- Metaphor Lab Amsterdam, Department of Argumentation Theory and Rhetoric, University of Amsterdam, Amsterdam, Netherlands
This study aims at modeling the semantic similarity between metaphor terms by means of a distributional method based on a Big Data stream: Flickr tags. As explained in the article, this distributional model, Flickr Distributional Tagspace (FDT), captures primarily relational similarity between concept pairs, that is, between tags that appear in similar tagsets (and therefore in similar pictures). A long established view in metaphor theory claims that metaphors pertain to the conceptual dimension of meaning, but while different models aim at explaining how language constructs and represents metaphorical conceptual structures, we still know very little about how other modalities (for example, images) achieve metaphor construction and expression. A comprehensive theory, which argues in favor of the conceptual nature of metaphor, cannot afford to be biased toward the analysis and modeling of one specific modality of expression, thus neglecting potential modality-specific differences. The present study, conducted through FDT, found that visual and linguistic metaphors behave differently, in that the similarity between two aligned concepts in a visual metaphor appears to be significantly higher than the similarity between two concepts aligned in a linguistic metaphor (which, in turn, does not differ substantially from the similarity between two randomly paired concepts). These findings suggest that the relational similarity between two metaphor terms (captured and modeled through FDT) is crucial for visual metaphors but not for linguistic metaphors. An additional content analysis, also reported here, shows that the type of semantic information encoded in the related tags (i.e., the contexts on which the contingency matrices of this distributional method are built) differs, in relation to the modality of the metaphor: while situation-related and entity-related features are typically associated with concepts aligned in visual metaphors, introspections, and taxonomic features are typically associated with concepts aligned in linguistic metaphors.
Introduction
Metaphor is recognized nowadays as a cognitive mechanism, rather than a simple figure of speech (Lakoff and Johnson, 1980; Lakoff, 1993). Through metaphors, we understand one concept in terms of another concept, the latter being generally perceived as easier or more concrete. On this view, metaphoric expressions, such as our love is at a crossroads, we can’t turn back now, together we are not going anywhere, suggest that when we deal with the abstract concept of LOVE (by convention, capital letters are used when referring to a concept or a conceptual domain), we might somehow envision a concrete journey going along a path. This conceptualization allows us to extend the metaphor, and imagine the two lovers as two travelers, the difficulties in the relationship as impediments on the journey, and the lovers’ goals as the travel destination. From this complex and rather subconscious conceptual structure (LOVE-IS-JOURNEY), we would then derive metaphoric linguistic expressions such as those mentioned above. Thus, metaphors transcend their linguistic manifestations to influence conceptual structures. The cornerstone theory that suggested such a revolutionary approach to metaphors is called Conceptual Metaphor Theory (CMT, Lakoff and Johnson, 1980) and has had a very strong influence in linguistics and cognitive science, generating a large amount of supportive research, as well as critical contributions [e.g., Steen (2008)]. Among them, the career-of-metaphor theory (Bowdle and Gentner, 2005) suggests that metaphors may not always be understood by means of cross-domain mappings between base and target domains, as suggested by CMT. Instead, the way in which metaphor mappings operate, according to Bowdle and Gentner, would switch from comparison to categorization processes as the metaphor becomes conventional. For example, in the expression mentioned above, our love is at a crossroads, the word crossroads is used metaphorically to describe a moment that requires important decisions to be taken. As a (hypothetically) novel metaphoric expression, the crossroads (base term) and the moment that requires decisions to be taken would be processed by aligning and comparing the conceptual structures of these two domains. Instead, as the metaphor becomes conventional and frequently used, crossroads would become an acquired member of the conceptual category instantiated by the target of the metaphor and thus understood in that context by means of semantic disambiguation among different senses held by crossroads.
While different models have been proposed to account for the mechanisms of metaphor processing and comprehension in cognitive linguistics and cognitive science [see, for example, Black (1979), Ortony (1979), Turner and Fauconnier (2002), and Giora (2008)], the computational modeling of such mechanisms appears to be quite an arduous task [see Veale et al. (2016), for a review of metaphor and natural language processing]. One of the main problems that computational models based on natural language processing encounter, when trying to reproduce metaphor comprehension, lies in the nature of the similarity (intended in its wider sense) that allows two concepts to be aligned in a metaphor. Two concepts that are aligned in a metaphorical comparison need to have something in common for them to be comparable, some latent piece of semantic information that allows the comparison to emerge and be meaningful. However, the similarity that characterizes two metaphor terms is not as consistent and stable as the similarity that characterizes, for example, two synonyms. In particular, while the similarity between two synonyms is arguably based on several common features, two concepts aligned in a metaphor might share only one or a few crucial (or even not quite salient) features. The type of semantic information encoded in the shared features, which accounts for the similarity between two metaphor terms, is still unexplored territory. In a recent study, for example, the two different cognitive processes of (verbal) metaphor comprehension (comparison and categorization) were modeled through a semantic space model (Utsumi, 2011). The author used a distributional method based on corpora of language to model the processing of verbal metaphors. However, being based on a stream of linguistic data, this approach accounts exclusively for the semantic information encoded in the shared linguistic patterns between the metaphor terms, thus modeling the similarity that characterizes their language use. In other words, this approach does not allow to explore different possible types of conceptual similarity existing between metaphor terms, which might not be captured by the shared linguistic patterns. On this same line, a previous attempt of modeling linguistic metaphor comprehension by means of distributional semantics was suggested by Kintsch and Bowles (2002). Very recently, a few other works used distributional semantics to model aspects of linguistic metaphor comprehension (Agres et al., 2016; Gutiérrez et al., 2016). Moreover, Shutova et al. (2015) proposed a method for metaphor identification based on distributional semantics, which draws knowledge from both linguistic and visual data.
The aim of this study is to explore and assess how, respectively, images and words afford the construction and representation of metaphor. In this perspective, metaphor similarity is here modeled by means of distributional semantics, to observe the emergence of potentially different patterns of similarity that can be ascribed to the different modalities of metaphoric expression. The present study is embedded in a larger project (COGVIM) that aims at modeling and contrasting the semantic similarity between metaphor terms in visual vs. linguistic metaphors, relying on the distributional hypothesis. Three different types of distributional similarity are investigated: attributional similarity, relational similarity, and linguistic similarity. This goal is achieved through extensive distributional analyses based on corpora that encode different streams of semantic information and that can therefore model the three different types of distributional similarity. The present study reports the analyses aimed at modeling the relational similarity.
A representative sample of visual metaphors and a representative sample of linguistic metaphors are analyzed and compared. It is hereby hypothesized that the two samples are likely to afford different patters of similarity between the involved domains. Such prediction is based on experimental evidence, showing different patterns of neural activation during matched word and picture recognition tasks (Gorno-Tempini et al., 1998; Moore and Price, 1999; Chee et al., 2000; Hasson et al., 2002; Bright et al., 2004; Gates and Yoon, 2005; Reinholz and Pollmann, 2005). Moreover, as indicated in Binder et al.’s (2009) meta-analysis, different studies argue against a complete overlap between the knowledge systems underlying word and object recognition, based on the existence of patients with profound visual object recognition disorders but relatively intact word comprehension (Warrington, 1985; Farah, 1990; Davidoff and De Bleser, 1994). Finally, a well-known and empirically supported account of cognition suggests that linguistic and visual stimuli trigger two functionally independent, but interconnected, multimodal systems, one specialized for non-verbal stimuli, which directly represents the perceptual properties and affordances of non-verbal objects and events, and the other specialized for linguistic stimuli, which deals directly with linguistic stimuli and responses (cf. Dual Coding Theory, Paivio, 1971, 1986, 2010).
To the best of my knowledge, there are no other studies that aim at comparing the structure and functioning of these two modalities of expression in relation to metaphor. A recent body of work on visual and audiovisual representations has shown in a qualitative fashion how some of the conventional (conceptual) metaphors extracted from language use can be expressed in images as well [see, for example, Forceville (2011), Hidalgo and Kraljevic (2011), Ning (2011), and Ortiz (2011)]. The approach used by the authors, however, is top-down: given a set of conventional conceptual metaphors, the scholars searched for possible realizations in the visual modality. The purpose of this study is different, in that it aims at observing how each of the two modalities of expression of metaphor functions, independently from one another, in a bottom-up fashion, in order to see potential differences emerge spontaneously. For this reason, the present study should be considered as the first exploratory analysis of these two modalities of expression.
The research questions tackled by the present investigation can be summarized as follows:
RQ1: Can the latent similarity between metaphor terms be modeled through Flickr Distributional Tagspace (FDT), a distributional method based on tags produced by Flickr users to describe salient concepts related to personal experiences, captured in Flickr photographs?
RQ2: Can FDT model the similarity between metaphor terms in visual and in linguistic metaphors equally well?
RQ3: What type of semantic information is typically encoded by FDT? And what type of semantic information is encoded in the shared features between metaphor terms in visual vs. linguistic metaphors?
Method
The analyses reported in this study are performed through FDT (Bolognesi, 2014, 2016), an innovative distributional method based on annotated images.
Flickr Distributional Tagspace is substantially similar to latent semantic analysis (LSA, Landauer and Dumais, 1997) in that it is not structured (i.e., it does not include information retrieved from syntactic patterns of word co-occurrences). The three main differences between LSA and FDT, as described in Bolognesi (2016), pertain to the type of context used to create the contingency table (text documents in LSA vs. tagsets of annotated images in FDT), the measure of association between an element and each context (term frequency–inverse document frequency in LSA vs. square pointwise mutual information in FDT), and the dimensionality reduction applied before the computation of the cosine (singular value decomposition in LSA vs. none in FDT, due to the density of the matrix, as opposed to its sparsity in LSA).1
Flickr Distributional Tagspace is based on a unique, but intrinsically variegated, source of semantic information: Flickr tags. This source of data contains spontaneous annotations of personal pictures, which are not suggested by the Flickr platform. The information encoded in Flickr tags has been classified by Beaudoin (2007) in 18 post hoc created categories, which include syntactic property types (e.g., adjectives, verbs), semantic classes (human participants, living things other than humans, non-living things), places, events/activities (e.g., wedding, Christmas, holidays), ad hoc created categories (such as photographic vocabulary, e.g., macro, Nikon), emotions, formal classifications such as terms written in any language other than English, and compound terms written as one word (e.g., mydog). Of all the 18 types of tags identified, Beaudoin reports that the most frequent are (i) geographical locations, (ii) compounds, (iii) inanimate objects, (iv) participants, and (v) events. Moreover, the reasons that push Flickr users to tag their own images have been classified in different ways, the most popular being a macro-distinction between categorizers (users who employ shared high-level features for later browsing) and describers (users who accurately and precisely describe resources for later searching) (Körner et al., 2010). Given the variability of tag types (which include both linguistic and perceptual information) and the variability of motivations that drive taggers’ behaviors, it has been claimed and shown that FDT can model semantic representations of perceptually derived information better than traditional text-based distributional models (Bolognesi, 2014). In the abovementioned study, it has been shown that FDT can cluster color terms (denoting primary and secondary colors, expressed in English language) according to their perceptual similarity, thus reproducing the order in which such colors appear in the rainbow. Instead, two language-based distributional methods (LSA, Landauer and Dumais, 1997, and DM, Baroni and Lenci, 2010) generate a different semantic space. Moreover, in Bolognesi (2016), it has been shown that FDT can categorize concepts into semantically coherent groups in accordance to feature norms for concrete living and non-living things (McRae et al., 2005) better than WordNet (Bolognesi, 2016).
As suggested by Beaudoin (2007) as well as by Bolognesi (2016), Flickr tags encode primarily semantic information about the situational properties of a given concept. In fact, given a tag X, the tags that typically appear together with X across Flickr pictures express on average locations and related entities that can be found in the same context (i.e., within the same picture). It follows that the distributional similarity between a tag X and a tag Y is computed on the basis of the relational properties of X and Y. As suggested by Bowdle and Gentner, metaphor similarity is often relational, in that is generated by aligning relational properties (and situational objects) of base and target, regardless of whether base and target are intrinsically similar. As the authors suggest, “people’s interpretations of metaphors tend to include more relations than simple attributes” (Bowdle and Gentner, 2005, p. 197). The centrality of relational predicates in metaphor comprehension described by Bowdle and Gentner is therefore arguably well modeled by FDT.
Using FDT, a sample of linguistic metaphors (identified through the MIPVU procedure, Steen et al., 2010) and a sample of visual metaphors (identified through the VISMIP procedure, Šorm and Steen, under review2) are contrasted in terms of the amount of knowledge that the metaphorical terms share. This similarity is also compared to the similarity between randomly paired concepts, to see whether there is a significant difference.
Materials
The sets of visual and linguistic metaphors for the distributional analyses were randomly selected from the VU Amsterdam Metaphor Corpus (linguistic metaphors) and the VisMet Corpus (visual metaphors), which are both open access digital resources accessible through the Metaphor Lab Amsterdam institutional website. These corpora are balanced and are representative of the two modalities, and therefore, they have modality-specific inherent variability.
A sample of 50 visual metaphors and a sample of 50 linguistic metaphors were randomly selected. However, as often happens when dealing with real-world data [see the discussion in Goodall et al. (2013)], in order to be suitable for the present investigation, the metaphors had to meet a number of criteria, described below:
1. The selected metaphors had to be taken from different genres. For linguistic metaphors, these are academic discourse, conversations, fiction, and news, while for visual metaphors, they are advertisements and social campaigns, illustrations, political cartoons, and photographs.
2. Different types of realization had to be taken into account, when possible. For linguistic metaphors, indirect metaphors were mainly taken into account [i.e., metaphors in which there is a contrast as well as comparison between the contextual and a more basic meaning, see Steen et al. (2010)], because of their significantly higher frequency in language use, compared to direct metaphors (see reference above). For visual metaphors, the different types in which base and target can be visually cued, according to established models, were taken into account (Forceville, 1996; Phillips and McQuarrie, 2004).
In addition to these criteria, only the visual metaphors without linguistic anchors were taken into account, which means that those images in which linguistic clues constituted a meaningful part of the metaphor and helped with its interpretation, were dropped, so that only strictly visual metaphors were included. To achieve this, those visual metaphors that were annotated in the VisMet corpus as including a metaphoric linguistic expression were dropped first,3 and subsequently, the visual metaphors were manipulated, so that all the linguistic clues presented in the images were covered. The images were then shown to three participants, who had to interpret the meaning of the image in an informal, think-aloud investigation, without relying on the linguistic information conveyed by the linguistic anchors (which were graphically blurred). Images where at least one participant could not understand the metaphor because the linguistic anchors were covered were dropped and then replaced, until the total number of 50 visual metaphors was reached.
Procedure
Identifying the Metaphor Terms
The metaphorical terms that are compared in these metaphors were identified through specific procedures that have been proposed in the field of metaphor studies. For linguistic metaphors, the MIPVU procedure was applied (Steen et al., 2010). This procedure relies on the idea that the majority of metaphors found in language are not direct comparisons expressed through words (such as, for example, “my lawyer is a shark”) but are instead words used in a metaphorical way in a given context. In this sense, the majority of linguistic metaphors are expressed indirectly, and they rely on a contrast between the contextual meaning of the word (which is metaphorical) and its basic meaning (which is literal). According to this procedure, given a text with a potentially metaphorical word, the contextual meaning and the basic meaning of that word express the contrast from which the metaphor is created. For example, in the sentence “I see what you mean,” the contextual meaning of see is understand, while the basic meaning refers to the physical ability of sight. The two meanings (understanding and physically seeing) are contrastive, and therefore, the word see is to be considered metaphorical in the linguistic context “I see what you mean.”
For the identification of the metaphor terms involved in visual metaphors, the VISMIP procedure was applied (See footnote text 2). This procedure relies on the idea that images displaying visual metaphors typically present (different types of) perceptually incongruous elements that violate an expected (or “literal”) scenario. Such incongruities need to be mentally replaced with other elements, whose function it is to restore the visual feasibility (i.e., perceptual congruency) of the scenario. Detecting the incongruous elements (step 3 of the VISMIP procedure) and replacing them with elements that would help restore the expected scenario (step 4) are cognitive operations required to unravel and interpret the metaphor. In this sense, the perceptual incongruities and their replacements constitute the metaphor terms, or part of them. For example, Figure 1 shows an advertisement for a car tire where the creative agent aims to emphasize the gripping power of the product by showing an octopus tentacle fused together with the tire. When the consumer sees this advertisement, she has to mentally replace the tire with the actual tentacle to restore the expected scenario and to identify the two terms of the metaphor: the tentacle and the tire. In fact, the gripping power of the suction cups on the tentacle is a positive characteristic that has to be transferred to the tire itself. The verbal slogan helps to convey the message (“Toyo: gripping performance”), which is nonetheless quite clear by simply looking at the image and being aware of its genre (advertising).
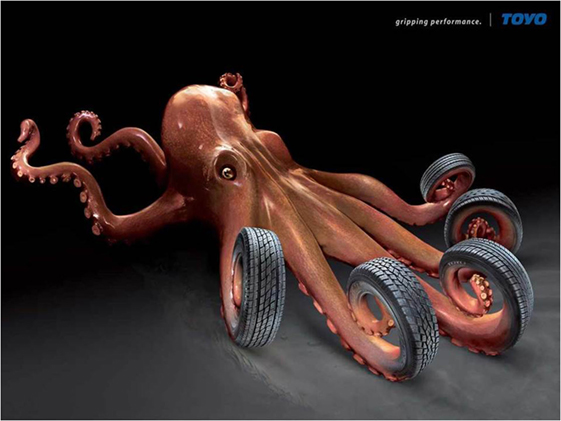
Figure 1. Advertisement for a car tire brand, showing an octopus tentacle fused with a tire. Image used with permission, Copyright owner: GMASCO creative agency.
To summarize the identification procedures, it can be argued that both MIPVU and VISMIP seem to rely on a paradigmatic relation between two entities that constitute the two terms of the metaphor: the contextual meaning of a word vs. its basic meaning in linguistic metaphors and the perceptual incongruous element vs. its replacement in visual metaphors.4
Once the two procedures were applied to (respectively) texts and images, a list of 100 metaphorical correspondences expressed in A-is-B form was obtained (reported in Table 1).
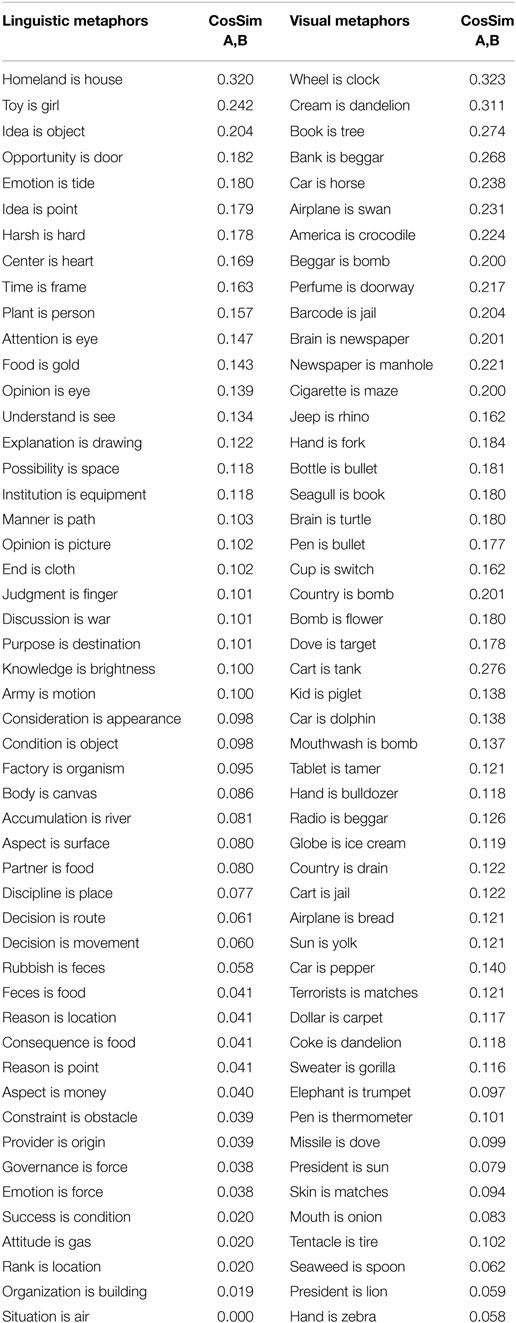
Table 1. Cosine values accounting for the relational similarity between metaphor terms (extracted through FDT) in visual and linguistic metaphors.
Setting Up the Distributional Semantic Space with FDT
In accordance with the FDT method [described in Bolognesi (2014, 2016)], for each of the metaphorical terms (which will be called A and B, as in the typical metaphor formalization A-is-B) that appeared in the visual and in the linguistic metaphors, roughly 100,000 tagsets were downloaded from Flickr through the API flickr.photos.search. Following the FDT procedure, the corpus of concatenated tagsets was then subsetted and filtered, so that each tagset (i.e., each list of tags associated with one picture by one user) was unique, and displayed 15 tags, with A or B appearing among the first 3 tags (so that the tagset could be considered a meaningful context for that concept).
Consequently, the contingency matrix was created, featuring all A and B concepts on the rows and all the other tags appearing in the corpus on the columns.5 The raw frequencies of co-occurrence between each A or B and each co-tag were then turned into measures of association. As described in Bolognesi (2014), the measure of association chosen for FDT is the square pointwise mutual information, defined as follows:
where w1 and w2 are two words (or tags), and f is the frequency of their co-occurrence or individual occurrence in a corpus of N words (or tags). For the purpose of this analysis, w1 was a metaphor term (i.e., A or B), while w2 was the co-tags appearing with w1 across Flickr tagsets.
Given the high level of noise in the data, due to the fact that Flickr tags are freely generated and not based on a dictionary, a list of stop words was compiled and the corpus of tags was filtered with this list. Words included in the stop list included non-words and spelling mistakes (such as “happpines” or “firstdayofschool”), meta-tags (camera brands such as “Nikon,” or technical terms about photography techniques such as “macro”), words expressed in languages other than English, and dates.6 The co-tags (w2) of each concept (w1, i.e., A or B) were then sorted in decreasing order according to their SPMI value, and the top 50 tags for each concept were considered for the cosine analysis. After these filtering operations, the final contingency matrix contained 161 rows (displaying the 161 unique words for A or B) and 2309 columns (displaying the unique co-tags that appeared with any A or B in the initial corpus).
The similarities between each pair of concepts (w1) were then computed in terms of cosines between concept vectors by means of the following established formula:
where a and b are two compared concept vectors, each with n dimensions.
Table 1 reports the cosine measures for the A-is-B pairs of visual and linguistic metaphors, extracted from the 161-by-161 table of cosines computed through FDT.7
Analysis
CosSim in Metaphors vs. Randomly Paired Concepts
The average cosine between two concepts aligned in a metaphorical comparison is CosSim = 0.130 (SD = 0.070). In order to evaluate the significance of this value, it was compared to the average cosine between randomly associated concept pairs. To achieve this, a sample of one million images featuring the neutral tags Nikon or Canon was downloaded from Flickr, and the cosines between each two tags appearing in the sample was computed, following the FDT method. The average cosine between the randomly associated tag pairs extracted from the sample is CosSim = 0.094 (SD = 0.089). The difference between the average cosine between two metaphor terms and the average cosine between two randomly aligned terms by conventional criteria is considered to be statistically significant (t = 3.593, p < 0.001). This suggests that the similarity between two terms of a metaphor is, in general, captured by the distributional analyses of tags across Flickr, performed through FDT, because, on average, the cosine between two concepts aligned in a metaphor does differ substantially from the similarity between two randomly associated concepts.
CosSim in Visual vs. Linguistic Metaphor
As a further analysis, the modality of expression was taken into account. The average cosine between two terms aligned in a visual metaphor was compared to the average cosine of two terms aligned in a linguistic metaphor, and both those values were compared to the average cosine between two randomly paired concepts. The results of the analyses are reported in Table 2. As the table shows, the average similarity between two concepts aligned in a visual metaphor is significantly higher than the average similarity between two randomly paired concepts, as well as between two concepts that are paired in a linguistic metaphor. On the other hand, the average similarity between two concepts aligned in a linguistic metaphor does not substantially differ from the average similarity between two randomly paired concepts.
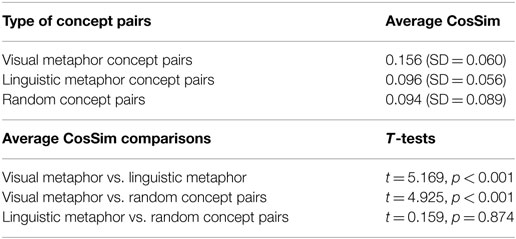
Table 2. Average cosine values for different types of A,B concept pairs: visual metaphor terms, linguistic metaphor terms, and randomly selected pairs, and statistical significance of the difference between average cosines values in different types of concept pairs.
Content Analysis
Semantic Information Encoded in the Dataset
In order to analyze what type of semantic information conveyed by Flickr tags accounts for the similarity between metaphor terms of visual and linguistic metaphors, a content analysis was performed. The 50 tags constituting each concept vector were manually annotated, using the coding scheme related to the knowledge-based taxonomy implemented by Wu and Barsalou (2009) and adapted by Bolognesi et al. (under review)8 to accommodate the annotation of features related to abstract concepts as well as to concrete ones. The coding scheme encompasses 4 macro-categories and 20 nested categories. The four macro-categories refer to properties of the entity (such as entity components: <airplane-wing>, perceptual features: <swan-white>, etc.); properties of the situation in which the concept typically appears (such as participants in the situation: <restaurant-waitress>, locations: <lion-Africa>, etc.); taxonomic properties (such as hypernyms: <octopus-animal>, coordinates: <knife-fork>, etc.); and introspections (such as emotions: <war-sadness>, contingencies: <winter-flu>, etc.). The nested categories, as shown in the examples mentioned above, provide a deeper classification of the 4 macro-categories into 20 nested categories.9 A sample of 1250 w1–w2 pairs, covering 15.3% of the whole dataset, was manually annotated by two independent coders. Given the good interrater agreement achieved by the two independent coders (k = 0.74), one coder then finalized the task.10
The types of w1–w2 semantic relations encoded in Flickr tags, for the concepts analyzed, are summarized in Figure 2 (macro types) and Figure 3 (nested categories).
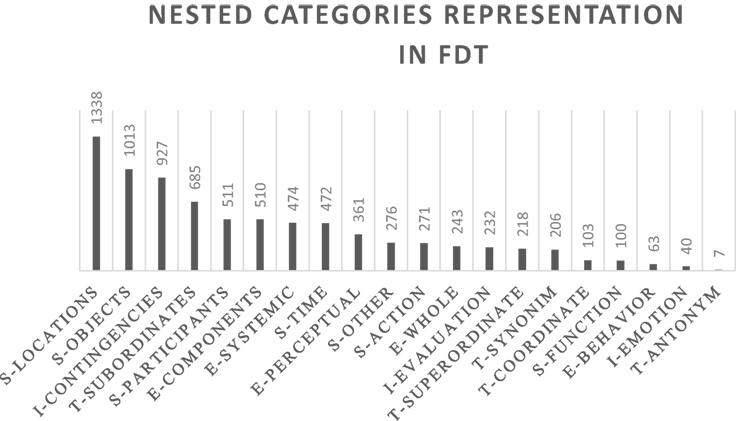
Figure 3. Nested categories representation in FDT. Numbers correspond to the frequency of the type of relationship.
As the graphs show, the semantic relations between tag pairs mainly encode properties of the situation in which a given concept appears and, in particular, identify locations and contextual objects that can typically be found in a situation where the target concept appears. This confirms the findings of Beaudoin (2007) and Bolognesi (2016), who showed that locations and contextual entities are the most frequent categories represented in Flickr tags.
Semantic Information in Relation to the Metaphor Type (Visual or Linguistic)
As the graph in Figure 4 shows, the related tags retrieved in FDT about the concepts involved in visual and in linguistic metaphors mainly express situational properties in which such concepts tend to occur. However, the graph also shows that the two modalities behave in slightly different ways for the other macro-categories, in that concepts involved in linguistic metaphors tend to trigger more introspections and taxonomic features, while concepts involved in visual metaphors tend to trigger more entity-related properties. A chi-square analysis confirms the significance of the different distributions (Pearson chi-square = 127.878; df = 3; p < 0.001). In Table 3, the analysis of the standardized residuals shows that the observed situation properties and entity-related properties are significantly higher than expected for concepts involved in visual metaphors (i.e., adjusted residual >2, as highlighted in the table) and that taxonomic features and introspections are significantly higher than expected for concepts involved in linguistic metaphors.
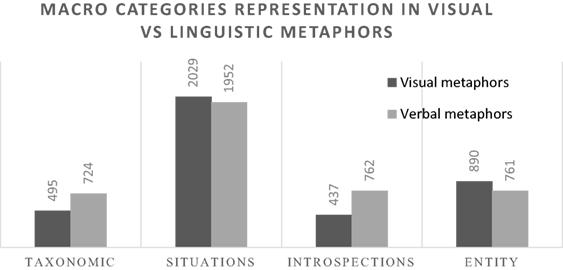
Figure 4. Macro-category distribution for visual vs. linguistic metaphor terms. Numbers correspond to the frequency of the type of relationship.
A closer analysis of the nested category distribution in visual vs. linguistic metaphors (Figure 5) shows that the two modalities of expression involve concepts for which the salient semantic information retrieved through FDT differs.
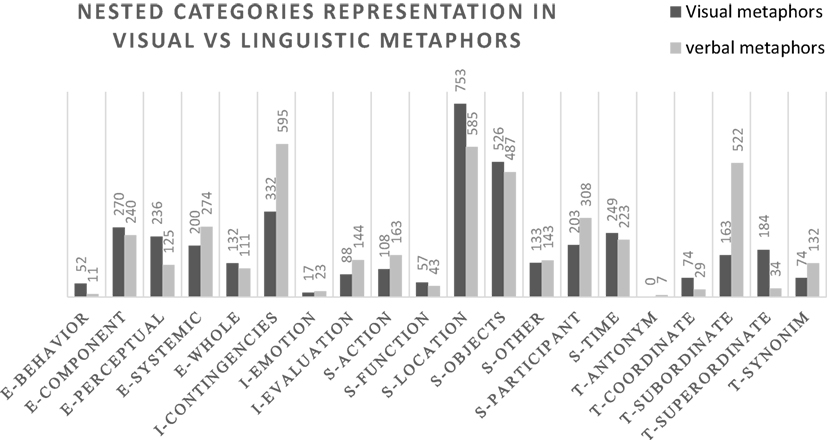
Figure 5. Nested category distribution for visual vs. linguistic metaphor terms. Numbers correspond to the frequency of the type of relationship.
A chi-square analysis was run on the nested categories data, and the results suggest that the nested category distributions of visual vs. linguistic metaphors differ (chi-square = 544.388, df = 19, p < 0.001). The table with analysis of the residuals (which is not reported because of the limited space available) shows that at the level of p = 0.05, there are various standardized residuals >2, which indicate the presence of categories for which there is a significant difference between expected and observed counts within each of the two modalities. Table 4 reports a summary of those nested categories that are significantly related to only one of the modalities of expression.
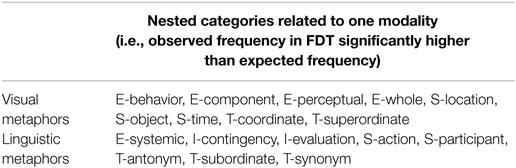
Table 4. Nested categories for which the adjusted residual value in the chi-square analysis exceeds the value 2, i.e., the category is significantly related to one specific modality.
Semantic Information Encoded in the Shared Features
The types of shared features between metaphor terms, which account for the relational similarity between the two concepts, retrieved by FDT, are summarized in Figure 6 (macro-categories) and Figure 7 (nested categories). As the figures show, these are mainly situational properties, followed by entity-related properties, introspections, and taxonomic features. More specifically, looking at the nested level, it can be observed in Figure 7 that the shared features between two metaphor terms retrieved by FDT primarily express locations and related objects within the situational properties, perceptual features within the entity-related properties, subordinates within the taxonomic features, and contingencies within the introspections macro-category.
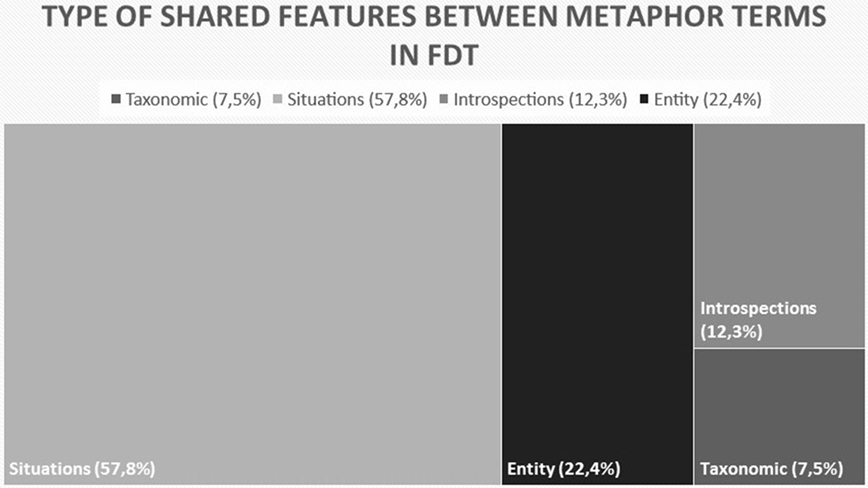
Figure 6. Types of shared features between metaphor terms retrieved by FDT (macro-categories). Numbers correspond to the frequency of the type of relationship.
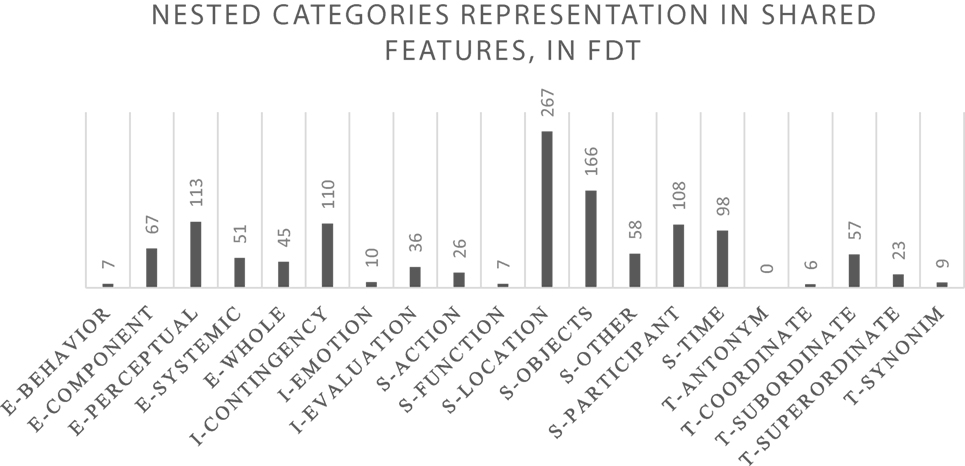
Figure 7. Types of shared features between metaphor terms retrieved by FDT (nested categories). Numbers correspond to the frequency of the type of relationship.
Semantic Information Encoded in the Shared Features in Relation to Metaphor Type (Visual or Linguistic)
The analysis of the distribution of shared feature types in relation to the modality of expression (visual or linguistic) is visualized in Figure 8 (macro level). A chi-square analysis confirms the significance of the different distributions (Pearson chi-square = 35.865; df = 3; p < 0.01), and the analysis of the residuals (Table 5) suggests that taxonomic features and introspections are significantly higher than expected in linguistic metaphors, while entity-properties are significantly higher than expected in visual metaphors (as indicated by the adjusted residuals values >2, highlighted in the table).
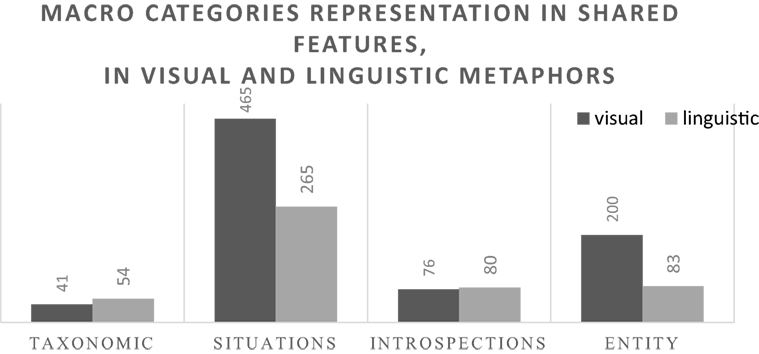
Figure 8. Types of shared features between metaphor terms in visual vs. linguistic metaphors (macro categories). Numbers correspond to the frequency of the type of relationship.
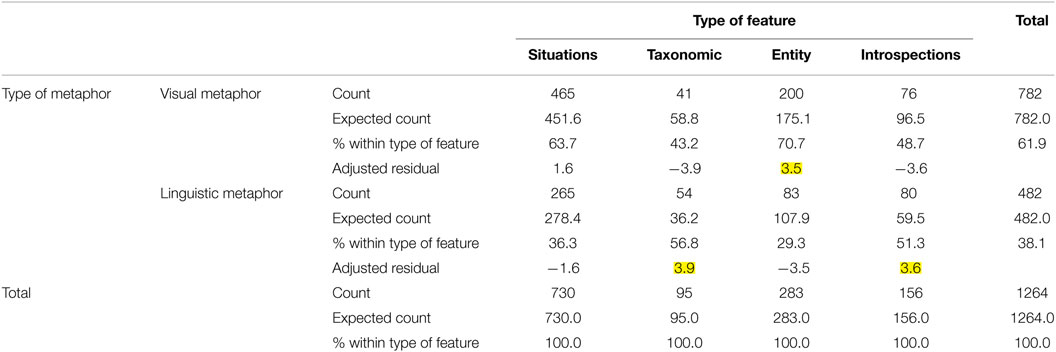
Table 5. Type of metaphor × type of feature cross-tabulation for shared features categorized at the macro level.
Finally, Figure 9 shows the distributions of feature types at the nested category level in the two different modalities. A chi-square analysis at the nested category level shows that the shared features between concepts aligned in visual metaphors and the shared features between concepts aligned in linguistic metaphors are significantly different (chi-square = 128.056, df = 18, p < 0.001). The analysis of the residuals is summarized in Table 6, where the categories related significantly to each modality are reported.
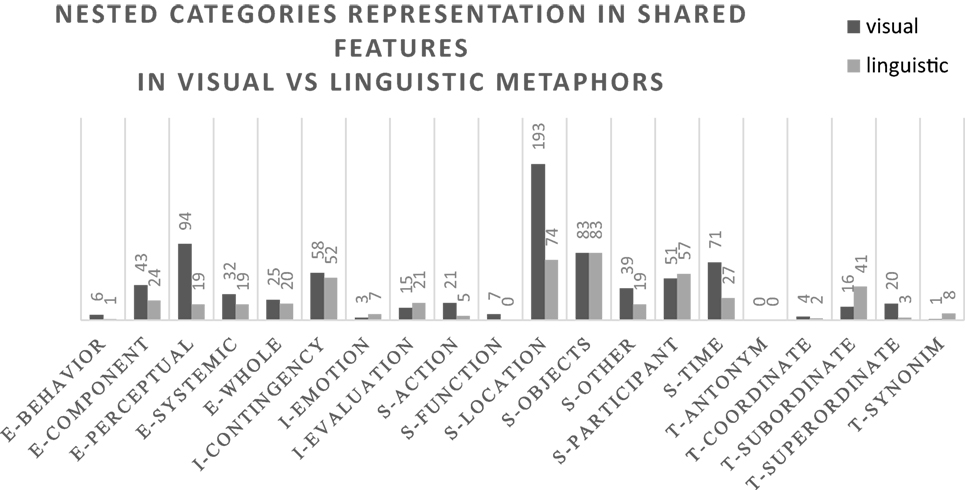
Figure 9. Types of shared features between metaphor terms in visual vs. linguistic metaphors (nested categories). Numbers correspond to the frequency of the type of relationship.
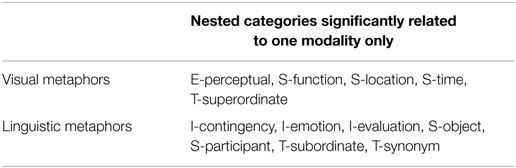
Table 6. Nested categories for shared features, for which the adjusted residual value in the chi-square analysis exceeds the value 2, i.e., the category is significantly related to one specific modality.
Discussion
The first research question that the present study aimed to answer was whether the latent similarity between two metaphor terms could be modeled through FDT, a distributional method that encodes different types of semantic information. In Section “CosSim in Metaphors vs. Randomly Paired Concepts,” it was shown that the cosine similarity between two metaphor terms obtained through FDT is significantly higher than the cosine similarity between two randomly paired concepts. This suggests that FDT does indeed capture a type of distributional similarity (based on relational properties) that is meaningful for metaphorical comparisons.
The second research question that the present study aimed to explore was whether FDT could model the similarity between metaphor terms in visual and in linguistic metaphors equally well. This question relates to the problem that the current scientific literature about metaphor is (still) strongly biased toward the analysis of the linguistic modality of expression. However, a comprehensive theory of metaphor that claims that metaphors relate to the conceptual dimension of meaning cannot be afford to be biased toward the study of one specific modality. Moreover, the studies focused on visual metaphor do not approach the analysis of this modality in a comparative and contrastive fashion with other modalities, while the current approach allows to explore how, respectively, words and images construct and represent metaphor. In Section “CosSim in Visual vs. Linguistic Metaphor,” it was found that while the average similarity between two concepts aligned in a visual metaphor is significantly different from the similarity between two randomly paired concepts, the similarity between two concepts aligned in a linguistic metaphor does not differ from the similarity between two randomly paired concepts. This suggests that the similarity between concepts aligned in linguistic metaphors is not captured well by FDT.
The third research question aimed at exploring the type of semantic information captured by FDT and how this distribution relates to the metaphor terms encompassed, respectively, in visual and linguistic metaphors. In Section “Content Analysis,” I reported the outcomes of a content analysis aimed at classifying, for each analyzed concept, the type of semantic information encoded in its related tags, retrieved by FDT. The analyses, based on an established knowledge-based taxonomy of semantic relation types between concept–feature pairs (Wu and Barsalou, 2009), led to the following observations:
(1) FDT primarily encodes situation-based relations (<seagull-beach>, or <judgment-court>), followed by entity-related properties (<seagull-wings>, or <location-public>), taxonomic properties (<lion-animal>, or <location-California>), and introspections (<war-sad>, <flower-beautiful>).
(2) Comparing the two modalities of expression, it appears that the concepts involved in linguistic metaphors, in relation to those involved in visual metaphors, typically involve more taxonomic features and introspections than normally expected, while the concepts involved in visual metaphors typically involve more situational properties and entity-related properties than expected.
(3) The shared features between two metaphor terms, which account for the similarity between them, primarily express situation-related properties, followed by entity-related properties, introspections, and taxonomic features.
(4) Looking at the shared features between metaphor terms and comparing the two modalities of expression (visual and linguistic), it appears once again that the shared features between metaphor terms in linguistic metaphors involve more taxonomic features and introspections than expected, while the shared features between metaphor terms in visual metaphors involve more situational properties and entity-related properties than expected.
Overall, the results suggest that FDT primarily captures relational properties (situation-based features), followed by attributional properties (entity-related features) and that it can thus successfully model the distributional similarity based on relational and (partially) attributional properties. This type of similarity seems to play a crucial role in visual metaphors but not so much in linguistic metaphors. The latter type of metaphor, therefore, seems to work in a dimension of meaning that is not captured well by FDT. As a matter of fact, the features that are shared by metaphor terms in linguistic metaphors (and which therefore account for the similarity between the two compared concepts) primarily express taxonomic features such as subordinates and synonyms, as well as introspections such as contingencies and emotions. Such features are not particularly well represented in FDT, compared to situational properties and entity-related features. Thus, the similarity between metaphor terms in linguistic metaphors is not high, when modeled through FDT. In a parallel study (embedded in the COGVIM project, as explained in Section “Introduction”), metaphor similarity has been investigated by means of a distributional method trained on linguistic corpora (distributional memory, Baroni and Lenci, 2010). As we predicted, this method captures higher similarity scores for the terms aligned in linguistic metaphors, compared to those aligned in visual metaphors (Bolognesi and Aina, under review11). This, we argue, is due to the fact that taxonomic and introspective information are well represented in the linguistic corpus. The difference in the patterns of similarity captured by FDT and Distributional Memory provides additional evidence to support the claim that the two modalities (images and words) construct and represent metaphor in significantly different ways.
The final remark regarding the present study: the reader might argue that there is a substantial difference in the nature of the visual stimuli, as opposed to the linguistic stimuli, which lies in the fact that the concepts involved as metaphor terms in visual metaphors are typically more concrete than those involved as metaphor terms in linguistic metaphors. This is due to the fact that the visual modality of expression requires the metaphor terms to be represented or cued by graphic means, while the verbal modality allows the direct expression of abstract concepts through dedicated words. From this perspective, the degree of concreteness of the concepts involved as metaphor terms in the two modalities can also be considered a variable that might influence the results reported here. A formal investigation, which is currently in progress, will explain how and to what extent visual and verbal metaphors differ in terms of concreteness, as well as modeling the cognitive operations that explain how abstract concepts emerge from metaphorical images. The present study and the distributional analyses currently in progress based on text corpora precisely suggest that language and images are two different modes of communication that classify knowledge and represent meaning in different ways. Because of their inner characteristics, the two modes have their inner strengths (i.e., they can easily express some types of knowledge) and inner weaknesses (they need to use indirect ways to express other types of knowledge). Such characteristics are reflected in the type of knowledge that is typically shared by two concepts compared in visual vs. linguistic metaphors, despite the fact that metaphors are matters of thought. A comprehensive theory of metaphor, not biased toward analyses of linguistic expressions, cannot avoid the differences between these two communication modes.
Conclusion
The results reported in this study show how FDT, a distributional method based on a stream of Big Data (Flickr tags), can successfully model the distributional similarity between metaphor terms and provide insightful distinctions between the degrees of relational similarity that characterize two different metaphor modalities (visual vs. linguistic).
As explained in Section “Method,” the investigation is based on a sample of visual metaphors and a sample of linguistic metaphors, randomly extracted from modality-specific corpora, and analyzed through established procedures for metaphor identification. The samples analyzed here are therefore considered to be representative for their respective modalities of expression. Further investigations currently in progress within the COGVIM project12 will provide additional insight into the peculiarities of and differences between these two modalities of expression, and how such characteristics affect metaphor construction and comprehension.
Author Contributions
MB, the first and unique author of the present contribution, has conceptualized and designed the present study, drafted the article, and submitted it.
Conflict of Interest Statement
The author declares that the research was conducted in the absence of any commercial or financial relationships that could be construed as a potential conflict of interest.
Acknowledgments
The author is extremely grateful to Professor Tony Veale for his comments on a previous draft of the article.
Funding
This study is sponsored by an EU Marie Curie Intra European Fellowship, awarded to Dr. MB (COGVIM no. 629076 – Project Acronym: COGVIM; Call identifier FP7-PEOPLE-2013-IEF).
Footnotes
- ^In the original description of FDT (Bolognesi, 2014, 2016), there is no dimensionality reduction on the matrices. However, for the present investigation, given the size of the contingency matrix, only the 50 tags with the highest measures of association for each concept were included in the contingency table, on which the cosines were then computed. See the description in Section “Setting up the Distributional Semantic Space with FDT.”
- ^Šorm, E., and Steen, G. (under review). “VISMIP: towards a method for visual metaphor identification,” in Visual Metaphor: How Images Construct Metaphorical Meaning, ed. G. Steen (Amsterdam: John Benjamins Publishing Company).
- ^See documentation on the Vismet corpus website for more information: http://www.vismet.org/VisMet/annotation.php.
- ^In visual metaphors, there are other types of realizations, such as similes (or alignments), as suggested in Forceville (1996) and Phillips and McQuarrie (2004). Here, both base and target are fully represented. This type of realization is comparable to that of direct metaphors in language (explicitly expressed in A-is-B form). Direct realizations are quite infrequent in both language and images, compared to indirect expressions.
- ^The total number of unique concepts is 161, because some concepts appear as base or as target domains more than once in different metaphors.
- ^The full stop-word list will be released together with the supplementary materials used for the analysis on the COGVIM website.
- ^The 161-by-161 table of cosines will be released on the COGVIM project website.
- ^Bolognesi, M., Pilgram, R., and Van den Heerik, R. (under review). Reliability in Content Analysis. The Case of Semantic Feature Norms Classification.
- ^The adapted taxonomy and its related coding scheme are available on the COGVIM project website: https://cogvim.files.wordpress.com/2016/04/coding-scheme-cogvim.pdf.
- ^The annotated pairs will be released on the COGVIM project website.
- ^Bolognesi, M., and Aina, L. (under review). Similarity is Closeness: Using Distributional Semantic Spaces to Model Similarity in Visual and Linguistic Metaphors.
- ^https://cogvim.org/
References
Agres, K. R., McGregor, S., Rataj, K., Purver, M., and Wiggins, G. A. (2016). “Modeling metaphor perception with distributional semantics vector space models,” in Workshop on Computational Creativity, Concept Invention, and General Intelligence. Proceedings of 5th International Workshop, C3GI at ESSLI, 1–14.
Baroni, M., and Lenci, A. (2010). Distributional memory: a general framework for corpus-based semantics. Comput. Linguist. 36, 673–721. doi:10.1162/coli_a_00016
Beaudoin, J. (2007). Flickr image tagging: patterns made visible. Bull. Am. Soc. Inform. Sci. Technol. 34, 26–29. doi:10.1002/bult.2007.1720340108
Binder, J. R., Desai, R. H., Graves, W. W., and Conant, L. L. (2009). Where is the semantic system? A critical review and meta-analysis of 120 functional neuroimaging studies. Cereb. Cortex 19, 2767–2796. doi:10.1093/cercor/bhp055
Black, M. (1979). “More about metaphor,” in Metaphor and Thought, ed. A. Ortony (Cambridge: University Press), 19–43.
Bolognesi, M. (2014). “Distributional semantics meets embodied cognition: Flickr as a database of semantic features,” in Selected Papers from the 4th UK Cognitive Linguistics Conference (London, UK).
Bolognesi, M. (2016). “Flickr Distributional Tagspace: evaluating the semantic spaces emerging from Flickr tags distributions,” in Big Data in Cognitive Science, ed. M. Jones (New York: Routledge).
Bowdle, B., and Gentner, D. (2005). The career of metaphor. Psychol. Rev. 112, 193–216. doi:10.1037/0033-295X.112.1.193
Bright, P., Moss, H., and Tyler, L. (2004). Unitary vs. multiple semantics: PET studies of word and picture processing. Brain Lang. 89, 417–432. doi:10.1016/j.bandl.2004.01.010
Chee, M. W., Weekes, B., Lee, K., Soon, C., Schreiber, A., Hoon, I., et al. (2000). Overlap and dissociation of semantic processing of Chinese characters, English words, and pictures: evidence from fMRI. Neuroimage 12, 392–403. doi:10.1006/nimg.2000.0631
Davidoff, J., and De Bleser, R. (1994). Impaired picture recognition with preserved object naming and reading. Brain Cogn. 24, 1–23. doi:10.1006/brcg.1994.1001
Farah, M. (1990). Visual Agnosia. Disorders of Object Recognition and What They Tell Us about Normal Vision. Cambridge: MIT Press.
Forceville, C. (2011). “The Journey metaphor and the Source-Path-Goal schema in Agnès Varda’s autobiographical gleaning documentaries,” in Beyond Cognitive Metaphor Theory: Perspectives on Literary Metaphor, ed. M. Fludernik (London: Routledge), 281–297.
Gates, L., and Yoon, M. (2005). Distinct and shared cortical regions of the human brain activated by pictorial depictions versus verbal descriptions: an fMRI study. Neuroimage 24, 473–486. doi:10.1016/j.neuroimage.2004.08.020
Giora, R. (2008). “Is metaphor unique?” in The Cambridge Handbook of Metaphor and Thought, ed. R. W. Gibbs Jr. (Cambridge, UK: University Press), 143–160.
Goodall, C., Slater, M., and Myers, T. (2013). Fear and anger responses to local news coverage of alcohol-related crimes, accidents, and injuries: explaining news effects on policy support using a representative sample of messages and people. J. Commun. 63, 373–392. doi:10.1111/jcom.12020
Gorno-Tempini, M., Price, C., Josephs, O., Vandenberghe, R., Cappa, S., Kapur, N., et al. (1998). The neural systems sustaining face and proper-name processing. Brain 121, 2103–2118. doi:10.1093/brain/121.11.2103
Gutiérrez, E. D., Shutova, E., Marghetis, T., and Bergen, B. (2016). “Literal and metaphorical senses in compositional distributional semantic models,” in Proceedings of the 54th Meeting of the Association for Computational Linguistics, 160–170.
Hasson, U., Levy, I., Behrmann, M., Hendler, T., and Malach, R. (2002). Eccentricity bias as an organizing principle for human high-order object areas. Neuron 34, 490–497. doi:10.1016/S0896-6273(02)00662-1
Hidalgo, L., and Kraljevic, B. (2011). “Multimodal metonymy and metaphor as complex discourse resources for creativity in ICT advertising discourse,” in Metaphor and Metonymy Revisited beyond the Contemporary Theory of Metaphor, eds F. Gonzálvez García, S. Peña, and L. Pérez-Hernández (Amsterdam/Philadelphia: John Benjamins), 153–178.
Kintsch, W., and Bowles, A. R. (2002). Metaphor comprehension: what makes a metaphor difficult to understand? Metaphor Symb. 17, 249–262. doi:10.1207/S15327868MS1704_1
Körner, C., Benz, D., Strohmaier, M., Hotho, A., and Stumme, G. (2010). “Stop thinking, start tagging – tag semantics emerge from collaborative verbosity,” in Proceedings of the 19th International World Wide Web Conference (WWW 2010) (Raleigh, NC).
Lakoff, G. (1993). “The contemporary theory of metaphor,” in Metaphor and Thought, ed. A. Ortony (Cambridge: Cambridge University Press), 202–251.
Landauer, T., and Dumais, S. (1997). A solution to Plato’s problem: the latent semantic analysis theory of the acquisition, induction and representation of knowledge. Psychol. Rev. 104, 211–240. doi:10.1037/0033-295X.104.2.211
McRae, K., Cree, G. S., Seidenberg, M. S., and McNorgan, C. (2005). Semantic feature production norms for a large set of living and nonliving things. Behav. Res. Methods 37, 547–559. doi:10.3758/BF03192726
Moore, C., and Price, C. (1999). Three distinct posterior basal temporal lobe regions for reading and object naming. Neuroimage 10, 181–192. doi:10.1006/nimg.1999.0450
Ning, Y. (2011). A decompositional approach to metaphorical compound analysis: the case of a TV commercial. Metaphor Symb. 26, 243–259. doi:10.1080/10926488.2011.609041
Ortiz, M. (2011). Primary metaphors and monomodal visual metaphors. J. Pragmatics 43, 1568–1580. doi:10.1016/j.pragma.2010.12.003
Ortony, A. (1979). Beyond literal similarity. Psychol. Rev. 86, 161–180. doi:10.1037/0033-295X.86.3.161
Paivio, A. (1986). Mental Representations: A dual Coding Approach. New York: Oxford University Press.
Paivio, A. (2010). Dual coding theory and the mental lexicon. Mental Lexicon 5, 205–230. doi:10.1075/ml.5.2.04pai
Phillips, B., and McQuarrie, E. (2004). Beyond visual metaphor: a new typology of visual rhetoric in advertising. Market. Theory 4, 113–136. doi:10.1177/1470593104044089
Reinholz, J., and Pollmann, S. (2005). Differential activation of object-selective visual areas by passive viewing of pictures and words. Cogn. Brain Res. 24, 702–714. doi:10.1016/j.cogbrainres.2005.04.009
Shutova, E., Kiela, D., and Maillard, J. (2015). “Black holes and white rabbits: metaphor identification with visual features,” in Proceedings of the North American Chapter of the Association for Computational Linguistics (San Diego, CA), 183–193.
Steen, G. (2008). The paradox of metaphor: why we need a three-dimensional model of metaphor. Metaphor Symb. 23, 213–241. doi:10.1080/10926480802426753
Steen, G., Dorst, L., Herrmann, B., Kaal, A., Krennmayr, T., and Pasma, T. (2010). A Method for Linguistic Metaphor Identification: From MIP to MIPVU. Amsterdam: John Benjamins Publishing Company.
Turner, M., and Fauconnier, G. (2002). The Way We Think. Conceptual Blending and the Mind’s Hidden Complexities. New York: Basic Books.
Utsumi, A. (2011). Computational exploration of metaphor comprehension processes using a semantic space model. Cogn. Sci. 35, 251–296. doi:10.1111/j.1551-6709.2010.01144.x
Veale, T., Shutova, E., and Klebanov, B. B. (2016). Metaphor: A Computational Perspective. Synthesis Lectures on Human Language Technologies. Morgan and Claypool Publishers.
Warrington, E. (1985). “Agnosia: the impairment of object recognition,” in Handbook of Clinical Neurology, ed. J. Frederiks (New York: Elsevier), 333–349.
Keywords: metaphor, semantic similarity, distributional semantics, visual metaphors, linguistic metaphors, Big Data, Flickr
Citation: Bolognesi M (2016) Modeling Semantic Similarity between Metaphor Terms of Visual vs. Linguistic Metaphors through Flickr Tag Distributions. Front. Commun. 1:9. doi: 10.3389/fcomm.2016.00009
Received: 29 June 2016; Accepted: 27 September 2016;
Published: 17 October 2016
Edited by:
Sidarta Ribeiro, Federal University of Rio Grande do Norte, BrazilReviewed by:
Juan Carlos Valle-Lisboa, Universidad de la Republica, UruguayElkin Dario Gutierrez, IBM Research, USA
Copyright: © 2016 Bolognesi. This is an open-access article distributed under the terms of the Creative Commons Attribution License (CC BY). The use, distribution or reproduction in other forums is permitted, provided the original author(s) or licensor are credited and that the original publication in this journal is cited, in accordance with accepted academic practice. No use, distribution or reproduction is permitted which does not comply with these terms.
*Correspondence: Marianna Bolognesi, TS5NLkJvbG9nbmVzaTJAdXZhLm5s