- 1The BrainTrain Project, Department of Psychology, Åbo Akademi University, Turku, Finland
- 2Turku Brain and Mind Center, University of Turku, Turku, Finland
During the past decades, working memory (WM) training has attracted considerable research attention, but its transfer to untrained tasks is still controversial. In a randomized controlled trial, we investigated the possible transfer effects of a novel sentence-level WM training regime. Sixty-eight healthy Finnish adults were randomized into either a WM training group or an active control group. The WM training group practiced for 4 weeks with two adaptive sentence-level WM training tasks, namely, a novel sentence-level updating task and a Reading span task. The active control group practiced on a quiz task that called for long-term memory but did not load on WM. There were no statistically significant training effects on the pre–post measures of near and far transfer. We suggest that the lack of training effects may reflect the specificity and automaticity of the sentence-processing system.
Introduction
Working memory (WM) refers to a mental platform for temporary maintenance, access, manipulation, and coordination of information (Baddeley, 2000). It is a cornerstone for several important cognitive abilities, such as reasoning (Süß et al., 2002; Conway et al., 2003), executive control (Poole and Kane, 2009), and multitasking (Konig et al., 2005; Hambrick et al., 2010). The influence of WM extends even further as WM has been shown to be highly predictive of academic and professional success (Gathercole et al., 2004; Alloway and Alloway, 2010).
The key role of WM in cognition has motivated numerous intervention studies that have sought to improve WM abilities with intensive computerized training. Despite very promising early findings (e.g., Jaeggi et al., 2008; Chein and Morrison, 2010), recent meta-analyses on WM training indicate mainly near transfer (i.e., improvements in other, untrained WM tasks), while far-transfer effects (i.e., improvements on tasks tapping other cognitive domains) have been very small (Melby-Lervåg and Hulme, 2013; Melby-Lervåg et al., 2016; Soveri et al., 2017). The most recent meta-analysis (Soveri et al., 2017) examined the near-transfer effects in more detail by separating task-specific near transfer (untrained tasks representing the same task paradigm as the training task and differing only by stimuli) from task-general near transfer (untrained WM tasks structurally different from the training task). The results showed that WM training studies yield moderate task-specific near transfer, while task-general near-transfer effects are very small (Soveri et al., 2017). Thus, current evidence indicates that WM training produces quite specific and temporary task improvements that do not affect everyday cognitive performances (Melby-Lervåg et al., 2016).
Given the limited generalizability of WM training, one way to move forward would be to start employing WM training tasks that bear more similarity to everyday cognitive challenges. Several researchers have pointed out that current WM training tasks represent rather artificial laboratory-based tasks employing random strings of digits, letters, words, or spatial positions (Klingberg, 2010; Shipstead et al., 2010; Holmes, 2011; Holmes and Gathercole, 2014; Moreau and Conway, 2014). For the present study, we designed a verbal WM training regime that employed meaningful sentences as training stimuli. Reading sentences is something most people do on a daily basis, and sentence comprehension correlates with performance on complex WM measures (for a meta-analysis, see Daneman and Merikle, 1996). Individuals with high vs. low WM capacity show both behavioral and neural differences when processing structurally demanding sentences (e.g., Prat et al., 2007). The ability to process textual information is related to academic achievements, financial success, and socioeconomic status, among others (Ritchie and Bates, 2013; Ricketts et al., 2014). These facts motivated the present attempt to study the possible effects of WM training at sentence level.
In the previous literature of sentence-level WM, the most commonly used WM task is the Reading span (RSpan) task (Daneman and Carpenter, 1980). In this task, participants are to simultaneously judge the semantic correctness of sentences and remember the final word in each sentence in correct order. It has previously been debated whether linguistic WM tasks, such as the RSpan task that entails syntactic processing, tap the same pool of verbal WM resources as tasks that lack syntactic structure, such as span repetition. Caplan and Waters (1999) put forth the dedicated resource hypothesis that separates syntactic processing from other verbal WM resources. Other researchers, on the other hand, have claimed for shared resources, where verbally mediated tasks call for the same pool of verbal WM resources irrespective of whether the stimuli tap syntactic processing or not (e.g., King and Just, 1991; Just and Carpenter, 1992). Both theories have found some support in subsequent studies (Gordon et al., 2002; Fedorenko et al., 2006). The mental architecture of verbal WM is nevertheless an important issue here, because the shared resources hypothesis would predict broader potential transfer effects after verbal WM training than the dedicated resource hypothesis. Sentence processing is a rapid and automatic process (Garrett, 1990; Kamide et al., 2003), suggesting that the cognitive demands in sentence-level verbal WM differ at least partly from those engaged by word, letter, or digit strings. Due to these differences, it is of interest to see if sentence-level WM training will show a somewhat different transfer pattern as has been seen in previous studies using unrelated verbal items.
Sentence repetition training has previously been administered primarily in aphasic patients, and the results indicate that WM training at sentence-level is beneficial for this clinical group (Francis et al., 2003; Koenig-Bruhin and Studer-Eichenberger, 2007; Eom and Sung, 2016). However, no previous WM training studies on healthy adults have employed exclusively sentences as training stimuli. A doctoral dissertation by Payne (2014) which was recently published (Payne and Stine-Morrow, 2017) comes closest. The study examined the effects of verbal WM training in healthy elderly on language performance by using three verbal WM training tasks, out of which one was a sentence-level task. Interestingly, the results showed selective improvements in offline language tasks in the training group compared with an active control group. The improvements were seen in sentence recall, comprehension of syntactically ambiguous sentences, and verbal fluency. The first two tasks that involve sentence processing bear relevance to the daily life as well.
The training regime developed for the present study included two adaptive sentence-level WM training tasks. The first one was the well-known RSpan task (Daneman and Carpenter, 1980). The second one was a novel sentence-level WM updating task, coined as the selective updating of sentences (SUS) task (Fellman et al., 2017). In this task, participants were to update semantically feasible sentences by selectively replacing some constituent words. We employed a randomized controlled design with an active control group that practiced with a computerized quiz task that called for long-term memory but did not load on WM. As WM training is expected to lead to improvements on both trained and structurally similar untrained WM tasks (Soveri et al., 2017), we included both task types in the pre–posttest battery. Due to the encouraging transfer effects reported by Payne and Stine-Morrow (2017) on offline language processing measures, we also employed three verbal WM tasks that were structurally different from the training tasks, and five far-transfer offline language measures (including sentence recall and word fluency employed by Payne and Stine-Morrow, 2017), even though task-general near transfer and far transfer after WM training is less likely.
Materials and Methods
Participants
Participants were recruited through an e-mail announcement sent to various student associations and student unions at the Universities and Polytechnics in Turku and Helsinki. The final sample consisted of 68 (51 women) monolingual healthy undergraduate students in the age range of 18–40 years (M = 24.58, SD = 3.95). The participants did not report any significant psychiatric or neurological illnesses. All participants completed a background questionnaire and were screened for language background. Moreover, all participants completed the Beck Depression Inventory-II (BDI-II; Beck et al., 2004). Participants who had been exposed to two (or more) languages before the age of 6 were excluded from the study, as well as those with BDI-II scores exceeding the cutoff score of 16. Those who had been drinking five or more units of alcohol (i.e., 50 g of pure alcohol or more) the day before the pretest were also excluded from the study. The participants who met the inclusion criterion were randomized to either a WM training group or an active control group, but they were not informed of the existence of the two groups. The groups did not differ in terms of age, t(66) < 1, p = 0.720, gender, χ2(1, N = 68) < 1, p = 0.866, years of education, t(66) < 1, p = 0.519, or BDI-II scores, t(66) < 1, p = 0.551 (see Table 1). All participants who successfully completed the whole study received a compensation of 70 euros. All research procedures were approved by the Institutional Review Board of the Departments of Psychology and Logopedics, Åbo Akademi University, and written informed consent was obtained from all participants.
Procedure
The study comprised three phases: a pretest session, a training period, and a posttest session. A flowchart depicting the study phases and dropout rates is shown in Figure 1. A total of 101 participants took part in the pretest session and underwent screening. Of those, 19 participants were discarded as they did not meet the inclusion criteria. Moreover, nine participants in the WM training group withdrew during the training period, and two participants did the same in the active control group. Of the participants who successfully completed all stages in the study, two from the WM training group and one from the active control group were excluded from the final analyses due to other issues (see Figure 1). The final sample thus consisted of 68 participants.
The posttest session comprised the same tasks as the pretest session with the exception of the background questionnaire, the language background screening, and the BDI-II. When the participants had completed the posttest session, they were asked to retrospectively evaluate their motivation in the beginning, midway, and at the end of training on a 10-point Likert scale (1 = not at all motivated, 10 = highly motivated). The pre- and posttest sessions were run in computer classes with 1–12 participants. The test order was randomized for each participant in each session.
The training period started during the week after the pretest session. The participants practiced four times (30 min each) a week for 4 weeks, after which they completed the posttest session during the following week (week 6). The WM training group practiced on two computerized verbal WM tasks (see The Training Tasks), while the active control group trained with quiz tasks that called for verbal long-term memory but did not load on WM. Both the WM training group and the active control group performed their training sessions at a peaceful place of their own choosing, for example, at home.
The Training Tasks
Selective Updating of Sentences Training Task
Based on the SUS task which has been shown to exhibit adequate psychometric properties (Fellman et al., 2017), we developed a sentence-level updating training task, coined as the selective updating of sentences training task (SUST). In the SUST, words were presented on the computer screen in a row of boxes. Each box contained one word, and conjointly they formed a meaningful sentence. The participant was to encode and keep in mind the initial sentence, after which it disappeared and a blank screen was shown for 500 ms, followed by an updating stage with a new row of boxes. At the updating stage, two of the boxes contained a new content word while the rest remained empty. The participant was prompted to replace the old words with the new ones while at the same time maintaining the unchanged words in the original sentence. Finally, a row of empty boxes appeared on the screen, and the participant was to type in the most recent version of the sentence, that is, the latest word in each box. The updates of the sentence constituents were pseudorandomized so that their positions could not be predicted.
In the SUST task, 40 difficulty levels were created. On the first eight levels, the sentences (and the updating stages) were presented for 4,000 ms, and the difficulty level was increased by adding more updating stages (range 1–8). If the participant successfully completed level 8, the sentence presentation time went down to 3,000 ms. The participant then proceeded with this exposure time through updating stages 1–8. The same adaptive procedure was applied at levels 17–24 (2,000 ms sentence presentation time), 25–32 (1,000 ms sentence presentation time), and 33–40 (500 ms sentence presentation time). At each difficulty level, the sentences appeared in blocks of four. In each block, the sentence length ranged from 4 to 7 words so that one trial of each sentence length was presented in all blocks. The participant had to type in the final words of a sentence verbatim in order to score a correct trial. To proceed to the next difficulty level, the participant had to respond correctly on at least three of the four trials in a block. If the participant responded correctly on one or two trials, the following block remained on the same level, and if the participant failed all trials within a block, the block level was decreased. Between the training sessions, we applied the n − 2 principle, that is, if the participant, for instance, reached level 5 on a training session, the next training session started from level 3.
The sentences included in the SUST task followed common sentence structures in the Finnish language, including predicative clauses, transitive clauses, intransitive clauses, and existential clauses. The sentence updates modified its semantic contents but did not change the syntactic structure. To avoid recognition and memorization of recurring words and sentences, we developed a sizable pool of sentences that comprised 640 unique trials (a single trial consisted of an initial sentence and its updating stages). Moreover, when the sentences were updated and replaced by other words, they still remained semantically and syntactically plausible. For example, in a sentence “The carpenter built a house” in which the words “carpenter” and “house” were updated, they were replaced by semantically adequate words such as “artisan” and “residence,” thus keeping the sentence meaningful.
Sentence Reading Span Training Task (SRST)
Following Daneman and Carpenter (1980), a Finnish SRST was created. Here the participant read a series of sentences, presented in sets of two or more sentences, and was asked to do two things. First, after reading each sentence, the participant was to judge whether the sentence was semantically acceptable. The second task was to memorize the last word of each sentence. The sentences were shown for up to 8,000 ms, and the next sentence appeared as soon as the participant had responded. At the end of each trial, the participant was to recall the last word of each sentence in the order that they were presented.
Altogether 500 different sentences were adopted and modified from the online quiz website Älypää (www.alypaa.com) used in the control group as training. In total, 250 sentences were identical to the questions presented on the website (e.g., Mitä maitoon pitää lisätä, jotta siitä saa tehtyä juustoa? “What do you have to add to milk to transform it to cheese?”) and 250 sentences were modified by transforming the questions from Älypää to declarative sentences (e.g., Oikeakätisen ihmisen kielikeskus sijaitsee vasemmalla puolella aivoja “A right-handed person has the speech center located in the left side of the brain”).
For each of the 500 acceptable sentences adopted from Älypää, a paired unacceptable sentence was developed on the basis of the “syntactic prose” conditions used in previous studies (Lee and Federmeier, 2012; Stites et al., 2013; Payne and Stine-Morrow, 2017). The unacceptable sentences were syntactically correct but lacked in semantic plausibility (e.g., F-18 Hornet hävittäjätyyppi on ravintolalaskun musta lentokone “The F-18 Hornet jet type is a restaurant bill’s black airplane”). The unacceptable sentences were created in a way that the participant had to read through the sentence before being able to judge its acceptability. The length of the sentences ranged between 60 and 100 characters, and the sentence-final words were between 4 and 14 characters.
The SRST task was adaptive, that is, the number of test items in a trial increased or decreased depending on the participant’s performance. Each level comprised a block of four trials and the participant had to score at least three trials correctly (i.e., correct on both the final words and the acceptability rating in each trial) to proceed to the next level. With 1–2 trials correct, the participant remained on the same level. The difficulty level was decreased by one if the participant failed all trials. As in the SUST task, the n − 2 principle was used to adjust the level of difficulty between training sessions. Due to the particularly rich morphology of the Finnish language, the participant did not have to recall the exact inflectional form of the final word but scored correct when the correct stem appeared in the response (e.g., typing in AUTO “car” or AUTOT “cars” for the target word form AUTOISSA “car” + plural marker + inessive case, “in the cars”).
The Training Task for the Active Control Group
The active control group played a free online quiz task called Älypää (www.alypaa.com; “classic” game mode). The game presented a question with four possible response alternatives, and the task was to choose the correct response alternative. The difficulty level of the questions increased for every correct consecutive answer. Participants played Älypää for 30 min during every training session. The game was selected on the basis of its limited WM demands, its general appeal to a wide audience, and its large pool of questions.
The Pre- and Posttests
WM Tasks
Selective Updating of Sentences Task
The overall setup was the same as in the SUST task, except that only the 4,000 ms sentence exposure time was used. Moreover, the words that were incorporated in the SUST task were not used in the pre–post task, thus eliminating possible word learning effects. The SUS task comprised 12 trials, each with an initial sentence followed by its updating stages. The order of the trials was randomized for each participant, and the updates of the sentence constituents were pseudorandomized so that their positions could not be predicted. The SUS trials were divided into three blocks. The first block comprised sentences with two updating stages, the second block sentences with three updating stages, and the third block sentences with five updating stages. All blocks included sentences ranging with four to seven words, one trial of each sentence length. One point was awarded for each correctly recalled word that was typed in the corresponding box. The percentage of correctly recalled words on all trials was used as the dependent variable.
RSpan Task
This task followed the SRST setup where, for each presented sentence, the participant was to make a semantic acceptability judgment and memorize the final word. In line with the SUS task, the words and sentences incorporated in the SRST training regime were not used in the RSpan pre–post task. At the end of each trial, the participant was to recall the last words by their order of presentation. Each sentence was shown on the screen for up to 8,000 ms. The next sentence appeared as soon as the participant had responded. The task included seven trials (with two to eight items to recall) with one trial per each sequence length, and the order of the trials was randomized. We used a partial-credit scoring system (Conway et al., 2005) where the number of correctly recalled elements per trial was counted, regardless of trial length (e.g., two correctly recalled elements from a three-element trial were worth as much as two correctly recalled elements from a five-element trial). In the intervening task, we used binomial probability that identified the cutoff score (one-tailed, p < 0.05) where there was less than 5% probability that the score would have been due to guessing. The binomial probability analysis revealed a cutoff score of ≥65.71% correctly solved problems in the RSpan task.
Operation Span (OSpan) Task
This task was based on Turner and Engle (1989). Here the participant was to make yes/no responses to simple math equations (e.g., 5 − 2 + 6 = 9?) while simultaneously trying to memorize a set of unrelated digits. The to-be-remembered digit was displayed on the computer screen for 1,000 ms, followed by a fixation point (asterisk) for 500 ms. After that, the equation appeared for 6,000 ms. At the end of each trial, a recall grid was shown, and the participant was to recall the digit sequence in the order it was presented. The participant completed six trials. The sequence lengths ranged between 4 and 9 (one trial per sequence length) and the order of the trials was randomized. We used the partial-credit scoring system following Conway et al. (2005) where the number of correctly recalled elements per trial was counted, regardless of trial length. In line with the RSpan task, we used a binomial probability analysis (one-tailed, p < 0.05) to define the cutoff value for the intervening task. The cutoff value was ≥66.67% correctly resolved problems in the OSpan task.
Alphabet WM Task
Following Was et al. (2011), an Alphabet WM task was administered. Here the participant was presented with either one letter or two alphabetically nonadjacent letters for 2,500 ms, followed by a transformation phase according to direction and number cues (−3, −2, −1, +1, +2, +3) which remained on the screen until the participant decided to proceed with the task. At the transformation phase, the task was to mentally move either up or down the alphabet according to the cues (e.g., JO + 3 = MR). This was followed by an empty column where the participant was to type the transformed letter/letters. The task included altogether 18 trials, nine trials with a single to-be-remembered letter, and nine trials with two letters to recall. The forward and backward recoding directions (− or +) and recoding distances (1, 2, or 3) varied systematically in both trial lengths. The order of the trials was randomized for each participant. The proportion of correctly recalled trials per minute was used as the dependent variable.
Minus 2 Span Task
In the Minus 2 span task (Waters and Caplan, 2003), sequences with digits occurred successively on-screen, and the participant was to subtract 2 from each digit. For example, a correct response for the sequence 4–8–3–5–6 would be 2–6–1–3–4. Each digit appeared on-screen for 1,000 ms, followed by a fixation point that was visible for 500 ms. At the end of each trial, a recall grid with horizontally aligned boxes was displayed. The boxes contained the numbers from one to nine, and prompted the participant to respond. The task included 12 trials with two trials of each sequence length. The sequence lengths ranged from four to nine digits, and the trials appeared in a randomized order. One point was given for each correctly recalled digit that was placed in the correct serial position.
Verbal Episodic Memory and Word Fluency
Two sets of test items were created for the verbal episodic memory tasks (i.e., the Sentence- and Paragraph recall tasks) and the Word fluency task, and the participants were counterbalanced across sets. Half of the participants (in both the WM training group and the active control group) received one set of items during the pretest and the other set during the posttest, while the order was reversed for the other half of the participants.
Sentence Recall
In this task, words of a sentence were presented successively on a computer screen at a rate of one word per 1,000 ms. Immediately after all the words in a sentence had been shown, the participant was asked to reproduce the sentence by typing it in an empty column.1 The sentences were 18–22 words long and their contents tapped diverse topics in science, nature, and history. The task was designed in both Finnish and English to test whether possible transfer effects would be language-specific. In both languages, we administered altogether five sentences in a randomized order. The proportion of correctly recalled words, regardless of the order they were recalled in, was used as the outcome variable. Moreover, a word was scored as correct if the participant was able to recall the word stem correctly. Suffix-triggered stem alterations in Finnish were ignored, as well as pure orthographical errors.
Paragraph Memory
In this task, the participant was instructed to read a paragraph and memorize its key points rather than trying to remember every word in the text. Nevertheless, when recalling the story, the participant was instructed to use the original words. The paragraphs were shown on a computer screen with no time limit for reading. When the participant decided to proceed, an empty box appeared on the screen, and the participant was to write down as much of the paragraph as possible. The length of the paragraphs ranged between 57 and 59 words. Two trials were completed in a randomized order. We scored this task in two ways, in verbatim and in terms of the semantic contents. The first dependent variable was thus the proportion of correctly recalled words, corresponding to the measure used in the Sentence recall tasks. The second dependent variable was the proportion of correctly recalled semantic contents that had been determined beforehand when designing the paragraphs.
Word Fluency
This task was a computerized Finnish version of the Word fluency task (Benton and Hamsher, 1978). In this task, a letter was shown on a computer screen and the participant was asked to type in as many words as possible beginning with that letter. The letter was visible on the upper part of the screen while an empty column was displayed on the lower section. The participant was to type in the first relevant word that came to mind and then press the “Enter” button. After that the column went blank again, and the participant could immediately type the next word. This procedure was repeated until 60 s had passed. The participant completed three trials, with a different letters for each trial, and the presentation order of the trials was randomized. A total score was calculated as the sum of unique correctly produced words in the three trials.
Expectation Survey
Following Payne and Stine-Morrow (2017), a survey was administered at the end of the posttest to assess the participants’ subjective evaluations of training benefits on specific tasks and on cognitive functions in general. The training benefits were assessed by asking participants whether they believed that their performance improved on a specific task as a function of training (e.g., “You completed a task called SUS. Do you believe that your training period led to better performance in this task?”). The responses were evaluated on a 5-point Likert scale (1 = no, 2 = maybe not, 3 = difficult to say, 4 = maybe yes, 5 = yes). The questions regarding expectations of improved cognitive functions following the training period were also assessed on a 5-point Likert scale (1 = strongly disagree, 2 = disagree, 3 = neither agree nor disagree, 4 = agree, 5 = strongly agree), by asking participants to rate separately for each cognitive function how strongly they agreed/disagreed with the statements (e.g., “I believe that the training period improved my memory”).
Statistical Analyses
All data analyses were conducted using IBM SPSS Statistics 23 software (Armonk, 2015). The background characteristics of the two groups at pretest were compared with independent-samples t-tests for continuous variables and χ2 tests for categorical variables. Progression on the two training tasks in the WM training group was evaluated with one-way repeated measures ANOVAs. Moreover, a 3 × 2 mixed-model ANOVA with time (start, halfway, end) and group (WM training group, active control group) was conducted to evaluate possible group differences in motivation.
Comparisons between the two groups on the cognitive variables were conducted with analyses of covariance (ANCOVAs) using posttest performance as the dependent variable and pretest performance as the covariate. Furthermore, Cohen’s d values were calculated from posttest scores adjusted for pretest scores in the ANCOVAs. We also conducted independent-samples t-tests to examine possible group differences in the subjective evaluations of posttest performance and general training benefits.
Before running the ANCOVA, the data were screened for multivariate outliers at pretest. This was done with the Cook’s d (Cook and Weisberg, 1982) and the Mahalanobis distance value χ2 (10, 80) = 29.59, p = 0.001 (Tabachnick and Fidell, 2007). No participant showed a value greater than 1 on the Cook’s d measure. One participant was close to the cutoff score determined by the Mahalanobis distance (25.92, p < 0.004).
Those participants scoring three times the interquartile range above or below the 1st or the 3rd quartile were excluded from the specific analyses. The rates of univariate outliers as well as those participants that scored below our binomial cutoff score in the RSpan task and/or the OSpan task are reported separately for each measure in Section “Results.”
Results
Progress in the Verbal WM Training Tasks during Training
Selective Updating of Sentences Training Task
In the SUST, a one-way repeated measures ANOVA on SUST progression (attained level) revealed a main effect of session, F(15, 450) = 41.14, p < 0.001, , indicating an increased SUST performance during the training period. The learning curve illustrated in Figure 2 showed a steady rate of improvement for the 12 initial sessions in the WM training group, after which the curve stabilized for the last four sessions.
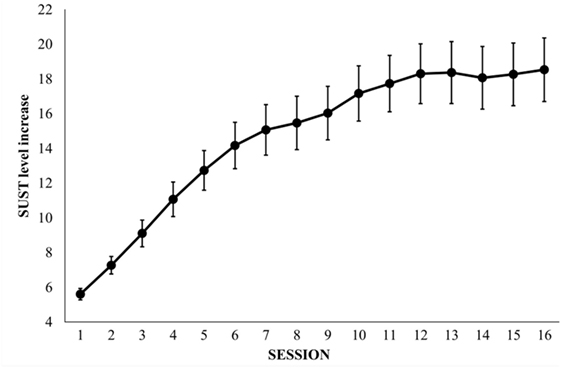
Figure 2. Level increases over 16 training sessions for the SUS training task in the training group (n = 31). SUS, selective updating of sentences.
Sentence Reading Span Training Task
As regards the SRST, the one-way repeated measures ANOVA on progression (attained span level) revealed a main effect of session, F(15, 450) = 3.55, p < 0.001, , indicating that the training increased span performance. The learning curve, illustrated in Figure 3, showed a steady rate of improvement for the six initial training sessions. In the middle of the training period, the performance seemed to fluctuate considerably, while sessions 13–16 indicated somewhat more stable performance at a ca 20% higher span compared with the first training session.
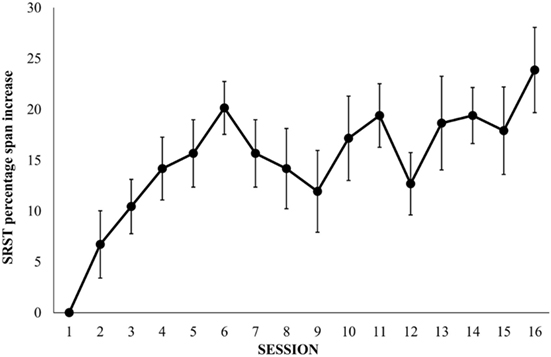
Figure 3. Percentage increases over 16 training sessions for the Reading span training task in the training group (n = 31). Results are expressed as a percentage increase relative to performance on the first day of training.
The Pre- and Posttests
The pre–post mean values per group are depicted in Table 2 and Figure 4.
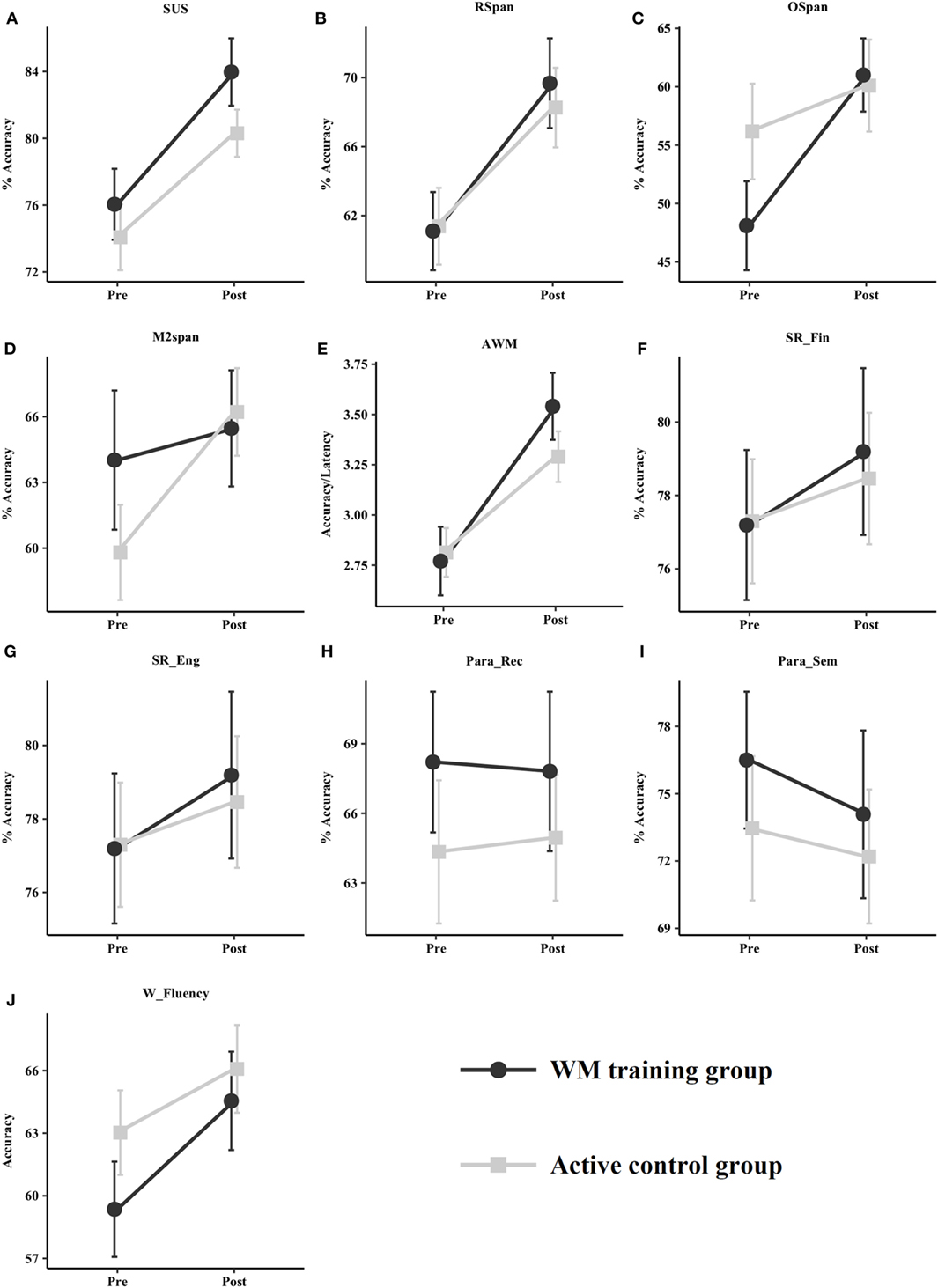
Figure 4. Pre–post means per group for the selective updating of sentences (SUS) task (A); Reading span (RSpan) task (B); Operation span (OSpan) task (C); Minus 2 span (M2span) task (D); Alphabet WM (AWM) task (E); Sentence recall (SR_Fin) in Finnish (F); Sentence recall (SR_Eng) in English (G); Paragraph recall (Para_Rec) (H); Paragraph semantic recall (Para_Sem) (I); and Word Fluency (W_Fluency) (J).
The SUS Criterion Task
No data were excluded from the SUS task analysis. The ANCOVA showed no statistically significant main effect of group, F(1, 65) = 2.05, p = 0.157, d = 0.35, 95% CI [−0.13, 0.83]. However, when leaving out the one participant who was close to the cutoff score determined by the Mahalanobis distance (25.92, p < 0.004), the main effect of group became statistically significant, F(1, 64) = 5.86, p = 0.018, d = 0.60, 95% CI [0.10, 1.09] with the training group being more accurate after training.
The RSpan Criterion Task
All participants were included in the analysis. The ANCOVA on the RSpan task performance was non-significant, F < 1.
OSpan Task
Seven participants (two participants in the training group and five in the control group) scored below the cutoff score in the intervening task and were excluded. No extreme outliers in pretest accuracy were observed. The ANCOVA on OSpan task performance was non-significant, F(1, 58) = 1.70, p = 0.197, d = 0.34, 95% CI [−0.17, 0.84].
Minus 2 Span Task
No data were excluded. The main effect of group was not statistically significant, F(1, 65) = 2.28, p = 0.136, d = −0.37, 95% CI [−0.85, 0.11].
Alphabet WM Task
On this task, no participants were excluded for being extreme outliers on pretest accuracy. The main effect of group failed to reach significance, F(1, 65) = 3.54, p = 0.064, d = 0.46, 95% CI [−0.03, 0.94].
Sentence Recall in Finnish and English
No data were excluded from these two tasks. The ANCOVAs showed no effect of group at posttest, Fs < 1.
Paragraph Recall
No extreme outliers were identified. Both for recall in verbatim and semantic recall, no group difference was observed, Fs < 1.
Word Fluency
No extreme outliers were found. There was no group difference after training, F < 1.
Training Motivation and Self-Assessed Training Benefits
A 3 × 2 mixed-model ANOVA on training motivation, with time (start, halfway, end) as the within-subjects factor and group (training group, control group) as the between-subjects factor was conducted. ANOVA revealed no significant main effect of group, F(1,66) = 0.37, p = 0.54, . However, there was a significant main effect of time, F(2,132) = 22.40, p < 0.001, , indicating that the motivation tended to decrease during the training period. No group × time interaction, F(2,132) = 0.77, p = 0.464, d = −0.15, 95% CI [−0.63, 0.33] was observed.
At the end of the posttest session, we also analyzed the participants’ subjective evaluations of training benefits on specific tasks (see Figure 5) and on cognitive functions in general (see Figure 6). For individual tasks, independent samples t-tests showed that the WM training group gave significantly higher evaluations of performance improvement on the SUS task, t(66) = −7.15, p < 0.001, the RSpan task, t(66) = −5.18 = p < 0.001, the OSpan task, t(66) = −2.88, p = 0.005, and the Paragraph recall task, t(66) = −2.19, p = 0.032. The group differences were non-significant in the Minus 2 span task, t(66) = −0.47, p = 0.637, the Alphabet working memory task, t(66) = −1.53, p = 0.131, the Sentence recall, t(66) = −1.74, p = 0.086 the Sentence recall in English, t(66) = −1.75, p = 0.085, and the Word fluency task, t(66) = 0.67, p = 0.508.
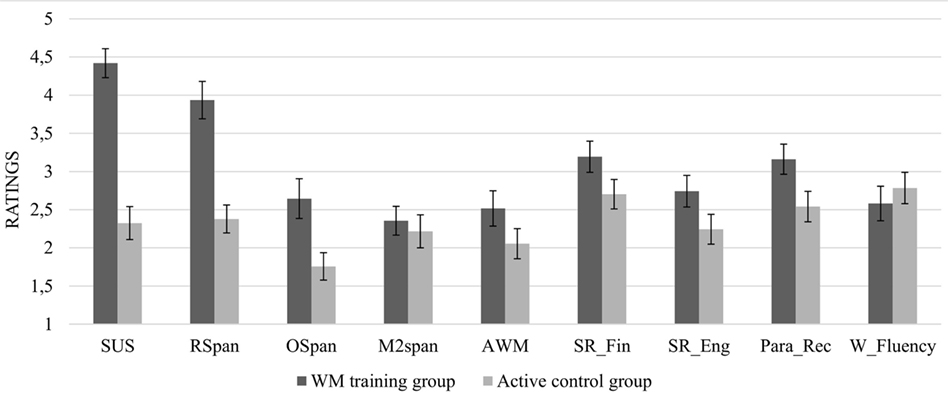
Figure 5. Perceived improvements on specific tasks at posttest in the two groups. Error bars represent SEMs. AWM, Alphabet working memory task; M2Span, Minus 2 span; OSpan, Operation span; Para_Rec, Paragraph recall; RSpan, Reading span; SR_Eng, Sentence recall in English; SR_Fin, Sentence recall in Finnish; SUS, selective updating of sentences; W_fluency, Word fluency.
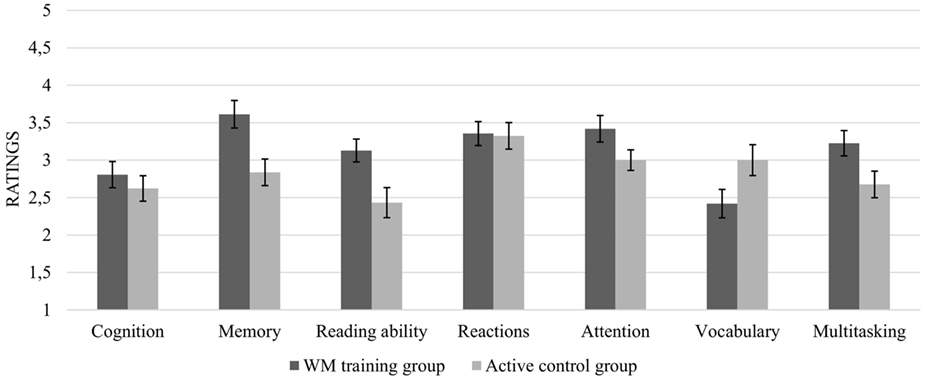
Figure 6. Perceived general cognitive improvements at posttest in the WM training group and the active control group. Error bars represent SEMs. WM, working memory.
As regards perceived improvement in general cognitive functions, independent samples t-test revealed that the WM training group had significantly higher evaluations of their improvement in memory, t(66) = −3.00, p = 0.004, reading ability, t(66) = −0.2.81, p = 0.007, and multitasking, t(66) = −2.23, p = 0.042. The groups did not differ in their evaluations of improvement in overall cognition, t(66) = −0.76, p = 0.456, reaction time, t(66) = −0.13, p = 0.898, or attention, t(66) = −1.81, p = 0.074. Finally, the active control group that had trained with the quiz gave a significantly higher evaluation of their vocabulary advancement, t(66) = 2.07, p = 0.042.
Discussion
This study examined the effects of WM training with a novel training regime that used sentences as training stimuli. The employment of sentences was motivated by the recent critique toward the current training regimes that employ random strings of stimuli (Klingberg, 2010; Shipstead et al., 2010; Holmes, 2011; Holmes and Gathercole, 2014; Moreau and Conway, 2014). The present training regime was also motivated by a recent study by Payne and Stine-Morrow (2017) who reported transfer effects in older adults after training with a partly similar training regime.
The present results showed no statistically significant transfer effects to any of the near or far-transfer tasks. The only statistically significant group difference at posttest emerged on the SUS task, but only when excluding one participant who was close to being a multivariate outlier. The results were thus clearly more meager than one would expect on the basis of recent meta-analytical evidence on WM training that shows improvement on the criterion tasks and closely related untrained tasks (Melby-Lervåg et al., 2016; Soveri et al., 2017). While the lack of significant training effects on the two criterion tasks was unexpected, there are several previous WM training studies that have also failed to show significant training effects in criterion tasks (e.g., Gray et al., 2012; Bürki et al., 2014; Minear et al., 2016; Payne and Stine-Morrow, 2017). It is also worth noting that, akin to the current results, Payne and Stine-Morrow (2017) did not observe any significant training effects on his sentence-level WM criterion task.
The absence of significant training group improvements on the criterion tasks cannot be explained by failed adaptive training results (see Figures 2 and 3). Especially the SUST task showed a steady performance increase on most of the training sessions. The learning curve for the SRST training task was less steep and more variable across the training sessions, and this could have contributed to the lack of posttest group difference on its respective criterion task. The present variability in the SRST during training deviates from the training results in Payne and Stine-Morrow (2017) where the participants showed a less-fluctuating improvement curve. Additionally, their participants performed at a ca 60% higher span level during the last training session compared with the first training session. In contrast, our participants did not show more than a ca 20% higher span level for the entire training period.
One possible explanation for the weak or lacking training effects on the criterion tasks might be that our training regimes and criterion tasks were not identical. Compared with our training tasks, both the SUS task and the RSpan task were non-adaptive with predetermined sequence lengths. Moreover, we used different stimulus words in training and in the pre–post tasks to eliminate lexical learning effects. This stands in contrast to WM training studies where the criterion tasks employed the same stimuli (usually digits or letters) that had been used during training (e.g., Lilienthal et al., 2013; Waris et al., 2015). This implies that our criterion tasks could actually be categorized as task-specific near-transfer tasks rather than criterion tasks (implying weaker transfer), as they are structurally similar to the training regimes, but differ in terms of stimuli (Soveri et al., 2017). As regards the study by Payne and Stine-Morrow (2017), it is not clear whether the sentence-level training regime comprised the same words that were administered in the criterion task. Nevertheless, Payne and Stine-Morrow (2017) did not find any significant improvement in their RSpan criterion task either, suggesting that it is difficult to obtain training effects in non-practiced novel sentences even though the task structure would be similar to the one used in training.
As regards the effect sizes from pre-post tasks, Figure 7 presents them in a forest plot (Cohen’s d) derived from group comparisons. When looking at the effect sizes on the present task-general near-transfer measures, only the Alphabet working memory task showed a close-to-moderate effect size (d = 0.46; Cohen, 1988) favoring the WM training group. Moreover, the OSpan task showed a small effect size favoring the WM training group (d = 0.36), while the Minus 2 span task showed a totally opposite small effect favoring the active control group (d = −0.37). This mixed pattern makes it difficult to draw any conclusions based on the effect sizes.
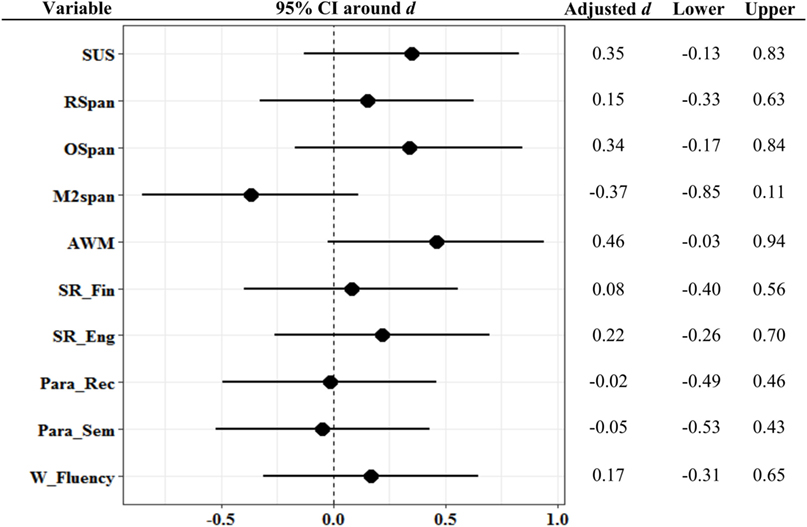
Figure 7. Forest plot of the training outcome with 95% confidence intervals represented by horizontal lines, from the group comparisons of training effects in the present study. Higher effect sizes indicate better performance in the WM training group. Cohen’s d is computed from estimated posttest measurement scores adjusted for pre-measurements in the ANCOVA. ANCOVA, analysis of covariance; AWM, Alphabet working memory task; M2Span, Minus 2 span; OSpan, Operation span; Para_Rec, Paragraph recall; Para_Sem, Paragraph semantic recall; RSpan, Reading span; SR_Eng, Sentence recall in English; SR_Fin, Sentence recall in Finnish; SUS, selective updating of sentences; W_fluency, Word fluency; WM, working memory.
We also measured training motivation and perceived training gains at posttest. Both groups reported being equally motivated in the beginning, half-way, and at the end of the training period. Thus, the motivational factors can be dismissed as a confounding variable regarding the current lack of significant training effects. As regards the perceived training gains, the training group reported widespread subjective improvements in both specific pre/post tasks and general cognitive functions, but these perceptions were not substantiated by their actual test results.
Compared with the results reported by Payne and Stine-Morrow (2017), the present results are clearly weaker in terms of transfer. In addition to near-transfer effects, Payne and Stine-Morrow (2017) observed far transfer to several offline language measures, namely, sentence recall, verbal fluency, and comprehension of syntactically ambiguous sentences. There are several possible reasons for the different outcomes in these two studies. In the study by Payne and Stine-Morrow (2017), the participants were healthy elderly, and it has been speculated that they might have more room for improvement than younger adults who are at or near the top of their cognitive potential (e.g., Borella et al., 2017). If this claim holds, it could also explain aphasic patients’ improvements in both trained and untrained language tasks following sentence-level WM training (Francis et al., 2003; Koenig-Bruhin and Studer-Eichenberger, 2007; Eom and Sung, 2016). However, as the most recent meta-analyses on WM training have failed to find moderating effects of age in adults (Melby-Lervåg and Hulme, 2013; Schwaighofer et al., 2015; Melby-Lervåg et al., 2016; Soveri et al., 2017) on training outcomes, it is not likely that the higher age of Payne and Stine-Morrow’s (Payne and Stine-Morrow, 2017) participants explains the better transfer they reported. This conclusion is of course made under the assumption that the moderating effects of age do not vary between WM domains.
Another possible reason for the present discrepancy may be related to the somewhat different training regimes. In Payne and Stine-Morrow (2017), apart from the sentence-level WM task (a RSpan training task), participants were also training on two other complex span tasks that required semantic categorization and lexical decision-making. Thus, it is possible that the observed transfer effects stemmed from the other training tasks rather than the RSpan training task. Also other differences such as choice of statistical approach, lack of control for possible motivational differences between study groups in Payne and Stine-Morrow (2017), and partly different pre- and posttest tasks makes it difficult to directly compare these two studies.
Returning to the models on WM and sentence processing, it has been debated whether linguistic WM tasks that require syntactic processing engage the same pool of verbal WM resources as verbal tasks that lack syntactic structure (King and Just, 1991; Just and Carpenter, 1992; Caplan and Waters, 1999). There is still no consensus concerning the mental architecture of the verbal WM domain, but the weak training effects in the present study may reflect the specialized and automatic nature of WM systems related to sentence processing. However, in contrast to our findings, Payne and Stine-Morrow (2017) found that, compared with the control group, the WM training group improved more on comprehension accuracy for garden-path sentences. Nevertheless, the accuracy rates of their groups did not differ at posttest on the other sentence types, namely, in long-distance dependency comprehension and object-relative processing. Based on our own results, as well as Payne and Stine-Morrow’s (Payne and Stine-Morrow, 2017) partly inconsistent findings, we suggest that WM tasks that include sentence processing may engage somewhat more crystallized abilities. People read texts (e.g., via newspaper, books, Internet) on a daily basis, and the skills involved in reading are for most literates highly overlearned as a result of years of practice (Fischler and Bloom, 1980). Hence, practice with ecologically valid tasks that tap ecologically valid tasks tapping processes that have become highly automatized long before the onset of the intervention may exhibit less training effects than training regimes using more artificial stimulus sequences (e.g., digits or letters) that rarely occur in daily life.
Limitations and Future Directions
In this study, we investigated the transfer effects of sentence-level WM training by comparing the training group performance to an active control group that practiced with a language-heavy quiz task. Ideally, the inclusion of a no-contact group would have been advantageous, thus allowing a separation of practice and expectancy effects. A passive control group would also have provided information regarding whether or not our quiz training elicited task improvement relative to no training at all. Current evidence, however, indicates that there is no difference in pre–post change between active and passive controls (Soveri et al., 2017). Another potential limitation in this study was that the training period was performed online in non-laboratory settings. Despite the advantages of online training, some concerns have been raised about the lack of control over the testing environment (for a review, see Ford, 2017). Studies have, for instance, shown that issues such as unreliable effort or careless responding exist during online testing (Feitosa et al., 2015; Smith et al., 2016). Thus, despite instructions we do not know if all participants performed the training sessions in the way they were expected to. Albeit there is some evidence that online cognitive task performance, in general, compares well to traditional laboratory studies (Linnman et al., 2006; Germine et al., 2012; Crump et al., 2013; Enochson and Culbertson, 2015), no study has to our knowledge compared a whole period of cognitive training at home against training in laboratory settings. This is an aspect that should be mapped more closely in future studies.
In this study, we implemented sentence-level WM training as this can be argued to offer a somewhat more ecological approach compared with previous WM training regimes. It is worth noting that also some previous studies have attempted to devise ecologically oriented cognitive training, such as verbal WM training in noise (Ingvalson et al., 2015; Wayne et al., 2016), or a combination of cognitive training with complex physical activity (Moreau et al., 2015). As WM training studies typically yield very limited transfer effects (Melby-Lervåg et al., 2016; Soveri et al., 2017), one approach for future studies would be to utilize tasks on which improved performance is directly relevant to the individual.
Ethics Statement
This study was carried out in accordance with the recommendations of the Declaration of Helsinki (Protocol no. 39) with written informed consent from all subjects. The protocol was approved by the Institutional Review Board of the Departments of Psychology and Logopedics, Akademi University (Protocol no. 197).
Author Contributions
ML developed the study concept and all authors contributed to the study design. Testing and data collection were conducted by DF who also performed the data analysis and interpretation together with AS. DF drafted the manuscript. All coauthors provided critical revisions and approved the final version of the manuscript for submission.
Conflict of Interest Statement
The authors declare that the research was conducted in the absence of any commercial or financial relationships that could be construed as a potential conflict of interest.
Acknowledgments
We wish to thank the rest of the BrainTrain group for their help with the study.
Funding
This work was supported by the Academy of Finland (grant number 260276 to ML) and the Abo Akademi University Endowment (the BrainTrain project).
Footnote
- ^A self-paced variant of the sentence recall task was also included in the pre- and posttest battery. This task version, however, resulted in great variance between participants in the reading time of the to-be-remembered sentences. This was considered as problematic in terms of task validity and therefore the self-paced version of the task was excluded from pre/posttest analyses.
References
Alloway, T. P., and Alloway, R. G. (2010). Investigating the predictive roles of working memory and IQ in academic attainment. J. Exp. Child Psychol. 106, 20–29. doi: 10.1016/j.jecp.2009.11.003
Baddeley, A. (2000). The episodic buffer: a new component of working memory? Trends Cogn. Sci. 4, 417–423. doi:10.1016/S1364-6613(00)01538-2
Beck, A., Steer, R., and Brown, G. (2004). BDI-II Käsikirja [Manual of the BDI-II Finnish Version]. Helsinki: Psykologien Kustannus.
Benton, A., and Hamsher, K. (1978). Multilingual Aphasia Examination, Rev. Edn. Iowa City: Department of Neurology, University of Iowa Hospitals.
Borella, E., Carbone, E., Pastore, M., De Beni, R., and Carretti, B. (2017). Working memory training for healthy older adults: the role of individual characteristics in explaining short-and long-term gains. Front. Hum. Neurosci. 11:99. doi:10.3389/fnhum.2017.00099
Bürki, C. N., Ludwig, C., Chicherio, C., and de Ribaupierre, A. (2014). Individual differences in cognitive plasticity: an investigation of training curves in younger and older adults. Psychol. Res. 78, 821–835. doi:10.1007/s00426-014-0559-3
Caplan, D., and Waters, G. S. (1999). Verbal working memory and sentence comprehension. Behav. Brain Sci. 22, 77–94. doi:10.1017/S0140525X99001788
Chein, J. M., and Morrison, A. B. (2010). Expanding the mind’s workspace: training and transfer effects with a complex working memory span task. Psychon. Bull. Rev. 17, 193–199. doi:10.3758/PBR.17.2.193
Cohen, J. (1988). Statistical Power Analysis for the Behavioral Sciences. 2nd ed. Hillsdale, NJ: Lawrence Erlbaum Associates.
Conway, A. R., Kane, M. J., Bunting, M. F., Hambrick, D. Z., Wilhelm, O., Engle, R. W. (2005). Working memory span tasks: A methodological review and user’s guide. Psychonom. Bull. Rev. 12, 769–786. doi:10.3758/BF03196772
Conway, A. R., Kane, M. J., and Engle, R. W. (2003). Working memory capacity and its relation to general intelligence. Trends Cogn. Sci. 7, 547–552. doi:10.1016/j.tics.2003.10.005
Cook, R. D., and Weisberg, S. (1982). Residuals and Influence in Regression. New York: Chapman and Hall.
Crump, M. J. C., McDonnell, J. V., and Gureckis, T. M. (2013). Evaluating Amazon’s Mechanical Turk as a tool for experimental behavioral research. PLoS ONE 8:e57410. doi:10.1371/journal.pone.0057410
Daneman, M., and Carpenter, P. A. (1980). Individual differences in working memory and reading. J. Verbal Learn. Verbal Behav. 19, 450–466. doi:10.1016/S0022-5371(80)90312-6
Daneman, M., and Merikle, P. M. (1996). Working memory and language comprehension: a meta-analysis. Psychon. Bull. Rev. 3, 422–433. doi:10.3758/BF03214546
Enochson, K., and Culbertson, J. (2015). Collecting psycholinguistic response time data using Amazon Mechanical Turk. PLoS ONE 10:e0116946. doi:10.1371/journal.pone.0116946
Eom, B., and Sung, J. E. (2016). The effects of sentence repetition-based working memory treatment on sentence comprehension abilities in individuals with aphasia. Am. J. Speech Lang. Pathol. 25, S823–S838. doi:10.1044/2016_AJSLP-15-0151
Fedorenko, E., Gibson, E., and Rohde, D. (2006). The nature of working memory capacity in sentence comprehension: evidence against domain-specific working memory resources. J. Mem. Lang. 54, 541–553. doi:10.1016/j.jml.2005.12.006
Feitosa, J., Joseph, D. L., and Newman, D. A. (2015). Crowdsourcing and personality measurement equivalence: a warning about countries whose primary language is not English. Pers. Individ. Dif. 75, 47–52. doi:10.1016/j.paid.2014.11.017
Fellman, D., Soveri, A., Viktorsson, C., Haga, S., Nylund, J., Johansson, S., et al. (2017). Selective updating of sentences: introducing a new measure of verbal working memory. Appl. Psycholinguist. 1–27. doi:10.1017/S0142716417000182
Fischler, I., and Bloom, P. A. (1980). Rapid processing of the meaning of sentences. Mem. Cognit. 8, 216–225. doi:10.3758/BF03197609
Ford, J. B. (2017). Amazon’s mechanical Turk: a comment. J. Adv. 46, 156–158. doi:10.1080/00913367.2016.1277380
Francis, D., Clark, N., and Humphreys, G. (2003). The treatment of an auditory working memory deficit and the implications for sentence comprehension abilities in mild “receptive” aphasia. Aphasiology 17, 723–750. doi:10.1080/02687030344000201
Garrett, M. F. (1990). “Sentence processing,” in An Invitation to Cognitive Science (Vol. 1): Language, eds D. N. Osherson and H. Lasnik (Cambridge, MA: MIT Press), 133–175.
Gathercole, S. E., Pickering, S. J., Knight, C., and Stegmann, Z. (2004). Working memory skills and educational attainment: evidence from national curriculum assessments at 7 and 14 years of age. Appl. Cogn. Psychol. 18, 1–16. doi:10.1002/acp.934
Germine, L., Nakayama, K., Duchaine, B. C., Chabris, C. F., Chatterjee, G., and Wilmer, J. B. (2012). Is the web as good as the lab? Comparable performance from web and lab in cognitive/perceptual experiments. Psychon. Bull. Rev. 19, 847–857. doi:10.3758/s13423-012-0296-9
Gordon, P. C., Hendrick, R., and Levine, W. H. (2002). Memory-load interference in syntactic processing. Psychol. Sci. 13, 425–430. doi:10.1111/1467-9280.00475
Gray, S., Chaban, P., Martinussen, R., Goldberg, R., Gotlieb, H., Kronitz, R., et al. (2012). Effects of a computerized working memory training program on working memory, attention, and academics in adolescents with severe LD and comorbid ADHD: a randomized controlled trial. J. Child Psychol. Psychiatry 53, 1277–1284. doi:10.1111/j.1469-7610.2012.02592.x
Hambrick, D. Z., Oswald, F. L., Darowski, E. S., Rench, T. A., and Brou, R. (2010). Predictors of multitasking performance in a synthetic work paradigm. Appl. Cogn. Psychol. 24, 1149–1167. doi:10.1002/acp.1624
Holmes, J. (2011). Baby brain: training executive control in infancy. Curr. Biol. 21, R684–R685. doi:10.1016/j.cub.2011.08.026
Holmes, J., and Gathercole, S. E. (2014). Taking working memory training from the laboratory into schools. Educ. Psychol. 34, 440–450. doi:10.1080/01443410.2013.797338
Ingvalson, E. M., Dhar, S., Wong, P. C., and Liu, H. (2015). Working memory training to improve speech perception in noise across languages. J. Acoust. Soc. Am. 137, 3477–3486. doi:10.1121/1.4921601
Jaeggi, S. M., Buschkuehl, M., Jonides, J., and Perrig, W. J. (2008). Improving fluid intelligence with training on working memory. Proc. Natl. Acad. Sci. U.S.A. 105, 6829–6833. doi:10.1073/pnas.0801268105
Just, M. A., and Carpenter, P. A. (1992). A capacity theory of comprehension: individual differences in working memory. Psychol. Rev. 99, 122–149. doi:10.1037/0033-295X.99.1.122
Kamide, Y., Altmann, G. T., and Haywood, S. L. (2003). The time-course of prediction in incremental sentence processing: evidence from anticipatory eye movements. J. Mem. Lang. 49, 133–156. doi:10.1016/S0749-596X(03)00023-8
King, J., and Just, M. A. (1991). Individual differences in syntactic processing: the role of working memory. J. Mem. Lang. 30, 580–602. doi:10.1016/0749-596X(91)90027-H
Klingberg, T. (2010). Training and plasticity of working memory. Trends Cogn. Sci. 14, 317–324. doi:10.1016/j.tics.2010.05.002
Koenig-Bruhin, M., and Studer-Eichenberger, F. (2007). Therapy of short-term memory disorders in fluent aphasia: a single case study. Aphasiology 21, 448–458. doi:10.1080/02687030600670593
Konig, C. J., Buhner, M., and Murling, G. (2005). Working memory, fluid intelligence, and attention are predictors of multitasking performance, but polychronicity and extraversion are not. Hum. Perform. 18, 243–266. doi:10.1207/s15327043hup1803_3
Lee, C., and Federmeier, K. D. (2012). Ambiguity’s aftermath: how age differences in resolving lexical ambiguity affect subsequent comprehension. Neuropsychologia 50, 869–879. doi:10.1016/j.neuropsychologia.2012.01.027
Lilienthal, L., Tamez, E., Shelton, J. T., Myerson, J., and Hale, S. (2013). Dual n-back training increases the capacity of the focus of attention. Psychon. Bull. Rev. 20, 135–141. doi:10.3758/s13423-012-0335-6
Linnman, C., Carlbring, P., Åhman, Å, Andersson, H., and Andersson, G. (2006). The Stroop effect on the Internet. Comput. Human Behav. 22, 448–455. doi:10.1016/j.chb.2004.09.010
Melby-Lervåg, M., and Hulme, C. (2013). Is working memory training effective? A meta-analytic review. Dev. Psychol. 49, 270–291. doi:10.1037/a0028228
Melby-Lervåg, M., Redick, T. S., and Hulme, C. (2016). Working memory training does not improve performance on measures of intelligence or other measures of “far transfer”: evidence from a meta-analytic review. Perspect. Psychol. Sci. 11, 512–534. doi:10.1177/1745691616635612
Minear, M., Brasher, F., Guerrero, C. B., Brasher, M., Moore, A., and Sukeena, J. (2016). A simultaneous examination of two forms of working memory training: evidence for near transfer only. Mem. Cognit. 44, 1014–1037. doi:10.3758/s13421-016-0616-9
Moreau, D., and Conway, A. R. A. (2014). The case for an ecological approach to cognitive training. Trends Cogn. Sci. 18, 334–336. doi:10.1016/j.tics.2014.03.009
Moreau, D., Morrison, A. B., and Conway, A. R. (2015). An ecological approach to cognitive enhancement: complex motor training. Acta Psychol. 157, 44–55. doi:10.1016/j.actpsy.2015.02.007
Payne, B. (2014). The Effects of Verbal Working Memory Training on Language Comprehension in Older Adulthood. Dissertation, University of Illinois at Urbana-Champaign, Illinois, IL.
Payne, B. R., and Stine-Morrow, E. A. (2017). The effects of home-based cognitive training on verbal working memory and language comprehension in older adulthood. Front. Aging Neurosci. 9:256. doi:10.3389/fnagi.2017.00256
Poole, B. J., and Kane, M. J. (2009). Working-memory capacity predicts the executive control of visual search among distractors: the influences of sustained and selective attention. Q. J. Exp. Psychol. 62, 1430–1454. doi:10.1080/17470210802479329
Prat, C. S., Keller, T. A., and Just, M. A. (2007). Individual differences in sentence comprehension: a functional magnetic resonance imaging investigation of syntactic and lexical processing demands. J. Cogn. Neurosci. 19, 1950–1963. doi:10.1162/jocn.2007.19.12.1950
Ricketts, J., Sperring, R., and Nation, K. (2014). Educational attainment in poor comprehenders. Front. Psychol. 5:445. doi:10.3389/fpsyg.2014.00445
Ritchie, S. J., and Bates, T. C. (2013). Enduring links from childhood mathematics and reading achievement to adult socioeconomic status. Psychol. Sci. 24, 1301–1308. doi:10.1177/0956797612466268
Schwaighofer, M., Fischer, F., and Bühner, M. (2015). Does working memory training transfer? A meta-analysis including training conditions as moderators. Educ. Psychol. 50, 138–166. doi:10.1080/00461520.2015.1036274
Shipstead, Z., Redick, T., and Engle, R. (2010). Does working memory training generalize? Psychol. Belg. 50, 245–276. doi:10.5334/pb-50-3-4-245
Smith, S. M., Roster, C. A., Golden, L. L., and Albaum, G. S. (2016). A multi-group analysis of online survey respondent data quality: comparing a regular USA consumer panel to MTurk samples. J. Bus. Res. 69, 3139–3148. doi:10.1016/j.jbusres.2015.12.002
Soveri, A., Antfolk, J., Karlsson, L. C., Salo, B., and Laine, M. (2017). Working memory training revisited: a multi-level meta-analysis of n-back training studies. Psychonom. Bull. Rev. 24, 1077–1096. doi:10.3758/s13423-016-1217-0
Stites, M. C., Federmeier, K. D., and Stine-Morrow, E. A. (2013). Cross-age comparisons reveal multiple strategies for lexical ambiguity resolution during natural reading. J. Exp. Psychol. Learn. Mem. Cogn. 39, 1823–1841. doi:10.1037/a0032860
Süß, H., Oberauer, K., Wittmann, W. W., Wilhelm, O., and Schulze, R. (2002). Working-memory capacity explains reasoning ability – and a little bit more. Intelligence 30, 261–288. doi:10.1016/S0160-2896(01)00100-3
Tabachnick, B. G., and Fidell, L. S. (2007). Using Multivariate Statistics, 5th ed. Boston: Pearson/Allyn & Bacon.
Turner, M. L., and Engle, R. W. (1989). Is working memory capacity task dependent? J. Mem. Lang. 28, 127–154. doi:10.1016/0749-596X(89)90040-5
Waris, O., Soveri, A., and Laine, M. (2015). Transfer after working memory updating training. PLoS ONE 10:e0138734. doi:10.1371/journal.pone.0138734
Was, C. A., Rawson, K. A., Bailey, H., and Dunlosky, J. (2011). Content-embedded tasks beat complex span for predicting comprehension. Behav. Res. Methods 43, 910–915. doi:10.3758/s13428-011-0112-x
Waters, G. S., and Caplan, D. (2003). The reliability and stability of verbal working memory measures. Behav. Res. Methods Instrum. Comput. 35, 550–564. doi:10.3758/BF03195534
Keywords: working memory training, verbal working memory, sentence processing, near transfer, task-specific near transfer
Citation: Fellman D, Soveri A, Waris O and Laine M (2017) Training of Verbal Working Memory at Sentence Level Fails to Show Transfer. Front. Commun. 2:14. doi: 10.3389/fcomm.2017.00014
Received: 21 June 2017; Accepted: 26 September 2017;
Published: 13 October 2017
Edited by:
Stefano F. Cappa, Istituto Universitario di Studi Superiori di Pavia (IUSS), ItalyReviewed by:
Chiara Crespi, Consiglio Nazionale Delle Ricerche (CNR), ItalyRandi Martin, Rice University, United States
Copyright: © 2017 Fellman, Soveri, Waris and Laine. This is an open-access article distributed under the terms of the Creative Commons Attribution License (CC BY). The use, distribution or reproduction in other forums is permitted, provided the original author(s) or licensor are credited and that the original publication in this journal is cited, in accordance with accepted academic practice. No use, distribution or reproduction is permitted which does not comply with these terms.
*Correspondence: Daniel Fellman, ZGFuaWVsLmZlbGxtYW5AYWJvLmZp