A. C. (2015). Gavagai Is as Gavagai Does: Learning Nouns and Verbs from Cross-Situational Statistics. Cogn. Sci. 39 (5), 1099–1112. doi:10.1111/cogs.12186
Moyle, M. J., Ellis Weismer, S., Evans, J. L., and Lindstrom, M. J. (2007). Longitudinal Relationships between Lexical and Grammatical Development in Typical and Late-Talking Children. J. Speech Lang. Hear. Res. 50 (2), 508–528. doi:10.1044/1092-4388(2007/03510.1044/1092-4388(2007/035)
Newell, B. R., Lagnado, D. A., and Shanks, D. R. (2007). Challenging the Role of Implicit Processes in Probabilistic Category Learning. Psychon. Bull. Rev. 14, 505–511. doi:10.3758/BF03194098
Obeid, R., Brooks, P. J., Powers, K. L., Gillespie-Lynch, K., and Lum, J. A. G. (2016). Statistical Learning in Specific Language Impairment and Autism Spectrum Disorder: A Meta-Analysis. Front. Psychol. 7. doi:10.3389/fpsyg.2016.01245
Pearce, M. T. (2018). Statistical Learning and Probabilistic Prediction in Music Cognition: Mechanisms of Stylistic Enculturation. Ann. N.Y. Acad. Sci. 1423 (1), 378–395. doi:10.1111/nyas.13654
Perruchet, P., and Pacton, S. (2006). Implicit Learning and Statistical Learning: One Phenomenon, Two Approaches. Trends Cogn. Sci. 10, 233–238. doi:10.1016/j.tics.2006.03.006
Plante, E., and Gómez, R. L. (2018). Learning without Trying: The Clinical Relevance of Statistical Learning. Lshss 49 (3S), 710–722. doi:10.1044/2018_lshss-stlt1-17-0131
Racsmány, M., Lukács, Á., Németh, D., and Pléh, C. (2005). A Verbális Munkamemória Magyar Nyelvű Vizsgálóeljárásai. Magyar Pszichológiai Szemle 60, 479–506. doi:10.1556/mpszle.60.2005.4.3
Raven, J., Court, J., and Raven, J. (1987). Raven’s Progressive Matrices and Raven’s Colored Matrices. London, United Kingdom: H. K. Lewis.
Rebuschat, P., Monaghan, P., and Schoetensack, C. (2021). Learning Vocabulary and Grammar from Cross-Situational Statistics. Cognition 206, 104475. doi:10.1016/j.cognition.2020.104475
Rindermann, H., Flores-Mendoza, C., and Mansur-Alves, M. (2010). Reciprocal Effects between Fluid and Crystallized Intelligence and Their Dependence on Parents' Socioeconomic Status and Education. Learn. Individual Differences 20 (5), 544–548. doi:10.1016/j.lindif.2010.07.002
Roembke, T. C., and McMurray, B. (2021). Multiple Components of Statistical Word Learning Are Resource Dependent: Evidence from a Dual-Task Learning Paradigm. Mem. Cogn. 49, 984–997. doi:10.3758/s13421-021-01141-w
Roembke, T. C., Wiggs, K. K., and McMurray, B. (2018). Symbolic Flexibility during Unsupervised Word Learning in Children and Adults. J. Exp. Child Psychol. 175, 17–36. doi:10.1016/j.jecp.2018.05.016
Saffran, J. R., Aslin, R. N., and Newport, E. L. (1996). Statistical Learning by 8-Month-Old Infants. Science 274, 1926–1928. doi:10.1126/science.274.5294.1926
Saffran, J. R. (2002). Constraints on Statistical Language Learning. J. Mem. Lang. 47, 172–196. doi:10.1006/jmla.2001.2839
Schmalz, X., Moll, K., Mulatti, C., and Schulte-Körne, G. (2019). Is Statistical Learning Ability Related to Reading Ability, and if So, Why?. Scientific Stud. Reading 23 (1), 64–76. doi:10.1080/10888438.2018.1482304
Scott, R. M., and Fisher, C. (2012). 2.5-Year-olds Use Cross-Situational Consistency to Learn Verbs under Referential Uncertainty. Cognition 122 (2), 163–180. doi:10.1016/j.cognition.2011.10.010
Siegelman, N., Bogaerts, L., Christiansen, M. H., and Frost, R. (2017a). Towards a Theory of Individual Differences in Statistical Learning. Phil. Trans. R. Soc. B 372 (1711), 20160059. doi:10.1098/rstb.2016.0059
Siegelman, N., Bogaerts, L., and Frost, R. (2017b). Measuring Individual Differences in Statistical Learning: Current Pitfalls and Possible Solutions. Behav. Res. 49 (2), 418–432. doi:10.3758/s13428-016-0719-z
Simor, P., Zavecz, Z., Horváth, K., Éltető, N., Török, C., Pesthy, O., et al. (2019). Deconstructing Procedural Memory: Different Learning Trajectories and Consolidation of Sequence and Statistical Learning. Front. Psychol. 9, 2708. doi:10.3389/fpsyg.2018.02708
Smith, L. B., and Yu, C. (2013). Visual Attention Is Not Enough: Individual Differences in Statistical Word-Referent Learning in Infants. Lang. Learn. Development 9 (1), 25–49. doi:10.1080/15475441.2012.707104
Smith, L., and Yu, C. (2008). Infants Rapidly Learn Word-Referent Mappings via Cross-Situational Statistics. Cognition 106 (3), 1558–1568. doi:10.1016/j.cognition.2007.06.010
Spencer, M., Kaschak, M. P., Jones, J. L., and Lonigan, C. J. (2015). Statistical Learning Is Related to Early Literacy-Related Skills. Read. Writ 28 (4), 467–490. doi:10.1007/s11145-014-9533-0
Squire, L. R., Knowlton, B., and Musen, G. (1993). The Structure and Organization of Memory. Annu. Rev. Psychol. 44, 453–495. doi:10.1146/annurev.ps.44.020193.002321
Stavrakaki, S. (2020). Introduction to the Special Issue on Syntax and Verbal Short Term/working Memory in Developmental Disorders. London, England: SAGE Publications Sage UK.
Stavrakaki, S., and Lely, H. (2010). Production and Comprehension of Pronouns by Greek Children with Specific Language Impairment. Br. J. Developmental Psychol. 28 (1), 189–216. doi:10.1348/026151010x485269
Suanda, S. H., Mugwanya, N., and Namy, L. L. (2014). Cross-situational Statistical Word Learning in Young Children. J. Exp. Child Psychol. 126, 395–411. doi:10.1016/j.jecp.2014.06.003
Suanda, S. H., and Namy, L. L. (2012). Detailed Behavioral Analysis as a Window into Cross-Situational Word Learning. Cogn. Sci. 36 (3), 545–559. doi:10.1111/j.1551-6709.2011.01218.x
Tager-Flusberg, H. (2000). “Language and Understanding Minds: Connections in Autism,” in Understanding Other Minds (New York, NY: Oxford University Press), 124–149.
Tomasello, M. (2000). The Social-Pragmatic Theory of Word Learning. Prag 10 (4), 401–413. doi:10.1075/prag.10.4.01tom
Uddén, J., Ingvar, M., Hagoort, P., and Petersson, K. M. (2012). Implicit Acquisition of Grammars with Crossed and Nested Non-adjacent Dependencies: Investigating the Push-Down Stack Model. Cogn. Sci. 36 (6), 1078–1101. doi:10.1111/j.1551-6709.2012.01235.x
Ullman, M. T., Corkin, S., Coppola, M., Hickok, G., Growdon, J. H., Koroshetz, W. J., et al. (1997). A Neural Dissociation within Language: Evidence that the Mental Dictionary Is Part of Declarative Memory, and that Grammatical Rules Are Processed by the Procedural System. J. Cogn. Neurosci. 9, 266–276. doi:10.1162/jocn.1997.9.2.266
Ullman, M. T., and Pierpont, E. I. (2005). Specific Language Impairment Is Not Specific to Language: The Procedural Deficit Hypothesis. Cortex 41, 399–433. doi:10.1016/s0010-9452(08)70276-4
Ullman, M. T., and Pullman, M. Y. (2015). A Compensatory Role for Declarative Memory in Neurodevelopmental Disorders. Neurosci. Biobehavioral Rev. 51, 205–222. doi:10.1016/j.neubiorev.2015.01.008
Ullstadius, E., Gustafsson, J.-E., and Carlstedt, B. (2002). Influence of General and Crystallized Intelligence on Vocabulary Test Performance. Eur. J. Psychol. Assess. 18 (1), 78–84. doi:10.1027/1015-5759.18.1.78
Verhagen, J., and Leseman, P. (2016). How Do Verbal Short-Term Memory and Working Memory Relate to the Acquisition of Vocabulary and Grammar? A Comparison between First and Second Language Learners. J. Exp. Child Psychol. 141, 65–82. doi:10.1016/j.jecp.2015.06.015
Virag, M., Janacsek, K., Horvath, A., Bujdoso, Z., Fabo, D., and Nemeth, D. (2015). Competition between Frontal Lobe Functions and Implicit Sequence Learning: Evidence from the Long-Term Effects of Alcohol. Exp. Brain Res. 233 (7), 2081–2089. doi:10.1007/s00221-015-4279-8
Vouloumanos, A. (2008). Fine-grained Sensitivity to Statistical Information in Adult Word Learning. Cognition 107 (2), 729–742. doi:10.1016/j.cognition.2007.08.007
Weiss, D. J., Gerfen, C., and Mitchel, A. D. (2010). Colliding Cues in Word Segmentation: The Role of Cue Strength and General Cognitive Processes. Lang. Cogn. Process. 25 (3), 402–422. doi:10.1080/01690960903212254
Werker, J. F., and Curtin, S. (2005). PRIMIR: A Developmental Framework of Infant Speech Processing. Lang. Learn. Development 1 (2), 197–234. doi:10.1080/15475441.2005.9684216
Wonnacott, E. (2011). Balancing Generalization and Lexical Conservatism: An Artificial Language Study with Child Learners. J. Mem. Lang. 65 (1), 1–14. doi:10.1016/j.jml.2011.03.001
Wonnacott, E., Newport, E. L., and Tanenhaus, M. K. (2008). Acquiring and Processing Verb Argument Structure: Distributional Learning in a Miniature Language. Cogn. Psychol. 56 (3), 165–209. doi:10.1016/j.cogpsych.2007.04.002
Young, A. R., Beitchman, J. H., Johnson, C., Douglas, L., Atkinson, L., Escobar, M., et al. (2002). Young Adult Academic Outcomes in a Longitudinal Sample of Early Identified Language Impaired and Control Children. J. Child. Psychol. Psychiat 43 (5), 635–645. doi:10.1111/1469-7610.00052
Yu, C. (2008). A Statistical Associative Account of Vocabulary Growth in Early Word Learning. Lang. Learn. Development 4 (1), 32–62. doi:10.1080/15475440701739353
Yu, C., and Smith, L. B. (2007). Rapid Word Learning under Uncertainty via Cross-Situational Statistics. Psychol. Sci. 18 (5), 414–420. doi:10.1111/j.1467-9280.2007.01915.x
Keywords: lexical development, vocabulary, statistical learning, verbal short-term memory, typical development, developmental language disorder
Citation: Kemény F and Lukács Á (2021) The Role of Statistical Learning and Verbal Short-Term Memory in Impaired and Typical Lexical Development. Front. Commun. 6:700452. doi: 10.3389/fcomm.2021.700452
Received: 26 April 2021; Accepted: 09 July 2021;
Published: 27 July 2021.
Edited by:
Xiaofei Lu, The Pennsylvania State University (PSU), United StatesCopyright © 2021 Kemény and Lukács. This is an open-access article distributed under the terms of the Creative Commons Attribution License (CC BY). The use, distribution or reproduction in other forums is permitted, provided the original author(s) and the copyright owner(s) are credited and that the original publication in this journal is cited, in accordance with accepted academic practice. No use, distribution or reproduction is permitted which does not comply with these terms.
*Correspondence: Ferenc Kemény, ZmVyZW5jLmtlbWVueUB1bmktZ3Jhei5hdA==