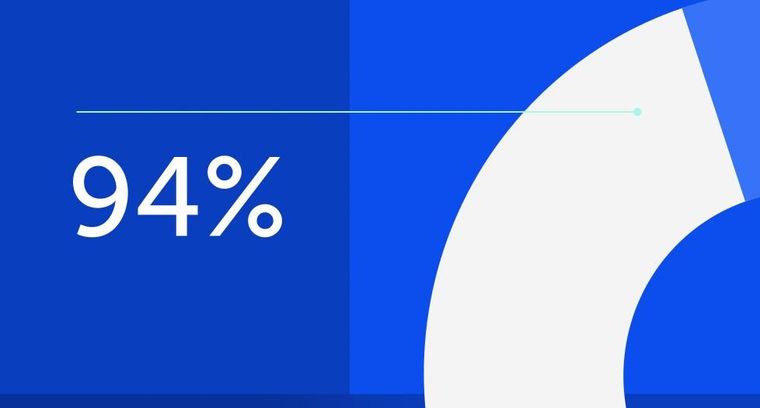
94% of researchers rate our articles as excellent or good
Learn more about the work of our research integrity team to safeguard the quality of each article we publish.
Find out more
ORIGINAL RESEARCH article
Front. Commun., 15 October 2021
Sec. Psychology of Language
Volume 6 - 2021 | https://doi.org/10.3389/fcomm.2021.674126
This article is part of the Research TopicRational Approaches in Language ScienceView all 30 articles
The relationship between pronoun production and pronoun interpretation has been proposed to follow Bayesian principles, combining a comprehender’s expectation about which referent will be mentioned next and their estimate of how likely it is that a potential referent will be re-mentioned using a pronoun. The Bayesian Model has received support from studies in several languages (English, Mandarin Chinese, Catalan, German), but tested contexts have been limited to two event participants, whereas natural language discourse often involves contexts with more than two event participants. In this study, we conducted three story continuation experiments to assess how the Bayesian Model performs in more complex contexts. Our results show that even in contexts with three event participants, comprehenders can behave rationally when interpreting pronouns, but that they appear to require sufficient context to build up a coherent representation of the situation to do so. In addition to testing the basic claim of the Bayesian Model (Weak Bayes), we test the central prediction of the Strong form of the hypothesis: that the two components of the model (next-mention expectations and choice of referring expression) are influenced by dissociated sets of factors. In a model comparison, Experiments 2 and 3 confirm the closest fit from the Bayesian Model, which supports Weak Bayes, and none of our experiments find evidence that the predictability of a referent affects pronominalization rates, which corroborates Strong Bayes. Finally, we test whether the rate of pronominalization is sensitive to factors related to ambiguity and argument/adjunct status of referents; we find that participants vary their production of pronouns most strongly based on the grammatical role of the antecedent (subject or not), with a smaller effect from the presence/absence of a gender-matched competitor and no effect from the syntactic position of this competing referent.
Reduced reference to previously mentioned entities–such as that achieved via pronominalization–is a hallmark of coherent discourse. Yet a speaker’s decision to employ a reduced form poses an interpretation problem to the hearer, who needs to recover the speaker’s intended referent.1 A commonly held view is that speakers and hearers coordinate on the reference problem through a notion of entity salience: Speakers consult a set of factors that contribute to salience in deciding to use a pronoun, and hearers consult those same factors when interpreting it. Much of the literature has engaged with the question of what these factors are–including, for example, order of mention, grammatical role, thematic role, parallelism, information structure, and world knowledge–and how they are weighed with respect to one another.
There is also evidence, however, to suggest that the factors that condition pronoun production and interpretation are to some degree dissociated. In a context like (1), hearers are more likely to interpret a subsequent pronoun she as in (1-a) as referring to Jill than speakers are to produce a pronoun when referring to Jill in a subsequent sentence as in (1-b); likewise, speakers are more likely to use a pronoun to refer to Sue in (1-b) even though Sue will not be the preferred referent for the hearer in (1-a) (e.g., Stevenson et al., 1994; Kehler et al., 2008; Kehler and Rohde 2013).
1) a. Sue fired Jill. She ________
b. Sue fired Jill. ________
This asymmetry between the production of pronouns and their interpretation is posited to reflect a separation between the factors that guide choice of referring expression and the factors that guide expectations of next mention (both of which in turn influence interpretation).
Kehler et al. (2008) (see also Kehler and Rohde 2013; Rohde and Kehler 2014; Kehler and Rohde 2019) propose a rational Bayesian approach that is capable of capturing this asymmetry, according to which a hearer combines their expectation about which referent will be mentioned next and their estimate of how likely a speaker is to use a pronoun when re-mentioning a potential referent. The model produces quantitative estimates of interpretation biases that can be compared directly against actual biases collected in passage completion studies, and also allows for the factors that contribute to production and interpretation to be evaluated separately. Thus far, studies on English (Rohde and Kehler 2014; Kehler and Rohde 2019; Cheng and Almor 2019), Mandarin Chinese (Zhan et al., 2020), Catalan (Mayol, 2018), and German personal and demonstrative pronouns2 have provided support for the model (but see Lam and Hwang 2021).
These studies have all focused on contexts with two event participants as potential referents for a pronoun. But natural language use, of course, often involves discourse contexts with more than two event participants. In light of the demands that a rational interpretation process might place on a hearer’s cognitive apparatus (e.g., working memory, attention, probability estimation), an open question is how the model performs in more complex contexts: How well can hearers behave rationally when interpreting pronouns when there is a greater number of event participants to keep track of?
To address this question, we will employ contexts using the benefactive construction, exemplified in (2).
2) Adam scolded Russell for Diana.
Benefactive sentences describe situations in which an Agent engages in an action that affects a Patient for the benefit of a Beneficiary; these event participants appear as the grammatical subject, direct object, and object of a prepositional phrase adjunct, respectively.
In addition to its ability to introduce three event participants into the discourse, the benefactive construction allows us to address two other questions that currently exist in the literature. The first bears on the distinction between referents introduced from argument and adjunct positions and the rate at which they are pronominalized. Previous work that has compared two types of transfer-of-possession contexts–Source-Goal and Goal-Source constructions–has found a limited effect of thematic role on pronoun production favoring the Goal (Arnold 2001; Rosa and Arnold 2017; but see Rohde 2008 Expt VIII). In contrast, studies that have compared two types of implicit causality contexts–subject-biased and object-biased–have not (Rohde, 2008; Fukumura and Van Gompel, 2010; Rohde and Kehler, 2014). As we explain in further detail in Section 1.2, it has been suggested (Fukumura and Van Gompel, 2010; Rohde and Kehler, 2014) that the argument-adjunct distinction may have confounded results using transfer verbs, since the Goal occurs in an obligatory argument position in both types, whereas the Source is an argument in the Source-Goal construction but an adjunct in the Goal-Source construction. Benefactives provide a novel way to examine this question, since the subject and object appear in argument positions but the beneficiary occurs within a prepositional phrase adjunct. By utilizing benefactive contexts in three different configurations in which reference is two-ways ambiguous, we can run controlled studies that shed new light on this question.
A second question bears on comparing pronominalization rates for entities in referentially ambiguous and unambiguous contexts, a question for which different perspectives on the role of pronominalization make different predictions. On the one hand, on the view that referential form selection is intimately connected to audience design and ambiguity avoidance, we might expect to witness a big difference in the two scenarios: Speakers might be expected to pronominalize whenever possible in referentially-unambiguous contexts since referential success is not at stake, whereas they would presumably pronominalize less when the resulting expression would risk being ambiguous. On the other hand, on the view that pronominalization is driven primarily by the topicality of the referent (Grosz et al., 1995; Rohde and Kehler, 2014), little or no difference might be expected. Past work (Arnold and Griffin, 2007; Rohde, 2008; Rosa and Arnold, 2017) has shown a mixed picture: Ambiguity does affect rate of pronominalization, counter to a pure topicality account, but not to the extent one would expect if ambiguity avoidance was the only concern. This work has drawn comparisons across different contexts, however. Again, by utilizing benefactive contexts in three different configurations–each of which having three event participants, two that participate in referential ambiguity and one that does not–we can analyze this question across three different grammatical role pairings in the context of a single experiment.
This paper reports on three experiments designed to examine these issues, using discourse contexts that employ the benefactive construction. We focus on four central questions:
1. How well does the Bayesian Model predict the actual biases that hearers bring to the interpretation of pronouns in benefactive contexts, as measured in passage completion experiments?
2. Are the factors that influence pronoun production the same as those that influence predictability, or is there a dissociation between them?
3. Do pronoun production rates vary depending on whether the referent is introduced in an argument or adjunct position?
4. Do pronoun production rates vary depending on whether pronominal reference is ambiguous, keeping all else equal?
We elaborate on these questions in the sections that follow.
The Bayesian Model posits that a comprehender, upon encountering a pronoun, interprets it by reverse-engineering the speaker’s intended referent following Bayesian principles (Kehler et al., 2008; Kehler and Rohde, 2013; Rohde and Kehler, 2014). The relationship between interpretation and production is captured by the model via the straightforward application of Bayes’ Rule shown in Eq. 1.
The posterior term P(referent|pronoun) represents the comprehender’s interpretation bias: upon encountering a pronoun, the probability that the comprehender will interpret it as referring to a particular referent. On the other hand, the likelihood term P(pronoun|referent) represents the production bias: the comprehender’s estimate of the probability that the speaker would use a pronoun to refer to the potential referent under consideration. Finally, the prior term P(referent) denotes the comprehender’s next-mention bias: the hearer’s estimate of the probability that the speaker would mention a specific referent at that point in the discourse, without regard for the form of referring expression that is chosen. On this model, therefore, pronoun interpretation biases result from comprehenders integrating their ‘top-down’ predictions about the content of the ensuing message (particularly, who will be mentioned next) with the ‘bottom-up’ linguistic evidence (particularly, the fact that the speaker opted to use a pronoun).
Kehler et al. offer two varieties of the Bayesian Model. As it stands, Eq. 1 says only that the relationship between pronoun interpretation and pronoun production follows Bayesian principles, without further specifying the types of contextual factors that affect the likelihood and prior terms. This claim is the sole prediction of the weak form of the hypothesis. That is, the weak hypothesis says that, given independent estimates of the prior, likelihood, and posterior probabilities, Eq. 1 will approximately hold.
Whereas this is the central claim of the Bayesian Hypothesis, Kehler et al. also cited evidence that the two terms in the numerator of Eq. 1 are conditioned by different types of contextual factors. On the one hand, they noted that the results of previous studies suggested that the factors that condition the next-mention bias P(referent) are primarily driven by meaning: semantic factors such as the verbs used in the context sentences and the eventualities they describe, and certain types of pragmatic inferences, including the coherence relations established between the clauses. On the other hand, the factors that condition the production bias P(pronoun|referent) appear to be grammatical and/or information structural in nature, for instance, based on grammatical role obliqueness or topichood respectively, both of which amount to a preference for pronouns when a sentential subject is re-mentioned. The resulting prediction, therefore, is that a speaker’s decision about whether or not to pronominalize a reference will be insensitive to a set of semantic and pragmatic contextual factors that the comprehender will nonetheless bring to bear in interpretation. This is the central prediction of the strong form of the hypothesis.
The empirical status of the strong hypothesis remains under debate; while it is supported by for instance Rohde’s (2008) (see also Rohde and Kehler (2014)) and Fukumura and Van Gompel’s (2010) studies using implicit causality contexts, Rosa and Arnold (2017) report an effect of referent predictability on pronominalization in transfer-of-possession contexts. One consistent finding, however, is that insofar as semantic factors influence production at all, they do not affect production biases to the same extent that they do interpretation. We will examine the predictions of the strong model in the current experiments as well.
In order to provide benchmarks against which to evaluate the performance of the Bayesian Model, we will compare its quantitative predictions against those of two other models, each of which represent particular operationalizations of ideas drawn from the literature. The first such model we call the Mirror Model, which is designed to capture the idea that there is a single notion of entity prominence that the speaker and comprehender jointly use to mediate pronoun production and interpretation (posited by accounts of coreference put forward by, for instance, Ariel 1990, Givón 1983, and Gundel et al., 1993). On this conception–under which the comprehender is using the same cues to referential prominence that the speaker is–the ultimate interpretation bias toward a referent on the comprehension side should be proportional to the likelihood of the referent being pronominalized by the speaker, as reflected in Eq. 2.
Here we use the assignment operator to capture the fact that this model, unlike (1), does not follow the standard laws of probability theory. This model captures the idea that comprehenders will assign pronouns based on their consideration of what entities the speaker is most likely to refer to using a pronoun instead of a competing referential form, which is cached out by taking the comprehenders’ estimate of the probability that a speaker will produce a pronoun for a particular referent, normalized by the sum of the probabilities for all suitably prominent referents that are consistent with any constraints imposed by the pronominal form (gender, number, etc).
The second competing model we refer to as the Expectancy Model, which represents a particular way of operationalizing of an insight from Arnold (1998) regarding the role of predictive processing. According to Arnold’s Expectancy Hypothesis, “listeners focus their attention on discourse entities in proportion to their estimation of the likelihood that the entity will be mentioned” (Arnold, 2008, p. 505). Comprehenders use referential expectations as a proxy for their estimates of speaker’s focus of attention (p. 506); the higher this level of attention for a particular entity, the higher the likelihood that the speaker, when uttering a pronoun, is using it to refer to that entity. Here we operationalize this idea using next-mention bias P(referent) in Eq. 3, normalized by the next-mention probability of all referents that are compatible with the constraints (gender, number) imposed by the pronominal form.
We again use the ← assignment operator to emphasize the fact that the equality of the terms on the left and right hand sides does not follow from the laws of probability theory. On this model, therefore, the influence of context is mostly ‘top-down’, creating expectations about who will be mentioned next, with pronoun interpretation biases following these expectations.
The primary evidence for the impact of thematic role on pronoun production comes from work by Arnold and colleagues. First, Arnold (2001) found an effect that favored the pronominalization of Goal antecedents over Source antecedents when comparing two types of transfer-of-possession contexts: Goal-Source frames (The butler got some ice from the chef) and Source-Goal frames (The chef gave some ice to the butler). However, the effect was relatively small, and only found when the antecedent was a non-subject. More recently, Rosa and Arnold (2017) ran three follow-up experiments using the same types of frames, one which used an event-retelling task with more situated contexts (Exp 1) and two standard story-continuation tasks (Exps 2-3). Effects were found in Exps. 1 and 3, but much more strongly for subject antecedents than non-subject antecedents in the same-gender condition of Exp. 1 and only for non-subject antecedents in Exp. 3.3 We will return to these findings in the General Discussion, after presenting our results using benefactive contexts.
There is an additional complication that arises when it comes to disentangling the effects of thematic role and grammatical role in transfer-of-possession frames. As expected, across Rosa and Arnold’s experiments there was a large effect of grammatical role whereby referents introduced in subject position are re-mentioned with pronouns at higher rates than those introduced in object position. The thematic role effect arises when comparing Goal and Source subjects and likewise Goal and Source non-subjects. However, there is a relevant asymmetry here: whereas the Goal in a Source-Goal frame is mentioned from an obligatory argument position (Sue handed the book *(to Mary)), the Source in a Goal-Source frame is mentioned from within an optional adjunct (Mary received the book (from Sue)). The reason this is relevant is that according to some theories of information structure (Lambrecht, 1994, inter alia), the potential for topicality of a constituent decreases as one moves down the obliqueness hierarchy (subjects > objects > other arguments > adjuncts). On a theory in which pronominalization biases are driven by topicality (Grosz et al., 1995; Rohde and Kehler, 2014), it follows that the increased pronominalization rates for Goals in subject position could be attributed to the fact that it competes for topicality with an adjunct, whereas Source subjects compete with another argument. Similar logic applies for non-subjects: as arguments, Goals may be more topical than adjunct Sources. To shed new light on this question, we use the benefactive construction in contexts with three event participants but where only two of them match the gender of the pronoun in the pronoun-prompt condition. By running all three possible configurations–where NP1 and NP2 compete, NP1 and NP3 compete, and NP2 and NP3 compete–we can hold constant the status of a given referent and analyze its pronominalization rate when it competes with a gender-matched referent in an argument or adjunct position.
Hearer-oriented models of pronoun production make the assumption that speakers take into account the hearer’s discourse model when producing referring expressions. Many studies suggest that speakers avoid producing ambiguous referring expressions to make sure they are understood correctly by their audience (e.g., Horton and Keysar 1996; Nadig and Sedivy 2002; Matthews et al., 2006; Hendriks et al., 2014). Under this assumption, speakers are less likely to produce a pronoun for a referent when there is another referent in the immediate discourse that matches the intended referent in features relevant to the pronoun (e.g., gender, number, or animacy in English).
Evidence for a role of ambiguity avoidance in pronoun production, however, is not undisputed. Fukumura and van Gompel (2012), for instance, find that speakers produce pronouns to refer to referents in the preceding discourse, regardless of whether their addressee has knowledge of the preceding discourse. In addition, Arnold and Griffin (2007) show that an additional potential referent in the discourse leads to a decrease in the proportion of pronouns produced, even if the pronoun would nonetheless be unambiguous. In explaining this effect, Arnold and Griffin take a speaker-oriented approach by arguing that additional referents influence pronoun production by competing for attention in the speaker’s representation of the discourse (and that similarity between referents, for instance in terms of gender, increases this effect; see also Fukumura et al., 2011). Offering a similar speaker-based explanation for Arnold and Griffin’s findings, Rohde and Kehler (2014) propose that more referents entering the discourse decreases the chance that a referent is the topic, which in turn reduces the pronominalization rate. The question thus remains whether speakers strive to avoid ambiguity when producing referring expressions.
In a story continuation experiment, we tested participants’ pronoun interpretations, re-mention preferences, and pronominalization rates in contexts containing sentence frames with three event participants: an Agent (NP1), a Patient (NP2), and a Benefactive (NP3), as in for instance Ben followed Sophia for David. We varied prompt type (pronoun vs full-stop) and the position of the pair of gender-matched referents (NP1&NP2 vs NP1&NP3 vs NP2&NP3).
Crucial to determining whether different factors influence the prior and the likelihood (i.e., Strong Bayes), we expect that in these sentence frames, like in the implicit-causality and transfer-of-possession constructions commonly used in previous research on pronoun production and interpretation, the topicality and predictability of the referents do not coincide. Regarding topicality, if we assume that the grammatical subject position is the default position for topics in English, then the Agent in these benefactive constructions is the most topical referent. On the other hand, the predictability for re-mention does not necessarily favor the subject. For coherence-driven reasons, the Benefactive may be preferred if the next sentence provides an explanation of the event and one assumes that the initiative for the event is attributed to the Benefactive (i.e., why did David want Sophia followed and why didn’t he do this himself?). Alternatively, the Patient may be preferred if the next sentence describes what happened next and the Patient is the referent most closely associated with the end state of the event. The point is that these benefactive sentences are posited to disfavor the subject referent for re-mention, a scenario that allows us to test the effectiveness of coreference models in contexts in which next-mention and pronominalization biases are dissociated.
Participants were recruited through Amazon Mechanical Turk. 143 monolingual speakers of English completed the experiment and wrote correct continuations for the catch trials (see Materials) (mean age 37.2, age range 18–66, 65 women). Monolingual status was defined as an answer of ‘no’ to a question of whether any other language was spoken at home before the age of 6. All participants were paid for their participation ($5.25).
Stimuli consisted of 30 target prompts that featured three referents (subject, direct object, benefactive) and varied in prompt type (full stop vs pronoun), as in (3). Proper names were used to manipulate which potential referents were gender-matched: NP1&NP2, as in (3), NP1&NP3, or NP2&NP3.4
3) a. AdamNP1 scolded RussellNP2 for DianaNP3. ________ [full-stop prompt]
b. AdamNP1 scolded RussellNP2 for DianaNP3. He ________ [pronoun prompt]
The target items were distributed over six lists, with each item occurring only once per list, in one of the six conditions. The target items were interspersed with 32 fillers, including two ‘catch’ items that had an obvious correct continuation (e.g., Caleb’s favorite TV series is Game of [Thrones]); these two items were used to filter out any participants who were not taking the task seriously. The other fillers varied in the number of (human) arguments they contained and whether they ended in a full stop or after the first word of a second sentence (similar to the pronoun prompt items).
Continuations were collected via a web-based interface embedded in the Amazon Mechanical Turk environment. After reading a short instruction, signing a consent form, and supplying some demographic information, participants were asked to write a natural continuation for the prompts in the supplied text box. Each item was displayed on a separate page.
For all target items in all three experiments, we annotated which referent was the subject of the continuation (next-mention: NP1, NP2, NP3) and how that referent was re-mentioned (form of referring expression: full NP vs pronoun). To ensure reliable coding, we (first author and a trained linguistics undergraduate student) double-coded data from approximately 85 participants for all three experiments (approx. 60% for Experiment 1, 100% for Experiment 2, and 55% for Experiment 3). Inter-annotator agreement was very high on both next-mention (Experiment 1: 93%, κ = 0.90, Experiment 2: 94%, κ = 0.90, Experiment 3: 93%, κ = 0.91) and form of referring expression (Experiment 1: 99.5%, Experiment 2: 100%, Experiment 3: 99.3%). In all three experiments, the majority of disagreements on next-mention were due to one coder making a decision, while the other indicated they were not completely sure who was being referred to. All disagreements on form of referring expression were due to coding errors (5 in Experiment 1 and 7 in Experiment 3). After considering all disagreements, one coder (first author) finished annotation of the data from Experiments 1 and 3.
We analyze the data in R (R Core Team, 2019). We compare the predictability and pronominalization rates of the referents using generalized linear mixed-effect regression (GLMM: Jaeger 2008) using the lme4 package (Bates et al., 2015).
For our questions regarding the efficacy of the Bayesian Model in benefactive contexts and the separation of referent predictability from pronominalization, we consider participants’ next-mention and pronoun production behavior in the full-stop condition. To compare the predictability of the referents, we model the binary value of next-mention (yes vs no) in the full stop prompt subset of the data, with fixed effects of Referent (three levels: NP1/NP2/NP3) and Ambiguous Pair (three levels: NP1&NP2, NP1&NP3, NP2&NP3), as well as the interaction between Referent and Ambiguous Pair. To compare the pronominalization rates, we model the binary value of form of referring expression (pronoun or not) with Referent, Ambiguous Pair, and their interaction as fixed effects. Finally, we compare whether the pronoun prompts resulted in more NP1 continuations than the full stop prompts by modeling the binary value of NP1 continuation (yes vs no) on the entire dataset, with Prompt Type as fixed effect.
For our questions regarding the effect of argument/adjunct status and referential ambiguity on pronominalization, we compare pronominalization rates for ambiguous and unambiguous referents across the three ambiguous pair conditions in which a referent’s gender-matched competitor is either an argument or adjunct. We model the binary value of form of referring expression (pronoun or not), with referent (three-level) and ambiguity (yes or no), as well as their interaction as fixed effects.
All models contained by-participant and by-item random effects. For each model, we started with a maximal random effects structure, only simplifying the model in case of non-convergence (cf. Barr et al., 2013). All categorical predictor variables in all analyses were deviation coded. The significance of fixed effects was determined by performing likelihood ratio tests to compare the fit of the model to that of a model with the same random effects structure that did not include the fixed effect. In case of significant three-level categorical predictor variables, we obtained pair-wise comparisons using a subset of the data that only contained the relevant conditions with re-centered predictor variables.
For a comparison between the three models of pronoun interpretation, we follow Rohde and Kehler (2014). We use the free prompt continuations to calculate Bayes-derived estimates of p(referent|pronoun) via the prior p(referent) and likelihood p(pronoun|referent), as well as estimates for the Expectancy Model (normalized prior) and the Mirror Model (normalized likelihood). We then compare the model estimates with the pronoun interpretations measured in the pronoun prompt condition. We calculate the correlation between the model estimates and the observed pronoun interpretations. For these estimates, we only consider the subset of continuations in a given Ambiguous Pair condition that mention the referent who the ambiguous pronoun could refer to. While Rohde and Kehler (2014) calculate observed pronoun interpretations and model estimate both by-participant and by-item, we only compare the by-item model estimates to the by-item observed pronoun interpretation rates. A crucial difference between our experiments and Rohde and Kehler (2014) study is that we have to take into account which two out of three referents compete with each other for coreference. Obtaining, per participant, a number of observations per ambiguous pair (NP1&NP2, NP1&NP3, NP2&NP3) similar to the number of observations on which the Rohde and Kehler (2014) calculations are based requires triple the number of target items. This would make the experiments infeasibly long and very likely diminish the quality of participants’ output. Since by-item and by-participant analyses in previous studies yielded similar results, we opt to only compare the model estimates to the observed pronoun interpretations by item. While this creates a similar data sparsity issue as the by-participant analyses, we compensate for this by increasing the number of participants.
First, we replicate the well-established finding that pronoun prompts yield more NP1 continuations than full stop prompts (β = 0.36, SE = 0.07, z = 4.85, p < 0.001); see Table 1 for the means collapsed across condition or Table 2 for the same data broken down by condition. When it comes to the predictability of the referents (measured in the full stop prompts), there is a main effect of Referent (reflecting the bias away from NP1 towards NP2 and NP3; p < 0.001), no main effect of Ambiguous Pair (p = 0.81) and a Referent × Ambiguous Pair interaction (p < 0.001), whereby the re-mention rates of NP2 and NP3 generally differ more across the ambiguous pair conditions than does the re-mention rate of NP1. Follow-up analyses confirm that there is a main effect of ambiguous pair in the NP2 and NP3 subsets of the data (p < 0.01 for both), but no main effect in the NP1 subset of the data (p = 0.16).
TABLE 2. Proportion of next-mention in Experiment 1, per referent, per prompt type, per ambiguous pair. The vertical columns sum to one (e.g., the re-mention rates in the NP1&NP2 condition are distributed 0.24/.25/.51 across the three referents).
In keeping with the strong Bayes account in which factors that influence the predictability of re-mention are distinct from those that influence pronominalization, the pronominalization rates of the referents (measured in the full stop prompts) did not differ across the ambiguous pair conditions (p = 0.98) and the interaction between Referent and Ambiguous Pair was also not significant (p = 0.84). There was, however, a main effect of Referent influencing pronominalization (p < 0.001): The subject referent NP1 is more often re-mentioned with a pronoun than NP2 (β = 49.23, SE = 15.60, z = −3.16, p < 0.001) or NP3 (β = 78.61, SE = 10.83, z = 7.26, p < 0.001). There is no difference between NP2 and NP3 (β = 4.28, SE = 9.53, z = 0.45, p = 0.68); see Table 3 for the pronominalization rates broken down by ambiguity of referent or Table 4 for those rates broken down by referent and by condition.
TABLE 3. Proportion of pronominalization by ambiguous vs unambiguous referents in Experiment 1 in the full stop prompt condition.
TABLE 4. Proportion of pronominalization of ambiguous referents in the full stop prompt items in Experiment 1, per referent, per ambiguous pair.
We also test the effect of referent ambiguity on pronoun production. We find that unambiguous referents were more often pronominalized than ambiguous referents (β = 0.50, SE = 0.18, z = 2.74, p < 0.01). The interaction between Ambiguity and Referent was not significant at p = 0.64; see Table 3.
Finally, we are interested in which model yields the best correlations with the observed pronoun interpretation behavior. As in earlier work, the Bayesian Model’s correlation with observed pronoun interpretation is stronger than that of the Expectancy Model; see Table 5. In contrast, however, the Mirror Model provided the best fit to the observed data. Figure 1 visualizes the estimates for all three models compared to the observed pronoun interpretations for each referent in each ambiguous pair. This first of all reveals that the Expectancy Model consistently overestimates the influence of predictability: In the NP1&NP2 and NP1&NP3 ambiguous pairs, for instance, the pronoun is often interpreted as NP1 by participants, but since it is not the preferred referent for re-mention, the Expectancy Model underestimates coreference to NP1. Similarly, the Bayesian also appears to place too much importance on predictability (the prior), though not to the same extent as the Expectancy Model.
TABLE 5. Correlations between observed data and model predictions in Experiment 1, by items. *indicates significance at or below 0.001.
FIGURE 1. By-item observed values and model estimates for each referent in each ambiguous pair in Experiment 1. Tilted squares indicate the means. Random jitter has been added to the individual data points to avoid overplotting.
The first question we ask is how well the Bayesian Model predicts the interpretation biases witnessed in the passage completions the participants provided, as compared to the other two models. Whereas the Bayesian Model outperformed the Expectancy Model, its predictions were not as accurate as those made by the Mirror Model. The difference between the two models is that the Bayesian Model incorporates the next-mention biases witnessed in the free-prompt data, which favored NP3 over the other two event participants. This overlaid effect of the prior was not witnessed as strongly in the interpretation biases estimated in the pronoun prompt condition, resulting in the Mirror Model being the most empirically adequate.
The second question we ask is whether there is evidence for the independence between factors that determine predictablity and pronominalization, as predicted by the strong form of the Bayesian Model. The answer here is affirmative. The most predictable referent (NP3) is not the one most often pronominalized, while the least predictable referent (NP1) is. Furthermore, comparing the re-mention rates in Table 1 and the pronominalization rates in Table 3, the overall re-mention rates of NP1 and NP2 are similar (0.24 vs 0.30), but their pronominalization rates are not (0.77 versus 0.26). Conversely, the re-mention rates of NP2 and NP3 differ (0.30 versus 0.46), but their pronominalization rates do not (0.26 for both). We thus find no evidence of a dependence between predictability and pronominalization.
The results, somewhat curiously, therefore appear to support the added predictions of the strong form of the Bayesian Model, but ultimately not the basic claims of the weak form, a result not seen in previous work. Comparing this study to previous ones that have found the Bayesian Model to make the best predictions, we see that our materials differ in two ways: We used a different construction in our context sentences than previous work, and also increased the number of event participants introduced in those sentences. We attempt to tease apart these two possible sources in Experiment 2 by keeping the benefactive sentence frame while reducing the number of human event participants it introduces by employing a non-human Patient. If the results witnessed in Experiment 1 are due to particular properties associated with benefactive contexts, we expect the Mirror Model to continue to outperform the Bayesian Model. On the other hand, if the issue bears on the cognitive load imposed by having to track three event participants who are introduced by name out of the blue in the context sentence, the Bayesian Model might do better in contexts where only two human event participants need to be tracked.
Our third question asks whether pronoun production is sensitive to argument/adjunct status. The results from Experiment 1 do not support the hypothesis that pronominalization rates vary systematically with argument/adjunct status beyond the well-known effects of subjecthood. If argument/adjunct status played a role in pronominalization, we would have expected variation by Ambiguous Pair such that the pronominalization rate of, for example, NP1 varied depending whether its gender-matched competitor was NP2 (an argument of the verb) or NP3 (an adjunct). Contra an account in which pronominalization rates of referents are consistently higher when their competing referent is an adjunct or consistently lower when the referent itself occupies an adjunct position (such as the account proposed to explain Rosa and Arnold 2017 thematic role effects), NP1 and NP2 show divergent behavior. For NP1, there is no increase in the pronominalization rate between the condition where the competing gender-matched referent is an argument (the NP1&NP2 condition) and that where the competing referent is an adjunct (the NP1&NP3 condition); rather there is a numeric decrease. This pattern is reversed for NP2, where the pronominalization rate does increase from the condition with an argument competitor (NP1&NP2) to the condition with an adjunct competitor (NP2&NP3). However, these numeric patterns were not sufficient to give rise to a main effect of Ambiguous Pair on pronominalization. There is thus no evidence of a consistent pattern which would support the proposed alternative explanation of the previously reported effects of thematic role on pronominalization.
Finally, the fourth question asks whether pronominalization rate is sensitive to the potential ambiguity of a pronoun. The results indicate that presence of other referents that make pronominal reference ambiguous does reduce the rate of pronominalization. Since this effect was the same across all three referents, the effect does not seem to have been influenced by the referents’ topicality or predictability. Looking at Table 3, however, the effect of ambiguity on pronominalization appears to be modest. If ambiguity avoidance is the primary concern, one might expect this effect to be larger; as is, it is not on a par with the larger main effect of grammatical role.
In order to ease the cognitive load of tracking three human, discourse-new referents, we replicate the setup for Experiment 1, except that we modify the stimuli so as to employ a non-human event participant in the NP2 position.
Participants were recruited through Amazon Mechanical Turk. 85 monolingual speakers of English completed the experiment and wrote correct continuations to the catch trials (see Materials) (mean age 36.9, age range 21–71, 47 women, 2 participants preferred not to supply their gender identity). All participants were paid in exchange for their participation ($5.25).
Stimuli consisted of 28 target prompts that featured three arguments. Unlike in Experiment 1, however, the second argument was a non-human, usually inanimate, event participant, as in (4). The two human event participants in this experiment were of the same gender, as signalled by the default gender associated with their names. Since we are only interested in whether and how the two human potential referents are picked up, the items in this experiment correspond only to the NP1&NP3 conditions from Experiment 1.
4) a. JacobNP1 called the hospital for MaxNP3. ________ [full stop prompt]
b. JacobNP1 called the hospital for MaxNP3. He ________ [pronoun prompt]
The prompts were adapted from the items from Experiment 1 as much as possible, but not all verbs were compatible with a non-human second argument. In total, half the prompts used a verb that was also included in Experiment 1.5
The target items were distributed over two lists, with each item occurring only once per list, in one of the two conditions. The target items were interspersed with 32 fillers, including the same two ‘catch’ items that were used in Experiment 1. The other fillers varied in the number of (human) arguments they contained and whether they ended in a full stop or after the first word of a second sentence (similar to the pronoun prompt items).
The task setup and the subsequent annotation followed that of Experiment 1.
The analysis followed that of Experiment 1, except that the fixed effect of Referent was binary (NP1/NP3) and there was no fixed effect of Ambiguous Pair.
As in Experiment 1, there were more NP1 re-mentions in the pronoun prompt condition than in the full stop condition (β = 2.88, SE = 0.25, z = 11.29, p < 0.001), as shown in Table 6. In addition, we again find no evidence that predictability influences pronominalization rates: While NP3 is more predictable than NP1 (β = 3.52, SE = 0.60, z = 5.87, p < 0.001), as shown in Table 6, NP1 is pronominalized more often than NP3 (β = 5.89, SE = 0.82z = 7.20, p < 0.001), as shown in Table 7.
TABLE 7. Pronominalization rates in Experiment 2, per referent. All pronominal references are ambiguous.
Unlike in Experiment 1, however, the Bayesian Model yields the best correlations with the observed pronoun interpretations, as shown in Table 8. As can be seen from Figure 2, the Expectancy Model again overestimates the importance of predictability: The pronoun is more often interpreted as NP1 by participants than would be expected on the basis of the next-mention rates. In contrast, by not taking into account the predictability of the referents at all, the Mirror Model overestimates how often participants interpret the pronoun as referring to NP1 in this experiment.
TABLE 8. Correlations between observed data and model predictions in Experiment 2, by items. * indicates significance at or below 0.001.
FIGURE 2. By-item observed values and model estimates for each referent in Experiment 2. Tilted squares indicate the means.
Regarding our research questions about the status of competing referents and the role of ambiguity, Experiment 2 does not provide data to speak to these since the items contain only two human referents.
Unlike in Experiment 1, the Bayesian estimates derived from the Experiment 2 data match the observed pronoun interpretation data more closely than the other two models. Also, Experiment 2 again finds no evidence in favor of a dependence between predictability and pronominalization, lending support for the strong form of the Bayesian Model.
These results suggest that the Bayesian Model’s poor fit for the observed pronoun interpretation data in Experiment 1 was likely not due to properties intrinsic to the benefactive construction, but rather to the number of human event participants in the prompts. Since Bayesian reasoning relies heavily on expectations about the upcoming discourse, it could be the case that the prompts in Experiment 1 were too complex–due to their introduction of three discourse-new event participants with no other supporting context–to enable participants to create a sufficiently rich mental representation to allow for fully rational reasoning processes to take hold. The Mirror Model might then function as a sort of ‘default’ pronoun interpretation strategy: If participants are unable to appropriately track priors and default to the uniform distribution over the three human event participants, the Bayesian and Mirror Models make the same predictions.
In fact, previous authors have worried about the limits of the passage completion paradigm with single-sentence contexts in this respect. For instance, in their analysis of predictability on pronoun production rates, Rosa and Arnold (2017) argued that a more richly contextualized paradigm than that offered by a simple passage completion task might facilitate the development of a richer discourse representation on the part of the participant. Whereas we opted not to adopt the type of continued-story task they used in their Experiment 1 (we return to this point in the General Discussion), we agree that contexts that support richer discourse representations might better approximate natural language understanding scenarios, particularly when constructions as syntactically and semantically complex as benefactives are involved. To test this potential explanation, in Experiment 3 we return to employing benefactive prompts with three human event participants, but provide more context to facilitate the building of a mental representation of the discourse.
In Experiment 3, like in Experiment 1, we use benefactive prompts with three human event participants, but use descriptive NPs instead of proper names. In addition, we add both a verbal and visual context to help participants build a mental representation of the situation.
Participants were recruited through Amazon Mechanical Turk. 157 monolingual speakers of English completed the experiment and wrote correct continuations for the catch trials (see Materials) (mean age 38.5, age range 20–71, 67 women, 2 participants preferred not to supply their gender identity). All participants were paid in exchange for their participation ($10).
Similar to Experiment 1, the stimuli consisted of a target sentence featuring three human event participants: a subject, a direct object, and a benefactive. This time, however, the referents were referred to using descriptive NPs (instead of proper names) and the target sentences followed a two-sentence context; the first sentence introduced the three event participants and the second provided a scene-setting transition that didn’t mention any event participants (see Figure 3). In the first sentence, the referents were introduced as conjoined NPs and thus had the same grammatical and thematic role. This was done to avoid effects of the linguistic context on next-mention biases as much as possible. Right above the sentences, images of the referents were displayed, along with the corresponding descriptive NPs.6 The order of the images corresponded to the surface order of the referents in both the context and the target sentence.
As in Experiment 1, we manipulated which two referents were gender-matched (NP1&NP2, NP1&NP3, NP2&NP3) and whether the prompt ended in a full stop or a pronoun. Pronoun prompts were ambiguous between two of the three referents (she in the sample item in Figure 3). The stimuli were distributed over 6 lists, interspersed with 30 fillers that were similar in length and composition to the target fillers and the 2 catch fillers used in Experiments 1 and 2, adapted to match the other experimental items.7
The task setup and the subsequent annotation followed that of Experiments 1 and 2.
The analysis followed that of Experiment 1, which also had three referents and a manipulation of Ambiguous Pair.
As in Experiments 1 and 2, there are more NP1 continuations following pronoun prompts than following full stop prompts (β = 1.63, SE = 0.18, z = 9.17, p < 0.001), as shown in Table 9. When it comes to the predictability of the referents (measured in the full stop prompts), the results follow those of Experiment 1: There is again a main effect of Referent (reflecting the bias away from NP1 towards NP2 and NP3; p < 0.01), no main effect of Ambiguous Pair (p = 0.12) and a Referent × Ambiguous Pair interaction (p < 0.01), whereby the re-mention rates of NP2 and NP3 generally differ more across the ambiguous pair conditions than does that of NP1, see Table 10. Unlike in Experiment 1, the follow-up analyses show no main effect of Ambiguous Pair in any of the Referent subsets (NP1 p = 0.94, NP2 p = 0.17, NP3 p = 0.32), indicating that the interaction is only apparent at the level of the whole dataset.
TABLE 10. Proportion of next-mention in Experiment 3, per referent, per prompt type, per ambiguous pair. The values in each column sum to one (e.g., the re-mention rates in the full-stop NP1&NP2 condition are distributed 0.22/.39/.39 across the three referents).
As in Experiment 1, the pronominalization rates of the referents do not differ between ambiguous pairs (p = 0.13), and the interaction between Ambiguous Pair and Referent is also not significant (p = 0.20). What does influence the rates of pronominalization is the grammatical role of the referent (p < 0.001), as shown in Tables 11 and 12: NP1 is pronominalized more than NP2 (β = 3.67, SE = 0.44, z = 8.27, p < 0.001) and NP3 (β = 4.37, SE = 0.68, z = 6.42, p < 0.001). There is no difference in pronominalization rate between NP2 and NP3 (β = 1.16, SE = 0.95, z = 1.23, p = 0.20). Since differences in re-mention rates are not matched by differences in pronominalization, we again find no evidence of predictability influencing choice of referring expression.
TABLE 11. Proportion of pronominalization overall and for ambiguous vs unambiguous referents in Experiment 3 in the full stop prompt condition.
TABLE 12. Proportion of pronominalization of ambiguous referents in the full stop prompt items in Experiment 3, per referent, per ambiguous pair.
For the effect of referent ambiguity on pronoun production, as in Experiment 1, we find that the unambiguous referents were more often pronominalized than ambiguous referents (β = 0.54, SE = 0.22, z = 2.40, p < 0.05). This effect is significant alongside a significant main effect of referent (p < 0.001) whereby NP1 is pronominalized more than the other two referents. The interaction between Ambiguity and Referent was not significant; see Table 11.
Looking at the correlations between the model estimates and the observed pronoun interpretations, we find that in this experiment, like in Experiment 2, the Bayesian Model makes the best predictions; see Table 13 and Figure 4.
TABLE 13. Correlations between observed data and model predictions in Experiment 3, by items. * indicates significance at or below 0.001.
FIGURE 4. By-item observed values and model estimates for each referent in each ambiguous pair in Experiment 3. Tilted squares indicate the means. Note: the medians at the extremes (0 and 1) in the graphs for the NP1&NP3 ambiguous pair arise in part due to the relatively low number of observations on which the model estimates are based for the NP1 and NP3 referents. In the NP1&NP3 condition, the majority of continuations were about the NP2 referent, who does not figure into the calculations here.
The results from Experiment 3, like the results from Experiments 1 and 2, indicate that the prior and the likelihood are driven by different factors, as captured by the strong form of the Bayesian Model. Unlike in Experiment 1, the Bayesian Model is indeed the best fit for the observed pronoun interpretation data in Experiment 3. The crucial difference between Experiments 1 and 3 was how much contextual information was offered alongside the prompts participants were asked to continue. Whereas in Experiment 1, participants were asked to continue prompts in isolation featuring three human event participants introduced by proper names, in Experiment 3 the referents were introduced using descriptive role nouns and embedded in a longer passage with more discourse context. In addition, the prompts were accompanied by both a verbal and a visual context. The fact that the Bayesian Model outperformed the Mirror Model in this experiment suggests that predictability played a bigger role in interpreting the ambiguous pronouns in Experiment 3 than in Experiment 1.
As in Experiments 1 and 2, participants again showed a bias away from NP1 in their next mention preferences, a feature of the benefactive contexts that is useful for testing the competing models because they make different predictions in such cases. We note that Experiments 1 and 3 differ in their bias to NP2 versus NP3. This difference likely reflects the fact that the materials for the two experiments are quite different: They use different verbs and Experiment 3 contains short preceding discourse contexts, descriptive role nouns, and visual context.
Regarding the rates of pronominalization across argument/adjunct positions, the results from Experiment 3 follow Experiment 1 in providing no support for the proposed alternative explanation of the previously reported effects of thematic role on pronominalization. For both NP1 and NP2, there is no increase in the pronominalization rate between the condition where the competing gender-matched referent is an argument (the NP1&NP2 condition) and the condition where the competing referent is an adjunct (the NP1&NP3 condition for NP1 and the NP2&NP3 condition for NP2); rather there is a numeric decrease.
Regarding ambiguity, Experiment 3, like Experiment 1, shows that ambiguity appears to play a role in pronoun production. Again, participants produced more unambiguous than ambiguous pronouns, an effect that did not differ between the different referents. As in Experiment 1, however, the effect of pronoun ambiguity was small; see Table 11.
Three experiments were conducted to evaluate the predictions of the Bayesian Model of pronoun use against those of two competing models: the Mirror Model, which derives an interpretation bias from the hearer’s estimates of which entities the speaker is most likely to refer to with a pronoun, and the Expectancy Model, which derives an interpretation bias from a hearer’s predictions about what entities the speaker is most likely to mention next. Previous work has supported the predictions of the weak Bayesian Model in passage completions with implicit causality contexts (Rohde and Kehler, 2014; Kehler and Rohde, 2019), whereby Bayes-derived estimates of pronoun interpretation behavior yielded the best fit to participants’ observed behavior when compared to those of the competing models. The current work extends the range of context types evaluated to include benefactive contexts, which mention three event participants rather than two. Interestingly, the Mirror Model yielded the best fit in Experiment 1, raising the question of what property of the target passages overrode the good fit achieved by the Bayesian Model in prior work: Was it the increased complexity from having three characters involved in the bias estimation process, or was it something about the benefactive construction itself? Experiment 2 used the benefactive construction again but reduced the number of event participants that are compatible with gendered personal pronouns he/she to two. The results revealed that the Bayesian Model has the best fit, suggesting that it was not the benefactive construction that derailed the Bayesian Model in Experiment 1. Experiment 3 then tested benefactive passages with three human event participants again, this time with enriched contexts including characters described with role nouns, a longer verbal context, and a visual context with images that corresponded to each event participant. In this more situated task, the Bayesian Model yielded the best fit.
These studies also lend support to a prediction of the strong Bayesian Model, revealing that the factors that influence referent re-mention are different from those that influence referent pronominalization. This pattern was evident in all three experiments, whereby re-mention biases consistently favored non-subjects and pronominalization biases consistently favored subjects. An example of this dissociation is provided by the results of Experiment 1, where there was no evidence of dependence between predictability and pronominalization: The re-mention rate of NP3 is higher than NP2 but the pronominalization rates do not differ between them, and conversely the re-mention rates of NP1 and NP2 do not differ but their pronominalization rates do. These findings uphold the strong Bayesian hypothesis.
The two experiments whose setups are most similar are Experiments 1 and 3, but they show several differences in the coreference behavior they give rise to. Overall the rate of pronominalization was higher in Experiment 1 than 3, perhaps reflecting the difficulty of tracking too many unanchored proper names in Experiment 1. Moreover, the referent who was favored for re-mention also differed. Whereas Experiment 1 favored NP3, the Beneficiary, Experiment 3 favored NP2, the Patient. This divergence may be due to the different verbs used across experiments or simply the cognitive availability of the referents for re-mention. Experiment 1 favored re-mention of NP3, often as part of an explanation of the event (e.g., Why did Ben follow David for Sophia? Because Sophia wanted to know what David has been doing), whereas Experiment 3 favored re-mention of NP2, possibly because the role nouns in the passages made the NP3 referent more peripheral to the situation (e.g., The security guard followed the alleged shoplifter for the store manager, with continuations about what happened to the two main characters involved in the scene: The shoplifter tried to run but the guard tackled him or The security guard stopped the shoplifter in the parking lot before she could get into her car). This comparison demonstrates how a variety of contextual factors–some of which might at first blush appear subtle or even inert–can have strong semantic and pragmatic effects on expectations about what event participants are most likely to be mentioned next. These effects on the prior in turn affect biases with respect to pronoun interpretation, as predicted by the Bayesian Model.
As we have discussed, the model fits likewise differed between Experiments 1 and 3, with the best fits being achieved by the Mirror and Bayesian Models respectively. A possible explanation for this difference is that Bayesian reasoning requires participants to have a sufficiently fine-grained mental model of the situation in order to engage in the estimation of both referent predictability and pronoun production likelihood, so as to combine them when interpreting a pronoun. Of these two components, there can be little doubt that the estimation of the prior is the more complex, as any of a number of factors that draw on semantics, pragmatics, world knowledge, and inference will come into play in predicting what the ensuing message is likely to be. The production bias, in being primarily governed by grammatical (e.g., subjecthood) and information structural (e.g., topichood) factors, does not similarly require an exploration of the (virtually infinite) ways in which a discourse might be continued in terms of content. With the more complex demands associated with making predictions from the short, one-sentence contexts in Experiment 1 that nonetheless introduced three new discourse participants with no additional information to ground them, it could be that participants proceeded with poor estimates of the priors, or even fell back on the uniform distribution. When the prior is uninformative, the Bayesian Model makes the same predictions as the Mirror Model. However, while the Bayesian Model achieved the best fit for the observed data in Experiment 3, it is clear from both the correlations (see Table 13) and the graphs from Figure 4 that the Mirror Model was a close competitor. If the poor fit of the Bayesian Model in Experiment 1 was indeed due to participants being unable to estimate a reliable prior, the enriched contexts in Experiment 3 still seem to have been fairly limited in helping them do so. Compared to natural language use, even the context provided by our more situated prompts is, of course, fairly insubstantial. The hypothesis that Bayesian reasoning requires enough context for language users to build a sufficient mental representation of the situation, especially when situations get more complex (for instance with more than two referents to keep track of), should be further tested in future work.
In addition to testing the predictions of the Bayesian Model, the data from Experiments 1 and 3 also provided an opportunity to consider the role of referential ambiguity in a speaker’s choice about whether to use a pronoun. Recall that the contexts in both experiments provided three potential referents, one of which could be referred to with a gender-unambiguous pronoun in the free prompt condition (for instance, NP3 in the NP1&NP2 condition) and two that would require a gender-ambiguous pronoun (NP1 and NP2 in the NP1&NP2 condition). Our comparison of the pronominalization rates of referents when they were and were not part of the pair sharing the same gender showed that ambiguity does indeed have an effect. That having been said, on an account in which likelihood of pronominalization is dependent on referential ambiguity (Hendriks et al., 2014; Horton and Keysar 1996; Matthews et al., 2006; Nadig and Sedivy 2002, though cf.; Fukumura and van Gompel 2012; Arnold and Griffin 2007), one might expect to see higher rates of pronominalization in contexts in which the referent can be referred to unambiguously, since referential success in such contexts is not at stake. What we see instead, however, is a remarkable similarity in pronominalization rates across the unambiguous and ambiguous cases. If ambiguity avoidance is as influential as grammatical role, for instance, one might expect to see an effect of similar magnitude. Instead, the effect of ambiguity, while significant, does not rival grammatical role in effect size. Such results raise the question of why ambiguity effects emerge but are far smaller than what an ambiguity avoidance account might predict.
Finally, we note that research on the Bayesian Model has primarily focused on two context types, Source-Goal transfer-of-possession verbs and object-biased implicit causality verbs. This is for good reason: Whereas in most contexts the next-mention and pronominalization biases are likely to both favor the subject, the next-mention biases for these two constructions point away from the subject, thereby providing an opportunity to study divergences between production biases that favor the subject and next-mention biases that favor a non-subject. A result of the current study is the identification of benefactives as a third construction type of this sort, whereby the re-mention rate of NP3 was consistently higher than that of NP1 (albeit lower than NP2 in Experiment 3). We see two potential explanations for the high next-mention bias to NP3. The first bears on the meaning of the benefactive construction and its role in generating discourse expectations. In the case of object-biased implicit causality verbs, the hypothesis is that these verbs have the ability both to generate an expectation for an ensuing explanation and to impute causality to the direct object, thereby creating an expectation that the object will be mentioned next. In the case of Source-Goal transfer-of-possession verbs, the bias plausibly results from an expectation that the speaker will next describe what the recipient did with the object-of-transfer they just received. It could be that benefactives generate a high next-mention bias to NP3 for similar reasons, e.g., by creating an expectation that the speaker will next describe why the beneficiary would want the event to be performed or how the beneficiary reacted to the event that was performed on their behalf.
As pointed out by a reviewer, however, another possible explanation stems from the fact that the NP3 argument is optional in the benefactive construction. Arnold (2001) previously compared next-mention biases within Source–Goal and Goal–Source transfer-of-possession contexts, and found that non-subject (Source) referents were re-mentioned unexpectedly often in Goal–Source cases. Unlike Source–Goal sentences, in which all three thematic roles are presented in obligatory arguments, the Source is optional in Goal–Source sentences (e.g., Mary received the book from Sue and Mary received the book are both acceptable). Arnold hypothesized that participants may have felt the need to re-mention the Source in the continuation in order to justify its inclusion in the story. In a study that compared active and passive IC contexts, Rohde and Kehler (2014) similarly found that the re-mention rate of the logical subject in their free-prompt condition was higher in passive contexts–where it is mentioned from within an optional by-adjunct–than in the active condition, and followed Arnold in speculating that the optionality of including the by-adjunct was the reason for the effect. As such, it is possible that the bias toward NP3 in benefactives is due to the same reason. The results presented here do not inform the question of which explanation is correct, but whichever one proves to be, benefactives can be added to the list of context types capable of evaluating claims concerning the dissociation between pronoun production and interpretation biases.
Our results using benefactive contexts largely revealed that the types of semantic factors that affect next-mention biases do not similarly affect production biases, in line with recent work using IC contexts, but in contrast with Rosa and Arnold (2017) results on transfer-of-possession. One of our goals was to evaluate a hypothesis expressed in previous work (Fukumura and Van Gompel, 2010; Rohde and Kehler, 2014) that the effects found for transfer contexts may be due to the imbalance between the argument status of the Goal in Source-Goal frames and adjunct status of the Source in Goal-Source frames. Whereas we investigated this question in the context of benefactive instead of transfer contexts, our results do not support that explanation of Rosa and Arnold’s results: Whereas in Experiment 1 the pronominalization rate of NP2 went up slightly when the competing referent was an adjunct compared to an argument, the effect wasn’t significant, and in the cases of NP1 in Experiment 1 and both NP1 and NP2 in Experiment 3, the differences went numerically in the wrong direction.
This leads us to wonder about other explanations for the effects found by Rosa and Arnold. One obvious possibility is that the results are sound, and that the strong form of the Bayesian analysis is, well, too strong. This conclusion would of course be welcome if it captures the reality of the facts, and would not itself provide any evidence against the weak form of the hypothesis. It should nonetheless give us pause in light of our current state of knowledge, however, since effects of predictability have been not been found in IC contexts nor (now) benefactive contexts. The most obvious explanation for why predictability would affect pronominalization is the rationale behind the common wisdom outlined in the introduction, whereby the speaker and hearer are coordinating via a singular notion of entity salience when producing and interpreting a pronoun respectively. The recent data however, when considered as an ensemble, provides little evidence to support that view: no effect of predictability has been found for IC and benefactive contexts, and the effects reported for transfer-of-possessive contexts are smaller and more varied than this explanation would predict. We are thus left with the question of what type of model would predict this mixed pattern of effects.
Further commentary must necessarily remain speculative. The primary support for an effect of thematic role on pronominalization comes from Rosa and Arnold’s first experiment, where an effect for both grammatical roles was found, albeit much more strongly for subjects.8 Their Experiment 1 utilized a paradigm in which the stimuli were presented as a continuous story, which carries with it complications that one does not find in the standard passage completion paradigm. In particular, while the continuous story paradigm clearly does not affect theoretical predictions regarding the effect of grammatical role on pronominalization, it is much less clear that the same is true for theories that tie pronominalization rates to topicality, since inferences about the relative topicality of referents can be affected by any of a number of factors as the mental models of the interlocutors evolve throughout a discourse. The fact that participants themselves produced half of the utterances that comprised each discourse means that each discourse was unique, and hence the topicality status of potential referents at different points in the discourse would be expected to vary as well. This worry receives support from the fact that Rosa and Arnold found a significant effect of stimulus order: Two lists were employed, and which list a participant saw reliably affected their pronominalization rates, despite the fact that the individual prompts were the same. In contrast, while the design of our Experiment 3 followed Rosa and Arnold in using more extended contexts, care was taken to control for topicality: The three event participants were introduced from a coordinate noun phrase that offered no topicality advantage for any potential referent, with an intervening scene-setting clause that did not mention any of them. Using these carefully constructed discourses that were nonetheless richer than the single-sentence contexts used in our Experiment 1, the expected effects of semantic factors on next-mention biases were found, but no effects of these factors were found on production biases. An obvious next step for future work is to examine transfer contexts with extended, albeit more carefully controlled, stimuli.9
In sum, the results presented here demonstrate that the Bayesian Model scales well from its previous applications to a new domain: benefactive constructions with two or three human event participants. However, this was only true in the three event participant case when a verbal and visual context was present to (by hypothesis) allow participants to track the available referents and build an adequate mental representation of the situation being described. This hypothesis, of course, immediately evokes questions for future work: For instance, do all language users use Bayesian reasoning when faced with ambiguous pronouns, regardless of mental capacity or task demands? Indeed, there is evidence that not everyone can always engage in predictive processing [e.g., children, non-native speakers, and non-student and older adults (Huettig, 2015; Pickering and Gambi, 2018; Grüter et al., 2012)]. For example, non-native speakers don’t make the same coreference predictions that native speakers do in contexts with transfer-of-possession verbs, a finding that has been attributed to the increased difficulty of real-time next-mention computations during second language processing (Grüter and Rohde, 2021). Further research can thus shed light on whether our hypothesis regarding the differences witnessed in Experiments 1 and 3 is on the right track.
The datasets presented in this study can be found in online repositories. The names of the repository/repositories and accession number(s) can be found below: https://tinyurl.com/BenefactivesFrontiers.
The studies involving human participants were reviewed and approved by School of Philosophy, Psychology, and Languages Sciences ethics panel, University of Edinburgh. The patients/participants provided their written informed consent to participate in this study.
JH contributed to conceptualisation, methodology, analysis, and writing—original draft. HR and AK contributed to design, methodology, and writing—review and editing.
The authors declare that the research was conducted in the absence of any commercial or financial relationships that could be construed as a potential conflict of interest.
All claims expressed in this article are solely those of the authors and do not necessarily represent those of their affiliated organizations, or those of the publisher, the editors and the reviewers. Any product that may be evaluated in this article, or claim that may be made by its manufacturer, is not guaranteed or endorsed by the publisher.
We thank Joseph Cohen and Emily Williamson for help in annotation. This work was supported by a Leverhulme Trust Prize in Languages and Literatures to HR.
1We use ‘speaker’ to generally refer to language producers and ‘hearers’ to generally refer to language comprehenders.
2Patterson, C., Schumacher, P. B., Nicenboim, B., Hagen, J., and Kehler, A. (2021). A Bayesian Approach to German Personal and Demonstrative Pronouns. Submitted for publication.
3Rosa and Arnold report reliable effects for their Exp 2, but it is clear from their descriptive statistics that no effect exists for the condition of interest for evaluating the predictions of the strong Bayesian Model, in which the two event participants are of the same gender and hence reference is ambiguous. Here the pronominalization rates for subjects were identical (69% for both Goal and Source antecedents), and only negligibly different for non-subjects and in the wrong direction (18% for Goals and 19% for Sources).
4All materials and analysis scripts can be found at https://tinyurl.com/BenefactivesFrontiers.
5All materials and analysis scripts can be found at https://tinyurl.com/BenefactivesFrontiers.
6The images were adapted from images from the open source illustration website https://undraw.co.
7All materials and analysis scripts can be found at https://tinyurl.com/BenefactivesFrontiers.
8As mentioned earlier, Rosa and Arnold’s Exp 2 yielded no apparent effect for gender-ambiguous contexts like those studied here and in previous work, and Experiment 3 revealed a small effect for non-subjects only. There is a potential worry concerning the results of both Exps. 2 and 3, however, in that role nouns were used without clip art to disambiguate gender, as used in their Experiment 1 and the studies presented here. This means that one cannot be sure what contexts were viewed by participants to be gender ambiguous vs unambiguous. This worry receives support from the fact that Rosa and Arnold saw cases of this based on the nature of the continuations that participants provided, which led them to recategorize the gender of two of their characters post-hoc.
9Indeed, there are other aspects of Rosa and Arnold’s stimuli that could potentially be cause for concern. For one, an examination of their stimuli suggests that in some context sentences, the event participants were introduced with different referential forms, varying among proper names, definite lexical NPs, and indefinite NPs (e.g., The maid handed a piece of cake to Sir Barnes; Sir Barnes bought earrings from a sales clerk). Information structure theorists have posited that form of reference, like grammatical role, influences the likelihood of an entity being the topic, with pronominalized antecedents being the strongest indicator, followed by other definites (proper names; the-NPs), and finally with indefinites being the poorest prospects (Lambrecht, 1994, p. 165, inter alia). Thus, mixing these forms across potential referents in a single context sentence potentially creates a confound. There are also other irregularities of smaller scope. First, included are transfer verbs that appear in the double object construction (The maid gave Lady Mannerly a glass of champagne; Lady Mannerly handed the maid a duster and a broom). The hypothesis that topicality conditions pronominalization rates does not treat Goals introduced as indirect objects to be on a par with those introduced as the object of a PP (with indirect objects being more topical, by virtue of their being higher on the obliqueness hierarchy), and no double object construction is available for the corresponding Goal-Source transfer verbs (* Lady Mannerly received the maid a glass of champagne). Second, some sentences we understand as being part of the stimuli are not transfer-of-possession verbs at all (ex 3b, Lady Mannerly gave a backrub to Sir Barnes)—such cases do not create an expectation that the next sentence will describe what the Goal did next with the object of transfer–and others only involve transfer-of-possession in an abstract, metaphorical sense (e.g., The chauffeur taught shooting techniques to the butler). Third, at least one stimulus–Sir Barnes received a painting of the two of them from Lady Mannerly, given in Figure 2 of the paper–contains a pronoun that refers to both participants, one anaphorically and one cataphorically. This should be avoided, since additional mentions can influence the salience and topicality status of event participants beyond the mentions that fill the grammatical and thematic roles under scrutiny. Finally, some verbs occurred multiple times in the same stimulus set (e.g., handed occurs seven times by our count), and verb re-use is not balanced between the Source-Goal and Goal-Source contexts. We of course cannot say for sure that any or all of these factors influenced the effects found, but do nonetheless suggest that a follow-on study that remedies these issues is in order.
Arnold, J. E., and Griffin, Z. M. (2007). The Effect of Additional Characters on Choice of Referring Expression: Everyone Counts. J. Mem. Lang. 56, 521–536. doi:10.1016/j.jml.2006.09.007
Arnold, J. E. (1998). Reference Form and Discourse Patterns. Ph.D. thesis. Stanford, CA: Stanford University.
Arnold, J. E. (2008). Reference Production: Production-Internal and Addressee-Oriented Processes. Lang. Cogn. Process. 23, 495–527. doi:10.1080/01690960801920099
Arnold, J. E. (2001). The Effect of Thematic Roles on Pronoun Use and Frequency of Reference Continuation. Discourse Process. 31, 137–162. doi:10.1207/s15326950dp3102_02
Barr, D. J., Levy, R., Scheepers, C., and Tily, H. J. (2013). Random Effects Structure for Confirmatory Hypothesis Testing: Keep it Maximal. J. Mem. Lang. 68, 255–278. doi:10.1016/j.jml.2012.11.001
Bates, D., Mächler, M., Bolker, B., and Walker, S. (2015). Fitting Linear Mixed-Effects Models Using Lme4. J. Stat. Softw. 67, 1–48. doi:10.18637/jss.v067.i01
Cheng, W., and Almor, A. (2019). A Bayesian Approach to Establishing Coreference in Second Language Discourse: Evidence from Implicit Causality and Consequentiality Verbs. Bilingualism 22, 456–475. doi:10.1017/s136672891800055x
Fukumura, K., and Van Gompel, R. P. G. (2010). Choosing Anaphoric Expressions: Do People Take into Account Likelihood of Reference?. J. Mem. Lang. 62, 52–66. doi:10.1016/j.jml.2009.09.001
Fukumura, K., Van Gompel, R. P. G., Harley, T., and Pickering, M. J. (2011). How Does Similarity-Based Interference Affect the Choice of Referring Expression?. J. Mem. Lang. 65, 331–344. doi:10.1016/j.jml.2011.06.001
Fukumura, K., and van Gompel, R. P. G. (2012). Producing Pronouns and Definite Noun Phrases: Do Speakers Use the Addressee's Discourse Model?. Cogn. Sci. 36, 1289–1311. doi:10.1111/j.1551-6709.2012.01255.x
Givón, T. (1983). Topic Continuity in Discourse: A Quantitative Cross-Language Study, Vol. 3. Amsterdam: John Benjamins Publishing.
Grosz, B. J., Joshi, A. K., and Weinstein, S. (1995). Centering: A Framework for Modelling the Local Coherence of Discourse. Comput. Linguistics 21, 203–225. doi:10.21236/ada324949
Grüter, T., Lew-Williams, C., and Fernald, A. (2012). Grammatical Gender in L2: A Production or a Real-Time Processing Problem?. Second Lang. Res. 28, 191–215. doi:10.1177/0267658312437990
Grüter, T., and Rohde, H. (2021). Limits on Expectation-Based Processing: Use of Grammatical Aspect for Co-Reference in L2. Appl. Psycholinguistics 42, 51–75. doi:10.1017/s0142716420000582
Gundel, J. K., Hedberg, N., and Zacharski, R. (1993). Cognitive Status and the Form of Referring Expressions in Discourse. Language 69, 274–307. doi:10.2307/416535
Hendriks, P., Koster, C., and Hoeks, J. C. J. (2014). Referential Choice Across the Lifespan: Why Children and Elderly Adults Produce Ambiguous Pronouns. Lang. Cogn. Neurosci. 29, 391–407. doi:10.1080/01690965.2013.766356
Horton, W. S., and Keysar, B. (1996). When Do Speakers Take into Account Common Ground?. Cognition 59, 91–117. doi:10.1016/0010-0277(96)81418-1
Huettig, F. (2015). Four central Questions about Prediction in Language Processing. Brain Res. 1626, 118–135. doi:10.1016/j.brainres.2015.02.014
Jaeger, T. F. (2008). Categorical Data Analysis: Away from ANOVAs (Transformation or Not) and Towards Logit Mixed Models. J. Mem. Lang. 59, 434–446. doi:10.1016/j.jml.2007.11.007
Kehler, A., Kertz, L., Rohde, H., and Elman, J. L. (2008). Coherence and Coreference Revisited. J. Semant 25, 1–44. doi:10.1093/jos/ffm018
Kehler, A., and Rohde, H. (2013). A Probabilistic Reconciliation of Coherence-Driven and Centering-Driven Theories of Pronoun Interpretation. Theor. Linguistics 39, 1–37. doi:10.1515/tl-2013-0001
Kehler, A., and Rohde, H. (2019). Prominence and Coherence in a Bayesian Theory of Pronoun Interpretation. J. Pragmatics 154, 63–78. doi:10.1016/j.pragma.2018.04.006
Lam, S. Y., and Hwang, H. (2021). “Interpretation of Null Pronouns in Mandarin Chinese Does Not Follow a Bayesian Model,” in Paper presented at the 34th Annual CUNY Conference on Human Sentence Processing. Philadelphia, United States.
Lambrecht, K. (1994). Information Structure and Sentence Form. Cambridge: Cambridge University Press.
Matthews, D., Lieven, E., Theakston, A., and Tomasello, M. (2006). The Effect of Perceptual Availability and Prior Discourse on Young Children's Use of Referring Expressions. Appl. Psycholinguistics 27, 403–422. doi:10.1017/s0142716406060334
Mayol, L. (2018). Asymmetries between Interpretation and Production in Catalan Pronouns. Dialogue & Discourse 9 (2), 1–34. doi:10.5087/dad.2018.201
Nadig, A. S., and Sedivy, J. C. (2002). Evidence of Perspective-Taking Constraints in Children's On-Line Reference Resolution. Psychol. Sci. 13, 329–336. doi:10.1111/j.0956-7976.2002.00460.x
Pickering, M. J., and Gambi, C. (2018). Predicting while Comprehending Language: A Theory and Review. Psychol. Bull. 144, 1002–1044. doi:10.1037/bul0000158
R Core Team (2019). R: A Language and Environment for Statistical Computing. Vienna, Austria: R Foundation for Statistical Computing.
Rohde, H. (2008). Coherence-Driven Effects in Sentence and Discourse Processing. Ph.D. thesis. UC San Diego.
Rohde, H., and Kehler, A. (2014). Grammatical and Information-Structural Influences on Pronoun Production. Lang. Cogn. Neurosci. 29, 912–927. doi:10.1080/01690965.2013.854918
Rosa, E. C., and Arnold, J. E. (2017). Predictability Affects Production: Thematic Roles Can Affect Reference Form Selection. J. Mem. Lang. 94, 43–60. doi:10.1016/j.jml.2016.07.007
Stevenson, R. J., Crawley, R. A., and Kleinman, D. (1994). Thematic Roles, Focus and the Representation of Events. Lang. Cogn. Process. 9, 519–548. doi:10.1080/01690969408402130
Keywords: coreference, pronoun production, pronoun interpretation, benefactives, ambiguity, bayesian coreference
Citation: Hoek J, Kehler A and Rohde H (2021) Pronominalization and Expectations for Re-Mention: Modeling Coreference in Contexts With Three Referents. Front. Commun. 6:674126. doi: 10.3389/fcomm.2021.674126
Received: 28 February 2021; Accepted: 01 September 2021;
Published: 15 October 2021.
Edited by:
Juhani Järvikivi, University of Alberta, CanadaReviewed by:
Torgrim Solstad, Bielefeld University, GermanyCopyright © 2021 Hoek , Kehler and Rohde. This is an open-access article distributed under the terms of the Creative Commons Attribution License (CC BY). The use, distribution or reproduction in other forums is permitted, provided the original author(s) and the copyright owner(s) are credited and that the original publication in this journal is cited, in accordance with accepted academic practice. No use, distribution or reproduction is permitted which does not comply with these terms.
*Correspondence: Hannah Rohde , aGFubmFoLnJvaGRlQGVkLmFjLnVr
Disclaimer: All claims expressed in this article are solely those of the authors and do not necessarily represent those of their affiliated organizations, or those of the publisher, the editors and the reviewers. Any product that may be evaluated in this article or claim that may be made by its manufacturer is not guaranteed or endorsed by the publisher.
Research integrity at Frontiers
Learn more about the work of our research integrity team to safeguard the quality of each article we publish.